Experimental Studies Lecture 6.3 PDF
Document Details
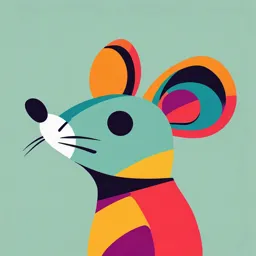
Uploaded by YouthfulAnaphora
Dr. Muhammad Ahmed Alshyyab
Tags
Related
- Experimental Studies PDF
- Topnotch Medical Board Prep Preventive Medicine and Public Health PDF (April 2024)
- Lecture 1: Course Introduction - Epidemiology and Biostatistics (BMS222) - PDF
- Epidemiologic Studies PDF
- Epidemiological Study Designs Lecture Notes PDF
- Experimental Studies (Lecture 8) - Zaroa University
Summary
This document presents a lecture on experimental studies, focusing on key messages, objectives, confounding factors, and control methods. The lecture notes include discussions about randomization, comparability, recruitment criteria, and follow-up procedures.
Full Transcript
Experimental Studies Dr. Muhammad Ahmed Alshyyab Lecture 3 Chapter 6 LEARNING OBJECTIVES: In this lecture, you will be able to demonstrate the following key massages: Epidemiologists must consider all sources of errors, and strive to reduce them. Epi...
Experimental Studies Dr. Muhammad Ahmed Alshyyab Lecture 3 Chapter 6 LEARNING OBJECTIVES: In this lecture, you will be able to demonstrate the following key massages: Epidemiologists must consider all sources of errors, and strive to reduce them. Epidemiologists devote much attention to minimizing errors and assessing the impact of errors that can not be eliminated Understanding techniques that epidemiologists should use to minimize errors such as; Randomization and comparability, Checking group comparability, Understanding the recruitment and eligibility criteria for participants in the experimental studies Understanding the data analysis for experimental studies such as the relative In a crossover design, the treatment is first randomized. After a period of observation and measurement a ‘‘washout’’ period follows, during which the effects of the treatment subside. This is then followed by a cross-over to the alternative treatment by study subjects: These are called factorial designs. Confounding Confounding can occur when extraneous factors that could otherwise confound the results of the study exists in the study population and is associated both with the treatment and the control group being studied. Confounding occurs when the effects of two groups have not been separated and the analysis concludes that the effect is due to one variable rather than the other. Age and social class are often confounders in epidemiological studies The control of confounding Several methods are available to control confounding, either through study design or during the analysis of results. The methods commonly used to control confounding in the design of an epidemiological study are: Randomization Restriction Matching. At the analysis stage, confounding can be controlled by: Stratification Statistical modeling. Stratified or blocked designs in which the treatment is randomized with in separate strata or ‘‘blocks.’’ Randomization and comparability Randomization is the defining feature of modern trial design. Randomization works by balancing potential confounding factors in the treatment & control group The principle of randomisation remains the same: the luck-of-the-draw determines the treatment. An Example of RCT (polio trial) Polio rates (per 100,000) The vaccinated group had a polio rate of 28. The placebo group had a polio rate of 69. The ‘‘refusers’’ had a polio rate of 46. Had the treatment group been compared with the ‘‘refusers,’’ the efficacy of the vaccine would have been underestimated by about 50%. Checking group comparability While randomization is of great benefit in creating group comparability, group differences can and do arise by chance, especially when the trial is small or when the randomization procedure is flawed. In addition, the study intervention itself may accidentally discover a group difference. Therefore, even with the benefits of randomization, it is still important to compare the groups with respect to relevant cofactors that could affect the results of the experiment. Recruitment and eligibility criteria The source population for an experiment consists of those individuals who may potentially serve as study subjects. Identifying an appropriate source population requires setting up specific criteria for inclusion and exclusion. These criteria are called admissibility (eligibility) criteria. Well-defined admissibility criteria increase the suitability of study subjects while establishing a meaningfully homogenous source population by which to study the effects of the exposure. An Example of Admissibility criteria (WHI trial) Source population: predominantly healthy postmenopausal women with an intact uterus were eligible for participation. Inclusion criteria; potential participants were considered to be postmenopausal if they had experienced no vaginal bleeding for 6–12 months, depending on their age. Exclusion criteria: subjects with a life threatening medical condition, alcoholism, dementia. Follow-up and outcome ascertainment The follow-up period of an experiment may be as brief as a few weeks for acute responses, or as long as years or decades for outcomes with long induction. During the follow-up period, subjects are periodically assessed for the occurrence of relevant outcomes. Outcomes are ascertained during follow-up in a uniform manner using criteria established by case definitions. Blinding (masking) is a study technique by which various parties are kept in the dark about the type of treatment each study subject is receiving. In addition, clinical evaluations were made without knowledge of the type of treatment each subject was receiving. This is a form of double blinding because two parties (study subjects themselves and the clinical evaluators) where masked about exposure status. Triple blinding may be applied by masking treatment assignments from additional parties such as the epidemiologists and statisticians responsible for interpreting the data. Although blinding does not eliminate diagnostic errors, it encourages errors to balance among the study groups. Sample size requirements Studying a sufficient number of subjects is essential to a trial’s success. Therefore, sample size requirements for trials are determined before data are collected. Two approaches are used for calculating a sufficient sample size. When hypothesis tests of statistical significance are of primary importance. When the objective is to estimate an effect size. In both instances, the sample size depends on expected rates of the outcome in the treatment and control groups and the ratio of the sizes of the two groups. Data analysis Data analysis for experimental studies can range from simple analysis (comparisons of incidences) and a complex one. Simple analysis: the relative effect The relative effect is quantified with this rate or risk ratio: R1 0.01928 RR 1.28 R0 0.01506 Where R1 represents the incidence rate or incidence proportion (risk) in the treatment group, and R0 represents the incidence rate or incidence proportion (risk) in the control group. An Example of Measures of effect (WHI trial) Exposure group: women who receive treatment The placebo group No treatment 164 R1 0.01928 8506 How to say it: The exposed group’s risk was 1.28× 122 that of placebo group. R0 0.01506 8102 In relative terms, the risk is 28% R1 0.01928 higher with E+P. RR 1.28 R0 0.01506