5 Guidelines in Scale Development PDF
Document Details
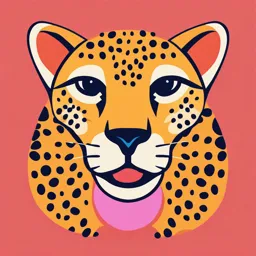
Uploaded by SmittenMesa
Tags
Full Transcript
5 Guidelines in Scale Development Thus far, our discussion has been fairly abstract. We now look at how this knowledge can be applied. This chapter provides a set of specific guidelines that investigators can use in developing measurement scales. Step 1: Determine Clearly What It Is You Want to Me...
5 Guidelines in Scale Development Thus far, our discussion has been fairly abstract. We now look at how this knowledge can be applied. This chapter provides a set of specific guidelines that investigators can use in developing measurement scales. Step 1: Determine Clearly What It Is You Want to Measure This is deceptively obvious, and many researchers think they have a clear idea of what they wish to measure only to find that their ideas are vaguer than they thought. Frequently, this realization occurs after considerable effort has been invested in generating items and collecting data—a time when changes are far more costly than if discovered at the outset of the process. Should the scale be based in theory, or should you strike out in new intellectual directions? How specific should the measure be? Should some aspect of the phenomenon be emphasized more than others? Theory as an Aid to Clarity As noted in Chapter 1, thinking clearly about the content of a scale requires thinking clearly about the construct being measured. Although there are many technical aspects involved in developing and validating a scale, one should not overlook the importance of being well grounded in the substantive theories related to the phenomenon to be measured. The types of scales that are the primary focus of this book are intended to measure elusive phenomena that cannot be observed directly. Because there is no tangible criterion against which one can compare this type of scale’s performance, it is important to have some clear ideas to serve as a guide. The boundaries of the phenomenon must be recognized so that the content of the scale does not inadvertently drift into unintended domains. Theory is a great aid to clarity. Relevant social science theories should always be considered before developing a scale of the type discussed in this volume. If it turns out that extant theory offers no guide to the scale developers, then they may decide that a new intellectual direction is necessary. However, this decision should be an informed one, reached only after reviewing appropriate theory related to the measurement problem at hand. Even if there is no available theory to guide the investigators, they must lay out their own conceptual formulations prior to trying to operationalize them. In essence, they must specify at least a tentative theoretical model that will serve as a guide to scale development. This may be as simple as a well-formulated definition of the phenomenon they seek to measure. Better still would be to include a description of how the new construct relates to existing phenomena and their operationalizations. 103 Specificity as an Aid to Clarity The level of specificity or generality at which a construct is measured also may be important. There is general agreement in the social sciences that variables will relate most strongly to one another when they match with respect to level of specificity (see Ajzen & Fishbein, 1980, for a discussion). Sometimes, a scale is intended to relate to very specific behaviors or constructs, while at other times, a more general and global measure is sought. As an illustration of measures that differ in specificity, consider the locus of control construct. Locus of control is a widely used concept that concerns individuals’ perceptions about who or what influences important outcomes in their lives. The construct can be applied broadly, as a means of explaining patterns of global behavior spanning many situations, or narrowly, to predict how an individual will respond in a very specific context. The sources of influence also can be described either broadly or specifically. Rotter’s (1966) Internal-External scale, for example, is concerned at a fairly general level with these perceptions. A single dimension ranging from personal control to control by outside factors underlies the scale, and the outcomes on which the items focus are general, such as personal success. The external sources of control also are described in general terms. The following external statement is from Rotter’s Internal-External scale: “The world is run by the few people in power, and there is not much the little guy can do about it.” Levenson (1973) developed a multidimensional locus of control scale that allows for three loci of control: oneself, powerful other people, and chance or fate. This permits an investigator to look at external sources of control a bit more specifically by characterizing them as either powerful others or fate. The outcomes on which she focused, however, remained general. An example of an item from Levenson’s Powerful Others subscale is “I feel like what happens in my life is determined by powerful others.” Wallston, Wallston, and DeVellis (1978) developed the Multidimensional Health Locus of Control (MHLC) scales using Levenson’s three loci of control, with outcomes specific to health, such as avoiding illness or getting sick. A sample item from the Powerful Others scale of the MHLC is “Having regular contact with my physician is the best way for me to avoid illness.” Wallston, Stein, and Smith (1994) subsequently developed an even more outcome-specific health locus of control measure (MHLC Form C) that consists of a series of “template” items. This measure allows the researcher to specify any health problem of interest by substituting the name of the illness or disorder for the phrase “my condition” in each of the template items. A sample item from the Powerful Others scale of MHLC Form C, as it might be used in a study of diabetes, is “If I see my doctor regularly, I am less likely to have problems with my diabetes.” Each of these progressively more specific locus of control scales is potentially useful. Which is most useful depends largely on what level of outcome or locus generality relates to the scientific question being asked. For example, if a locus of control scale is intended to 104 predict a general class of behavior or will be compared with other variables assessing constructs at a general level, then Rotter’s scale may be the best choice because it, too, is general. On the other hand, if a researcher is interested in predicting specifically how beliefs about the influence of other people affect certain health behaviors, then the Wallston et al. (1994) scale may be more appropriate because the level of specificity matches that research question. During its development, each of these scales had a clear frame of reference that determined what level of specificity was appropriate, given the intended function of the scale. The point is that scale developers should make this determination as an active decision and not merely generate a set of items and then see what they look like after the fact. The locus of control example illustrated specificity with respect to outcomes (e.g., how the world is run vs. problems with diabetes) and the loci of control (i.e., external generally vs. fate and powerful others separately). However, scale specificity can vary along a number of dimensions, including content domains (e.g., anxiety vs. psychological adjustment more broadly), setting (e.g., questionnaires designed specifically for relevance to particular work environments), or population (e.g., children vs. adults or military personnel vs. college students). Being Clear About What to Include in a Measure Scale developers should ask themselves if the construct they wish to measure is distinct from other constructs. As noted earlier, scales can be developed to be relatively broad or narrow with respect to the situations to which they apply. This is also the case with respect to the constructs they cover. Measuring general anxiety is perfectly legitimate. Such a measure might assess both test anxiety and social anxiety. This is fine if it matches the goals of the scale developer or user. However, if one is interested in only one specific type of anxiety, then the scale should exclude all others. Items that might “cross over” into a related construct (e.g., tapping social anxiety when the topic of interest is test anxiety) can be problematic. Sometimes, apparently similar items may tap quite different constructs. In such cases, although the purpose of the scale may be to measure one phenomenon, it may also be sensitive to other phenomena. For example, certain depression measures, such as the Center for Epidemiological Studies Depression Scale (Radloff, 1977), have some items that tap somatic aspects of depression (e.g., concerning the respondent’s ability to “get going”). In the context of some health conditions, such as arthritis, these items might mistake aspects of the illness for symptoms of depression (see Blalock, DeVellis, Brown, & Wallston, 1989, for a discussion of this specific point). A researcher developing a new depression scale might choose to avoid somatic items if the scale was to be used with certain populations (e.g., the chronically ill) or with other measures of somatic constructs (such as hypochondriasis). Used for other purposes, of course, it might be very important to include somatic items, as 105 when the line of investigation specifically concerns somatic aspects of negative affect. Step 2: Generate an Item Pool Once the purpose of a scale has been clearly articulated, the developer is ready to begin constructing the instrument in earnest. The first step is to generate a large pool of items that are candidates for eventual inclusion in the scale. Choose Items That Reflect the Scale’s Purpose Obviously, these items should be selected or created with the specific measurement goal in mind. The description of exactly what the scale is intended to do should guide this process. Recall that all items making up a homogeneous scale should reflect the latent variable underlying them. Each item can be thought of as a test, in its own right, of the strength of the latent variable. Therefore, the content of each item should primarily reflect the construct of interest. Multiple items will constitute a more reliable test than individual items, but each must still be sensitive to the true score of the latent variable. Theoretically, a good set of items is chosen randomly from the universe of items relating to the construct of interest. The universe of items is assumed to be infinitely large, which pretty much precludes any hope of actually identifying it and extracting items randomly. However, this ideal should be kept in mind. If you are writing items anew, as is so often the case, you should think creatively about the construct you seek to measure. What other ways can an item be worded so as to get at the construct? Although the items should not venture beyond the bounds of the defining construct, they should exhaust the possibilities for types of items within those bounds. The properties of a scale are determined by the items that make it up. If they are a poor reflection of the concept you have worked long and hard to articulate, then the scale will not accurately capture the essence of the construct. It is also important that the “thing” the items have in common be truly a construct and not merely a category. Recall, once again, that our models for scale development regard items as overt manifestations of a common latent variable that is their cause. Scores on items related to a common construct are determined by the true score of that construct. However, as noted in Chapter 1, just because items relate to a common category, that does not guarantee that they have the same underlying latent variable. Such terms as attitudes, barriers to compliance, or life events often define categories of constructs rather than the constructs themselves. A pool of items that will eventually be the basis of a unidimensional scale should not merely share a focus on attitudes, for example, but on specific attitudes, such as attitudes toward punishing drug abusers. One can presumably envision a characteristic of the person—a latent variable, if you will—that would “cause” responses to items dealing with punishing drug abusers. It is quite a challenge to imagine a characteristic that accounts for attitudes in general. The same is true for the other examples cited. Barriers 106 to compliance are typically of many types. Each type (e.g., fear of discovering symptoms, concern over treatment costs, anticipation of pain, distance of treatment facilities, perceptions of invulnerability) may represent a latent variable. There may even be nontrivial correlations among some of the latent variables. However, each of these barriers is a separate construct. Thus, the term barriers describes a category of constructs rather than an individual construct related to a single latent variable. Items measuring different constructs that fall within the same category (e.g., perceptions of invulnerability and concerns over treatment costs) should not be expected to covary the way items do when they are manifestations of a common latent variable. Redundancy Paradoxically, redundancy is both a good and a bad feature of items within a scale. Resolving this paradox entails distinguishing between item features that strengthen a scale through repetition and those that do not. Because this topic is often a source of confusion, I will discuss it in some detail. I will first make the case in favor of redundancy. At this stage of the scale development process, it is better to be more inclusive, all other things being equal. Redundancy is not a bad thing when developing a scale. In fact, the theoretical models that guide our scale development efforts are based on redundancy. In discussing the Spearman-Brown prophecy formula in Chapter 3, I pointed out that reliability varies as a function of the number of items, all else being equal. We are attempting to capture the phenomenon of interest by developing a set of items that reveal the phenomenon in different ways. By using multiple and seemingly redundant items, the content that is common to the items will summate across items while their irrelevant idiosyncrasies will cancel out. Without redundancy, this would be impossible. Not all forms of redundancy are desirable, however. Useful redundancy pertains to the construct, not incidental aspects of the items. Consider two items: an original, “A really important thing is my child’s success,” and an altered version, “The really important thing is my child’s success.” Changing nothing more than an “a” to “the” in an item will certainly give you redundancy with respect to the important content of the item, but the original and altered items will also be redundant with respect to many things that you want to vary, such as their basic grammatical structure and choice of words. On the other hand, two items such as “I will do almost anything to ensure my child’s success” and “No sacrifice is too great if it helps my child succeed” may be usefully redundant because they express a similar idea in somewhat different ways. They are redundant with respect to the variable of interest but not with respect to their grammatical structure and incidental vocabulary. When irrelevant redundancies are avoided, relevant redundancies will yield more reliable item sets. Moreover, although redundancy in the final instrument may be undesirable, it is less of an 107 issue during the early stages of item development. Consequently, even the two item versions differing by only a single word might be worth including in initial item testing. By doing so, it can be ascertained whether one or the other version is superior, and then the superior item can be incorporated into the final version of the scale. The argument against redundancy has partially been made: Redundant superficial item characteristics, such as incidental (i.e., construct-irrelevant) vocabulary or grammatical structure, are not an advantage. Construct-irrelevant similarities in wording may result in respondents’ reacting similarly to items in a way that produces an inflated estimate of reliability. For example, if several items begin with a common phrase (e.g., “When I think about it,…”), merely sharing that phrase may cause those items to correlate more strongly with one another. A reliability indicator such as Cronbach’s alpha would not distinguish between item covariation arising from that common wording and covariation attributable to the common influence of the variable of interest. Thus, reliability would be inflated. While similar grammatical or other superficial features can constitute unwanted content similarity, redundancy that is not completely unrelated to the construct of interest can also create a problem under some circumstances. This can occur when certain items differ from most other items in a set with respect to specificity. As an example, consider a hypothetical scale intended to measure attitudes toward pet lovers. A wide variety of items might be suitable for inclusion. Other items, although relevant to the construct of interest, may be too specific—and consequently too redundant—to work well. The items “African grey parrot lovers are kind” and “I think people who like African grey parrots are good people” may be too much alike not merely because of grammatical similarity but because of potentially relevant but overly specific content that the two items share. They may pull the item set as a whole away from the intended latent variable (attitudes toward pet lovers) to an alternative, more specific latent variable (attitudes toward African grey parrot lovers). Given the wide range of pets that exist, two items about a specific and uncommon pet species are glaringly similar and likely to undermine the intent of the instrument. More generally, how global or specific the construct of interest is can alter the impact of redundancy. Although the African grey parrot example may seem a bit extreme, inclusion of items that do not match the specificity of the construct of interest can occur in less exotic contexts. For example, in an instrument that has been designed to capture all aspects of emotion, several items about anxiety may pose a problem. Correlations among those items are likely to be greater than correlations between those items and others not about anxiety. As a consequence, these items may form a subcluster of anxiety items within the broader cluster of emotion items. This can create a number of problems. First, it may undermine the unidimensionality of the item set (which would be a problem if the investigator’s intent was to develop a single measure of a unitary variable). Also, it may create an unintended focal point that results in items more similar to those in the anxiety cluster appearing to perform better than ones that are less similar. For example, although an item about worry and another about fear may be equally relevant to a broad view of emotions, the former 108 might contribute more strongly to reliability than the latter if there is a preponderance of anxiety items in the instrument. As a result, the average correlation of the worry item might exceed the average correlation of the fear item, resulting in its stronger contribution to an estimate of reliability. De facto, an instrument including an overrepresentation of anxiety items as described would not be about all emotions equally but would be biased toward anxiety. In contrast, the same type of anxiety items described as problematic in the preceding paragraph may not be overly redundant in an instrument with a narrower focus. Obviously, if the instrument is designed to assess anxiety, all the items should be related to that variable and that similarity would not be an instance of unwanted redundancy. In contrast, items that included a more general phrase, such as “my overall feelings,” might form a subcluster if included in an anxiety scale because of their nonspecific emotional focus. What appears to be a redundancy issue, however, may actually be a matter of how well items match the specificity of the construct the investigator intends to assess. In an instrument intended to tap a more specific variable, it is likely that the items will appear more similar to one another. Typically, for example, items in a scale measuring public speaking anxiety will appear more similar to one another (because of the specificity of the variable of interest) than items from a scale measuring emotional states more broadly. This may not be a problem as long as the similarities relate to the construct of interest. As stated earlier, items that are similar insofar as they share relevance to the intended variable and not in any other regard can be good items. Number of Items It is impossible to specify the number of items that should be included in an initial pool. Suffice it to say that you want considerably more than you plan to include in the final scale. Recall that internal consistency reliability is a function of how strongly the items correlate with one another (and hence with the latent variable) and how many items you have in the scale. As the nature of the correlations among items is usually not known at this stage of scale development, having lots of items is a form of insurance against poor internal consistency. The more items you have in your pool, the fussier you can be about choosing ones that will do the job you intend. It would not be unusual to begin with a pool of items that is three or four times as large as the final scale. Thus, a 10-item scale might evolve from a 40-item pool. If items are particularly difficult to generate for a given content area or if empirical data indicate that numerous items are not needed to attain good internal consistency, then the initial pool may be as small as 50% larger than the final scale. In general, the larger the item pool, the better. However, it is certainly possible to develop a pool too large to administer on a single occasion to any one group of subjects. If the pool is exceptionally large, the researcher can eliminate some items based on a priori criteria, such 109 as lack of clarity, questionable relevance, or undesirable similarity to other items. Beginning the Process of Writing Items Getting started writing items is often the most difficult part of the item generation process. Let me describe how I begin this process. At this point, I am less interested in item quality than in merely expressing relevant ideas. I often begin with a statement that is a paraphrase of the construct I want to measure. For example, if I were interested in developing a measure of self-perceived susceptibility to commercial messages, I might begin with “I am susceptible to commercial messages.” I then would try to generate additional statements that get at the same idea somewhat differently. My next statement might be “Commercial messages affect me a lot.” I would continue in this manner, imposing virtually no quality standards on the statements. My goal at this early stage is simply to identify a wide variety of ways that the central concept of the intended instrument can be stated. As I write, I may seek alternative ways of expressing critical ideas. For example, I might substitute “the things that I see in TV or magazine ads” for “commercial messages” in the next set of sentences. I find that writing quickly and uncritically is useful. After generating perhaps three or four times the number of items I anticipate including in the final instrument, I will look over what I have written. Now is the time to become critical. Items can be examined for how well they capture the central ideas and for clarity of expression. The sections that follow delineate some of the specific item characteristics to avoid or incorporate in the process of selecting from and revising the original statement list. Characteristics of Good and Bad Items Listing all the things that make an item good or bad is an impossible task. The content domain, obviously, has a significant bearing on item quality. However, there are some characteristics that reliably separate better items from worse ones. Most of these relate to clarity. As pointed out in Chapter 1, a good item should be unambiguous. Questions that leave the respondent in a quandary should be eliminated. Scale developers should avoid exceptionally lengthy items, as length usually increases complexity and diminishes clarity. However, it is not desirable to sacrifice the meaning of an item in the interest of brevity. If a modifying clause is essential to convey the intent of an item, then include it. However, avoid unnecessary wordiness. In general, an item such as “I often have difficulty making a point” will be better than an unnecessarily longer one, such as “It is fair to say that one of the things I seem to have a problem with much of the time is getting my point across to other people.” Another related consideration in choosing or developing items is the reading difficulty level at which the items are written. There are a variety of methods (e.g., Dale & Chall, 1948; Fry, 1977) for assigning grade levels to passages of prose, including scale items. These 110 typically equate longer words and sentences with higher reading levels. Reading most local newspapers presumably requires a sixth-grade reading level. Fry (1977) delineates several steps to quantifying reading level. The first is to select a sample of text that begins with the first word of a sentence and contains exactly 100 words. (For scales having only a few items, you may have to select a convenient fraction of 100 and base subsequent steps on this proportion.) Next, count the number of complete sentences and individual syllables in the text sample. These values are used as entry points for a graph that provides grade equivalents for different combinations of sentence and syllable counts from the 100-word sample. The graph indicates that the average number of words and syllables per sentence for a fifth-grade reading level are 14 and 18, respectively. An average sentence at the sixth-grade level has 15 or 16 words and a total of 20 syllables; a seventh- grade-level sentence has about 18 words and 24 syllables. Shorter sentences with a higher proportion of longer words or longer sentences with fewer long words can yield an equivalent grade level. For example, a sentence of 9 words and 13 syllables (i.e., as many as 44% polysyllabic words) or one with 19 words and 22 syllables (i.e., no more than about 14% polysyllabic words) are both classified as sixth-grade reading level. Aiming for a reading level between the fifth and seventh grades is probably an appropriate target for most instruments that will be used with the general population. The items of the Multidimensional Health Locus of Control scales, for example, were written at a fifth- to seventh-grade reading level. A typical item at this reading level is “Most things that affect my health happen to me by accident” (Wallston et al., 1978). The item’s 11 words and 15 syllables place it at the sixth-grade level. Fry (1977) notes that semantic and syntactic factors should be considered in assessing reading difficulty. Because short words tend to be more common and short sentences tend to be syntactically simpler, his procedure is an acceptable alternative to more complex difficulty-assessment methods. However, as with other criteria for writing or choosing good items, one must use common sense in applying reading level methods. Some brief phrases containing only short words are not elementary. “Eschew casque scorn,” for example, is more likely to confuse someone with a grade-school education than “Wear your helmet” will, despite the fact that both have three words and four syllables. Another source of potential confusion that should be avoided is multiple negatives. “I am not in favor of corporations stopping funding for anti-nuclear groups” is much more confusing than “I favor continued private support of groups advocating a nuclear ban.” (It is also instructive to observe that these two statements might convey different positions on the issue. For example, the latter might imply a preference for private over public support of the groups in question.) So-called double-barreled items should also be avoided. These are items that convey two or more ideas so that an endorsement of the item might refer to either or both ideas. “I support civil rights because discrimination is a crime against God” is an example of a double-barreled item. If a person supports civil rights for reasons other than its affront to a 111 deity (e.g., because it is a crime against humanity), how should he or she answer? A negative answer might incorrectly convey a lack of support for civil rights, and a positive answer might incorrectly ascribe a motive to the respondent’s support. Another problem that scale developers should avoid is ambiguous pronoun references. “Murderers and rapists should not seek pardons from politicians because they are the scum of the earth” might express the sentiments of some people irrespective of pronoun reference. (However, a scale developer usually intends to be more clear about what an item means.) This sentence should be twice cursed. In addition to the ambiguous pronoun reference, it is double-barreled. Misplaced modifiers create ambiguities similar to ambiguous pronoun references: “Our members of Congress should work diligently to legalize prostitution in the House of Representatives” is an example of such modifiers. Using adjective forms instead of noun forms can also create unintended confusion. Consider the differences in meaning between “All vagrants should be given a schizophrenic assessment” and “All vagrants should be given a schizophrenia assessment.” Individual words are not the only sources of item ambiguity. Entire sentences can have more than one meaning. I have actually seen one survey of adolescent sexual behavior that included an item to assess parental education. Given the context of the survey as a whole, the wording was unfortunate: “How far did your mother go in school?” The investigators had totally failed to recognize the unintended meaning of this statement until it evoked snickers from a group of professionals during a seminar presentation. I suspect that a fair number of the adolescent respondents also got a laugh from the item. How it affected their responses to the remainder of the questionnaire is unknown. Positively and Negatively Worded Items Many scale developers choose to write negatively worded items that represent low levels or even the absence of the construct of interest as well as the more common positively worded items, which represent the presence of the construct of interest. The goal is to arrive at a set of items, some of which indicate a high level of the latent variable when endorsed and others that indicate a high level when not endorsed. The Rosenberg (1965) Self-Esteem Scale, for example, includes items indicative of high esteem (e.g., “I feel that I have a number of good qualities”) and of low esteem (e.g., “I certainly feel useless at times”). The intent of wording items both positively and negatively within the same scale is usually to avoid an acquiescence, affirmation, or agreement bias. These interchangeable terms refer to a respondent’s tendency to agree with items irrespective of their content. If, for example, a scale consists of items that express a high degree of self-esteem, then an acquiescence bias would result in a pattern of responses appearing to indicate very high esteem. If the scale is made up of equal numbers of positively and negatively worded items, on the other hand, then an acquiescence bias and an extreme degree of self-esteem could be differentiated from one another by the pattern of responses. An “agreer” would endorse items indicating both 112 high and low self-esteem, whereas a person who truly had high esteem would strongly endorse high-esteem items and negatively endorse low-esteem items. Unfortunately, there may be a price to pay for including positively and negatively worded items. Reversals in item polarity may be confusing to respondents, especially when completing a long questionnaire. In such a case, the respondents may become confused about the difference between expressing their strength of agreement with a statement, regardless of its polarity, versus expressing the strength of the attribute being measured (esteem, for example). As an applied social science researcher, I have seen many examples of items worded in the opposite direction performing poorly. For example, DeVellis and Callahan (1993) described a shorter, more focused alternative to the Rheumatology Attitudes Index (an unfortunate name, as the instrument does not assess attitudes and is not an index). We selected items from the original, longer version based on empirical criteria and ended up with four items expressing negative reactions to illness and one expressing the ability to cope well with illness. The intent was that users should reverse score the “coping” item so that all items expressed a sense of helplessness. More recently, Currey, Callahan, and DeVellis (2002) have examined the performance of that single item worded in the positive direction. It consistently performs poorly. When the item was reworded simply by adding the word not to change its valence so as to be consistent with other items, its performance improved dramatically. We suspect that, although many respondents recognized the different valence of the original item, others did not. This would result in a portion of individuals for whom the original item had positive correlations with the other four items and another portion for whom the same correlations were negative. As a consequence, for the sample as a whole, correlations of that item with the other four would be markedly diminished and, thus, would produce the type of unsatisfactory performance we observed for the original, opposite-valence item. Personal experience with community-based samples suggests to me that the disadvantages of items worded in an opposite direction outweigh any benefits. Conclusion An item pool should be a rich source from which a scale can emerge. It should contain a large number of items that are relevant to the content of interest. Redundancy with respect to content is an asset, not a liability. It is the foundation of internal-consistency reliability which, in turn, is the foundation of validity. Items should not involve a “package deal” that makes it impossible for respondents to endorse one part of the item without endorsing another part that may not be consistent with the first. Whether or not positively and negatively worded items are both included in the pool, their wording should follow established rules of grammar. This will help avoid some of the sources of ambiguity discussed above. 113 Step 3: Determine the Format for Measurement Numerous formats for questions exist. The researcher should consider early on what the format will be. This step should occur simultaneously with the generation of items so that the two are compatible. For example, generating a long list of declarative statements may be a waste of time if the response format eventually chosen is a checklist composed of single- word items. Furthermore, the theoretical models presented earlier are more consistent with some response formats than with others. In general, scales made up of items that are scorable on some continuum and are summed to form a scale score are most compatible with the theoretical orientation presented in this volume. In this section, however, I will discuss common formats that depart from the pattern implied by the theoretical models discussed in Chapter 2 as well as ones that adhere to that pattern. Thurstone Scaling There are a number of general strategies for constructing scales that influence the format of items and response options. One method is Thurstone scaling. An analogy may help clarify how Thurstone scaling works. A tuning fork is designed to vibrate at a specific frequency. If you strike it, it will vibrate at that frequency and produce a specific tone. Conversely, if you place the fork near a tone source that produces the same frequency as the tuning fork, the fork will begin to vibrate. In a sense, then, a tuning fork is a “frequency detector,” vibrating in the presence of sound waves of its resonant frequency and remaining motionless in the presence of all other frequencies. Imagine a series of tuning forks aligned in an array such that, as one moves from left to right along the array, the tuning forks correspond to progressively higher frequency sounds. Within the range of the tuning forks’ frequency, this array can be used to identify the frequency of a tone. In other words, you could identify the tone’s frequency by seeing which fork vibrated when the tone was played. A Thurstone scale is intended to work in the same way. The scale developer attempts to generate items that are differentially responsive to specific levels of the attribute in question. When the “pitch” of a particular item matches the level of the attribute a respondent possesses, the item will signal this correspondence. Often, the signal consists of an affirmative response for items that are “tuned” to the appropriate level of the attribute and a negative response for all other items. The tuning (i.e., determination of what level of the construct each item responds to) is typically determined by having judges place a large pool of items into piles corresponding with equally spaced intervals of construct magnitude or strength. This is quite an elegant idea. Items could be developed to correspond with different intensities of the attribute, could be spaced to represent equal intervals, and could be formatted with agree-disagree response options, for example. The investigator could give these items to respondents and then inspect their responses to see which items triggered agreement. Because the items would have been precalibrated with respect to their sensitivity to specific levels of the phenomenon, the agreements would pinpoint how much of the 114 attribute the respondent possessed. The selection of items to represent equal intervals across items would result in highly desirable measurement properties because scores would be amenable to mathematical procedures based on interval scaling. Part of a hypothetical Thurstone scale for measuring parents’ aspirations for their children’s educational and career attainments might look like the following: As Nunnally (1978) points out, developing a true Thurstone scale is considerably harder than describing one. Finding items that consistently “resonate” to specific levels of the phenomenon is quite difficult. The practical problems associated with the method often outweigh its advantages unless the researcher has a compelling reason for wanting the type of calibration that it provides. Although Thurstone scaling is an interesting and sometimes suitable approach, it will not be referred to in the remainder of this text. Note, however, that methods based on item response theory, discussed in a later chapter, share many of the goals of Thurstone scales while taking a somewhat different approach to achieving them. Guttman Scaling A Guttman scale is a series of items tapping progressively higher levels of an attribute. Thus, a respondent should endorse a block of adjacent items until, at a critical point, the amount of the attribute that the items tap exceeds that possessed by the subject. None of the remaining items should be endorsed. Some purely descriptive data conform to a Guttman scale. For example, a series of interview questions might ask, “Do you smoke?” “Do you smoke more than 10 cigarettes a day?” “Do you smoke more than a pack a day?” and so on. As with this example, endorsing any specific item on a Guttman scale implies affirmation of all preceding items. A respondent’s level of the attribute is indicated by the highest item yielding an affirmative response. Note that, whereas both Thurstone and Guttman scales 115 are made up of graded items, the focus is on a single affirmative response in the former case but on the point of transition from affirmative to negative responses in the latter case. A Guttman version of the preceding parental aspiration scale might look like this: Guttman scales can work quite well for objective information or in situations where it is a logical necessity that responding positively to one level of a hierarchy implies satisfying the criteria of all lower levels of that hierarchy. Things get murkier when the phenomenon of interest is not concrete. In the case of our hypothetical parental aspiration scale, for example, the ordering may not be uniform across individuals. Whereas 20 cigarettes a day always implies more smoking than 10, responses to Items 3 and 4 in the parental aspiration scale example may not always conform to the ordering pattern of a Guttman scale. For example, a person might agree with Item 3 but disagree with Item 4. Ordinarily, agreement with Item 3 would imply agreement with Item 4, but if a respondent viewed success as a complex factor that acted simultaneously as a help and a hindrance to happiness, then an atypical pattern of responses could result. Like Thurstone scales, Guttman scales undoubtedly have their place, but their applicability seems rather limited. With both approaches, the disadvantages and difficulties will often outweigh the advantages. It is also important to reiterate that the measurement theories discussed thus far do not always apply to these types of scales. Certainly, the assumption of equally strong causal relationships between the latent variable and each of the items would not apply to Thurstone or Guttman scale items. Nunnally and Bernstein (1994) describe briefly some of the conceptual models underlying these scales. For situations in which ordered items are particularly appropriate, models based on item response theory (discussed in Chapter 7) are potentially an appropriate choice, although implementing these methods can be quite burdensome. 116 Scales With Equally Weighted Items The measurement models discussed earlier fit best with scales consisting of items that are more or less equivalent “detectors” of the phenomenon of interest—that is, they are more or less parallel (but not necessarily parallel in the strict sense of the parallel tests model). They are imperfect indicators of a common phenomenon that can be combined by simple summation into an acceptably reliable scale. One attractive feature of scales of this type is that the individual items can have a variety of response-option formats. This allows the scale developer a good deal of latitude in constructing a measure optimally suited for a particular purpose. Some general issues related to response formatting will be examined below, as will the merits and liabilities of some representative response formats. How Many Response Categories? Most scale items consist of two parts: a stem and a series of response options. For example, the stem of each item may be a different declarative statement expressing an opinion, and the response options accompanying each stem might be a series of descriptors indicating the strength of agreement with the statement. For now, let us focus on the response options —specifically, the number of choices that should be available to the respondent. Some item-response formats allow the subject an infinite or very large number of options, whereas others limit the possible responses. Imagine, for example, a response scale for measuring anger that resembles a thermometer, calibrated from “no anger at all” at the base of the thermometer to “complete, uncontrollable rage” at its top. A respondent could be presented with a series of situation descriptions, each accompanied by a copy of the thermometer scale, and asked to indicate, by shading in some portion of the thermometer, how much anger the situation provoked. This method allows for virtually continuous measurement of anger. An alternative method might ask the respondent to indicate, using a number from 1 to 100, how much anger each situation provoked. This provides for numerous discrete responses. Alternatively, the format could restrict the response options to a few choices, such as “none,” “a little,” “a moderate amount,” and “a lot,” or to a simple binary selection between “angry” and “not angry.” What are the relative advantages of these alternatives? A desirable quality of a measurement scale is variability. A measure cannot covary if it does not vary. If a scale fails to discriminate differences in the underlying attribute, its correlations with other measures will be restricted and its utility will be limited. One way to increase opportunities for variability is to include lots of scale items. Another is to provide numerous response options within items. If circumstances restrict an investigator to two questions regarding anger, for example, it might be best to allow respondents more latitude in describing their level of anger. Assume that the research concerns the enforcement of nonsmoking policies in a 117 work setting. Let us further assume that the investigators want to determine the relationship between policy and anger. If they were limited to only two questions (e.g., “How much anger do you feel when you are restricted from smoking?” and “How much anger do you feel when you are exposed to others smoking in the workplace?”), they might get more useful information from a response format that allowed subjects many gradations of response than from a binary response format (e.g., “angry” and “not angry”). For example, a 0-to-100 scale might reveal wide differences in reactions to these situations and yield good variability for the two-item scale. On the other hand, if the research team were allowed to include 50 questions about smoking and anger, simple “angry” versus “not angry” indications might yield sufficient variability when the items were added to obtain a scale score. In fact, being faced with more response options on each of 50 questions might fatigue or bore the respondents, lowering the reliability of their responses. Another issue related to the number of response options is the respondents’ ability to discriminate meaningfully. How fine a distinction can the typical subject make? This obviously depends on what is being measured. Few things can truly be evaluated into, say, 50 discrete categories. Presented with this many options, many respondents may use only those corresponding to multiples of 5 or 10, effectively reducing the number of options to as few as five. Differences between a response of 35 and 37 may not reflect actual difference in the phenomenon being measured. Little is gained with this sort of false precision. Although the scale’s variance might increase, it may be the random (i.e., error) portion rather than the systematic portion attributable to the underlying phenomenon that is increasing. This, of course, offers no benefit. Sometimes, the respondent’s ability to discriminate meaningfully between response options will depend on the specific wording or physical placement of those options. Asking a respondent to discriminate among vague quantity descriptors, such as “several,” “few,” and “many,” may create problems. Sometimes, the ambiguity can be reduced by the arrangement of the response options on the page. Respondents often seem to understand what is desired when they are presented with an obvious continuum. Thus, an ordering such as Many Some Few Very Few None may imply that “some” is more than “few” because of the ordering of these items. However, if it is possible to find a nonambiguous adjective that precludes the respondents’ making assumptions based on location along a continuum, so much the better. At times, it may be preferable to have fewer response options than to have ones that are ambiguous. So, for example, it may be better in the above example to eliminate either “some” or “few” and have four options rather than five. The worst circumstance is to combine ambiguous words with ambiguous page locations. Consider the following example: Very Helpful Not Very Helpful 118 Somewhat Helpful Not at All Helpful Terms such as somewhat and not very are difficult to differentiate under the best of circumstances. However, arranging these response options as they appear above makes matters even worse. If a respondent reads down the first column and then down the second, “somewhat” appears to represent a higher value than “not very.” But if a respondent reads across the first row and then across the second, the implicit ordering of these two descriptors along the continuum is reversed. Due to ambiguity in both language and spatial arrangement, individuals may assign different meanings to the two options representing moderate values, and reliability would suffer as a consequence. Still another issue is the investigator’s ability and willingness to record a large number of values for each item. If the thermometer method described earlier is used to quantify anger responses, is the researcher actually going to attempt a precise scoring of each response? How much precision is appropriate? Can the shaded area be measured to within a quarter of an inch? A centimeter? A millimeter? If only some crude datum—say lower, middle, or upper third—is extracted from the scale, what was the point in requesting such a precise response? There is at least one more issue related to the number of responses. Assuming that a few discrete responses are allowed for each item, should the number be odd or even? Again, this depends on the type of question, the type of response option, and the investigator’s purpose. If the response options are bipolar, with one extreme indicating the opposite of the other (e.g., a strong positive vs. a strong negative attitude), an odd number of responses permits equivocation (e.g., “neither agree nor disagree”) or uncertainty (e.g., “not sure”); an even number usually does not. An odd number implies a central “neutral” point (e.g., neither a positive nor a negative appraisal). An even number of responses, on the other hand, forces the respondent to make at least a weak commitment in the direction of one or the other extreme (e.g., a forced choice between a mildly positive or mildly negative appraisal as the least extreme response). Neither format is necessarily superior. The researcher may want to preclude equivocation if it is felt that subjects will select a neutral response as a means of avoiding a choice. In studies of social comparison choices, for example, the investigators may want to force subjects to express a preference for information about a more advantaged or less advantaged person. Consider these two alternative formats, the first of which was chosen for a study of social comparisons among people with arthritis (DeVellis et al., 1990): 1. Would you prefer information about: (a) Patients who have worse arthritis than you have (b) Patients who have milder arthritis than you have 2. Would you prefer information about: (a) Patients who have worse arthritis than you have (b) Patients who have arthritis equally as bad as you have 119 (c) Patients who have milder arthritis than you have A neutral option such as 2b might permit unwanted equivocation. A neutral point may also be desirable. In a study assessing which of two risks (e.g., boredom vs. danger) people prefer taking, a midpoint may be crucial. The researcher might vary the chance or severity of harm across several choices between a safe, dull activity and an exciting, risky one. The point at which a respondent is most nearly equivocal about risking the more exciting activity could then be used as an index of risk taking: Indicate your relative preference for Activity A or Activity B from the alternatives listed below by circling the appropriate phrase following the description of Activity B. Activity A: Reading a statistics book (no chance of severe injury) 1. Activity B: Taking a flight in a small commuter plane (very slight chance of severe injury) 2. Activity B: Taking a flight in a small open-cockpit plane (slight chance of severe injury) 3. Activity B: Parachute jumping from a plane with a backup chute (moderate chance of severe injury) 4. Activity B: Parachute jumping from a plane without a backup chute (substantial risk of severe injury) 5. Activity B: Jumping from a plane without a parachute and attempting to land on a soft target (almost certain severe injury) 120 The other merits or liabilities of this approach aside, it would clearly require that response options include a midpoint. Specific Types of Response Formats Scale items occur in a dizzying variety of forms. However, there are several ways to present items that are used widely and have proven successful in diverse applications. Some of these are discussed below. Likert Scale One of the most common item formats is a Likert scale. When a Likert scale is used, the item is presented as a declarative sentence, followed by response options that indicate varying degrees of agreement with or endorsement of the statement. (In fact, the preceding example of risk taking used a Likert response format.) Depending on the phenomenon being investigated and the goals of the investigator, either an odd or even number of response options might accompany each statement. The response options should be worded so as to have roughly equal intervals with respect to agreement. That is to say, the difference in agreement between any adjacent pair of responses should be about the same as for any other adjacent pair of response options. A common practice is to include six possible responses: “strongly disagree,” “moderately disagree,” “mildly disagree,” “mildly agree,” “moderately agree,” and “strongly agree.” These form a continuum from strong disagreement to strong agreement. A neutral midpoint can also be added. Common choices for a midpoint include “neither agree nor disagree” and “agree and disagree equally.” There is legitimate room for discussion concerning the equivalence of these two midpoints. The first implies apathetic disinterest, while the latter suggests strong but equal attraction to both agreement and disagreement. It may very well be that most respondents do not focus much attention on subtleties of language but merely regard any reasonable response option in the center of the range as a midpoint, irrespective of its precise wording. Likert scaling is widely used in instruments measuring opinions, beliefs, and attitudes. It is often useful for these statements to be fairly (though not extremely) strong when used in a Likert format. Presumably, the moderation of opinion is expressed in the choice of response option. For example, the statements “Physicians generally ignore what patients say,” “Sometimes, physicians do not pay as much attention as they should to patients’ comments,” and “Once in a while, physicians might forget or miss something a patient has told them” express strong, moderate, and weak opinions, respectively, concerning physicians’ inattention to patients’ remarks. Which is best for a Likert scale? Ultimately, of course, the one that most accurately reflects true differences of opinion is best. In choosing how strongly to word items in an initial item pool, the investigator might profitably ask, “How are people with different amounts or strengths of the attribute in question likely to respond?” In the case of the three examples just presented, the investigator might conclude 121 that the last question would probably elicit strong agreement from people whose opinions fell along much of the continuum from positive to negative. If this conclusion proved correct, then the third statement would not do a good job of differentiating between people with strong versus moderate negative opinions. In general, very mild statements may elicit too much agreement when used in Likert scales. Many people will strongly agree with such a statement as “The safety and security of citizens is important.” One could strongly agree with such a statement (i.e., choose an extreme response option) without holding an extreme opinion. Of course, the opposite is equally true. People holding any but the most extreme views might find themselves in disagreement with an extremely strong statement (e.g., “Hunting down and punishing wrongdoers is more important than protecting the rights of individuals”). Of the two (overly mild or overly extreme) statements, the former may be the bigger problem for two reasons. First, our inclination is often to write statements that will not offend our subjects. Avoiding offensiveness is probably a good idea; however, it may lead us to favor items that nearly everyone will find agreeable. Another reason to be wary of items that are too mild is that they may represent the absence of belief or opinion. The third of our inattentive physician items in the preceding paragraph did not indicate the presence of a favorable attitude so much as the absence of an unfavorable one. Items of this sort may be poorly suited to the research goal because we are more often interested in the presence of some phenomenon than in its absence. A useful way of calibrating how strongly or mildly a statement should be worded is to do the following: imagine the typical respondent for whom the scale is intended. Try to imagine how that person would respond to items of different forcefulness. Now, think about what sort of item wording would be most likely to elicit a response from that typical respondent that was at or near the center of the Likert scale response options you plan to use. So, for example, if you had chosen a 6-point scale that had slightly disagree and slightly agree as the centermost response options, you would want to create an item that would elicit one of those responses from a typical respondent in the population of interest. Such an item should be able to accommodate people whose views are either less or more strong than the typical or average respondent and thus theoretically should have substantial observed and true score variance. By virtue of its variance across respondents, the item will have a better chance of correlating well with other items (because covariation is tied to the extent of variation) and thus will have the potential to enhance scale reliability. In contrast, an item that is likely to produce extreme responses, such as strongly disagree or strongly agree, from the typical respondent would do a poor job of discriminating across the full spectrum of respondents. In summary, a good Likert item should state the opinion, attitude, belief, or other construct under study in clear terms. It is neither necessary nor appropriate for this type of scale to span the range of weak to strong assertions of the construct. The response options provide the opportunity for gradations. 122 The following are examples of items in Likert response formats: 1. Exercise is an essential component of a healthy lifestyle. 2. Combating drug abuse should be a top national priority. Semantic Differential The semantic differential scaling method is chiefly associated with the attitude research of Osgood and his colleagues (e.g., Osgood & Tannenbaum, 1955). Typically, a semantic differential is used in reference to one or more stimuli. In the case of attitudes, for example, the stimulus might be a group of people, such as automobile salesmen. Identification of the target stimulus is followed by a list of adjective pairs. Each pair represents opposite ends of a continuum, defined by adjectives (e.g., honest and dishonest). As shown in the example below, there are several lines between the adjectives that constitute the response options: In essence, the individual lines (seven and nine are common numbers) represent points along the continuum defined by the adjectives. The respondent places a mark on one of the lines to indicate the point along the continuum that characterizes his or her evaluation of the stimulus. For example, if someone regarded auto salesmen as extremely dishonest, he or she might select the line closest to that adjective. Either extreme or moderate views can be expressed by choosing which line to mark. After rating the stimulus with regard to the first adjective pair, the person would proceed to additional adjective pairs separated by lines. The adjectives one chooses can be either bipolar or unipolar, depending, as always, on the logic of the research questions the scale is intended to address. Bipolar adjectives each express the presence of opposite attributes, such as friendly and hostile. Unipolar adjective 123 pairs indicate the presence and absence of a single attribute, such as friendly and not friendly. Like the Likert scale, the semantic differential response format can be highly compatible with the theoretical models presented in the earlier chapters of this book. Sets of items can be written to tap the same underlying variable. For example, items using trustworthy/untrustworthy, fair/unfair, and truthful/untruthful as endpoints might be added to the first statement in the preceding example to constitute an “honesty” scale. Such a scale could be conceptualized as a set of items sharing a common latent variable (honesty) and conforming to the assumptions discussed in Chapter 2. Accordingly, the scores of the individual “honesty” items could be added and analyzed as described in a later section concerning the evaluation of items. Visual Analog Another item format that is in some ways similar to the semantic differential is the visual analog scale. This response format presents the respondent with a continuous line between a pair of descriptors representing opposite ends of a continuum. The individual completing the item is instructed to place a mark at a point on the line that represents his or her opinion, experience, belief, or whatever is being measured. The visual analog scale, as the term analog in the name implies, is a continuous scale. The fineness of differentiation in assigning scores to points on the scale is determined by the investigator. Some of the advantages and disadvantages of a continuous-response format were discussed earlier. An additional issue not raised at that time concerns possible differences in the interpretation of physical space as it relates to values on the continuum. A mark placed at a specific point along the line may not mean the same thing to different people, even when the end points of the line are identically labeled for all respondents. Consider a visual analog scale for pain such as this: Does a response in the middle of the scale indicate pain about half of the time, constant pain of half the possible intensity, or something else entirely? Part of the problem with measuring pain is that it can be evaluated on multiple dimensions, including frequency, intensity, and duration. Also, recollections of the worst pain a given person has ever experienced are likely to be distorted. Comparisons across individuals are further complicated by the fact that different people may have experienced different levels of “the worst pain.” Of course, some of these problems reside with the phenomenon used in this example—pain (see Keefe, 2000, for an excellent discussion of pain measurement)—and not with the scale per se. However, the problem of idiosyncratic assignment of values along a visual analog scale can exist for other phenomena as well. 124 A major advantage of visual analog scales is that they are potentially very sensitive (Mayer, 1978). This can make them especially useful for measuring phenomena before and after some intervening event, such as an intervention or experimental manipulation, that exerts a relatively weak effect. A mild rebuke in the course of an experimental manipulation, for example, may not produce a shift on a 5-point measure of self-esteem. However, a subtle but systematic shift to lower values on a visual analog scale might occur among people in the “rebuke” condition of this hypothetical experiment. Sensitivity may be more advantageous when examining changes over time within the same individual rather than across individuals (Mayer, 1978). This may be so because, in the former case, there is no additional error due to extraneous differences between individuals. Another potential advantage of visual analog scales when they are repeated over time is that it is difficult or impossible for subjects to encode their past responses with precision. To continue with the example from the preceding paragraph, a subject would probably have little difficulty remembering which of five numbered options to a self-esteem item he or she had previously chosen in response to a multiresponse format such as a Likert scale. Unless one of the end points of a visual analog scale were chosen, however, it would be difficult to recall precisely where a mark had been made along a featureless line. This could be advantageous if the investigator were concerned that respondents might be biased to appear consistent over time. Presumably, subjects motivated to be consistent would choose the same response after exposure to an experimental intervention as prior to such exposure. The visual analog format essentially rules out this possibility. If the post-manipulation responses departed consistently (i.e., usually in the same direction) from the premanipulation response for experimental subjects and randomly for controls, then the choice of a visual analog scale might have contributed to detecting a subtle phenomenon that other methods would have missed. Visual analog scales have often been used as single-item measures. This has the sizable disadvantage of precluding any determination of internal consistency. With a single-item measure, reliability can be determined only by the test-retest method described in Chapter 3 or by comparison with other measures of the same attribute having established psychometric properties. The former method suffers from the problems of test-retest assessments discussed earlier, notably the impossibility of differentiating instability of the measurement process from instability of the phenomenon being measured. The latter method is actually a construct validity comparison. However, because reliability is a necessary condition for validity, one can infer the reliability if validity is in evidence. Nonetheless, a better strategy may be to develop multiple visual analog items so that internal consistency can be determined. Numerical Response Formats and Basic Neural Processes A study by Zorzi, Priftis, and Umilitá (2002) appearing in Nature suggests that certain response options may correspond to how the brain processes numerical information. 125 According to these authors, numbers arrayed in a sequence, as with the typical Likert scale, express quantity not only in their numerical values but in their locations. They suggest that the visual line of numbers is not merely a convenient representation but corresponds to fundamental neural processes. They observed that people with various brain lesions that impair spatial perception in the visual field make systematic errors in simple, visually presented mathematical problems. The spatial anomaly and the type of errors are closely linked. Individuals who could not perceive the left visual field, when asked to indicate the midpoint between two values presented in a linear array, consistently erred “to the right.” For example, when individuals were asked what would be midway between points labeled “3” and “9,” errors were shifted to the right (i.e., to higher values). Reversing the scale from high to low continued to produce shifts to the right (now, lower values). When the same tasks were presented in nonvisual form (e.g., by asking what the average of 3 and 9 was), the pattern did not appear. In fact, these individuals showed no deficit in performing arithmetic when it was not presented visually. Control subjects without the visual anomaly did not show the shift pattern of those with brain lesions. The authors conclude that their work constitutes “strong evidence that the mental number line is more than simply a metaphor” and that “thinking of numbers in spatial terms (as has been reported by great mathematicians) may be more efficient because it is grounded in the actual neural representation of numbers” (p. 138). Although this study, by itself, may not warrant hard- and-fast conclusions, it provides tantalizing preliminary evidence that evaluating a linear string of numbers may correspond to fundamental neural mechanisms involved in assessing quantity. If this is truly the case, then response options presented as a row of numbers may have special merit. Binary Options Another common response format gives subjects a choice between binary options for each item. The earlier examples of Thurstone and Guttman scales used binary options (“agree” and “disagree”), although scales with equally weighted items could also have binary response options. Subjects might, for example, be asked to check off all the adjectives on a list that they think apply to themselves. Or they may be asked to answer “yes” or “no” to a list of emotional reactions they may have experienced in some specified situation. In both cases, responses reflecting items sharing a common latent variable (e.g., adjectives such as “sad,” “unhappy,” and “blue” representing depression) could be combined into a single score for that construct. A major shortcoming of binary responses is that each item can have only minimal variability. Similarly, any pair of items can have only one of two levels of covariation: agreement or disagreement. Recall from Chapter 3 that the variance of a scale made up of multiple equally weighted items is exactly equal to the sum of all the elements in the covariance matrix for the individual items. With binary items, each item contributes precious little to that sum because of the limitations in possible variances and covariances. 126 The practical consequence of this is that more items are needed to obtain the same degree of scale variance if the items are binary. However, binary items are usually extremely easy to answer. Therefore, the burden placed on the subject is low for any one item. For example, most people can quickly decide whether certain adjectives are apt descriptions of themselves. As a result, subjects often are willing to complete more binary items than ones using a format demanding concentration on finer distinctions. Thus, a binary format may allow the investigator to achieve adequate variation in scale scores by aggregating information over more items. Item Time Frames Another issue that pertains to the formatting of items is the specified or implied time frame. Kelly and McGrath (1988), in another volume in this series, discuss the importance of considering the temporal features of different measures. Some scales will not make reference to a time frame, implying a universal time perspective. Locus of control scales, for example, often contain items that imply an enduring belief in causality. Items such as “If I take the right actions, I can stay healthy” (Wallston et al., 1978) presume that this belief is relatively stable. This is consistent with the theoretical characterization of locus of control as a generalized rather than specific expectancy for control over outcomes (although there has been a shift toward greater specificity in later measures of locus of control beliefs—e.g., DeVellis et al., 1985). Other measures assess relatively transient phenomena. Depression, for example, can vary over time, and scales to measure it have acknowledged this point (Mayer, 1978). For example, the widely used Center for Epidemiological Studies Depression Scale (Radloff, 1977) uses a format that asks respondents to indicate how often during the past week they experienced various mood states. Some measures, such as anxiety scales (e.g., Spielberger, Gorsuch, & Lushene, 1970), are developed in different forms intended to assess relatively transient states or relatively enduring traits (Zuckerman, 1983). The investigator should choose a time frame for a scale actively rather than passively. Theory is an important guide to this process. Is the phenomenon of interest a fundamental and enduring aspect of individuals’ personalities, or is it likely to be dependent on changing circumstances? Is the scale intended to detect subtle variations occurring over a brief time frame (e.g., increases in negative affect after viewing a sad movie) or changes that may evolve over a lifetime (e.g., progressive political conservatism with increasing age)? In conclusion, the item formats, including response options and instructions, should reflect the nature of the latent variable of interest and the intended uses of the scale. Step 4: Have Initial Item Pool Reviewed by Experts Thus far, we have discussed the need for clearly articulating what the phenomenon of interest is, generating a pool of suitable items, and selecting a response format for those items. The next step in the process is having a group of people who are knowledgeable in 127 the content area review the item pool. This review serves multiple purposes related to maximizing the content validity (see Chapter 4) of the scale. First, having experts review your item pool can confirm or invalidate your definition of the phenomenon. You can ask your panel of experts (e.g., colleagues who have worked extensively with the construct in question or related phenomena) to rate how relevant they think each item is to what you intend to measure. This is especially useful if you are developing a measure that will consist of separate scales to measure multiple constructs. If you have been careful in developing your items, then experts should have little trouble determining which items correspond to which constructs. In essence, your thoughts about what each item measures are the hypothesis, and the responses of the experts are the confirming or disconfirming data. Even if all the items are intended to tap a single attribute or construct, expert review is useful. If experts read something into an item you did not plan to include, subjects completing a final scale might do likewise. The mechanics of obtaining evaluations of item relevance usually involve providing the expert panel with your working definition of the construct. They are then asked to rate each item with respect to its relevance vis-à-vis the construct as you have defined it. This might entail merely rating relevance as high, moderate, or low for each item. In addition, you might invite your experts to comment on individual items as they see fit. This makes their job a bit more difficult but can yield excellent information. A few insightful comments about why certain items are ambiguous, for example, might give you a new perspective on how you have attempted to measure the construct. Reviewers also can evaluate the items’ clarity and conciseness. The content of an item may be relevant to the construct, but its wording may be problematic. This bears on item reliability because an ambiguous or otherwise unclear item, to a greater degree than a clear item, can reflect factors extraneous to the latent variable. In your instructions to reviewers, ask them to point out awkward or confusing items and suggest alternative wordings, if they are so inclined. A third service that your expert reviewers can provide is pointing out ways of tapping the phenomenon that you have failed to include. There may be a whole approach that you have overlooked. For example, you may have included many items referring to illness in a pool of items concerned with health beliefs but failed to consider injury as another relevant departure from health. By reviewing the variety of ways you have captured the phenomenon of interest, your reviewers can help you maximize the content validity of your scale. A final word of caution concerning expert opinion: The final decision to accept or reject the advice of your experts is your responsibility as the scale developer. Sometimes, content experts might not understand the principles of scale construction. This can lead to bad advice. A recommendation I have frequently encountered from colleagues without scale 128 development experience is to eliminate items that concern the same thing. As discussed earlier, removing all redundancy from an item pool or a final scale would be a grave error because redundancy is an integral aspect of internal consistency. However, this comment might indicate that the wording, vocabulary, and sentence structure of the items are too similar and could be improved. Pay careful attention to all the suggestions you receive from content experts. Then make your own informed decisions about how to use their advice. At this point in the process, the scale developer has a set of items that has been reviewed by experts and modified accordingly. It is now time to advance to the next step. Step 5: Consider Inclusion of Validation Items Obviously, the heart of the scale development questionnaire is the set of items from which the scale under development will emerge. However, some foresight can pay off handsomely. It might be possible and relatively convenient to include some additional items in the same questionnaire that will help in determining the validity of the final scale. There are at least two types of items to consider. The first type of item a scale developer might choose to include in a questionnaire serves to detect flaws or problems. Respondents might not be answering the items of primary interest for the reasons you assume. There may be other motivations influencing their responses. Learning this early is advantageous. One type of motivation that can be assessed fairly easily is social desirability. If an individual is strongly motivated to present herself or himself in a way that society regards as positive, item responses may be distorted. Including a social desirability scale allows the investigator to assess how strongly individual items are influenced by social desirability. Items that correlate substantially with the social desirability score obtained should be considered as candidates for exclusion unless there is a sound theoretical reason that indicates otherwise. A brief and useful social desirability scale has been developed by Strahan and Gerbasi (1972). This 10-item measure can be conveniently inserted into a questionnaire. There are other sources of items for detecting undesirable response tendencies (Anastasi, 1968). The Minnesota Multiphasic Personality Inventory (Hathaway & McKinley, 1967; Hathaway & Meehl, 1951) includes several scales aimed at detecting various response biases. In some instances, it may be appropriate to include these types of scales. The other class of items to consider including at this stage pertain to the construct validity of the scale. As discussed in Chapter 4, if theory asserts that the phenomenon you are setting out to measure relates to other constructs, then the performance of the scale vis-à-vis measures of those other constructs can serve as evidence of its validity. Rather than mounting a separate validation effort after constituting the final scale, it may be possible to include measures of relevant constructs at this stage. The resultant pattern of relationships can provide support for claims of validity or, alternatively, provide clues if the set of items 129 does not perform as anticipated. Step 6: Administer Items to a Development Sample After deciding which construct-related and validity items to include in your questionnaire, you must administer them, along with the pool of new items, to some subjects. The sample of subjects should be large. How large is large? It is difficult to find a consensus on this issue. Let us examine the rationale for a large sample. Nunnally (1978) points out that the primary sampling issue in scale development involves the sampling of items from a hypothetical universe (cf. Ghiselli, Campbell, & Zedeck, 1981). In order to concentrate on the adequacy of the items, the sample should be sufficiently large to eliminate subject variance as a significant concern. Nunnally suggests that 300 people is an adequate number. However, practical experience suggests that scales have been successfully developed with smaller samples. The number of items and the number of scales to be extracted also have a bearing on the sample size issue. If only a single scale is to be extracted from a pool of about 20 items, fewer than 300 subjects might suffice. There are several risks in using too few subjects. First, the patterns of covariation among the items may not be stable. An item that appears to increase internal consistency may turn out to be a dud when it is used on a separate sample. If items are selected for inclusion (as they very well may be) on the basis of their contribution to alpha, having a small developmental sample can paint an inaccurately rosy picture of internal consistency. When the ratio of subjects to items is relatively low and the sample size is not large, the correlations among items can be influenced by chance to a fairly substantial degree. When a scale whose items were selected under these conditions is readministered, the chance factors that made certain items look good initially are no longer operative. Consequently, the alpha obtained on occasions other than the initial development study may be lower than expected. Similarly, a potentially good item may be excluded because its correlation with other items was attenuated purely by chance. A second potential pitfall of small sample size is that the development sample may not represent the population for which the scale is intended. Of course, this can also be the case if the development sample is large, but a small sample is even more likely to exclude certain types of individuals. Thus, a scale developer should consider both the size and composition of the development sample. A careful investigator might choose to address the generalizability of a scale across populations (or some other facet) with a G-study, as discussed in Chapter 3. Not all types of nonrepresentativeness are identical. There are at least two different ways in which a sample may not be representative of the larger population. The first involves the level of attribute present in the sample versus the intended population. For example, a sample might represent a narrower range of the attribute than would be expected of the 130 population. This constriction of range may also be asymmetrical so that the mean score obtained on the scale for the sample is appreciably higher or lower than one would expect for the population. Opinions regarding the appropriate legal drinking age, for example, might very well differ on a college campus from opinions on the same topic in a community at large. A mean value of the attribute that is not representative does not necessarily disqualify the sample for purposes of scale development. It may yield inaccurate expectations for scale means while still providing an accurate picture of the internal consistency the scale possesses. For example, a sample of this sort might still lead to correct conclusions about which items are most strongly interrelated. A more troublesome type of nonrepresentativeness involves a sample that is qualitatively rather than quantitatively different from the target population. Specifically, a sample in which the relationships among items or constructs may differ from the population is reason for concern. If a sample is quite unusual, items may have a different meaning for them than for people in general. The patterns of association among items might reflect unusual attributes shared among sample members but rare in the broader community. In other words, the groupings of interrelated items that emerge (e.g., from a factor analysis) may be atypical. Stated a bit more formally, the underlying causal structure relating variables to true scores may be different if a sample is unlike the population in important ways. Consider some rather obvious examples: If the members of the chosen sample do not understand a key word that recurs among the items and has relevance to the construct, their responses may tell little or nothing about how the scale would perform under different circumstances. The word sick means “ill” in the United States but “nauseated” (i.e., sick to one’s stomach) in England. A set of questions about illness developed for one group may have a markedly different meaning for the other. If the scale concerns a specific health problem not usually associated with nausea (e.g., arthritis), items that use the word ill might cluster together because of their distinct meaning if the sample were British. An American sample, on the other hand, would be unlikely to differentiate statements about being ill from other health-related items. Even within the United States, the same word can have different meanings. Among rural Southerners, for example, “bad blood” is sometimes used as a euphemism for venereal disease, whereas in other parts of the country it means animosity. If an item discussing “bad blood between relatives” performed differently among a sample of rural Southerners versus other samples, it would hardly be surprising. The consequences of this second type of sample nonrepresentativeness can severely harm a scale development effort. The underlying structure that emerges—the patterns of covariation among items that are so important to issues of scale reliability—may be a quirk of the sample used in development. If a researcher has reason to believe that the meaning ascribed to items may be atypical among a development sample, great caution should be used in interpreting the findings obtained from that sample. Step 7: Evaluate the Items 131 After an initial pool of items has been developed, scrutinized, and administered to an appropriately large and representative sample, it is time to evaluate the performance of the individual items so that appropriate ones can be identified to constitute the scale. This is, in many ways, the heart of the scale development process. Item evaluation is second perhaps only to item development in its importance. Initial Examination of Items’ Performance When discussing item development, we referred to some of the qualities that are desirable in a scale item. Let us reconsider that issue. The ultimate quality we seek in an item is a high correlation with the true score of the latent variable. This follows directly from the discussion of reliability in Chapter 3. We cannot directly assess the true score (if we could, we probably would not need a scale) and, thus, cannot directly compute its correlations with items. However, we can make inferences based on the formal measurement models that have been discussed thus far. When discussing parallel tests in Chapter 2, I noted that the correlation between any two items equals the square of the correlation between either item and the true score. This squared value is the reliability of each of the items. So, we can learn about relationships to true scores from correlations among items. The higher the correlations among items are, the higher the individual item reliabilities are (i.e., the more intimately they are related to the true score). The more reliable the individual items are, the more reliable the scale that they compose will be (assuming that they share a common latent variable). So, the first quality we seek in a set of scale items is that they be highly intercorrelated. One way to determine how intercorrelated the items are is to inspect the correlation matrix. Reverse Scoring If there are items whose correlations with other items are negative, then the appropriateness of reverse scoring those items should be considered. Earlier, I suggested that items worded in opposite directions can pose problems. Sometimes, however, we may inadvertently end up with negatively correlated items. This might happen, for example, if we initially anticipated two separate groups of items (e.g., pertaining to happiness and sadness) but decide for some reason that they should be combined into a single group. We could then wind up with statements that relate equally to the new, combined construct (e.g., affect), but some may be positive and others negative. “I am happy” and “I am sad” both pertain to affect; however, they are opposites. If we wanted high scores on our scale to measure happiness, then we would have to ascribe a high value to endorsing the “happy” item but a low value to endorsing the “sad” item. That is to say, we would reverse score the sadness item. Sometimes, items are administered in such a way that they are already reversed. For example, subjects might be asked to circle higher numerical values to indicate agreement with a “happy” item and lower values to endorse a “sad” one. One way to do this is by having the verbal descriptors for the response options (e.g., “strongly disagree,” “moderately 132 disagree,” etc.) always be in the same order for all items but having the numbers associated with them either ascend or descend, depending on the item: 1. I am sad often. 2. Much of the time, I am happy. This process may confuse the subject. People may ignore the words after realizing that they are the same for all items. However, it is probably preferable to altering the order of the descriptors (e.g., from “strongly disagree” to “strongly agree” from left to right for some items and the reverse for others). Another option is to have both the verbal descriptions and their corresponding numbers the same for all items but to enter different values for certain items at the time of data coding. Changing scores for certain items at the time of coding is both tedious and potentially error prone. For every subject, every item to be reverse scored must be given the special attention involved in reverse scoring. This creates numerous opportunities for mistakes. The easiest method for reverse scoring is to do so electronically once the data have been entered into a computer. A few computer statements can handle all the reverse scoring for all subjects’ data. If the response options have numerical values and the desired transformation is to reverse the order of values, a simple formula can be used. For example, assume that a set of mood items formatted using a Likert scale was scored from 1 to 7, with higher numbers indicating agreement. Assume further that, for ease of comprehension, both positive mood and negative mood items used this same response format. However, if endorsing positive mood items is assigned a high score, then the scale is essentially a positive mood scale. Endorsing a positive mood item should result in a high value, and endorsing a negative mood item should yield a low value. This is what would be obtained if, for all negative mood items, responses of 7 were changed to 1, 6 to 2, and so forth. This type of transformation can be accomplished by creating a new score from the old score with the following formula: NEW = (J + 1 1) − OLD, where NEW and OLD refer to the transformed and original scores, respectively, and J is the original number of response options. In the example presented, J would equal 7 and (J + 1) would be 8. Subtracting a score of 7 from 8 would yield 1, subtracting 6 would yield 2, and so forth. Some negative correlations among items may not be correctable by reverse scoring items. 133 For example, reverse scoring a given item might eliminate some negative correlations but create others. This usually indicates that some of the items simply do not belong because they are not consistently related to other items. Any item that is positively correlated with some and negatively correlated with others in a homogeneous set should be eliminated if no pattern of reverse scoring items eliminates the negative correlations. Item-Scale Correlations If we want to arrive at a set of highly intercorrelated items, then each individual item should correlate substantially with the collection of remaining items. We can examine this property for each item by computing its item-scale correlation. There are two types of item- scale correlation. The corrected item-scale correlation correlates the item being evaluated with all the scale items, excluding itself, while the uncorrected item-scale correlation correlates the item in question with the entire set of candidate items, including itself. If there were 10 items being considered for a scale, the corrected item-scale correlation for any one of the 10 items would consist of its correlation with the other 9. The uncorrected correlation would consist of its correlation with all 10. In theory, the uncorrected value tells us how representative the item is of the whole scale. This is analogous, for example, to correlating one subset of an IQ test with the entire test to determine if the subscale is a suitable proxy. However, although an uncorrected item-total correlation makes good conceptual sense, the reality is that the item’s inclusion in the scale can inflate the correlation coefficient. The fewer the number of items in the set, the bigger the difference the inclusion or exclusion of the item under scrutiny will make. In general, it is probably advisable to examine the corrected item-total correlation. An item with a high value for this correlation is more desirable than an item with a low value. Item Variances Another valuable attribute for a scale item is relatively high variance. To take an extreme case, if all individuals answer a given item identically, it will not discriminate at all among individuals with different levels of the construct being measured and its variance will be 0. In contrast, if the development sample is diverse with respect to the attribute of interest, then the range of scores obtained for an item should be diverse as well. This implies a fairly high variance. Of course, increasing variance by adding to the error component is not desirable. Comparing item variances may also be useful, especially if the goal is to develop a tool that meets the assumptions of essential tau equivalence. Although essential tau equivalence does not assume equal item variances, it does assume equal covariances across items with the true score. Thus, when the assumptions of this model are satisfied, differences in item variances are due to inconsistency in item error variances. As a practical consideration, markedly different item variances may be undesirable, because they probably signify either substantially different sources of error from item to item or a failure to meet the 134 requirement for equal item-to-true-score covariances required by the essentially tau- equivalent model. Of course, this would not apply if the model guiding development is the congeneric model, which does not require anything beyond items sharing a common latent variable. Item Means A mean close to the center of the range of possible scores is also desirable. If, for example, the response options for each item ranged from 1 (corresponding with “strongly disagree”) to 7 (for “strongly agree”), an item mean near 4 would be ideal. If a mean were near one of the extremes of the range, then the item might fail to detect certain values of the construct. A piling up of scores at the value 7, for example, would suggest that the item was not worded strongly enough (i.e., that it was rare to find anyone who would disagree with it). Generally, items with means too near to an extreme of the response range will have low variances, and those that vary over a narrow range will correlate poorly with other items. As stated previously, an item that does not vary cannot covary. Thus, either a lopsided mean or a low variance for any reason will tend to reduce an item’s correlation with other items. As a result, you can usually concentrate primarily on the pattern of correlations among items as a gauge of their potential value. Inspecting means and variances, however, is a useful double-check once a tentative selection of items has been made on the basis of the correlations. Dimensionality A set of items is not necessarily a scale. Items may have no common underlying variable (as in an index or emergent variable) or may have several. Determining the nature of latent variables underlying an item set is critical. For example, an assumption underlying alpha is that the set of items is unidimensional. The best means of determining which groups of items, if any, constitute a unidimensional set is by factor analysis. This topic is sufficiently important to merit an entire chapter (see Chapter 6). Although factor analysis requires substantial sample sizes, so does scale development in general. If there are too few respondents for factor analysis, the entire scale development process may be compromised. Consequently, factor analysis of some sort should generally be a part of the scale development process at this stage. Reliability One of the most important indicators of a scale’s quality is the reliability coefficient, alpha. Virtually all the individual-item problems discussed thus far—a noncentral mean, poor variability, negative correlations among items, low item-scale correlations, and weak inter- item correlations—will tend to