AI, Robotics, and Automation in Diagnostic Labs PDF
Document Details
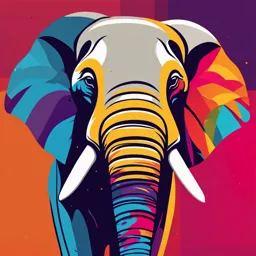
Uploaded by KnowledgeablePegasus
Misr University for Science and Technology
Tags
Summary
This document discusses how artificial intelligence, robotics, and automation are transforming diagnostic laboratories. It explores the challenges these labs face, including staff shortages, human error, and uneven access to care. The document also examines the potential improvements that AI and automation can bring, such as enhanced diagnostic accuracy, faster turnaround times, and personalized medicine.
Full Transcript
How AI, Robotics and Automation Will Reshape The Diagnostic Lab Of The Future The healthcare and diagnostics fields are facing significant challenges in terms of staff shortages, burnout, human errors and inequitable distribution of prices and resources. Art...
How AI, Robotics and Automation Will Reshape The Diagnostic Lab Of The Future The healthcare and diagnostics fields are facing significant challenges in terms of staff shortages, burnout, human errors and inequitable distribution of prices and resources. Artificial intelligence and data analytics will improve diagnostic accuracy and early disease detection, reduce treatment time, personalize medicine and increase access in underserved areas. “Smart laboratories” will emerge that will use robots for repetitive tasks and intelligent decision-making, require minimal human supervision, use data to predict disease outbreaks and inform public health decisions. Laboratory test results supply the foundation for most medical decisions. However, they are likely to feature more prominently in healthcare, given persistent trends in human health. These include ageing populations, higher rates of non-communicable diseases, and upticks in early-onset cancers, obesity, type 2 diabetes and other cardiometabolic and neurodegenerative diseases. Indeed, tomorrow’s global health hinges largely on the future of diagnostic testing. But what will clinical laboratories look like in the years ahead? Critical challenges in healthcare and diagnostics Accuracy, fast turnaround times and access will always be the pillars of quality diagnostic testing. 1 Yet, certain conditions within the healthcare landscape threaten that vital triad. Devising solutions to ensure these pillars remain intact will be essential to advancing human health worldwide. Over the past decade, the healthcare industry has faced a reverberating shortage of qualified professionals from lab technologists to nurses and doctors. Burnout fatigue among overworked staff is prevalent, and its ramifications are significant. In traditional diagnostic laboratories, for instance, mislabeled samples, transcription and other human errors compromise patient safety while driving up healthcare costs. Factor in rising prices and the deficiency of medical resources. In combination, these systemic stressors limit access to care, with the heaviest hit on remote and already-underserved parts of the world. Such ongoing challenges set off cascading consequences for patient care: diagnostic errors, delayed diagnosis and treatment or missed diagnoses completely, adding cost and resulting in poorer outcomes. So, in today’s challenging environment, how do diagnostic labs better ensure accurate and early detection so clinicians can deliver the most appropriate treatment for the best possible patient outcomes? The smart and strategic use of new and emerging technologies is critical. 2 The power of AI and data analytics Into the mix enters artificial intelligence- (AI)-powered diagnostics (Figure 1): AI algorithms can analyse vast datasets of diagnostic data, medical images, and patient history to improve diagnostic accuracy and identify diseases earlier. These algorithms can enhance analytic capabilities and address the medical data overload that doctors face. Notably, AI-assisted analysis can help reduce turnaround times. The sooner clinicians receive diagnostic test results, the sooner patients can begin treatment. This is pivotal, as timing is often a differentiator in how an illness responds to therapy. Moreover, AI can be a boon for personalised medicine, enabling realistic, time-efficient analysis of a patient’s unique medical history and genetic data to predict disease risk and tailor treatment plans. For remote and underserved areas, AI-powered lab systems, with telemedicine, could expand access to care by equipping advanced diagnostics where none were available. Figure 1 - Innovations in AI will continue to transform and digitalize healthcare and laboratory diagnostics. 3 The promise of ‘smart laboratories’ Lab automation is not new. Robots can already handle repetitive tasks, such as sample preparation and analysis, to improve turnaround times and reduce the risk of human error (Figure 2). But AI-powered robots of the future will handle more than the mechanical part of lab automation. They will make intelligent decisions based on the information they see. Such fully automated “smart labs” may be able to operate 24 hours a day, seven days a week and 365 days a year with minimal human supervision, thereby increasing efficiency and providing real-time results. The advancement of humanoid robot technologies capable of performing intelligent operations without human intervention or a pre-programmed set of rules will enable scenarios wherein such robots are indeed future lab technologists – working round the clock and throughout the year. Staff shortages, a slowdown in the number of professionals entering the field and the need for existing professionals to remain focused on higher-level tasks will make a monumental difference in the ability of labs to keep pace with demand. Figure 2 - Mobile robots that can perform specific tasks in a diagnostic laboratory, such as navigate the corridors and elevators alongside humans to replenish reagents, in a test conducted by Siemens Healthineers, United Robotics Group and HUS Diagnostic Centre, Helsinki, Finland. 4 Using digitally connected diagnostics and drawing from vast amounts of data generated during the diagnostic process, smart labs running on AI and robotics will also be able to identify trends, patterns and correlations that might otherwise be missed. That translates into the prediction of disease outbreaks, the identification of high-risk populations and contributions to epidemiological and pandemic research. AI-based analytical tools that are scalable, easy to use and transparently deployed can help interpret data to improve decision-making, especially during rapidly evolving public health crises. We learned from the COVID-19 pandemic that actionable clinical data can inform prompt evidence-based policymaking and adjustments to healthcare systems. 5 Uses of AI/ML in the lab There are numerous ways that both simple and complex AI tools can aid a clinical laboratory. These include: Automated spectroscopic data analysis and disease detection; multivariate analysis of disease conditions; test interpretation; Digital image analysis for microbiology, haematopathology, immunology, and forensics; Data entry automation for specific tasks and processes; Creating standardised reports for lab test results and automated entry into Laboratory Information System (LIS); Minimising laboratory testing for inappropriate test orders, predicting test results from other available data on patient chart, and reducing redundancy and duplication of lab tests, based on prior type and date of tests already performed; Data analytics for laboratory operations planning, such as predicting volume workflow, employee staffing requirements, etc.; Identifying and alerting for abnormal test results; Auto-verification of test results for quality control. 6 Automating spectral analysis for kidney stones and fecal analysis Mayo Clinic spent six years developing an AI model to automate the spectral analysis of stones passed by patients. It is based on the classification of 708 unique kidney stone types. In the first 90 days of implementation, commencing April 2023, 20,000 stones have been reviewed, 40% of which were newly able to be bulk auto-released to patients’ medical records. Before the model was implemented, the conventional workflow began with cleaning and drying the stone, after which it was ground into a fine powder and manually analyzed with FTIR spectroscopy. A technician manually entered the results into a LIS, followed by a second technician reviewing the interpretation. Only then are results uploaded to a patient’s electronic medical record (EHR). 7