Exam Prep Summary MMSR PDF
Document Details
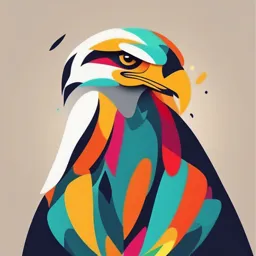
Uploaded by GoodlyBeech2908
Tags
Related
- Minimum Medical Standards Requirements Manual 2021 PDF
- MMSR Manual 2021-Standard 9 Medical Records, Policies, and Procedures PDF
- MMSR Manual 2021-Standard 8 Infection Control & Housekeeping PDF
- MMSR Manual 2021-Standard 5 (Disaster Response Plan) PDF
- MMSR Manual 2021- Standard 4 (Pharmaceutical Services) PDF
- MMSR Exam Summary PDF
Summary
This document is a summary of exam preparation materials for MMSR (likely a course in Multivariate Statistical Methods). It covers various topics, including basic statistical concepts, base knowledge, regression analysis and factor analysis. The document provides definitions, formulas, and information relevant to exam preparation.
Full Transcript
To do ===== What to do (+- 4 days and time to go over again): - Per topic: **1 Make sure all 130 pages of summary have been read** **1.2 make sure all appl lecture extensions have been studied (is first time)** **2 Read HAIR key terms (write down difficult terms) and chapter summary** 3. **...
To do ===== What to do (+- 4 days and time to go over again): - Per topic: **1 Make sure all 130 pages of summary have been read** **1.2 make sure all appl lecture extensions have been studied (is first time)** **2 Read HAIR key terms (write down difficult terms) and chapter summary** 3. **End with key topic overview one pager of every topic** 4. **Make quiz ai** Introduction ============ Interpretation start - Missings - Normality Variance = how much the data spread out or differ from their mean. Interdependence or dependence? Is the variable metric or categorical?  Understand these! In the summary **these will be marked and coloured.** 1)Asses model fit and then 2) assess the individual coefficient Afbeelding met tekst, schermopname, Lettertype, nummer Automatisch gegenereerde beschrijving understand the decision stages of Hair Base knowledge ============== **Type 1 error** = when you reject the null hypothesis when it is true (false-positive) **Type 2 error** = when you accept the null hypothesis when it is false (false-negative) **Theory** = a proposed description, explanation or model of the manner of interaction of a set of phenomena. **Hypothesis** = usually consist of a condition and a consequence. Each part contains a construct of an independent variable and a dependent variable. Non-directional hypothesis = no specific view of how a variable influences the other variable. **Construct** = a conceptual term used to describe a phenomenon of theoretical interest. It is quantifiable and either directly or indirectly observable. Exogenous variables = measured outside the model and imposed on the model Endogenous variables = variables that depends on other variables in a statistical model (not exactly the same as independent and dependent variables) [Overview of possible effects]; **Mediation** effect (Z as a mediator) **Spurious** relationship; a third variable influences the other two variables  **Bidirectional**/**cyclic** causal relationships **Moderation** effect The strength or direction of A on B depends on the level of M  [Conceptual models] Measurement model is the complete model, the structural model is the part we can observe Symbols (Nomenclature) Indicators are normally presented a squares Latent variables are normally drawn as circles or ovals. Latent variables represent phenomena that cannot be measured directly (e.g. motivation, intention) When the circle is left off, structural error is modelled. Few researchers expect their model to represent reality perfectly  Formative/emerging vs reflective/latent models - Formative (''de indicatoren vormen het construct'') From measure (Indicators) to construct. No reason to assume that indicators will be correlated. Alters meaning of construct when you drop an indicator - Reflective (''de indicatoren reflecteren het construct) Direction is from construct to measure, indicators are expected to be correlated. The meaning of the construct does not alter when an indicator is dropped. Correlation = two constructs are linked, this can be coincidental (there is a linkage) Causality = one construct causes a reaction in the other construst (the reaction is because of the other variable) Validity = if a model measures what it is supposed to measure Reliability = consistency and stability of a measure Transforming when no normality: - Log - INV - Square - Square root transformation **Dummy variables** Dummy variables are made when variables that need to be metrically scaled for the model, are categorically scaled. For every category you assign one 1 and give the others a 0. When running the model, one dummy is left out as a reference category. The reference category is chosen with argumentation of previous experience. If none, you choose the dummy with the highest N. Interpretation of dummy's in regression analysis: - Positive coefficient: The dummy has more impact than the reference dummy - Worse coefficient and significant: The dummy has less impact than the reference dummy - Not significant: no effect of dummy with the reference dummy. No difference between the two **Difficult key terms:** Multicollinearity = when two independent variables correlate too much. - Multicollinearity inflates standard errors of coefficients - Larger standard errors = smaller t-values - Formula: t = (coefficient) / (standard error) Calculating a confidence interval Take the mean + and - two times the Standard Deviation **Causality vs correlation: Correlation is simply that there is a relationship between the variables. Causality means that A causes B to react.** Factor analysis ===============  **LO1: Differentiate exploratory factor analysis techniques from other multivariate techniques** EFA is the only data reduction technique that gives a researcher the control over the data reduction process. It can play a big role in the application of other multivariate techniques by providing the tools for the structure of interrelations among a large number of variables (so it reduces data which makes it better applicable for other data sets). Factor analysis can function as a starting point. **LO2: Distinguish between exploratory and confirmatory uses of factor analysis techniques** Exploratory factor analysis do not set a priori constraints on the estimation of components. Confirmatory factor analysis has a restricted amount of factors that are based on a priori hypothesis. **LO4: Distinguish between R and Q factor analysis** R factor analysis is the most common type, it analyses a set of variables to identify the dimensions for the variables that are latent. Q factor analysis is a method that combines large numbers of cases into distinctly different groups within a larger population. It is grouping people with the same patterns. **When to use and purpose** **To reduce the amount of variables, in simple words.** Primary purpose is to define the underlying structure (with interdependencies) among the variables in the analysis **Why -\> It increases reliability and validity** and allows assessment of measurement. **Assumptions** [Measurement properties] need to be ratio or interval level (metric). For non-metric variables we need to make dummy variables. **All variables need to metric!** [Sample size] - should be above 50 but rather above 100 or even 200. - Ratio of minimum of 5 times the amount of observations as variables. - A sample size of 100 is sufficient if all the communalities are above.70. If communalities fall between.40 and.70 the sample size should be at least 200. Communalities below.40 need a sample size of up to 400. [Sufficient correlations among the variables]. Tested with Bartlett's test of sphericity that needs to be significant. [Sampling adequacy.] Tested with MSA (measure of sampling adequacy) that needs to be above.50. But we use the KMO test which is the overall test (MSA is a test within KMO and gives more detail). **Important extra information** - Two types of factor analysis: Exploratory factor analysis = generation of hypothesis, assumptions of an underlying structure. It reveals interrelationships Confirmatory factor analysis = tests hypothesis, a prior (you have them already) of underlying hypothesis derived from theory. - Two forms of measurement models: [Reflective/latent] (mostly seen): From construct to measure, construct make the indicators (Satisfaction influences how someone answers on a question). Indicators correlate [Formative/emergent]: Direction is from indicators to construct, indicators make the construct. (e.g. work experience and degree form socio-economic status) Factor loading = correlation between item and factor Communalities = squared loadings for each item Eigenvalue = squared loading of all items loading on that factor Measurement error = the variance of each variable that cannot be explained by other variables. Even a random data set will give factors. This means that theoretical considerations always should be prioritized. **LO3: Understand the Process** 1. [Problem formulation ] Is it data summarization or data reduction? Which variables are we going to measure? Check - If all variables are metrically scaled - If normally distributed - Not more than 10% missing values 2. [Constructing correlation matrix ] **KMO measure** to determine if the sample is adequate. If variables correlate with each other - Should be 0.5 or above (closer to 1, the better). **Bartlett's test of sphericity.** To determine if variables in the population are sig. uncorrelated. - Should be significant (\ check if you have a reversed coding* - Orthogonal *unrelated* rotation (varimax), check rotated factor matrix - Oblique *related* rotation (oblimin), check pattern matrix **LO6: How to determine the number of factors to extract** The goal is achieving simple structure. Variables load high on only one factor. The rules we assume in this course are as follows - If the **communality** of the item is below.20 it usually means that the item does not share much with the other variables. - **The factor loadings** often correspond with this. In exploratory use, with large samples, we accept a factor loading of.30 or higher. With more confirmatory and smaller samples we accept a factor loading of.50 or higher. - A cross-loading harms discriminant validity and therefore you don't want that in your model. The difference between two loadings should be a least.20. (otherwise it does not discriminate enough between the two items). **Model fit:** Via residuals, difference between observed correlations and reproduced correlations should be lower than 0.2 (not a hard criteria). A model can be: - Under identified factors = more parameters to estimate than unique terms - Just identified = the same amount of parameters to estimate as unique terms - Overidentified -- less parameters to estimate than unique terms 7. [Using factors in other analysis ] We learn three options: - **Surrogate variable** is a simple method in which you use one variable with the highest loading for the whole factor. (if the loadings are similar, use theoretical considerations) - Advantage is that it is very simple, disadvantage is that it does not represent all facets of a factor - **Factor scores** are scores based on how strongly the item aligns with the factor. You get a precise numerical summary for each variable and observation. - Most complete option, interpretation is more difficult - **Summated scores** you add or average all variables that load high on the factor. This sum score is used instead of the factor score. - A compromise between the other two options. It excludes variables that have marginal impact (low loading) which is a disadvantage. The biggest difference between summated scores and factor scores is that factor scores is computed based on all variables factor loading and summated scores is computed based on selected variables. These options will provide a new variable for use, for example, as the independent variable in a regression analysis. **Assumptions of summated scores**: Measurement error = the degree to which the observed values are not representative of the actual values. Summated scores reduce this by using varies indicators. **Content validy** (face validity) = correspondence of the variables to be included in a scale and its conceptual definition. **Unidimensionality** = strongly associated with each other and represent one single concept. (almost same meaning as the simple structure) Reliability = degree of consistency between multiple measurements of a variable - Cronbach's alpha above.70 or sometimes in exploratory research above.60. Construct validity = content validity is a form of construct validity. Construct validity is the degree to which a construct represents what is intended with the concept. Other forms: - **Convergent validity** = degree to which two measures of the same concept are correlated - **Discriminant validity** = degree to which two measures of the same concepts are distinct - **Nomological validity** = degree that the summated scale makes accurate predictions of other predictions of other concepts in a theoretically-based model (scale is able to predict other concepts in a theoretical model) *In summary, convergent validity confirms that the scale is correlated with other known measures of the concept; discriminant validity ensures that the scale is sufficiently different from other similar concepts to be distinct; and nomological validity determines whether the scale demonstrates the relationships shown to exist based on theory or prior research.* 8. [Determining model fit ] Assessment of reliability and validity is a iterative process, so do not forget to check this (KMO and Bartlett) after every iteration. A criteria for the model fit is that it is generalizable. In the book split sampling is explained in which you split the set in two equal samples and you run these both and compare if they are similar (indicating robustness). Cumulative extraction of sums give you the percentage that is explained by the factor Add on: Confirmatory Factor Analysis ------------------------------------ A CFA is theory-driven and has the number of factors and pattern of indicator loadings specified in advance. The variance-covariance matrix of unstandardized observed variables is analysed (instead of correlation matrix). Step 1: model specification The number of factors and observed variables that load on each construct are specified in advance. Each parameter (e.g. variance, loading = things you need to measure) can either be - Fixed (by the researcher) - Free (estimated) - Constrained (estimated while acknowledging the constraints) Step 2: identification Deductive: have the a priori assumption (test theory) -\> the structure of a model and its parameter values determine the variances and covariances of the observed variables Inductive: data and the model run together (make new theory) -\> the empirical variances and covariances yield estimates of unknown parameter given the structure of the model Variance = how variables differ Covariance = how variables cohere Step 3 Estimation Estimate parameters (a number that describes a whole population, e.g. population mean) so that the discrepancy between the sample covariance matrix and the implied covariance matrix is minimal: - Maximum Likelihood (ML) -- most used (standard option) - Unweighted Least Squares (ULS) - Generalized Least Squares (GLS) - Distribution-free (ADF) Step 4 Testing model fit Types of fit measures: - Descriptive measures - Statistical inference - Measurement of approximate fit - Comparative fit measures - Information theoretical measure **The goodness of fit (GFI): \>.90 is good** measures the relative amount of the variances and covariances in S that of a model relative to the number of variables Degrees of freedom (amount of independent observations of a sample) indicate how complex your model is; AGFI adjusted goodness of fit, adjust for the degrees of freedom of a model relative to the number of values Statistical inference: **You want this to be insignificant** Significant result means that the implied covariance matrix differs significantly from the empirical covariance matrix. This means that the model is wrong (happens a lot in marketing and management because we want to simplify reality which is not possible). Measure of approximate fit in CFA: **RMSEA \_\<.08 is good enough** 5\. Model respecification If model is overfitted. Relax some constraints and/or add parameters. **What is the key difference between EFA and CFA?** EFA is a data-driven, exploratory technique used when researchers don\'t have strong preconceptions about the underlying factor structure. It lets the data suggest how many factors exist and how variables might group together. CFA, on the other hand, is theory-driven and confirmatory in nature. Researchers start with a specific hypothesis about how variables relate to factors, based on prior research or theory, and then test whether the data supports this predetermined structure. **Difficult key terms:** ------------------------ Cluster analysis -- similar to Q factor analysis = multivariate technique with the objective of grouping respondents or cases with similar profiles on a defined set of characteristics. Communality = total amount of variance an original variable shares with all other variables included in the factor analysis. Calculated as the sum of the squared loadings for a variable across the factors. Convergent validity = the degree to which two measures of the same concept are correlated. It is an aspect of construct validity. Construct validity = broad approach to ensure the validity of a set of items a representative of a conceptual definition. Includes specific sub-elements of convergent validity , discriminant validity and nomological validity. Content validity = assessment of the degree of correspondence between the items selected to constitute a summated scale. Cross-loading = a variable has two or more factor loading exceeding the threshold value deemed necessary for significance in the factor interpretation process. Discriminant validity = one element of construct validity focusing on the degree to which two concepts are distinct. Eigenvalue = represent the amount of variance accounted for by a factor. (also referred to as latent root) Latent root criterion = criterion of Eigenvalue threshold of 1 Measure of sampling adequacy (MSA) = measure calculated for the entire correlation matrix and each individual variable. Value above.50 is appropriate. Multicollinearity = extent to which variables can be explained by other variables in the analysis Nomological validity = an element of construct validity focusing on the extent to which the scale makes accurate predictions of other concepts in a theoretically based model. Q factor analysis = forms groups of respondents or cases based on their similarity on a set of characteristics R factor analysis = analyses relationships among variables to identify groups of variables forming latent dimensions Reliability = extent to which a variable or set of variables is consistent in what is being measured Unidimensional = variables are only correlated with the hypothesized factor Unique variance = portion of a variable's total variance that is not shared variance. Has two portions; specific variance relating to the variance of the variable not related to any other variables and error variance attributable to the measurement errors in the variable. Validity = extent to which a single variable or a set of variables (construct validity) correctly represents the concept of the study. Variate = Linear combination of variables formed by deriving empirical weights applied to a set of variables specified by the researcher. (M)AN(C)OVA =========== **Read summary** **When to use and purpose** To test if there are significant differences between two or more group means. H0: all population means are equal Ha: population means are significantly different When there is more than one DV: The reason you use manova instead of testing every relationship with a separate anova is that it would raise the chance of type 1 error a lot. A type 1 error is when you reject the null hypothesis when it is actually true in the population. Doing separate anova tests will also ignore the possibility of lineair combinations between the dependent variables. **Assumptions (LO3)** Sample size At least one of the IV's is categorical, there is one DV that must be metrically scaled (except from MANOVA) Assumptions: 1. **[Normality] of sampling distribution** - Skewness and Kurtosis in range of -3 and 3. No problem if there are at least 30 observations per group (not normal? Transform) 2. **[Independence]** of observations - Cannot calculate this, this lies in the design of the experiment (for example, students in the same group can influence each other which leads to biases) 3. **[Linearity ]** - Test with plots 4. **[Sample sizes]** - Cells/groups of combining IV's times each other. - Recommended is 20 observations per cell (group) - Min size per group must be greater than the number of DV's 5. **[Homogeneity of variance]**. Very important because it affect the F test. - Levene's Test not significant is the goal (=the variances of the DV is the same for each group) - If Levene's test is significant [and group sizes differ], we use the **Welch Statistic** instead of the F test. - For covariance matrix (MANOVA) we use Box M test (also not significant is what we want) 6. **Multicollinearity** (two IV's are highly correlated) - VIF above 10 indicates this - Or Bartlett's test of sphericity Off course for there to be an effect, F-statistic needs to be significant at.05 level. **Interaction effect** If an interaction effect is non-significant, use the main effects. If an interaction effect is significant, decide (based on a plot) whether it is an **ordinal or disordinal interaction**. In case it is an ordinal interaction, you still need to describe the main effect for each level of the treatment (by means of a post-hoc analysis). In case it is a disordinal interaction, this will interfere with the interpretation of main effects (thus, do no interpret them). Within disordinal interactions, we divide between non crossover and crossover**. So, always plot with interaction effect** - Ordinal interaction is when the lines in the graph do not cross (study method A and B, study method A always performance better but the gap is larger when combined with prior knowledge. Making it an interaction effect) - Disordinal interaction is when the lines differ in steepness or direction, this could be a crossover or non-crossover interaction. **Important information** Types of ANOVA: - One-way ANOVA: One factor (DV) with at least two levels (groups) - N-way ANOVA: Two or more independent variables, which are independent - ANCOVA: if independent variables contain both categorical and metric variables. Normal ANOVA only has categorical IV's ( so a metric IV is added compared to one way anova). The difference between a covariate and a control variable is that a covariate always metrically scaled is. Two purposes of ANCOVA: - Removing effects of variables which modify the relationship of categorical and DV's (Reduce error) - Control for factors which cannot be randomized but which can be measures on an interval scale. - Repeated measures ANOVA (more than one moment of measurement): One factor with at least two levels; levels are dependent this time. - You need at least a before and after measurement in which you do an ANOVA. - MANOVA: more than one DV at the same time. This takes correlation between dependent variables into account. Statistics: F = SSx (between group variance) / SSerror (within group variance) **In SPSS: Mean square corrected model / mean square error** - SSx; Sum of squares: the variation in Y related to the variation in the means of categories X. Represents variation between the categories of X. - SSerror: Variation in Y due to the variation within each of the categories of X. This is the variation not accounted for by X - SSy: total variation in Y **Model statistics (Goodness of fit)** **Eta2**: Effect size: Measures the strength of X (IV) on Y (DV). From 0 to 1, closer to 1 is stronger (0.01 -- 0.06 -- 0.14). (SSx / SSy) **F**: Group means are different or not: Test the null hypothesis that the means are equal in this population. SSx / (c-1)) / (SSy / (N -- c)) -\> c is the numerator and N is the denominator which you find in the table. F test is the overall effect and must be significant for an effect. - Table test of Between-Subjects Effects: the mean square of a variable / mean square error = F-value of that variable (easy to find in test of between subjects table) R squared (adjusted) is the total variance explained by the model (.10 weak,.30 moderate,.50 strong) **Parameter statistics** **Coefficients**: Represent the differences between groups **T-test** (df2): Tests the significance of group differences or covariates [A priori]: To examine differences among means, we use **contrasts (K-matrix)**. Which are a priori hypothesis from the researcher. Use when you have a clear hypothesis - **Deviation** ([default] of anova)= group mean (A) vs grand mean (A,B,C) - **Simple** = group mean 1 (A) vs group mean 2 (B) -\> here you can interpret the significance one sided! In SPSS they gave a two sided test. [So with a **directional** hypothesis you divide the sig. level by 2.] Which could make it significant. [A posteriori]: Choosing a Post-Hoc by looking at equal group size and heterogeneity of variance (Levene). No hypothesis specified yet - **Tukey** = homogeneity and equal group size - **Hochberg** = homogeneity and inequal group size - **Games-Howell** = heterogeneity The difference between contrasts and post-hoc is that contrasts use a priori hypothesis and directly test these given it more statistical power. Post-hoc test all possible combinations. For contrasts you check the K-matrix, here you can see in there is a significant differences between categories (simple contrast) or between category and variable mean (deviation contrast). Post-hoc cannot include covariates in SPSS so that made the assignment difficult Unbalanced design = different N per category of a variable (Leads with post-hoc to Games-Howell) To check a variable with multiple categories it is advisable to - look at the estimated mean table with the two variables. In this table you look for non overlapping confidence intervals of categories because that means that there is a significant difference. - Or look at error bars for the plots with a **group mean plot (always check mean plot in interaction effects)**, here you can see if intervals overlap ass well. So scan for non-overlapping intervals. **Confounders:** Impact both the treatment and the control variables while not being included in the model. **Blocking variable** = a control variable that is not of particular interest to the researcher Check residuals for model fit. Look at residual graphs. In the exam also write down the F test in your answer. Example: F(1,1764), 28,468, p \