Emerging Technologies Lecture Notes PDF
Document Details
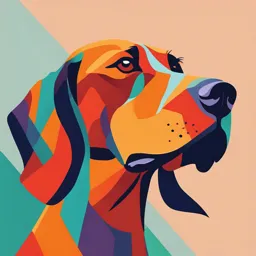
Uploaded by SmootherChrysoprase3281
Tags
Summary
This document is lecture notes on emerging technologies and design thinking, featuring a discussion on the human-centered, non-linear, and iterative aspects of design thinking. The document also covers web design, including visual and functional elements, content strategy, SEO, and user psychology.
Full Transcript
Emerging technologies Lecture 1 – 11/09/24 Design Thinking - Design of digital tools to solve real-life problems Design thinking is an approach, a methodology we can use in order to build our project from the beginning to the end. A pioneer of design thinking, Tim Brown, defines design thinking a...
Emerging technologies Lecture 1 – 11/09/24 Design Thinking - Design of digital tools to solve real-life problems Design thinking is an approach, a methodology we can use in order to build our project from the beginning to the end. A pioneer of design thinking, Tim Brown, defines design thinking as a human- centered, non-linear, iterative process that teams use to understand users, challenge assumptions, redefine problems and create innovative solutions to prototype and test. Human centered – Because nowadays, designers and all of us, all the specialists, have understood that human is the center, is the core of our business, whatever the business is. So human is at the centre of the whole the project, the whole design and all the processes to make a product or a service. Non-linear – Because there are different steps, but these steps are not following a single path. So we can go back and forth in order to redefine the process or change something that is not working. Iterative – Because there are multiple ways in which the process can change and reiterate itself. Design got small, it became a tool of consumerism. We should focus less on the object and more on the design thinking to have a bigger impact with the result. Design thinking begins with integrated thinking which is exploiting several constraints to create something desirability and viability. Perhaps design is getting big again which arises with the application of design thinking to large problems. Tim Brown Ted Talk The speech addresses the transition from traditional design to design thinking, emphasizing how design thinking can create broader, more impactful innovations by focusing on human- centered approaches and systemic problem-solving rather than merely improving aesthetics. The speaker reflects on their early career projects, which involved superficial product improvements, contrasting them with the larger, more meaningful outcomes achieved through design thinking. Key concepts discussed include: 1. Design vs. Design Thinking: Traditional design often focuses on improving the look or usability of individual products. In contrast, design thinking is an integrative, problem-solving approach that balances human needs, technical feasibility, and economic viability to address broader challenges. 2. Historical Context of Design: The speaker highlights the work of 19th-century engineer Isambard Kingdom Brunel, who tackled complex, system-level challenges, such as designing the Great Western Railway and envisioning an integrated transportation system. Brunel exemplifies the broader view of design that design thinking aims to revive. 3. Human-Centered Design: Design thinking starts by focusing on human needs, understanding culture and context before building solutions. This approach has been applied to global challenges, such as vision screening programs in India and ultra-low-cost hearing aids. 4. Prototyping for Innovation: Rapid prototyping helps refine ideas quickly by making them tangible. An example is the Aravind Eye Institute, which reduced the cost of intraocular lenses through early prototyping, resulting in a significant impact on healthcare for low-income patients. 5. Participation Over Consumption: Design thinking encourages participatory systems, moving beyond passive consumption. The shift involves engaging people in meaningful ways, creating value that transcends traditional consumer relationships. The Southwark Circle project is an example, where elderly residents were actively involved in designing a service to help with household tasks. 6. Design Thinking as a Tool for Change: In times of societal change, design thinking helps explore new alternatives by fostering divergent thinking. The speaker references ongoing projects with the Acumen Fund and Bill and Melinda Gates Foundation, where local water providers in India were involved in creating innovative solutions for clean water access. In conclusion, the speaker advocates for a return to a more expansive view of design, similar to the approach of historical figures like Brunel, where the right questions are asked, and design thinking is applied to address complex global challenges. Design Thinking in a nutshell The feasibility is the technical feasibility. So with the technological innovation, is my idea feasible? And then the viability is from an economic point of view, so is it economically viable? So the design thinking methodology is a really simple methodology, but it is really complete. It allows people who are not designers to use creative tools in order to solve some challenges and to discover and find solutions that are really effective. So we will see design thinking from three points of view: Design Thinking in context As we said, design thinking offers an approach for addressing the big problems and the big questions. As we saw during the video, the important aspects are: - learning by doing: we go deep in the problem in order to solve it. - think out of the box. The first stage of design thinking is divergent, where creativity flows freely. Brainstorming leads to unconventional, even seemingly impossible ideas. While some ideas may appear unrealistic at first, they serve as catalysts for innovation and can lead to out-of-the-box solutions. These early, imaginative concepts are key to finding viable, practical outcomes that may not seem obvious initially but offer great potential for solving complex problems. We live and work in a world of interlocking systems, where many of the problems we face are dynamic, multifaceted, and inherently human. Think of some of the big questions being asked by businesses, government, educational and social organizations: How will we navigate the disruptive forces of the day, including technology and globalism? How will we grow and improve in response to rapid change? How can we effectively support individuals while simultaneously changing big systems? Design thinking offers an approach for addressing these and other big questions. There’s no single definition for design thinking. It is a way to solve problems through creativity. There are 2 main phases: 1. We have to diverge – create choices through brainstorming or processes of inspiration. 2. We have to converge – once we have created all the choices and we put it in a whiteboard we have to think logically about the problem and try to select the choices we created in order to get the best solution for the specific problem. Designt thinking is a methodology used by the majority of the brands in order to create new products, new services, or think about experiences. Design Thinking in numbers 3 Main Stages of Design Thinking If we think about design thinking in numbers, we have 3 main stages: 1. Inspiration: during this phase we have to create choices, to write down all the ideas and try to give creativity all the space needed. 2. Ideation: we need to select some of these solutions as the most feasible ones. 3. Implementation: it is not the end of a process, bc design thinking process is iterative. So after the implementation we have the test phase through which we can understand what works and what not and eventually modify things. 4 Pillars of Design Thinking EMPATHY – Empathize with people’s needs COLLABORATION – Collaborate with others, across disciplines, skill sets, and perspectives INCLUSION – Include every idea in visible form for evaluation ITERATION – Repeat, iterating and testing solutions to perfect them, always with human needs at the center 5 Action phases of Design Thinking PROCESS Then there are five action phases in design thinking: empathize, define, ideate, prototype, and test. The first, the divergent phase, is empathizing. After that, we narrow down to define, selecting some of our ideas. We diverge again during the ideation phase, and finally, we build a prototype and test it, focusing on one final solution. As you can see, the process is non-linear. We may go from empathizing to testing and back again, constantly refining our solution. There are three key stages: inspiration, where we empathize and define; ideation, where we further refine, prototype, and test; and implementation, which brings the final product or service to life. Design thinking is all about alternating between diverging to brainstorm solutions and converging to focus on the most viable idea. We can think of the design challenge as a messy situation, solved through creativity to arrive at the best solution. Web Design What is web design? Web design is the process of conceptualizing, planning, and creating the visual layout and functionality of websites. It involves various disciplines such as graphic design, user interface (Ul) design, user experience (UX) design, and (in some cases) coding to produce websites that are aesthetically pleasing, user-friendly, and effective in conveying information or facilitating interactions. There are different disciplines of web design: o Graphic Design: Focuses on the visual aesthetics (layout, colors, images). o User Experience (UX) Design: Centers on creating an intuitive and positive user experience. o User Interface (UI) Design: Focuses on how users interact with the website's interface. o Search Engine Optimization (SEO): Enhances the site's visibility on search engines. o Content Strategy: Organizes and plans content creation. How to present our information within the website. o Development: Converts designs into functional code (HTML, CSS, Java Script) The key elements of web design: o Visual elements: layouts, colors, fonts, logos, images, videos, icons, shapes – we can select colors, fonts, layouts in order to create a website that is the most possible effective for the end user. We will see that there is another discipline called user psychology that is pretty important in the building of a website, because in our psychology we have a pattern, for example, in how we scan the website or the colours that we see and that we use in a website express different feelings and so influence in some way users to do or not do something. o Functional elements: navigation, information architecture (where the different contents are put), user interaction, speed, responsiveness, usability, accessibility – this is all about the user experience o Content: data and how it's communicated Steps in web design: o Research – understand the users, competitors, and goals. o Wireframing – create a simple blueprint of the website structure. o Design – develop the look and feel of the site. o Development – build the actual website. o Testing – Ensure everything works correctly on all devices. o Launch and Maintenance - Make the site live and keep it updated. The process of web design includes research, wireframing, design, development, testing, launch, and maintenance. Like design thinking, it’s an iterative process: we constantly prototype, test, and refine based on user feedback. Web Design evolution: This is important to understand the actual trends of design. Nowadays, human centered and responsive design is essential because most websites are accessed via mobile devices. Responsive web design means creating a single flexible layout that adapts to different screens, ensuring a seamless experience across devices. Then there is the advent of AI, chatbot etc. and we have a trend in minimalism, so in the previous year the websites were more detailed, colored, while nowadays we have eliminated all this stuff and we create functional and simple web sites with info architecture and navigation as the most important things. The website must be useful and simple to navigate for users. The benefits of responsive design include improved user experience, better rankings on search engines (as Google prioritizes mobile-friendly sites), and consistency across devices. User Centered Design The main 2 approaches of web design: User-centered design and system-centered design are not mutually exclusive. A balance between the two ensures a website is both functional and user-friendly, catering to technical needs while also prioritizing user satisfaction. User-centered design is vital today because users demand more intuitive, accessible, and responsive web experiences. User Centered Design Definition «User-centered design (UCD) is an iterative design process in which designers and other stakeholders focus on the users and their needs in each phase of the design process. UCD calls for involving users throughout the design process via a variety of research and design techniques so as to create highly usable and accessible products for them.» — Definition of user-centered design (UCD) by the Interaction Design Foundation Characteristics of User-Centered Design (UCD) Empathy: Designing with the user's experience in mind. Usability Testing: Continuously improving based on user feedback. Iterative Process: Repeated cycles of testing and refining the design. Accessibility: Ensuring inclusivity for users of all abilities. Personalization: Tailoring content and functionality to user needs. UCD steps Understand Users’ Needs: Analyze the problem users are facing. Specify the Problem: Define the exact issue to solve. Design a Solution: Develop potential solutions based on research. Evaluate the Solution: Test and refine the design through usability feedback. Þ System Centered Design and User Centered Design should not be considered two alternative approaches, from which to choose depending on the situation. Þ User Centered Design can be considered a more mature approach, which includes within it the technical problems of the system centralized design, but puts them in a wider context, which allows us to understand in a much deeper way the purpose of the system. So they can work together in order to solve user problems and to build a website that is in one way functional but also usable and accessible for all users. Why is User-Centered Design Critical Today? § User Expectations: Modern users expect websites to be easy to use and responsive to their needs. § Competitive Advantage: A user-centered approach leads to higher satisfaction and retention. § Increased Conversions: Better usability directly correlates with higher conversion rates. § Mobile-First: The shift towards mobile devices demands a user-friendly and accessible experience across platforms. Here we have an overview about design thinking, user centered design and human centered design: Here we can see the intersection between the three approaches: The main idea is that in each of these approaches, the user is always at the centre. Problem-solving and "learning by doing" are key principles, meaning that you continuously iterate and repeat the design steps. This repetitive process helps refine the solution until you find the most effective one. Responsive design There are two main approaches: responsive web design and adaptive web design. In adaptive web design, we create fixed layouts for specific screen sizes—essentially, different websites for different devices. In contrast, responsive web design uses one flexible layout that automatically adjusts to various screen sizes, offering a seamless experience across devices. With the increasing use of mobile devices, responsive websites have become essential, as they provide a consistent user experience, improve rankings on Google (which prioritizes mobile-friendly sites), and eliminate the need for separate mobile versions. Think about how frustrating it is when a website doesn’t work well on your phone—chances are, you’d leave that site. So, by ensuring your site is responsive, you reduce frustration and increase user engagement. Responsive Design benefits: - Enhances user experience across all devices. - Improves SEO rankings (Google prioritizes mobile friendly sites). - Ensures consistent experience without the need for separate mobile sites. Lecture 2 – 17/09/24 Landing pages A landing page is When you have a specific message to convey it is essential to be guided by a real intention and a well structured objective. For we have to deliver to our audience a targeted info. There 3 possible web solutions that can be effective: - Landing page 3 - Minisite/Microsite These tools allow you to create customized content and guide users toward a desired action, whether it's generating leads, driving conversions, or promoting products or events. A landing page is only one page, a single web page that is design to achieve a specific goal. Generally the goal is a sort of conversion for our audience. A minisite is a small standalone website with its own domain or subdomain and it is used for particular brand, campaign or initiative. The main difference is that the minisite is a small site with more pages, while the landing page is one single page. Example of microsite This has a specific goal so it is created by Adobe, but it allows us to do a specific action, it allows users to start a test to discover his creative personality. This type of website is a small one with only 2 pages: the page with the test and the one with. Landing Page o A landing page is a web page specifically designed to direct visitors to a specific action. Unlike a generic web page, which may contain multiple pieces of information and links, a landing page has only one main objective: to convert visitors into leads or customers. o The term ‘landing’ is derived from the fact that it is the page on which a user ‘lands’ after clicking on a link, which might come from an email, an advertisement, a social media post, or other traffic sources. How does it work? Landing pages are basically used in marketing campaigns to convert visitors into leads or customers. When a user clicks on a link from an ad, email, or search engine result, they "land" on this page, designed to focus their attention on a single call-to-action (CTA). Common goals Lead Generation: collecting user information through forms (e.g., email, name) to nurture potential customers. Product or Service Promotion: driving awareness and encouraging users to purchase or sign up for a specific product or service. Event Registration: promoting events like webinars, conferences, or workshops and encouraging users to register. Free Trials or Demos: encouraging users to sign up for a free trial or demo to experience the product. Downloads: offering content like eBooks, guides, or whitepapers in exchange for user information There are different types of objects but also different types of landing pages: (4) Squeeze Page Also known as lead generation page, the squeeze page focus on collecting users’ information via forms. The attention is on the form, bc the main Click-through Page This type of landing page is primary used in ecommerce and it acts as a middle step between an ad and the purchase page, giving users additional info before driving them to buy. Sales Pages They are designed to drive conversion, and the main conversion is the slae of a product or service. They contain detailed info to persuade users to make a purchase. Secondary Landing pages: Splash page: middle page used to introduce a site or direct users to different areas of a website based on preferences. Coming Soon Page: tease an upcoming product or service and encourage users to sign up for updates. Landing Page vs. Website Þ Landing Page: A targeted page designed for a single goal, like lead generation or sales conversion. Þ Website: A multi-purpose platform that provides comprehensive information about a business, offering multiple pages and sections. Key differences FOCUS Landing Page: Single, specific objective (e.g., sign-up, purchase). Website: Multiple objectives and content areas (e.g., product info, blog, contact). NAVIGATION Landing Page: Minimal or no navigation, focused on a single CTA. Website: Complex navigation, allowing users to explore various sections. USE CASE Landing Page: Campaign-specific, conversion-focused. Website: Long-term brand building, broad information. Characteristics recap Single Objective: Clear call-to-action (CTA) like "Sign Up" or "BuyNow". Simplified Design: No navigation menus to avoid distractions. Targeted Messaging: Customized content based on user intent from the ad or campaign source. Optimized for Conversions: Focused on achieving a specific action from visitors. Structure o Headline: A clear, concise statement that grabs attention. o Subheadline: Provides additional information supporting the headline. o Visuals: Relevant images or videos that support the message. o Key Benefits: List of benefits or features of the product/service. o Call to Action (CTA): A button or form that prompts the desired action. o Social Proof: Testimonials, reviews, or client logos that build trust. What is a Call to Action? A CTA is a brief phrase designed to prompt visitors to take an action on your website, landing page, email, or advertisement. Often it is presented as text, images, or clickable buttons, CAs guide users to the next step, whether it's making a purchase, signing up for a newsletter, or booking a service. An effective CTA is crucial for 3 reasons: Boost Conversions: A well-crafted CTA can significantly increase the number of conversions on your site. Drive Sales: Encourages users to complete a purchase or sign up for a service. Generate Leads: Captures potential customer information for future marketing efforts. Key Elements of an Effective CTA Use Action-Oriented Words (e.g. Subscribe, Download, Learn More, Buy, Add to Cart) Keep It Short and Simple: limit the number of words and CTAs on a page to avoid overwhelming users. Understand Your Audience's Needs: tailor CTAs based on user readiness, e.g., "Buy Now" vs. "Download First." Make CTAs Easy to Find: use contrasting colours, strategic placement, and appealing design. Write in the First Person: personalizes the action for the user, e.g., "Start My Free Trial." How to Create a Landing Page Use a pre-designed template or build from scratch. Ensure the page reflects your brand colours and messaging. Test different versions (A/B testing) to see which design and content perform best. Make sure to communicate the value of your product or service clearly communicate the value of your product or service clearly. Key Elements of a Successful Landing Page Compelling Headline: Grabs attention and states the offer clearly. Strong Call-to-Action (CTA): Actionable and prominent (e.g., "Sign Up Now"). Minimal Design: Focused layout with no distractions or navigation. Relevant Content: Directly related to the marketing campaign or ad that led users to the page. Best Practices Run A/B Testing: Experiment with different elements (headlines, design, and CTAs) to see what works best. Minimize Navigation: Minimize navigation menus or links to reduce distractions and keep users focused on the CTA. Maximize Readability: Keep text concise and use white space effectively to guide users' eyes to the CTA. Be Consistent: Ensure the landing page content aligns with the ad or email that directed users to the page. Make the CTA Stand Out: Use contrasting colors and place multiple CAs on the page for easy access. User Targeting: Tailor landing pages to specific customer segments based on their stage in the marketing funnel. Simplify the Required Action: Keep forms short and only request essential information like name and email. Focus on the Customer: The content should highlight the benefits to the customer, not just your company's achievements. Place Key Content Above the Fold: Ensure important elements, like the CTA and headline, are immediately visible without scrolling. Make It Mobile-Friendly: Optimize the page for mobile users by ensuring it loads quickly and displays properly on smaller screens. Effective Landing Pages Example: LinkedIn Clear Value Proposition People-Centred Imagery Concise and Engaging Copy Netflix Low Friction Sign-Up Short-Form Content Effective CTA Airbnb Clear Value Proposition User-Friendly DesignPersonalization Social Proof Lecture 3 – 18/09/24 User Psychology In web design, particularly for landing pages, understanding user psychology is crucial. Psychological studies help us grasp how users think, behave, and make decisions, enabling us to craft structures that effectively communicate our message and achieve our goals. By applying these insights, we can design landing pages that influence user interactions and guide them toward desired actions. Key Points User Behaviours Insights: understand how users navigate and process info Emotional Impact: use colour theory and design elements to evoke the right emotions. Decision-making: implement principles like reciprocity and FOMO to encourage user engagement Eyetracking Eye-tracking studies monitor where users look and for how long It uses sensors or cameras to detect eye movements, tracking where users focus on a page This data helps designers understand which elements of a page attract the most attention Key Findings from Eyetracking Studies Studies showed that users often follow an F-shaped pattern, focusing more on the top and left parts of the page. At the top left usually there is the title or the logo, so the first focus is on trying to present the brand to the user and make it recognizable. This visual hierarchy is essential: large or bold elements attract more attention. Users scan the page rather than reading it fully. Best Practices from Eyetracking Studies Place important info and CTAs above the fold (the part of the page visible without scrolling). Use bold headlines and images to grab attention quickly Ensure that most important elements follow the F-pattern Colour Psychology Colours are more than just aesthetics, they can evoke specific emotions and can influence user behaviour. That’s why colour choices play a crucial role in how users experience and interact with digital interfaces. A well-designed colour palette can transform an interface from functional to emotionally engaging. Each colour has its own emotional impact: We have to choose a colour palette that must express what we are trying to share with the customers, other than being aesthetically pleasing. White is a particular colour bc it is generally used by many brands bc it conveys transparency and clearness. Cultural Significance of Colours We said colours have an emotional impact on us, but this impact is also influenced by our traditions and culture. Indeed the meaning of colours varies across cultures: - White: purity in western cultures, but mourning in some Asian culture - Red: May signify danger or celebration depending on the region. Cultural sensitivity is crucial when designing global interfaces. You should consider these cultural differences when choosing the colour palette for your website. Using colours for hierarchy Colours can hierarchize info, guiding users through the interface: - Contrasting colours can highlight the primary actions or important elements - Softer hues help differentiate secondary content Conscious and effective use of colours helps to create intuitive navigation and focus user attention on key areas. Best practices from colour psychology - Use contrasting colours for CTAs to make them stand out - Match your brand’s colour scheme with the emotions you want to evoke in your users - Avoid using too many colours, which can overwhelm the user Case Study: Start-up “EcoLife” o Industry: Eco-friendly consumer products (e.g., reusable water bottles, biodegradable packaging, sustainable home goods) o Target Audience: Environmentally conscious consumers, ranging from young adults to middle- aged professionals who prioritize sustainability. o Mission: To promote sustainable living by offering eco-friendly alternatives to everyday products, reducing plastic waste, and encouraging a greener lifestyle. o Core Values: Sustainability, innovation, community, and responsibility. Think about EcoLife colour palette. Green bc it reminds us of ecology, nature but also bc green by users is associated with health, eco- friendly, sustainability, freshness, growth. Yellow for the buttons. Case Study: Startup "FitFuel" o Industry: Health and fitness (providing personalized meal plans and nutritional supplements for athletes and fitness enthusiasts). o Target Audience: Active individuals and athletes aged 18-40 who prioritize fitness, health, and performance. o Mission: To fuel athletic performance through scientifically-backed nutrition, offering high-quality supplements and meal plans that help users reach their fitness goals. o Core Values: Energy, strength, vitality, and performance. Red, Black, Orange à colours that convey vitality, optimism and activity. Additional User Psychology Studies Þ Reciprocity Principle: offering something of value (like a free eBook) encourages users to reciprocate (e.g., providing their email). We are trying to build the trust of our users. Þ Fear Of Missing Out (FOMO): Limited-time offers create urgency, pushing users to act quickly. UX Principles & Web Design Step Web design determines the look, feel, and structure of a website. A good website is one that displays a careful balance between appearance and functionality. Principles and standard in web design are provided to acquire those analytical, critical and problem-solving skills essential to create a quality website. Visual elements principles Þ Visual Hierarchy: defines the importance of elements on the page. Using sizes, colours and positioning allows the user to scan the contents of a page more easily and quickly identify which info is most relevant. Þ Balance: when navigating a web site it is essential to ensure that the design is harmonious. Distributing elements evenly on the page, considering both symmetrical and asymmetrical balance, leads to providing an overview of the site that combines aesthetics and functionality. Þ Repetition: using repeated elements like colours, shapes, or text styles creates coherence and a sense of unity in the overall design of the site. In this way you create a memory for the user. (e.g., buttons with the same style throughout the site helps maintain a cohesive look and facilitates recognition of the function). In this way you facilitate the recognition of the website. Þ Contrast: essential for capturing the user’s attention and guiding them through the site. It can be used to create significant differences between elements on a page, colors, sizes, and font formatting. Þ Spacing: often the most overlooked but crucial principle. Good spacing between elements improves readability and understanding. Avoiding overcrowding of texts, buttons, images allow users to distinguish elements more easily on the page. Þ Colour: Used to aid comprehension, give structure and hierarchy, evoke emotions or convey messages, give coherence, increase readability and accessibility through contrasts. Choosing which colours to use requires quantitative reflection and strategy. A well-studied colour palette should result from a limited choice of colours to use. Colour pairing types There are some studies trying to give us the instruments to use colours in a logic and effective way. There are different types of pairing to structure a functional and aesthetically pleasing design. Monochromatic scheme: use different shades of the same colour. Complementary scheme: we take 2 opposites colours. The 60/30/10 rule The 60/30/10 rule is used to provide visual clarity, facilitate navigation, and ensure that key elements are adequately emphasized without creating confusion. This rule suggests dividing the colour palette into three proportions: o 60% - Dominant Colour: constitutes the majority of the palette and is used for backgrounds, basic elements, and the overall design structure. o 30% - Secondary Colour: contributes to creating variety and balance. It can be used for specific sections of the website or to emphasize certain elements. o 10% - Accent Colour: is used for key details that you want to be particularly noticeable, such as call-to-action buttons, links, or interactive elements. Typography Plays a fundamnetal role I nthe visual communication of a website, influencing the readability and perception of content. Two fundamental aspects of typography in websites are hierarchy and combination: 1. Typography hierarchy in three levels (main headings, subheadings and supporting text, body text) 2. About the font combination, a key rule is to opt for fonts that harmonize with each other, avoiding excessively contrasting combinations that may appear messy Fundamental elements principles Responsiveness With the increasing use of mobile devices, websites should be designed to be responsive, meaning they adapt and display correctly on various screen sizes and devices. Responsive design ensures a consistent user experience across different devices. Accessibility Websites should be designed to be accessible to all users, including those with disabilities. This involves providing alternatives for non-text content (such as images and videos), ensuring compatibility with assistive technologies (such as screen readers), and following accessibility standards (WCAG). Web Content Accessibility Guidelines (WCAG) is a set of rules that define how to make Web content more accessible to people with disabilities. Usability Websites should be easy to use and navigate, allowing users to find the information they need quickly and efficiently. Usability principles emphasize simplicity, clarity, and intuitive navigation. Jakob Nielsen, a usability expert and co-founder of the Nielsen Norman Group*, invented several usability methods, including the ten heuristic evaluation principles of usability. *The Nielsen Norman Group is a renowned UX research and consulting firm that has significantly impacted the field of web and software design. UX best practices for Landing Page UX principles for a Successful Landing Page Simplicity: Keep the design clean and focused. Avoid clutter that distracts users from the CTA. Consistency: Use the same visual elements (colours, fonts, button styles) throughout the page to avoid confusion. Visual Hierarchy: Arrange content in a way that leads users naturally to the CTA. Impact of UX on Landing Page Design Good UX design increases conversion rates by making it easy for users to navigate the page and take the desired action. A seamless, user-centred design helps retain users and reduces bounce rates. Dos and Don’ts recap Do: Keep the message clear and concise, and use visual hierarchy to guide users. Don’t: Overwhelm users with too much text or distract them with unnecessary links or images. Web Design steps The importance of planning Creating a website that stands out requires a strategic approach and meticulous planning. Web Design follows an iterative method, with designers continuously evaluating and adjusting to improve usability and appeal. Essential Web Design Statistics Attention Span: On average, humans have an attention span of 8 seconds. Prioritizing ease of use and navigation is essential. Visitor Retention: 70–80% of visitors are likely to stay longer on a website with appealing design. Functionality Perception: According to 48% of users, a malfunctioning website reflects poorly on the business. Ensuring operational efficiency is paramount. Engagement Impact: 38% of users will disengage with unattractive content or layout, emphasizing the significance of aesthetic appeal. First Impressions: 92% of consumers consider initial impressions crucial when visiting a website for the first time, highlighting the importance of a captivating design. Mobile-Friendliness: 67% of consumers are more inclined to purchase from a mobile-friendly site, underlining the necessity of mobile optimization. Recommendation Potential: 59% of internet users would only recommend a business with a visually appealing mobile website, emphasizing the role of mobile design. Crucial Steps in the Web Design Process Setting the Right Goal Before designing the landing page, define the primary goal: What do you want users to do? (e.g., fill out a form, make a purchase). All elements on the page should drive users toward this goal. Design the Landing Page Content visualisation Creation of a cohesive visual style. It is important to ensure that visual elements are professional, responsive, and consistent with the overall design of the website. Development and testing Translation of design and project into a functional web page. The testing phase is critical to verify functionality, compatibility, and responsiveness across devices and browsers. Data Storytelling Data storytelling shares complex data through narrative. It informs and influences specific audiences effectively. Utilizes principles of rhetoric and narratology. Aims to simplify data comprehension and promote transparency. Key elements of Data Storytelling Narrative: Transforms complex information into stories. Visuals: Illustrate and make the story vivid. Data: Forms the basis of the narrative and supports it. Benefits of Data Storytelling Makes data easily understandable. Contextualizes data and explains significance Improves data memorization and engagement. Converts boring data into compelling content. Enhances relationships with users. Facilitates collaboration and speeds up decision-making. Data Storytelling Examples Spotify Wrapped Guns Periscopic project Giorgia Lupi The Pudding Lecture 4 – 24/09/24 WordPress Intro to WordPress Basic concepts What is a Web Platform? A web platform is the environment that allows you to build and run websites. Web platforms provide the tools and infrastructure needed to create everything from simple blogs to complex websites. Examples include WordPress, Wix, Squarespace, and Shopify. What is a CMS? A Content Management System (CMS) is a software that helps users create, manage, and modify digital content on a website without requiring extensive coding knowledge. What does open-source mean? Open-source refers to software or a project where the source code is made freely available to the public for use, modification, and distribution. Unlike proprietary software, which is controlled by its creator, open-source projects encourage collaboration from developers around the world. Anyone can contribute improvements, fix bugs, or adapt the software for their specific needs. What is Web Hosting? Web hosting refers to the service that makes your website accessible on the internet. When you create a website, all the files (images, code, etc.) need to be stored on a server that’s connected to the internet. Hosting providers rent out space on their servers to store these files. Types of Web Hosting Shared Hosting: Multiple websites share the same server, which makes it affordable but can result in slower speeds if traffic increases. VPS Hosting: A virtual private server with dedicated resources but still in a shared environment. Dedicated Hosting: An entire server is reserved for your website. Cloud Hosting: Scalable and flexible, pulling resources from multiple servers. What is WordPress? WordPress is a free, open-source Content Management System (CMS) that allows users to create and manage websites easily without needing to code. Originally designed for blogging, WordPress is now used for building all types of websites, from personal blogs to large e-commerce platforms. WordPress is the most popular CMS, used by over 40% of websites worldwide. Why WordPress? Easy Customization SEO-Friendly Responsive Design Integration with Marketing Tools (e.g. Mailchimp, Analytics) ~ third-level domain subdomain What is a Page Builder? A Page Builder is a tool that allows users to design and customize the layout of web pages without needing to write code. These tools offer drag-and-drop functionality, making it easy for non-developers to visually create, arrange, and style elements on their site, such as text blocks, images, buttons, and more. In fact, as Alan Turing included in his paper, a machine will never be kind, resourceful, beautiful, friendly, so like a human being. It will never make mistakes. This was already in the seminal paper of Alan Turing (1950), when he wrote: “A machine will never: be kind, resourceful, beautiful, friendly, have initiative, have a sense of humour, tell right from wrong, make mistakes, fall in love, enjoy strawberries and cream, make someone fall in love with it, learn from experience, use words properly, be the subject of its own thought, have as much diversity of behaviour as a man, do something really new” The Turing idea was that in order to be intelligent, you had to be like this. You could not make mistakes. The new idea of AI in these days is that in order to be intelligent you need the flexibility to make mistakes in order to learn something new. Just drawing down logical rules does not make the machine intelligent. The idea was to introduce probabilistic concepts within a deterministic framework, like that of Turing machines. However, this could never replace us. We are intelligent because we make mistakes — ChatGPT does mistakes. We had to inject uncertainty in the system, the probability in the logical reasoning. This is at the base of machine learning, in order to learn something you need the machine to experiment, make mistakes and adjust itself. This is the way our brains work. Our parents will give us examples and we will then make mistakes and adjust our answers based on what we learned. “On Intelligence” — the idea is to emulate our brains in order to configure machines which can produce an intelligent way of thinking. 7 This has to do with the complexity of the systems. Here you have Facebook which is a set of Che complex interactions between people and then the schematisation of a brain, which has neurons and significal electrical impulses that travel across the brain. These are two kinds of cognitive systems. It is a commonality between AI and complex systems, like social networks or real social systems or transportation networks. This is the situation right now: we are providing a lot of fresh personal data to machines in order to let them learn something new. We are feeding them to train them. At the beginning, machines are empty, there is no intelligence. So you must feed them with data and algorithms to extract information from data. They use data as examples. They are studying, reading, watching at videos and so on. And we are constantly producing them for them to eat. After the 50s (AI winter) we have the - new AI summer which is due to: I. We went beyond the idea of simple neural networks, because we have discovered deep learning. There are many more layers. New kind of algorithms emerged in the 80s and are related to deep learning neural networks. II. GPUs — for capitalisation one of the first companies in the world is Nvidia which is a GPUs factory in Taiwan. The graphic processing units are the right tools to run these kinds of algorithms. GPU III. Big data — without the availability of these amounts of data, it is impossible to train AI. Overview of the different kinds of data available online: 21 A. Physical devices, like smartphones. We are constantly connected, even if the GPS sensors is switched off, you are localised through antennas with the precision of 30/40 meters. Our position is constantly followed by social networks. B. For extension, we have IoT. The smartphone is connected, but you have also other devices like smartwatches, smart cities to measure temperature, cameras, and all of them are connected to the internet. Instead of having just website pages, we have replications of physical things. Sensorisation of the things to create their digital replicas. C. Open data movement — semantification of open data. National institutions such as ISTAT and EUROSTAT provide data for free which is available for everyone. D. Social network — big data comes especially from social media. There is a live website which tells in real time the amount of data created by each social networks in one second. Of course, there are privacy concerns with these systems. You are providing your data and accessing these systems for free. And they are using these data for mainly two things: I. Train machines II. Targeted advertising They looked at the presence of people in Cagliari based on their connections to Facebook. Facebook constantly follows you even thought the GPS is switched off. If a restaurant has a Facebook page and it is close to you, Facebook will emit a check in which will be anonymous. We do not know who exactly is there, but Meta gathered data and was able to see the population density each 15 minutes in different areas of a city. This can then be done for geomarketing, or also to check on the tourism impact. How machines learn? I. Get data II. Clean, prepare and manipulate data — certain columns have a special role, this is the shape of a dataset and you must check the data. Maybe you upload an excel file and the column is supposed to be numerical but it is checked as string, or you have missing data. III. Train models — you provide the data as an example of something. Imagine that you want to teach the machine to distinguish between cats and dogs, thus provide a lot of images of dogs and cats so that they can store the data. IV. Test data — we must test to make sure that the machine has learned properly this concepts. Machines can fail, this is why we test. V. Improve — we can fine-tune parameters to get the best configuration of the model which will be able to understand our data. The knowledge must be included in the data provided, if you provide just images of cats and dogs, it won’t be able to recognise cows. It is limited to the kind of knowledge you provided Different kinds of learning we have (main subdivision of algorithms and training models): I. Supervised learning: the main kind of learning is classification II. Unsupervised learning: the main kind of learning is clustering 22 III. Reinforcement learning Supervised learning is classification. You start with the input raw data, in this case they are fruits. In classification you know at the beginning the classification of the inputs that you have. You have the knowledge attached to your objects. This is why we call it supervised it, because the human being is classifying them for the machine. This machine will understand through these examples how to classify them. Unsupervised learning we have input data, but we do not have any label attached to each of these objects. They collect and group objects according to their similarities. The result is a cluster (statistics). You have some features related to the object and then you compute the different similarities in order to group them together. Then there is the reinforced learning which is used for ChatGPT and the idea is that you let the machine to explore the environment freely and there is a reward function. Imagine a trivial machine which goes around and learns by looking at things and experiencing things and having a feedback and adjust its behaviour by reacting with the environment. Machine learning — high level process 1. Data pre-processing — Clean up data and perform appropriate transformation 2. Feature selection — Selecting the most important variables able to solve the problem 3. Modelling — Compare multiple models looking for the most suited 4. Validation — Measure performances with different test data 23 5. Deployment — Deploy the best model with the selected features Iris flowers case in which we have the iris flower in three species which differ from each other for some features. These three species are versicoloured, setosa and virginica. We do not have pictures right now, but another way to characterise each specific flower we have. Imagine having 150 flowers of different species. In this specific case we have 50-50-50 instances of each species. The way we characterise each flower (features we use) matters. We had 4 features: length and width of sepal and petal. The machine will be able to read the feature, knowing the answer, it can calculate the probability of each flower of being a certain species and deliver an answer. There are different models able to gather this information and then after the learning process, you present an unlabelled flower of which you provide all the features and with high probability this machine will be able to give you an answer. In clustering, at the beginning you do not have labels. Even if we do not have the labels, data can reveal them forming the clusters. Training and testing — when you have a dataset like this one (150 instances equally divided), you have to split the dataset into two parts. The first part is the training dataset, while the other will be the testing part. Otherwise, the accuracy of the machine cannot be tested. Usually accuracy is not 100%, the machine can fail but if you have 90% it is not that bad. We have two features because it is a bidimensional space while the color is a label. The model is just to separate the two clusters — just draw a line between them and then establish that below the line are blue and the ones above are red. This line is the line of best fit — margin support machine which is equidistant between the points. We have two main types of parameters: I. Internal parameters of the model (in the case of the line they are a and b y=ax+b) which are automatically optimised through the learning process. For neural connections we have neurons with connections between them, and then we have weights associated with these connections. So you provide a lot of data, instances, features and there is a very complicated process inside these models which optimise this internal parameter. II. Then we have hyper parameters to fine-tune the model such as regularisation parameters, parameters to choose the training sets and so on. Lecture VI We design a process with widgets connected to a workflow. In classification the key idea is that of being able to separate things in a multidimensional space. You have a problem and do not know at the beginning which model to use, thus you test different models. You must connect the test and score widget to the model to test it and also to the data. O The best value of performance in 1, so 0.997 is very good. “Prec” is percentage. We extracted a portion of this dataset and tested the model on this portion. 24 7 In a dataset you have features, then a target data which is the data the machine has to guess. In this case, we could use gender. Then we have metadata. Metadata means "data about data". Metadata is defined as the data providing information about one or more aspects of the data; it is used to summarize basic information about data that can make tracking and working with specific data easier. SVMs are commonly used within classification problems. They distinguish between two classes by finding the optimal hyperplane that maximizes the margin between the closest data points of opposite classes. The number of features in the input data determine if the hyperplane is a line in a 2-D space or a plane in a n-dimensional space. Since multiple hyperplanes can be found to differentiate classes, maximizing the margin between points enables the algorithm to find the best decision boundary between classes. This, in turn, enables it to generalize well to new data and make accurate classification predictions. The lines that are adjacent to the optimal hyperplane are known as support vectors as these vectors run through the data points that determine the maximal margin. Lecture VII Machine learning 8 vs deep learning: you need features in the beginning, you need an expert able to label your examples and your dataset (supervised learning). … and colleagues managed to automatically - - extract feature form the input, in many cases it is very difficult to extract features and he was able to do so with deep learning (deep means many knowledge layers). Machine Learning (ML): A branch of AI that enables computers to learn from data and make decisions or predictions without being explicitly programmed. It focuses on algorithms that learn from structured data to perform tasks like classification, regression, and clustering. - Deep Learning (DL): A subset of machine learning that uses artificial neural networks (ANNs), particularly deep neural networks with many layers, to model complex patterns in data. Deep learning excels in tasks that involve unstructured data, like images, speech, or natural language. 25 A Convolutional Neural Network (CNN) is a type of deep learning model primarily used for analyzing visual data like images and videos. CNNs are widely used in tasks such as image recognition, object detection, and facial recognition. The fundamental idea behind convolution is to detect local patterns in the input data. Unlike traditional fully connected layers where each neuron is connected to all inputs, in a convolutional layer, neurons are connected to local regions of the input image, called the receptive field. Then there is the contribution of Hopfield which is one of the first examples of neural networks and the point is that this is connected to the isin model. Giorgio Parisi got the Nobel prize for the study of the complex systems. Classification — “Eventually, the performance of a classifier—computational performance as well as predictive power—depends heavily on the underlying data that is available for learning” Supervised means that you have a labelled dataset in order to train the machine. 1. Selecting features and collecting labeled training examples 2. Choosing a classifier and optimisation algorithm 3. Choosing a performance metric 4. Tuning the algorithm (through hyper-parameters) 5. Evaluating the performance of the model At the beginning you could have many features, so we have to select them, select subsets of both the features and instances. If you have less instances the final outcome will be less efficient. You are not guaranteed that a model is the best, you have to undergo model selection, you have to test more than one classifier (trial and error process). Then we have internal parameters (automatically adjusted during the optimisation process) and macro/hyper parameters (which have to be fine-tuned by hand). Then we can evaluate the global performance of the model. We have many performance statistics, but these are the most important ones: I. Classification accuracy is the proportion of correctly classified examples II. Precision is the proportion of the true positives among instances classified as positive, e.g. the proportion of Iris Virginia correctly identified as such; (disregards true negatives) — TP Precision = TP + FP III. Recall is the proportion of the true positives among all positive instances in the data, e.g. the TP number of sick among all diagnosed as sick; Recall = TP + FN IV. F-1 is a weighted harmonic mean of precision and recall. V. Area under ROC (AUC) is the area under the receiver-operating curve. AUC provides an aggregate, measure of performance across all possible classifications. Logistic regression (for classification) — p is the conditional probability that a particular example belongs to class 1 given its features. You are trying to connect features to probability. We get a 26 sigmoid meaning that if that is related to the features you will have 1, below 0 it is in the class 0. It is a kind of filter. Then you have to introduce the cost function to minimise the distance of the true result with the function connected to the features. The idea is trying to optimise the w (internal parameters) in order to minimise the distance between features and the true result (Y). After this, you will have the proper combination of internal parameters which are the main ingredients of the model. λ In some cases you have to add to avoid overfitting. Overfitting is when the line tries too hard to fit 2 in the data that no longer becomes statistically relevant. It is too tied to the sample. We cannot λ generalise then. In order to avoid this effect, we add a parameter which is. 2 27 When we generate the coloured fields (which act as classifiers) we will introduce a new sample which will be classified immediately. Then we have support-vector machines. 28 - Regularisation is the parameter which regulates the overfitting effect. You can also have two kinds of regularisations lasso and ridge regularisation. We are teaching the machine through the examples. Hyper parameters let you change the performance of the model. C is the inverse of lambda. Ridge regularisation helps with this by adding a rule that says: "Don't let the model's weights (the numbers that decide how important each input is) get too big." The model still learns, but this rule forces it to spread out the importance across all the inputs. The key point is that none of the weights are reduced to zero—they just get smaller. Lasso does something similar but goes a step further. It adds a rule that says: "It's okay if some inputs don’t matter at all, let's set their importance (weights) to zero." So, in addition to keeping the weights small, Lasso can make some weights exactly zero, which means the model will ignore those inputs altogether. This is like picking only the most important features. Think of C as a dial that controls how strict these rules are. If C is a small number, the model gets ? E punished more for big weights (strong regularisation). If C is large, the punishment is weaker, and the model is allowed to put more weight on some inputs (weak regularisation). So: - Ridge keeps the model from relying too much on any one input but uses all of them. - Lasso not only limits how important inputs can be but can also remove some inputs entirely. - And C controls how strictly the model follows these rules. Then we can introduce SVM — Imagine you have two groups of points in a 2D space, and you want to draw a line that separates them into two classes. An SVM tries to find the best line (or hyperplane in higher dimensions) that not only separates the groups but also keeps them as far apart as possible from the line. This "best line" is chosen to maximise the distance between the closest points of each class and the line. This distance is called the margin. - The last part of classification is to introduce an instance which is not in the model and to check whether the model is able to classify it. Decision tree — Based on the features in our training dataset, the decision tree model learns a series of questions to infer the class labels of the examples. Using the decision algorithm, we start at the tree root and split the data on the feature that results in the largest information gain (IG). In an iterative process, we can then repeat this splitting procedure at each child node until the leaves are pure. The lower the impurities of the child nodes, the larger the information gain. Decision trees — it is very powerful, especially in the version of the random forest. ↳ How it works: - Start with one big group of data. - Ask a question (like "Is the age over 30?”). - Split the data based on the answer. 29 - Keep asking questions and splitting until you can't split anymore or you're happy with the groups. - At the end of the branches, you make your prediction. Advantages: - Easy to interpret and visualize. - Handles both numerical and categorical data. Disadvantages: - Prone to overfitting, especially if not pruned or regularized. A random forest can be considered as an ensemble of decision trees. The idea behind a random forest is to average multiple (deep) decision trees that individually suffer from high variance to build a more robust model that has a better generalisation performance and is less susceptible to overfitting. How it works: - Grow lots of different decision trees by using random parts of the data. - Each tree makes its own prediction. - The final answer is the one that most trees agree on (for example, if most trees say "yes," the forest says "yes"). Advantages: - High accuracy and robustness. - Less prone to overfitting due to averaging over many trees. - Handles missing values well. Disadvantages: - More complex and less interpretable than a single decision tree. - Requires more computational resources. k-NN — is a simple, non-parametric supervised learning algorithm used for both classification and regression. It works by finding the "k" closest data points (neighbors) in the feature space and using them to predict the target variable. 30 How it works: - Choose the number of neighbors (k). - For a given data point, compute the distance (usually Euclidean distance) between it and all other points in the dataset. - Select the "k" closest data points. - For classification: Predict the majority class among the k neighbors. - For regression: Predict the average value among the k neighbors. Advantages: - Simple and intuitive. - Works well with small datasets. - No training phase; it’s a lazy learning algorithm, as it simply memorizes the training data. Disadvantages: - Computationally expensive at prediction time, as it requires calculating the distance to all other points. - Sensitive to the choice of k and the scale of features. - Doesn’t work well with large datasets or high-dimensional data unless properly optimized. Lecture 15/10 … You also add the classes (colours and shape of the colours). Even for clustering we will need our points embedded in a space. We need some kind of metric to measure the position of the point in the space. Having a point in a space allow us to calculate distances. In networks you do not have points in space but just connections — topology. We have points and instances with some features, in a bi-dimensional space you have 2 features. The difference between classification and clustering is that you do not have a class. Maybe you do not have it because you do not, maybe you have to discover some kind of aggregation in the dataset. 31 This is also an idea on how to face a clustering problem just starting from a dataset like this one, just eliminate one column. In this case, we must leverage on the embedding in the space in order to discover these clusters. Classes tend to be aggregated instances. The idea is to have a several number of instances without classes and you are trying to measure the dissimilarity and similarity differences between instances. In order to do this, you need a set of feature. Imagine to embed each instance in a space of features and then couple by couple we need to measure the distance to define which points can be clustered all together. We do not need any expert which needs to label the dataset, so we do not know the right answer upfront. The idea is to cluster (find a natural grouping of the items) and to put together the points which are similar to each other. “Clustering, a category of unsupervised learning techniques, allows us to discover hidden structures in data where we do not know the right answer upfront. The goal of clustering is to find a natural grouping in data so that items in the same cluster are more similar to each other than to those from different clusters.” There are several algorithms : I. Finding centers of similarity using the popular k-means algorithm II. Taking a bottom-up approach to building hierarchical clustering trees III. Identifying arbitrary shapes of objects using a density-based clustering approach K-means — the intuition is that you decide for a certain number of centroids (centres of hypothetical clusters) and the bad news is that you do not know in advance the number of clusters. In the case of k-means you need to put as input data the number of clusters you want. The first choice is to put the k-centroids randomly. You decide 3 random points and decide to start with them. Then you have to assign each point to the closest centroid. After you have to move the centroids in order to minimise the differences between the points and the centroids (using first euclidean formula and SSE). Then you have to repeat the process until you reach a stable solution. 1. Randomly pick k centroids from the examples as initial cluster centers 2. Assign each example to the nearest centroid 3. Move the centroids to the center of the examples that were assigned to it 4. Repeat steps 2 and 3 until the cluster assignments do not change or a user-defined tolerance or maximum number of iterations is reached. 32 m 2 (xj − yj )2 = | | x − y | |22 ∑ Similarity d(x, y) j=1 ottimizza la Salta dei n k ↑ centro i di w i, j | | x i − μ j | |22 iniziali ∑∑ SSE SSE = i=1 j=1 {0, otherwise 1 1, if x ∈ j w i, j = - misura la qualità Silhouette score tells us how good is the final result. dei cluster 1. Calculate the cluster cohesion, a i , as the average distance between an example, x i, and all other points in the same cluster. 2. Calculate the cluster separation, b i , from the next closest cluster as the average distance between the example, x i, and all examples in the nearest cluster. 3. Calculate the silhouette, s i , as the difference between cluster cohesion and separation divided by the greater of the two, as shown here: E Vicino a 1 = Buon CUSTERING i bi − ai s = vicillo allo O m a x[b 1, a i ] CANIVO CLUSTERING = Hierarchical clustering is a bottom up linkage — you start with each cluster being each point, then you merge the clusters which are the closest to each other according to a couple of criteria: most similar members (single linkage) and most dissimilar members (complete linkage). 33 You start from this situation, then you start to build the dendrogram and then you start to merge the points according to these criteria. You have different levels and this is the procedure. In order to get and perform this clustering procedure you need an extra widget which contains all the possible distances inside the dataset. This widget is called- distances and after that you can attach hierarchical clustering and can see the clustering. 1. Compute the distance matrix of all examples. 2. Represent each data point as a singleton cluster. 3. Merge the two closest clusters based on the distance between the most similar/dissimilar members. 4. Update the similarity matrix. 5. Repeat steps 2-4 until one single cluster remains. Density-based spatial clustering of applications with noise. You have to introduce 3 kinds of points (noise points are outliers). You have to② decide for a minimum number of points. W have two parameters: mean points and epsilon. In this case, giving epsilon this radius the mean points are 3. If inside epsilon radius you have at least 3 points then it is a core point. OUTLIERS ↑ O G 34 Devi decident : * E : maggio ↓ Mimpts : il minimo considerare per un punto come Core Border point because its radius falls on the radius of a core point. Noise points are all other points which are not border nor core. DBSCAN (procedure) 1. Form a separate cluster for each core point or connected group of core points (core points are connected if they are no farther away than epsilon). 2. Assign each border point to the cluster of its corresponding core point. Lecture 16/10 Dimensionality reduction — “Similar to feature selection, we can use different feature extraction techniques to reduce the number of features in a dataset. The difference between feature selection and feature extraction is that while we maintain the original features when we use feature selection algorithms, we use feature extraction to transform or project the data onto a new feature space.” With scatterplots we were looking for the best features to separate the classes that we had. 35 To better understand the content of the widgets, we need to experiment with some parameters to see how they affect the outcome. However, in the end, it is essential to design a workflow that starts with a choice, like a personal assignment. I’m not asking you to fully grasp the theory, but rather to understand how to work with the tools effectively. For example, when you have a gap in features, it becomes important to select the most relevant features, especially when optimizing for efficiency in your task. The challenge is that it’s not always clear upfront which features are the most significant. One of the strategies we discussed is using a scatter plot to find the best pair of features—variables in a two-dimensional space—that help separate the classes in your data. This is another method for selecting important features. Dimensionality reduction is another key concept. When you reduce the dimensionality of your data, you are reducing the number of coordinates or variables. For instance, instead of having a three- dimensional space, you may project the data into a two-dimensional space. A technical term associated with this is "projection." When you project the data onto a lower-dimensional space, you simplify the dataset while retaining as much of the original information as possible. The goal here is to find directions of maximum variance in the data. This is where principal component analysis (PCA) comes in. PCA helps identify new directions or components that capture the most variance in the data. These new components are ranked based on how much variance they explain. For example, if you project the data onto two dimensions and use the first two principal components, you might retain around 60% of the information from the original dataset. 1. Standardize the d-dimensional dataset - 2. Construct the covariance matrix 3. Decompose the covariance matrix into its eigenvectors and eigenvalues 4. Sort the eigenvalues by decreasing order to rank the corresponding eigenvectors 5. Select k eigenvectors, which correspond to the k largest eigenvalues, where k is the dimensionality of the new feature subspace 6. Construct a projection matrix, W, from the "top" k eigenvectors 7. Transform the d-dimensional input dataset, X, using the projection matrix, W, to obtain the new k- dimensional feature subspace 36 While PCA is powerful in terms of efficiency, it has its drawbacks. One major limitation is that it strips away the original meaning of the variables. In the transformed space, the new components don’t carry the same meaning as the original features, which can make interpretation difficult. When applying PCA, it is useful to check how many components are necessary to explain a large portion of the variance in the data. For example, in a certain dataset, you may find that two components are enough to explain nearly all the variance. This allows you to reduce the complexity of the data significantly without losing too much information. Finally, when working with data, especially during exercises, it is helpful to document your process. This includes using screenshots of your work and describing the results you obtain. Start with basic statistics and distributions, and then move on to more advanced techniques such as classification. Including visualizations and explanations of what you are doing will help structure your report. In today's exercise, we will work with the wine dataset, where you can try different algorithms to compare the results. If you want to change the dataset or modify it for experimentation, you can use the paint widget to alter the original data slightly and see how this impacts your analysis. This process will guide you in selecting the best features, applying classifiers, and understanding the results. Lecture 22/10/2024 Complexity connects everything, there are more levels of complexity (micro and macro). The results of the complexity are intelligence, the topics we dealt with so far explained how to implement, how to transfer in some sense, our intention. And the simplest possible idea of intelligence, of classification and clustering in a kind of artificial brain, there could be a neural network but also a simple algorithm like the SVM. However, there is also another kind of complexity, macro complexity. Such as a social network. O There are transfers of information between micro and macro networks. Someone is always learning from us. So far our datasets were of this kind: we had points embedded in some spaces with L coordinates (we called features). If you have points embedded in the space you can imagine to compute a distance which could be a euclidean distance. So points are described by features. Now we will deal with different kinds of points. Even if you see these points in space, you cannot see 37 coordinates because they are not embedded in metrical space, now in these systems we have connections, not coordinates. So you make it to have elements and then connections. This is a starting point to think about connections. In the simplest case of these networks you do not have features, you just have points (nodes or vertices) and then some kind of connection. You have a complicated system in which you have to solve this extended system. The strategy is to cut the smaller pieces of the system and then trying to get the overall solution as the sum of the solutions of the smaller pieces. When you try to cut or simplifying the problem, you can have some interaction between the pieces and you lose this interaction if you cut the pieces. These can contain some valuable connections (shortcuts) and when these interactions are complex and as important as single elements, you are in 38 a real complex system. From an holistic point of view, you have elements and interactions which have the same importance. You can see organisation networks (employees, processes), social networks (twitter users; the interactions are following, likes, comments and so on). taga e Tabcome There is no preferential direction in Facebook, it is both ways (different from instagram), this is exactly represented by an arrow. Imagine to map some data onto this kind of structure. The idea is that you measure something and what we will do today is introducing something about the role and importance of these networks and try to give a meaning to them. The idea is the following: as we had from classification, we start from data, we try to find relations, map them, then measure something here (some centrality, clustering measures) and try to give some meaning to our results. 39 These are twitter spheres — imagine putting all the connections, for example for a scientific subject. It is relatively easy when you have the proper data, you can generate this representation. This is just due to interaction in content, in mentioning, re-tweetting, liking and so forth. You can also imagine to create a network in terms of co-occurrence, it could also be a semantic network. This is another way to build this kind of network. Co-occurrence networks are graphical representations used to show the relationships between elements (often words, terms, or events) that frequently appear together within a particular dataset or context. These networks help to visualize and analyze patterns of association, making it easier to understand complex relationships between different components. to This is an example of influencer marketing. They generate a network for Diesel, imagine to have all the content related from the fashion brand, bringing about all the interaction of people talking about Diesel. The idea is to have a measure of centrality which can be related to the influencing role of the twitter user who is taking part of the discussion. This influencer, Formichetti, is directly connected to Renzo Rosso, and Formichetti is officially paid by the brand to be an influencer to diffuse knowledge about the brand. But you also see unknown influencers. This is a typical tool for influencer detection. Another interesting application is to study organisations. You need a way to map the interaction between the people. You can do this in many ways, for example, you can provide questionnaires of take data from the daily activity for the employees, for example chat-bots, emails and so on. Or, in this case there was an app (yumi) which asked to disclose, every day, the interactions with other colleagues. To check the difference between the top down structure of the company with respect to the bottom up structure. The interactions which emerge spontaneously are not always the ones you would expect. So, the clustering could be different. So the aggregation, the teams, the force spontaneously can be different from what’s expected. 40 See how locally various aggregations of nodes can interact in order to, for example, represent a kind of efficiency inside a program. You can regard this as an efficient motif if everybody is collaborating with the other people in the team, or, for example you can have an innovation motif if each component of the teams is connected to different sources of information. In this case, you can think of innovative teams. Then, another example, is this. A Pharma brand was launching a new product but had no idea about target segmentation and which type of message to deliver to this target. The idea was to create a network of similarities between elements of the target community and build clusters with personas and brand concepts finally creating the target through the cluster. Personas emerge spontaneously from the similarities of the group of people connected by these concepts. So you have these concepts in order to generate the message. For example, you can pick specific term from here and 41 then we have the idea of personas that are in a sense characterised by this cloud of terms which gets communicated in an organic way. RECOMMENDATION SUSTEMS Another example about networks are recommendation systems. These are networks. Recommender systems are networks in this sense: instead of having just one kind of nodes, you have two: for example, buyers and products. And this system checks the similarity between users because they buy or stream the same movie. So these users are similar if they read the same book, and if this buyer buys this book, you can suggest the same book to the other user that is similar given the previous behaviour. This is collaborative filtering. Then you have content-based filtering, which checks for similarities of items and then trying to suggest something similar to something which has been bought before by the same barrier. This is content-based filtering. These networks are bipartite networks because they have two nodes. Then you have other kind of networks, such as semantic networks or webs. You can imagine to give meaning to the content you have over the web. Imagine to have all the content in Wikipedia which has a specific semantic meaning and you can try to relate all the content you have on wikipedia through a semantic network. Not only network of people but also of contents. 42 Then you can imagine to embed these networks in a smart city environment. Imagine to have more than one of these kinds of networks. You can also have virtual networks for example social network of people that are living and travelling across. So here the partition is that you have a multilevel network in which you have different kinds of networks that can in some way interact with each other. The paradigm is that you have a multilevel network in which you have different interactive networks. This is true for 5G or wifi or other IoT networks. Network Science: a primer. Technicalities — the technical part is that we have this abstract idea of a network. Nodes and links, this is a complex system. The point is that, is there a way to extract information? We are able to characterise the regions and the role of single nodes, and, of course, this system can represent anything (transport, social, chemical, company, etc.). Thus, its measures’ interpretations depend on their use: - Centrality — importance of the node in the system - Global measures — clusters (check for possible aggregation and their meaning, a cluster of people chatting about a product). In the first place, I need to extract data. - Meso-scales — intermediate structures in the shape of motifs. We have three levels: centrality, meso-scales, global measures. Nodes or vertices are the elements, while links or edges are the relations. Then we can add some extra features, for example a notion of the intensity of the interaction, the direction and size of it. You can have directed (Instagram) or undirected network (Facebook); algorithms can change according to this. You can also have the size of the node which is related to the nature you associate with the node. For example, the degree is the basic nature you can associate with a node. A degree is associated with each node and expresses its number of connections with the other nodes. The important numero di connessioni DEGREE = di con altri 43 un nodo Modi measure for twitter is the number of incoming links. If you do not have arrows, you can just count the number of links attached. This is the basic idea of the importance of the nodes. - You can have the out-degree (out-going) and in-degree (incoming) if it is directed. Then you have a brand new idea of a distance, when you have something embedded in space, the distance is the euclidean distance. When you have networks, you do not have any kind of metric. They are just embedded in space. Anyway we can introduce as a kind of measure which is the hop distance. You have to imagine to travel across the links. Of course, you choose the shortest path. This notion lets you calculate the diameter of the graph or network. This is the maximum distance you can have in your system. Distance measures the shortest path between two nodes in a network is the path where the number of hops is minimised. Whereas the diameter associated with a network represents the maximum distance obtainable for each possible pair of nodes. 44 Then you have the notion of density, given a certain number of nodes, how many link you have of all possible connections you can have? Centrality expresses the geometric property of the nodes to be in ‘important’ (central) positions of the network, possibly relevants for the system as a whole. Example from social networks: A. I am an important person because I know important people B. I know an important person through that dear friend of mine C. If I say something I know that many will listen to it D. I hang out with like-minded people Social networks have higher density with respect to technological network. This is useful to classify networks. In social networks we have a lot of clicks because we are induced to close them. If you send a request to follow me and you are followed and following one of my friends, I will likely accept you. This is called assortative behaviour. Assortative behaviour of people — trying to be with people as important as me. The very first centrality measure is just the degree. We have to distinguish between the in-degree and out-degree if we have arrows. 45 Another interesting measure is the closeness centrality. The idea to be barycentric, just in the middle of the network. Rome, for example, is barycentric. Another measure is a complementary one, the betweenness centrality. You can be important because you are in the middle of a densely populated region or because there are a lot of connections, so you are a bridge between different regions. We can measure numerically this bridgedness measure. You have a weight because if you are bridge, a lot of shortest paths will pass through you. So it is measured by computing all possible shortest paths passing through a certain node. Then you have a very important centrality measure: The page rank of a node is the sum of the page ranks of the nodes that surround it. Used by Google (SEO) to shape the content of a webpage in order to be in the highest possible position of the SERP. The ranking was due basically to the connection that the webpage had with other web pages. Thus, the hyperlinks because one web is basically a network. Imagine the web pages as nodes and 46 hyperlinks as connections between pages. So, if a lot of web pages point to a certain page for specific subject, this means that the page is relevant for that subject. more refined algorithm to compute organically the importance of the page. Y This is the idea to be central, to be important. In 1998 it was the most crucial point to emerge as a search engine. For directed networks, such as for web pages (hyperlinks are the arrows). Basically you can just count the incoming links. Here we have a more refined concept of centrality. They use a This webpage has just one incoming link, but it comes from the most liked page. This is why it has a very high page value, because it is not just the number of incoming links, but the fact that these incoming links are coming from a very important page. This was the idea for page ranking in 1998. We can apply this for the influencer network of diesel. Motifs (meso-scales) Then we have communities (or clusters). Clustering is community detection. You have a network and the aggregation of nodes that have a lot of links outside the cluster and many links going inside the clusters. 47 You will have a button to compute the modularity in Gephi and Gephi will get the emerging from any kind of network, just associating power. The first thing I need when working with networks is that we need specific formats for networks, Gephi needs specific network formats. There are many of these network formats. A study about the Italian parliament, the idea was that you can try to connect deputies, and the idea was to see the dynamics inside the parliament by looking at similarities between deputies connected with the votes. They had a very strong connection because they voted the same time in the same way in a certain moment. So it is a kind of similarity network inside the Italian parliament. So it catches the rotation behaviour of the deputies, the dynamics of coalitions inside the party and so on. There are spontaneous coalitions and aggregations that emerged during the parliament rotation. Then we had the separation between clusters, but also the distance, the polarisation. So you have an algorithm to aggregate the cluster, but it also represents the distances and the polarisation, because there is a kind of gravitational algorithm taking into account the strength of the connection. So the cohesion of the parties. The first thing you need to do is rearrange the positions of the nodes in order to reveal the structure. This is the so-called layout. Usually, the lay-outs are related to a kind of gravitational procedure, meaning that nodes that have high weights tend to be attracting each other and form eventually clusters. We can then associate a centrality measure, like the weighted degree. The weighted degree means you sum the weights of all the links that are attached to a certain node, not just the number of links. Lecture 23/10/2024 We related the page rank to influences in social networks, so to the idea to be in the middle of something, to be attractive and to have a lot of connections. To differentiate them, we can use measures such as degree. The other thing we saw, was the clustering part (aggregation of nodes). A cluster is a group of nodes which has a lot of links inside the cluster. In the end we will start with a regular dataset for ML and generate a network through a distance matrix. First thing when uploading data is the layout, good layouts are Forza Atlas 2 e Fruchter… Modularity — a key measure used in network science to quantify the strength of division of a network into modules (or communities). A module is a group of nodes that are more densely 48 connected to each other than to the rest of the network. The modularity measure helps assess how well a network can be decomposed into such substructures. Un valore alto di modularità indica che i nodi all’interno delle comunità sono fortemente connessi tra loro, mentre le connessioni con altri gruppi sono deboli. In the case of a regular dataset in ML, you have points with features that in this case are coordinates. With networks, you basically have same kind of points, but we do not have coordinates because the points are not embedded in space. We instead have specific connections. The idea is that you can think of linking the points in a regular dataset where you have features, numerical features and you can consider the distance. So you can imagine the link to be a distance in that space, so you can generate a network of distances. We already did this for hierarchical clustering. The idea is to have a list of instances, the list of elements that you have in the dataset, and then couple by couple you can generate the distances just using, for example, euclidean distance. Then, if you have a matrix of differences, you can imagine to have a link between each couple of nodes in a form of a link with a weight, and the weight in some sense is related to the distance. You can generate a network out of a generic dataset in which you have some certain number of features and you have introduced a notion of distances to have a complete graph. So each node is connected to all the other nodes with a certain distances. So everything is connected and each node is connected to the other nodes. In order to extract information, you can try to prune edges according to the weight. Lecture 06/11 Blockchain technology was introduced in 2008 as the underlying framework for Bitcoin, the world's first cryptocurrency. It has evolved to support a wide array of applications beyond digital currencies, including smart contracts, supply chain management, and more. Ongoing developments in blockchain technology are paving the way for more scalable, secure, and interoperable blockchain systems, meeting diverse industry needs. Blockchain technology represents a significant leap in the way we handle data and transactions. Essentially, blockchain is a decentralised digital ledger that records transactions across multiple 49 computers, making it extremely difficult to alter. This immutability, or resistance to changes, is a key - feature that supports the security of blockchain systems. With fiat money we just issue more money, with bitcoin it is not possible, to create a bitcoin you have to mine a block (solve a very complex mathematical thing). To create this money you have to do some work. Moreover, bitcoins are limited at 21 millions. Where are we now? - Bitcoin moved a lot of money (more than a half of U.S. debt has moved to bitcoin) moreover the blockchain allowed the creation of NFTs. - Bitcoin (BTC) has generated a total transaction volume valued at over 15 trillion dollars, but the actual value could be even higher, given the continuous growth and global adoption. - The most expensive Van Gogh ever sold is "Portrait of Dr. Gachet," auctioned by Christie's in 1990 for th