Final Exam Review PDF
Document Details
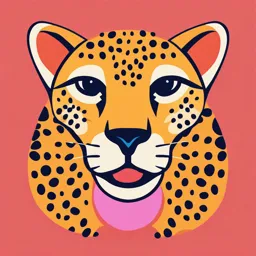
Uploaded by WellBehavedCognition9443
Tags
Summary
This document reviews research paradigms and philosophical perspectives, including positivism and interpretivism. It covers ontological and epistemological aspects, methodologies, and the role of the researcher in different research approaches. The document also touches on pragmatism and steps involved in research, along with operational and conceptual definitions of variables, and various types of hypotheses.
Full Transcript
Final Exam Review Research Paradigms and Philosophical Perspectives: Positivism: 1. Ontology (Nature of Reality): • Assumes an objective and tangible reality that exists independently of individuals. • Reality can be observed, measured, and studied through empirical methods. 2. Epistemology (Nature...
Final Exam Review Research Paradigms and Philosophical Perspectives: Positivism: 1. Ontology (Nature of Reality): • Assumes an objective and tangible reality that exists independently of individuals. • Reality can be observed, measured, and studied through empirical methods. 2. Epistemology (Nature of Knowledge): • Knowledge is discovered through systematic observation and experimentation. • Emphasizes the importance of empirical evidence and the scientific method. 3. Methodology: • Quantitative methods dominate. • Research is often structured, controlled, and replicable. • Objective measurements and statistical analyses are crucial. 4. Role of the Researcher: • A detached observer striving for objectivity. • Strives to eliminate bias and personal values. 5. Purpose of Research: • To discover laws and universal truths. • Emphasis on generalizability and prediction. Interpretivism (Constructivism): 1. Ontology: • Reality is subjective and socially constructed. • Multiple realities exist, shaped by individual experiences and perspectives. 2. Epistemology: • Knowledge is subjective and context-dependent. • Understanding is gained through the interpretation of meanings and social interactions. 3. Methodology: • Qualitative methods are prominent, such as interviews, observations, and content analysis. • Emphasis on understanding context and exploring subjective experiences. 4. Role of the Researcher: • Acknowledges the researcher's influence on the study. Recognizes the importance of the researcher's interpretation and subjectivity. 5. Purpose of Research: • To understand and interpret phenomena from the participants' perspectives. • Emphasis on context, cultural influences, and the uniqueness of each situation. Pragmatism: 1. Ontology: • Reality is both objective and subjective. • The focus is on what works or is practical. 2. Epistemology: • Emphasizes the importance of both quantitative and qualitative methods. • Values the flexibility to choose methods based on the research question. 3. Methodology: • Methodological flexibility based on the research question. • A willingness to use both quantitative and qualitative approaches. 4. Role of the Researcher: • A problem solver who uses the most effective methods for addressing the research question. • Acknowledges the value of diverse perspectives. 5. Purpose of Research: • Pragmatic researchers seek solutions to real-world problems. • Focus on the practical application of research findings. • Steps Involved in Research: • • • • • • • • Identify the problem Conduct a literature review Formulate a hypothesis Design the study Collect data Analyze data Interpret results Communicate findings Operational and Conceptual Definitions of Variables: • Independent Variable: The variable that is manipulated or controlled by the researcher. • Example: Teaching method in an education study. • • • • Dependent Variable: The variable that is observed or measured and is influenced by the independent variable. • Example: Student performance in the same education study. Extraneous Variable: Variables that may affect the dependent variable but are not the main focus of the study. • Example: Prior knowledge, socioeconomic status, or motivation in the education study Conceptual Definition: Provides a theoretical understanding of a variable without specifying how it will be measured or manipulated. • Purpose: Establishes a clear and shared understanding of the key concepts in a study. It is the foundation for developing operational definitions • Example: “Motivation in workplace” Operational Definition: Defines a variable in terms of specific procedures or operations used to measure or manipulate it, translating abstract concepts into observable and measurable terms • Purpose: Provides a concrete and standardized way to assess or manipulate variables, ensuring consistency and replicability in research • Example: "Motivation will be operationalized as the total time spent on voluntary, non-assigned educational activities over the course of a week, measured in hours." Types of hypotheses: 1. Directional Hypothesis: • Definition: A directional hypothesis makes a specific prediction about the direction of the expected relationship between variables. • Example: "An increase in study time will lead to a statistically significant improvement in exam scores." 2. Non-Directional Hypothesis: • Definition: A non-directional hypothesis predicts the existence of a relationship between variables without specifying the direction of that relationship. • Example: "There is a significant relationship between study time and exam scores." 3. Null Hypothesis (H₀): • Definition: The null hypothesis states that there is no significant effect or relationship. It suggests that any observed differences or correlations are due to random chance. • Example: "There is no significant difference in exam scores between students who engage in different study methods." 4. Alternative Hypothesis (H₁ or Ha): • Definition: The alternative hypothesis proposes a specific relationship or effect and is typically what researchers aim to support. • Example: "Students who use a mnemonic study technique will have significantly higher exam scores than those who do not." 5. Complex Hypothesis: • Definition: A complex hypothesis involves the relationship between three or more variables, specifying the nature of the relationship. • Example: "The interaction effect of study time, teaching method, and student motivation will significantly predict academic performance." 6. Statistical Hypothesis: • Definition: In quantitative research, statistical hypotheses involve specific statements about the population parameters. • Example: "The mean difference in exam scores between Group A and Group B is significantly different from zero." 7. Research Hypothesis: • Definition: The research hypothesis is a general statement that predicts a relationship between variables based on existing theories or evidence. • Example: "There is a positive correlation between physical activity and cognitive performance." 8. Associative Hypothesis: • Definition: An associative hypothesis predicts a relationship or association between two variables without implying causation. • Example: "There is a significant association between hours of sleep and stress levels." Research Designs: Experimental Research Designs: 1. Quasi-Experimental Design: • Definition: Quasi-experimental designs resemble experimental designs but lack random assignment of participants to groups. Researchers have some control over the experimental conditions but cannot randomly assign participants. • Key Characteristics: • No random assignment. • Manipulation of independent variables. • May use pre-existing groups or natural variations. • Example: Investigating the impact of a teaching intervention in existing classrooms without randomly assigning students to the intervention. 2. True Experimental Design: • Definition: True experimental designs involve random assignment of participants to different conditions or groups, allowing researchers to make cause-and-effect inferences. • Key Characteristics: • Random assignment. • Manipulation of independent variables. • Control over extraneous variables. • Example: Randomly assigning students to two different teaching methods and comparing their subsequent exam scores. 3. Factorial Designs: • Definition: Factorial designs involve the manipulation of two or more independent variables simultaneously, allowing researchers to study their individual and interactive effects on the dependent variable. • Key Characteristics: • Manipulation of multiple independent variables. • Examination of main effects and interaction effects. • Example: Investigating the impact of both teaching method and student motivation on academic performance. 4. Randomized Control Trials (RCTs): • Definition: Randomized Control Trials are a type of true experimental design where participants are randomly assigned to either an experimental group (receives the treatment) or a control group (receives no treatment or a standard treatment). • Key Characteristics: • Random assignment. • Control group for comparison. • Rigorous experimental design. • Example: Conducting a medical trial where individuals with a certain condition are randomly assigned to receive either a new drug or a placeb Non-experimental Research Designs: 1. Descriptive Studies: • Definition: Descriptive studies aim to describe the characteristics of a phenomenon without manipulating variables. The focus is on providing a detailed account of what is observed. • Key Characteristics: • No manipulation of variables. • Emphasis on describing the present state of affairs. • Common methods include observations, surveys, and content analysis. Example: Conducting a survey to describe the dietary habits of a specific population. 2. Correlational Studies: • Definition: Correlational studies examine the relationship between two or more variables to determine if they are associated. However, correlation does not imply causation. • Key Characteristics: • No manipulation of variables. • Measures the strength and direction of relationships. • Common statistical measure: correlation coefficient. • Example: Investigating the relationship between hours of study and academic performance to determine if they are positively correlated. 3. Retrospective and Prospective Studies: • Retrospective Study: • Definition: A retrospective study looks back in time to examine relationships between variables or to analyze the effects of past events. • Key Characteristics: • Data collection occurs after the events have occurred. • Relies on existing records or participant recall. • Example: Studying the association between smoking habits and the development of lung cancer by examining medical records of individuals diagnosed with lung cancer. • Prospective Study: • Definition: A prospective study follows participants over time, collecting data in the present and future to examine the occurrence of events or changes in variables. • Key Characteristics: • Data collection occurs over an extended period, usually involving follow-ups. • Participants are often selected based on specific characteristics. • Example: Observing a group of individuals over several years to investigate the long-term effects of a specific medication on health outcomes. • Qualitative Designs: 1. Ethnography: • Definition: Ethnography is a qualitative research design that involves in-depth, immersive study and description of a cultural group or community. Researchers often spend extended periods in the field, engaging with participants to gain an insider's perspective. Key Characteristics: • Participant observation is a common method. • Emphasis on understanding the culture from the perspective of the participants. • Rich, detailed descriptions of social practices and behaviors. • Example: Living in a community to study and document the daily lives, rituals, and social interactions of its members. 2. Phenomenology: • Definition: Phenomenology is a qualitative research design focused on exploring and understanding the lived experiences of individuals. Researchers aim to uncover the essence of a phenomenon by examining how individuals perceive and make sense of their experiences. • Key Characteristics: • In-depth interviews and open-ended questioning. • Emphasis on capturing subjective experiences. • Seeks to identify common themes and patterns. • Example: Investigating the essence of the lived experience of patients coping with a chronic illness through in-depth interviews. 3. Grounded Theory: • Definition: Grounded theory is a qualitative research design that involves systematically generating theory from the data. Researchers collect and analyze data concurrently, allowing themes and concepts to emerge organically. • Key Characteristics: • Data collection and analysis occur simultaneously. • Constant comparison of data to generate theoretical insights. • Development of categories and concepts grounded in the data. • Example: Studying the experiences of individuals transitioning to parenthood and developing a theory on the stages and processes involved. 4. History: • Definition: Historical research involves the systematic examination of past events, practices, and contexts. Researchers analyze documents, artifacts, and other sources to reconstruct and interpret historical phenomena. • Key Characteristics: • Relies on historical records and primary sources. • Seeks to understand the context and significance of past events. • Often involves critical analysis and interpretation. • Example: Investigating the social and political factors that led to a specific historical event, using documents, letters, and other archival materials. 5. Action Research: • • • • Definition: Action research is a research design that involves collaboration between researchers and practitioners to address real-world problems. It aims to bring about positive change through a cyclical process of planning, acting, observing, and reflecting. Key Characteristics: • Collaboration between researchers and participants. • Focus on solving practical problems in a specific context. • Iterative cycles of action and reflection. Example: Working with teachers in a school to identify and address issues in the classroom, with the goal of improving teaching practices Sampling: • • Non-Probability Sampling: • Selection based on judgment, convenience, or specific criteria. • Limited generalizability. • Common types include convenience, purposive, snowball, and quota sampling. Probability Sampling: • Every individual has a known and equal chance of being selected. • Facilitates statistical inference and generalizability. • Common methods include simple random sampling, stratified random sampling, and cluster sampling. Types of Sampling: 1. Random Sampling: • Definition: Random sampling is a probability sampling technique where every individual in the population has an equal chance of being selected. The selection is entirely based on chance, often facilitated by using random number generators or a random process. • Key Characteristics: • Ensures each member of the population has an equal probability of being chosen. • Minimizes bias and supports generalizability. • Common in simple random sampling and systematic random sampling. • Example: Assigning each member of a population a unique number and then using a random number generator to select a subset for the study. 2. Convenience Sampling: probabilitya ↳non-probabilityple Definition: Convenience sampling is a non-probability sampling method where participants are selected based on their easy accessibility or availability to the researcher. It is often chosen for its practicality and convenience. • Key Characteristics: • Participants are selected based on convenience or accessibility. • Quick and cost-effective. • May introduce bias due to non-random selection. • Example: Surveying individuals who happen to be in a specific location at a given time, such as people in a shopping mall. 3. Multistage Sampling: • Definition: Multistage sampling is a complex sampling method involving multiple stages of sampling. It often starts with a broad sampling of clusters and then narrows down to individual units within those clusters. • Key Characteristics: • Involves multiple stages of sampling. • Clusters are sampled first, followed by further sampling within selected clusters. • Common in large-scale surveys or studies with a hierarchical structure. • Example: Sampling states randomly, then sampling cities within those states, and finally sampling individuals within those cities. 4. Quota Sampling: • Definition: Quota sampling is a non-probability sampling method where the researcher selects participants based on pre-defined characteristics or quotas to ensure the sample represents specific subgroups in the population. • Key Characteristics: • Targets specific subgroups based on characteristics (e.g., age, gender, occupation). • Non-random selection within each quota. • Used when random sampling is challenging, but some diversity is desired. • Example: Ensuring a survey sample includes a proportional representation of different age groups or income levels. 5. Purposive/Selective Sampling: • Definition: Purposive or selective sampling is a non-probability sampling method where participants are deliberately chosen based on specific characteristics or criteria that align with the research objectives. • Key Characteristics: • Participants are selected purposefully based on specific criteria. • Often used when studying a particular subgroup or phenomenon. • May lack generalizability due to non-random selection. • Probability Sampling: 1. Simple Random Sampling: Definition: • Simple random sampling is a method of sampling where each member of the population has an equal probability of being selected, and the selection of one individual does not affect the probability of selecting another. Example: • If you have a list of 100 students and you use a random number generator to select 20 students for your sample, ensuring every student has an equal chance of being chosen, it's a simple random sample. 2. Stratified Random Sampling: Definition: • Stratified random sampling involves dividing the population into subgroups or strata based on certain characteristics that are important to the study. Samples are then randomly selected from each stratum. Example: • If you're studying a population of students and you stratify by grade level (e.g., freshman, sophomore, junior, senior), and then randomly sample from each grade level, it's a stratified random sample. 3. Cluster Sampling: Definition: • Cluster sampling involves dividing the population into clusters or groups and randomly selecting entire clusters for inclusion in the study. The sampling occurs in two stages: first, clusters are chosen, and then individuals within the selected clusters are sampled. Example: • If you're studying a population of schools and you randomly select a few schools, and then include all students from the selected schools in your study, it's a cluster sample. • Example: Selecting individuals with a specific medical condition for an in-depth study on their experiences with a particular treatment. 6. Matched Sample • Matching Process: • Participants are paired or matched based on specific characteristics that are known or suspected to influence the dependent variable. • Purpose: • The purpose of matching is to create comparable groups, minimizing the impact of individual differences on the outcomes of interest. • Variables for Matching: • Variables chosen for matching are typically those believed to be potential sources of variation and could act as confounding variables. • Random Assignment: • After the matching process, participants within each pair are randomly assigned to different conditions, ensuring that the experimental manipulation is applied equally across comparable groups. Target population vs. accessible population Target Population: • Definition: The target population is the entire group of individuals or elements that the researcher is interested in studying and to which they intend to generalize their research findings. • Characteristics: • Represents the broader group under investigation. • May be defined based on specific criteria or characteristics. • The ideal or theoretical group to which study findings are meant to apply. • Example: If a researcher is interested in studying the sleep habits of teenagers in the United States, the target population would be all teenagers in the U.S. Accessible Population: • Definition: The accessible population is the subset of the target population that the researcher can realistically reach and include in the study. • Characteristics: • Represents the portion of the target population that is accessible or available for study. • Defined by practical constraints such as time, budget, and geographical limitations. The group from which the actual sample will be drawn. Example: Using the example of studying sleep habits in teenagers, the accessible population may be limited to teenagers attending a specific school or living in a particular city due to practical constraints. • • Data Collection: Researcher as an Instrument: • Concept: • The "researcher as an instrument" refers to the idea that, in some research settings, the primary tool for data collection is the researcher themselves. This is particularly relevant in qualitative research, where the researcher's skills, perceptions, and interactions play a crucial role in gathering and interpreting data. • Characteristics: • The researcher's subjectivity and role in the research process are acknowledged. • Data collection involves direct engagement, interpretation, and understanding of participants and the research context. • The researcher's qualities, such as empathy and cultural sensitivity, influence the quality of data. • Example: In ethnographic research, a researcher immerses themselves in a community, observing and interacting with participants, with their own experiences and interpretations shaping the data collection process. Types of Instruments: • Concept: • Instruments in research refer to tools or methods used for collecting data. These can be quantitative or qualitative, and the choice depends on the research design and goals. • Examples: • Likert Scale: • Description: A Likert scale is a common type of rating scale used in surveys or questionnaires to measure attitudes or opinions. Participants express their level of agreement or disagreement with a statement using a scale, typically ranging from "Strongly Disagree" to "Strongly Agree." • Example: "Please rate your satisfaction with the product on a scale from 1 (Very Dissatisfied) to 5 (Very Satisfied)." • Visual Analogue Scale (VAS): Description: A visual analogue scale is a measurement tool often used to assess subjective experiences or perceptions. Participants mark a point along a continuous line to indicate their response, providing a more nuanced measurement. • Example: A scale representing pain intensity, where participants mark a point on a line to indicate their pain level, with "No Pain" on one end and "Worst Pain Imaginable" on the other. Qualitative Data Collection Methods: • Field Notes: • Description: Field notes are detailed, narrative descriptions recorded by a researcher during or after direct observation of a phenomenon. These notes capture the context, behaviors, and interactions observed in the field. • Example: A researcher observing a classroom might record details about teacher-student interactions, student engagement, and environmental factors. • Interviews: • Description: Interviews involve direct communication between the researcher and participants, with the goal of gathering in-depth information. Structured, semi-structured, or unstructured interview formats may be used. • Example: Conducting one-on-one interviews with individuals to explore their experiences with a particular health condition. • Observations: • Description: Observations involve systematic and purposeful watching of participants in their natural setting. This method is often used to understand behavior, interactions, and contexts. • Example: Observing and recording playground behavior to study social interactions among children. • Validity/Reliability/Rigour: Threats to Internal and External Validity: • Internal Validity: • Definition: Internal validity refers to the extent to which the observed effects in a study can be attributed to the manipulation of the independent variable rather than other factors. • Threats: Common threats include selection bias, history effects, maturation, testing effects, and instrumentation. • External Validity: Definition: External validity concerns the generalizability of study findings to other populations, settings, or times. • Threats: Threats to external validity include population validity (how well findings generalize to a larger population), ecological validity (how well findings generalize to real-world settings), and temporal validity (how well findings generalize over time). Tests of Reliability (Cronbach Alpha, KR-20): • Cronbach Alpha: • Definition: Cronbach's alpha is a measure of internal consistency reliability, indicating how well the items in a scale or test correlate with each other. • Use: Commonly used in the social sciences to assess the reliability of survey instruments or scales. • Interpretation: Higher values (closer to 1.0) indicate greater internal consistency. • KR-20 (Kuder Richardson Coefficient): • Definition: Similar to Cronbach's alpha, KR-20 assesses internal consistency reliability for tests with dichotomous items (e.g., true/false questions). • Use: Specifically applied when dealing with tests that involve dichotomous response categories. • Interpretation: Similar to Cronbach's alpha, higher values indicate greater internal consistency. Types of Validity (Face, Content): • Face Validity: • Definition: Face validity is the extent to which a measurement or test appears to measure what it claims to measure based on its face value. • Example: If a teacher creates an exam, face validity involves the perception that the questions on the exam are relevant to the course material. • Content Validity: • Definition: Content validity assesses whether a measurement reflects the entire range of the construct being measured. • Example: In the development of a mathematics test, content validity would involve ensuring that the test covers a representative sample of the math skills taught in the curriculum. Qualitative Rigor (Credibility, Transferability, Dependability, Confirmability): • Credibility: • ¡ 3. Criterion Validity ¡ indicates how well, or poorly, an instrument compares to either another instrument or another predictor. There are 2 types of criterion validity: ¡ Concurrent: is used to determine the accuracy of a data collec=on instrument by comparing it with another data collec=on instrument i.e. compare to a “gold standard” ¡ Predic,ve validity: how accurately a measurement instrument or test will predict the outcomes at a future =me. ¡ 4. Construct Validity ¡ The extent to which a test measures a theore=cal construct or trait and aDempts to validate a body of theory underlying the measurement and tes=ng of the hypothesized rela=onships ¡ Indicates how well the scale measures the construct it was designed to measure ¡ The most complex type of validity, and involves rela=ng an instrument for data collec=on to a theore=cal framework – usually involves hypothesis tes=ng ¡ Example, a researcher inven=ng a new IQ test might spend a great deal of =me aDemp=ng to "define" intelligence in order to reach an acceptable level of construct validity. Reliability - Consistency with which a measuring instrument yields a certain, consistent result when the entity being measured hasn’t changed - Extent that the instrument yields the same result on repeated measures - Analogous to variance (low reliability = high variance) - A reliability coefficient of r= .85 means that 85% of variability in observed scores is presumed to represent true individual differences and 15% of variability is due to random error § Weak Reliability: 0.00-0.04 § Moderate Reliability: 0.41-0.60 § Strong Reliability: 0.61-0.80 § Very Strong Reliability: 0.81-1.00 o Stability: the degree to which an instrument generates similar findings from the same (or similar) group of individuals on different occasions o Internal Consistency: the degree to which items on a questionnaire measure a particular variable. o Equivalence: of two instruments (interrater reliability) § Intra-rater reliability – assesses how one person rates same observation on 2 or more occasions: consistency. § Inter-rater reliability – degree to which two or more independent observers agree. P-value: - Expressed on a scale from 0-1 - 0 signifying there is no chance of its occurrence - 1 signifying it is certain to happen - Probability is defined as the likelihood that the results were not obtained by chance alone if the null hypothesis is true - The p value is calculated based on the assumption that the null hypothesis is true and tells researchers how rarely they would observe a difference as large (or larger) than the one they did if the null hypothesis were true Qualitative Rigour Credibility: Definition: Credibility refers to the extent to which the findings of a qualitative study accurately represent the participants' experiences or the phenomenon under investigation. • Strategies: Triangulation, member checking, and prolonged engagement with participants enhance credibility. • Transferability: • Definition: Transferability involves assessing the extent to which qualitative findings can be applied or transferred to other contexts or settings. • Strategies: Providing detailed descriptions of the research context, participants, and methods enhances transferability. • Dependability: • Definition: Dependability is concerned with the consistency and stability of qualitative findings over time and across different researchers. • Strategies: Clearly documenting the research process, using an audit trail, and maintaining consistency in data collection and analysis enhance dependability. • Confirmability: • Definition: Confirmability is the degree to which the results of a qualitative study are shaped by the participants and the context rather than the biases and perspectives of the researcher. • Strategies: Maintaining reflexivity, keeping an audit trail, and involving multiple researchers in data analysis enhance confirmability. Audit Trail in Qualitative Research: • Definition: • An audit trail in qualitative research refers to a detailed record or documentation of the research process, from data collection to analysis and interpretation. • It provides transparency and allows other researchers to follow the steps taken to arrive at the study's conclusions. • Components: • An audit trail may include field notes, interview transcripts, coding schemes, decision logs, and any other documentation relevant to the research process. • It serves as a means to ensure rigor and accountability in qualitative research. • Purpose: • The audit trail allows other researchers to assess the reliability and validity of the study by retracing the steps and decisions made during the research process. • It contributes to the dependability and confirmability of qualitative research findings. Triangulation: Triangulation in research refers to the use of multiple methods, data sources, theories, or researchers to study the same phenomenon. The goal of triangulation is to enhance the validity and reliability of research findings by cross-verifying information from different perspectives. Types of Triangulation: 1. Data Triangulation: • Involves using multiple data sources to validate findings. For example, a researcher might use both interviews and participant observations to study a phenomenon. 2. Methodological Triangulation: • Involves using multiple research methods to study the same phenomenon. This could include combining surveys, interviews, and experiments. 3. Theoretical Triangulation: • Involves using multiple theoretical perspectives to analyze data. Researchers may apply different theories to interpret the findings and gain a more comprehensive understanding. 4. Researcher Triangulation: /Investigator Triangulation • Involves having multiple researchers independently analyze and interpret the data. Any discrepancies in their interpretations can be discussed and resolved to enhance reliability. : • It contributes to the dependability and confirmability of qualitative research findings. Data Analysis: Quantitative: 1. Parametric/Non-parametric Tests: • Parametric Tests: • Definition: Parametric tests assume that the data being analyzed follow a specific probability distribution, typically the normal distribution. They make certain assumptions about population parameters. • Examples: t-tests, ANOVA, Pearson correlation. • Non-parametric Tests: • Definition: Non-parametric tests do not assume a specific probability distribution and are more robust in the face of deviations from normality. They are used when data may not meet parametric assumptions. • Examples: Mann-Whitney U test, Kruskal-Wallis test, Spearman correlation. 2. T-tests, ANOVA, MANOVA, Correlation Coefficients: • T-tests: • Definition: A t-test is used to compare the means of two groups to determine if there is a statistically significant difference between them. • Types: Independent samples t-test (for independent groups), paired samples t-test (for related groups). • Independent Samples T-test: - The independent samples t-test is used when comparing the means of two independent groups to determine if there is a statistically significant difference between them. • Independent Groups: • The groups being compared are independent, meaning that the individuals in one group are not related or matched to the individuals in the other group. • Assumes Equal Variances: • Assumes that the variances of the two groups are equal. If this assumption is violated, a modified version of the test called Welch's t-test may be used. Dependant Sample T Test - The dependent samples t-test, also known as the paired samples t-test, is used when comparing the means of two related groups, such as when measurements are taken from the same group at different time points or under different conditions. Dependent or Matched Groups: • The groups being compared are dependent, meaning that the measurements in one group are related to the measurements in the other group (e.g., repeated measures on the same individuals). • Assumes Equal Differences: • Assumes that the differences between the paired observations are normally distributed. • ANOVA (Analysis of Variance): • Definition: ANOVA is used to compare means across more than two three groups. It assesses whether there are any statistically significant differences among the means of these groups. • Types: One-way ANOVA (for one independent variable), Two-way ANOVA (for two independent variables). • MANOVA (Multivariate Analysis of Variance): • Definition: MANOVA extends ANOVA to multiple dependent variables, allowing the simultaneous analysis of relationships across these variables. • Use: Useful when there are multiple outcome variables. • Correlation Coefficients: • Definition: Correlation coefficients quantify the strength and direction of a linear relationship between two continuous variables. • Types: Pearson correlation coefficient (for linear relationships between normally distributed variables), Spearman rank correlation coefficient (for monotonic relationships), Kendall's tau (another measure of rank correlation). 3. Levels of Measurement (Nominal, Ordinal, Interval, Ratio): • Nominal: • Definition: Nominal data represent categories without any inherent order or ranking. They are used for labeling variables. • Example: Gender, color. • Ordinal: • Definition: Ordinal data represent categories with a meaningful order or ranking, but the intervals between the categories are not consistent. • Example: Educational attainment levels (e.g., high school, bachelor's degree, master's degree). • Interval: • Definition: Interval data have a meaningful order, and the intervals between values are consistent. However, there is no true zero point. • Example: Temperature measured in Celsius or Fahrenheit. • - • Ratio: Definition: Ratio data have a meaningful order, consistent intervals, and a true zero point, meaning zero represents the absence of the measured quantity. • Example: Height, weight, income. 4. Type I and Type II Errors: • Type I Error (False Positive): • Definition: Type I error occurs when a null hypothesis that is actually true is rejected. It represents the probability of mistakenly concluding that there is an effect when there is none. • Significance Level: Often denoted by alpha (α). • Type II Error (False Negative): • Definition: Type II error occurs when a null hypothesis that is actually false is not rejected. It represents the probability of failing to detect an effect that truly exists. • Power: The probability of correctly rejecting a false null hypothesis is called statistical power. 5. Measures of Central Tendency: • Mean: • Definition: The arithmetic mean is the sum of all values divided by the number of values. • Use: Sensitive to extreme values, appropriate for interval and ratio data. • Median: • Definition: The median is the middle value when data are ordered. It is less influenced by extreme values. • Use: Suitable for ordinal, interval, and ratio data. • Mode: • Definition: The mode is the most frequently occurring value in a dataset. • Use: Appropriate for nominal, ordinal, interval, and ratio data. Qualitative: 1. Reflexivity: • Definition: • Reflexivity in qualitative research refers to the researcher's awareness and consideration of their own role, biases, and influence on the research process. It involves acknowledging and critically reflecting on the impact of the researcher's background, experiences, and perspectives on the study. • Significance: • Reflexivity is essential for maintaining transparency and rigor in qualitative research. It allows researchers to recognize how their subjectivity may influence data collection, interpretation, and the overall study. • Application: • Researchers engage in reflexivity by documenting their personal biases, beliefs, and assumptions. They may also reflect on how their presence and interactions with participants shape the research outcomes. 2. Constant Comparison (Coding, Theoretical Coding): • Constant Comparison: • Definition: • Constant comparison is a qualitative analysis method where data are systematically compared as they are collected and analyzed. It involves continuously comparing new data with previously collected data to identify patterns, similarities, and differences. • Coding: • Definition: • Coding is the process of systematically categorizing and labeling segments of qualitative data to identify themes or patterns. It helps organize and structure data for analysis. • Example: Assigning labels or codes to segments of interview transcripts that represent common themes or concepts. • In-vivo coding is a qualitative research coding technique where researchers use participants' own words or phrases to label and categorize segments of data. • Theoretical Coding: • Definition: • Theoretical coding goes beyond simple categorization and involves the development of higher-level concepts or theories that explain relationships between codes. It aims to build theoretical insights from the data. • Example: Identifying overarching themes that connect and explain the coded segments, contributing to the development of a theoretical framework. 3. Computer Programs in Qualitative Analysis: • Definition: • Computer programs, often referred to as Computer-Assisted Qualitative Data Analysis Software (CAQDAS), assist researchers in managing, organizing, and analyzing large volumes of qualitative data. • Examples: NVivo, ATLAS.ti, MAXQDA. • Functions: • These programs facilitate coding, sorting, and querying qualitative data. They often include features for organizing and visualizing data, supporting the analysis process. • Benefits: • CAQDAS enhances the efficiency and rigor of qualitative analysis. It allows researchers to code data systematically, explore patterns, and generate visual representations of the data. 4. Thematic Analysis: • Definition: • Thematic analysis is a method of qualitative data analysis that involves identifying, analyzing, and reporting patterns (themes) within the data. It is a flexible and widely used approach suitable for various research questions. • Steps: • Familiarization: Becoming familiar with the data. • Generating Initial Codes: Coding interesting features in the data. • Searching for Themes: Identifying potential themes across codes. • Reviewing Themes: Checking if themes make sense in relation to the coded extracts. • Defining and Naming Themes: Developing clear definitions and names for each theme. • Writing the Report: Presenting the analysis, often supported by quotes from the data. 5. Data Display and Its Purpose: • Definition: • Data display in qualitative research involves presenting selected portions of data in an organized and visually accessible format. Displays may include tables, matrices, charts, or diagrams. • Purpose: • Facilitating Analysis: Data displays aid researchers in visually organizing and comparing data, making patterns and themes more apparent. • Enhancing Transparency: Displaying data allows readers to see the evidence supporting the findings, promoting transparency and credibility. • Communication: Data displays are used in research reports or presentations to communicate complex information more effectively. • Examples: • Matrix Table: Displaying themes across participants or cases. • Conceptual Diagram: Visualizing relationships between key concepts. • • Quotes and Excerpts: Presenting illustrative quotes from participants to support findings. Ethics: 1. Principlism (Autonomy, Beneficence, Non-maleficence, Justice): • Principlism: • Definition: Principlism is an ethical framework commonly applied in bioethics and health research. It involves the consideration of four key ethical principles: autonomy, beneficence, non-maleficence, and justice. • Autonomy: • Definition: Respecting individuals' right to make their own informed decisions about their participation in research or healthcare. It involves obtaining voluntary and informed consent. • Beneficence: • Definition: Acting in the best interest of participants by maximizing benefits and minimizing potential harms. Researchers must strive to maximize the positive outcomes of their research. • Non-maleficence: • Definition: The principle of "do no harm." Researchers should avoid causing harm to participants and minimize any potential risks associated with the research. • Justice: • Definition: Ensuring fairness in the distribution of the benefits and burdens of research. This involves addressing issues of inclusivity, avoiding exploitation, and treating participants and communities justly. 2. Health Research Ethics Boards (HREBs): • Definition: • Health Research Ethics Boards (HREBs), also known as Institutional Review Boards (IRBs), are committees responsible for reviewing and approving the ethical aspects of research involving human participants. Their primary goal is to protect the rights, welfare, and well-being of research participants. • Functions: • HREBs assess research protocols to ensure ethical standards are met, including the principles of autonomy, beneficence, non-maleficence, and justice. • They evaluate the informed consent process, the risks and benefits of the research, the qualifications of the researchers, and the protection of vulnerable populations. Approval Process: • Researchers must submit their research proposals to the HREB for ethical review and approval before commencing the study. The board may request modifications or clarifications before granting approval. 3. Handling of Data: • Data Management: • Definition: Handling data in research involves the collection, storage, analysis, and sharing of information gathered during the research process. • Confidentiality: • Principle: Researchers must take measures to protect the confidentiality of participants. Identifiable information should be kept secure, and data should be anonymized when possible. • Data Security: • Principle: Researchers are responsible for ensuring the security of data to prevent unauthorized access or breaches. This includes secure storage and transmission of data. • Data Retention: • Principle: Researchers should establish clear guidelines for the retention and disposal of data. Retention periods should align with ethical standards and legal requirements. 4. Obligations to Participants: • Informed Consent: • Definition: Researchers must obtain voluntary and informed consent from participants before their involvement in the study. Participants should be aware of the purpose, procedures, risks, and benefits of the research. • Respect for Autonomy: • Principle: Researchers must respect participants' autonomy by allowing them to make decisions about their involvement in the study. Coercion and undue influence should be avoided. • Protection from Harm: • Principle: Researchers have an obligation to minimize the risks of harm to participants and to promptly address any adverse events that may occur during the research. 5. Scientific Misconduct: • Definition: • Scientific misconduct refers to unethical practices in the conduct of research, which may include fabrication, falsification, or plagiarism. It undermines the integrity of the research process and the trust placed in scientific findings. • • Types: Fabrication: Making up data or results. • Falsification: Manipulating or altering data or results. • Plagiarism: Presenting someone else's work or ideas as one's own without proper attribution. Consequences: • Scientific misconduct can lead to serious consequences, including retraction of publications, loss of research funding, damage to reputation, and, in extreme cases, legal action. Prevention: • Institutions and researchers must promote a culture of research integrity, provide education on ethical conduct, and have mechanisms in place for reporting and addressing allegations of misconduct. • • • Reading/Critiquing Research Reports: Descriptive and Inferential Statistics in Research Articles: • Descriptive Statistics: • Definition: Descriptive statistics summarize and describe the main features of a dataset. They include measures such as mean, median, mode, range, standard deviation, and frequency distributions. • Purpose: Descriptive statistics provide a clear and concise overview of the data, helping researchers understand central tendencies, variability, and the distribution of scores. • Inferential Statistics: • Definition: Inferential statistics involve drawing inferences or making predictions about a population based on a sample of data. They include techniques such as t-tests, ANOVA, regression analysis, and correlation. • Purpose: Inferential statistics help researchers generalize findings from a sample to a larger population, assess the statistical significance of relationships, and test hypotheses. Order of Reporting Statistics in a Research Article: In a research article, the reporting of statistics typically follows a specific order: 1. Descriptive Statistics: • Location Measures: Begin with measures of central tendency (e.g., mean, median, mode). • Spread Measures: Follow with measures of variability or dispersion (e.g., range, standard deviation). Distribution Shape: If relevant, describe the shape of the distribution (e.g., normal, skewed). 2. Inferential Statistics: • Test Selection: Clearly state the statistical tests used to analyze the data (e.g., t-test, ANOVA, regression). • Results: Report the results of the statistical tests, including test statistics, degrees of freedom, p-values, and effect sizes. • Interpretation: Discuss the meaning and implications of the statistical findings in relation to the research question. 3. Graphs and Tables: • Present visual representations: Include graphs or tables to visually display key findings and enhance readers' understanding of the data. • Caption and Interpretation: Provide clear captions and interpretations for each visual element. 4. Consistency in Reporting: • Consistency: Maintain consistency in the reporting format throughout the results section to ensure clarity and facilitate readers' understanding. • Supplementary Material: Place extensive or detailed statistical information in supplementary materials or appendices, referring readers to these additional resources when necessary. •