EDTE 301: Quantitative Research Methods in Education PDF
Document Details
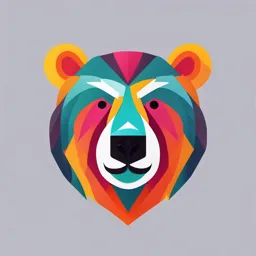
Uploaded by MatchlessHamster2654
University of Ghana
Dr Paul Kwame Butakor
Tags
Summary
This document is a syllabus for a quantitative research methods course in education. It covers topics like introduction to research, quantitative data collection, and research designs.
Full Transcript
EDTE 301 : Quantitative Research Methods in Education Dr Paul Kwame Butakor Senior Lecturer School of Education and Leadership UG [email protected]...
EDTE 301 : Quantitative Research Methods in Education Dr Paul Kwame Butakor Senior Lecturer School of Education and Leadership UG [email protected] 1 Outline Introduction to research Ways of knowing Definition and characteristics of research The research process Research problem and research topic Quantitative and qualitative research paradigms Collecting Quantitative data Variables Sampling terminologies and techniques Data collection tools Reliability and validity Quantitative research designs Experimental Quasi-experimental Correlation Surveys 2 Introduction to educational research: Ways of Knowing Ways of knowing Sensory experience (incomplete/undependable) Agreement with others (common knowledge wrong) Experts’ opinion (they can be mistaken) Logic/reasoning things out (can be based on false premises) Why research is of value Scientific research (using scientific method) is more trustworthy than expert/colleague opinion, intuition, etc. Scientific Method (testing ideas in the public arena) Put guesses (hypotheses) to tests and see how they hold up All aspects of investigations are public and described in detail so anyone who questions results can repeat study for themselves Replication is a key component of scientific method 3 Scientific Method Scientific Method (requires freedom of thought and public procedures that can be replicated) Identify the problem or question Clarify the problem Determine information needed and how to obtain it Organize the information obtained Interpret the results All conclusions are tentative and subject to change as new evidence is uncovered 4 Definition of Research Research is a process of steps used to collect and analyze information to increase our understanding of a topic or issue. At a general level, research consists of three steps: 1. Pose a question. 2. Collect data to answer the question. 3. Present an answer to the question. Using a “scientific method,” researchers: ◆ Identify a problem that defines the goal of research ◆ Make a prediction that, if confirmed, resolves the problem ◆ Gather data relevant to this prediction ◆ Analyze and interpret the data to see if it supports the prediction and resolves the question that initiated the research 5 Importance of Research Research adds to our knowledge Research improves practice Research informs policy debates There are four main purposes of educational research: Exploratory Research - to achieve new insights and formulate research questions; Descriptive Research - to describe some purpose, situation or group; Relational Research - to discover of or how 2 or more variables are related; Explanatory Research - to draw conclusions about the causal connections between variables. 6 Process of Research 1. Identifying a research problem 2. Reviewing the literature 3. Specifying a purpose for research 4. Collecting data 5. Analyzing and interpreting the data 6. Reporting and evaluating research 7 The Research Process Cycle 8 Process of Research Identifying a research problem consists of specifying an issue to study, developing a justification for studying it, and suggesting the importance of the study for select audiences that will read the report. Reviewing the literature means locating summaries, books, journals, and indexed publications on a topic; selectively choosing which literature to include in your review; and then summarizing the literature in a written report. The purpose for research consists of identifying the major intent or objective for a study and narrowing it into specific research questions or hypotheses. Collecting data means identifying and selecting individuals for a study, obtaining their permission to study them, and gathering information by asking people questions or observing their behaviors. Analyzing and interpreting the data involves drawing conclusions about it; representing it in tables, figures, and pictures to summarize it; and explaining the conclusions in words to provide answers to your research questions. Reporting research involves deciding on audiences, structuring the report in a format acceptable to these audiences, and then writing the report in a manner that is sensitive to all readers. 9 Research Problem The most critical factor in the research process is the selection of a suitable research topic, followed by the identification of an appropriate methodology for carrying out the study Sources of research problems, questions or issues exist everywhere: in your professional experience, theoretical writings, and prior research reports. Research problems are initially identified from the literature you have read, from a conference you attended, from a course you have taken, from your supervisor, and/or from a felt need. Statement of the Problem (identify a problem/area of concern to investigate) Must be feasible, clear, significant, ethical Research Questions (serve as focus of investigation) Some info must be collected that answers them (must be researchable) Cannot research “should” questions 10 Research Problem A good research topic should: be researchable (i.e., a question/problem/hypotheses that can be answered/solved through data collection) be important, not trivial and make a contribution to theory or practice or both be realistic, a researchable unit and ethically possible be delimited (having clear boundaries) be worded in a clear and concise statement or question involve interpretation lead to new problems, issues, questions, and further research be able to be broken down into sub-components. These sub-components should: be mutually exclusive yet totally exhaustive imply which data are required, the location of those data, and how they will be collected, analyzed and interpreted. 11 Research Questions Research Questions should be feasible (can be investigated with available resources) Research Questions should be clear (specifically define terms used…operational needed, but give both) Constitutive definitions (dictionary meaning) Operational definitions (specific actions/steps to measure term; IQ=time to solve puzzle, where 7)=? 4 The collection of sample means for all the possible random samples of a particular size (n) obtained from a population is called the distribution of sample means. The distribution of sample means is different from distributions we have considered before. We have discussed distributions of scores. For the distribution of sample means, the values in the distribution are not individual scores, but statistics (sample means). Because statistics are obtained from samples, a distribution of statistics is referred to as a sampling distribution. The distribution of sample means is also called the sampling distribution of mean. 5 General characteristics for the distribution of sample means: The sample means pile up around the population mean. Most of the sample means are relatively close to the population mean. The pile of sample means tend to form a normal shaped distribution. The larger the sample size, the closer the sample means should be to the population mean. We used an overly simplified example to construct a distribution of sample means. However, it will be virtually impossible to construct the distribution by selecting all the possible samples for larger populations and samples. 6 Fortunately, central limit theorem tells us what the distribution of sample means looks like without taking hundreds or thousands of samples. Central limit theorem: for any population with mean and standard deviation , the distribution of sample means for sample size will have a mean of and a standard deviation of and will approach a normal distribution as n approaches √ infinity. 7 Actually, the distribution of sample means will be almost perfectly normal under either of the following conditions: The population from which the samples are selected is normal; The sample size is relatively large, 30 or more, regardless the shape of the original population. The mean of the distribution of sample means, , is equal to (the population mean) and is called the expected value of. 8 The standard deviation of the distribution of sample means, is called the standard error of. The standard error measures the standard amount of difference between M and that is reasonable to expect simply by chance. √ The magnitude of is determined by two factors 1) The size of the sample 2) The standard deviation of the population from which the sample is selected 9 10 A population has μ = 80 with σ = 8. The distribution of sample means for samples of size n = 4 selected from this population would have an expected value of ________. a. 80 b. 8 c. 20 d. 40 11 When the sample size is greater than n = 30: A. The distribution of sample means will be approximately normal and the sample mean will equal the population mean B. The sample mean will be equal to the population mean C. The distribution of sample means will be approximately normal D. None of the other 3 choices is correct 12 A population has μ = 80 with σ = 8. The distribution of sample means for samples of size n = 4 selected from this population would have a standard error of ________. a. 8 b. 4 c. 2 d. 80 13 If random samples, each with n = 25 scores, are selected from a normal population with μ = 80 and σ = 20, and the mean is calculated for each sample, then the average distance between M and μ would be ________. a. 80 points b. 4 points c. 0.80 points d. 20 points 14 A sample of n = 4 scores has a standard error of 12. What is the standard deviation of the population from which the sample was obtained? a. 6 b. 24 c. 3 d. 48 15 If sample size (n) is held constant, the standard error will ________ as the population variance increases. a. increase b. decrease c. stay constant d. Cannot answer with the information given. 16 7.3 Probability and the distribution of sample means The primary use of the distribution of sample means is to find the probability associated with any specific sample. Because the distribution of sample means presents the entire set of all possible means, we can use proportions of this distribution to determine probabilities. For example, the population of scores on the SAT forms a normal distribution with 500 and 100. If you take a random sample of 25 students, what is the probability that the sample mean will be greater than 540? 17 Based on the central limit theorem The distribution of sample means is _______ The distribution of sample means have a mean of _______ The distribution of sample means have a standard error of _______ 18 A sample of n = 16 scores is obtained from a population with μ = 70 and σ = 20. If the sample mean is M = 75, then the z‐score corresponding to the sample mean is ________. a. z = 0.25 b. z = 1.00 c. z = 0.50 d. z = 2.00 19 A sample is obtained from a population with μ = 50 and σ = 8. Which of the following samples would produce the most extreme z‐score (farthest from zero)? a. A sample of n = 4 scores with M = 52. b. A sample of n = 16 scores with M = 54. c. A sample of n = 4 scores with M = 54. d. A sample of n = 16 scores with M = 52. 20 A random sample of n = 4 scores is obtained from a normal population with μ = 20 and σ = 4. What is the probability of obtaining a mean greater than M = 22 for this sample? a. 0.3085 b. 0.1587 c. 1.00 d. 0.50 21 7.4 More about standard error Sampling error is the discrepancy, or amount of error between a sample statistic and its corresponding population parameter. Different samples tend to have different sampling errors. The standard error provides a way to measure the typical sampling error. 22 23 7.5 Looking ahead to inferential statistics 24 Chapter 8: Introduction to Hypothesis Testing 8.1 The logic of hypothesis testing 8.2 Uncertainty and errors in hypothesis testing 8.3 An example of a hypothesis test 8.4 Directional hypothesis tests 8.5 The general elements of hypothesis testing: a review 8.6 Concerns about hypothesis testing 8.7 Statistical power 1 8.1 The logic of hypothesis testing A hypothesis test is a standardized statistical method that uses sample data to evaluate a hypothesis about a population. The logic underlying a hypothesis test is as follows: First, state a hypothesis about a population Before actually selecting a sample, use the hypothesis to predict the characteristic that the sample should have Then, obtain a random sample from the population Finally, compare the obtained sample with the prediction made from the hypothesis 2 Consider a research study that investigates the effect of stimulation during infancy on human development. It is hypothesized that if parents give their children increased handling and stimulation, children should be able to grow faster. The weight for 2‐year‐old children is normally distributed with 26 and 4. Research design: 16 infants with treatment. A hypothesis test is needed to use sample data to answer questions about the unknown population. 3 Four steps of a hypothesis test Step 1: state two opposing hypotheses about the unknown population. Null hypothesis – treatment has no effect : 26 Scientific or alternative hypothesis – treatment has an effect : 26 4 Step p 2: set the t criteeria for a decisioon. To evaluate e the credibility of o the null hypo othesis, w we need d to decide Sample S means that t are likely to o be obttained iff H0 is tru ue Sample S means that t are very un nlikely to o be obtained if H0 is true t 5 To find the boundaries that separate the high‐probability samples from low‐probability samples, we need to define what is meant by “low” and “high” probabilities. We use a small probability to identify low‐probability samples if the null hypothesis is true. This small probability is called the level of significance or the alpha level ( ). Commonly used alpha levels are.05,.01,.001. For.05, we separate the most unlikely 5% of the sample means from the most likely 95% of the sample means. 6 The T extrremely unlikely u values, as defin ned by th he alphaa level, maake up what w is called c th he critica al region n. If I the daata from a reseaarch stud dy produ uce a sample mean that t is lo ocated inn the criitical reggion, whhich is veery unlikkely to t happeen when n the nuull hypotthesis is true, wee will conclude c e that th he data are inco onsistent with th he null hypothe h esis. We will rejeect the null n hypo othesis. 7 Step 3: collect data and compute sample statistics. Select a random sample of infants Train parents to provide additional daily handling that constitutes the treatment for this study Measure the weight for each infant at 2 years of age Compute sample mean Compare the sample mean with the null hypothesis by computing a z‐score that describes exactly where the sample mean is located relative to the hypothesized population mean from √ 8 The sample of n=16 infants produced a sample mean of M=30, 4 26 1 √ √16 30 26 4 1 Step 4: make a decision. With an alpha level of.05, 4 is located in the critical region. We reject the null hypothesis and conclude that the special handling did have an effect on the infants’ weights. 9 The z‐score statistic is called a test statistic in hypothesis testing to simply indicate that the sample data are converted into a single, specific statistic that is used to test hypothesis. sample mean hypothesized population mean standard error between and obtained difference difference due to chance 10 The critical region for a hypothesis test consists of: a) outcomes that have a high probability if the null hypothesis is true b) outcomes that have a high probability whether or not the null hypothesis is true c) outcomes that have a very low probability if the null hypothesis is true d) outcomes that have a very low probability whether or not the null hypothesis is true 11 In a hypothesis test, a z‐score value near zero: a. is strong evidence of a statistically significant effect. b. None of the other options are correct. c. means that you should probably reject the null hypothesis. d. is probably in the critical region. 12 The null hypothesis: a. is always stated in terms of sample statistics. b. states that the treatment has no effect. c. is denoted by the symbol H1. d. All of the other choices are correct. 13 In a typical hypothesis testing situation, the null hypothesis makes a statement about: a. the sample before treatment. b. the population before treatment. c. the population after treatment. d. the sample after treatment. 14 8.2 Uncertainty and errors in hypothesis testing In hypothesis testing, we use limited information from a sample as a basis for reaching a general conclusion. Although a sample usually is representative of the population, there is always a chance that the sample is misleading and will cause a researcher to make wrong inferences about the population. There are two different kinds of errors that can be made Type I errors Type II errors 15 16 17 A Type I error is defined as: a. failing to reject a false null hypothesis. b. rejecting a false null hypothesis. c. failing to reject a true null hypothesis. d. rejecting a true null hypothesis. 18 8.3 An example 8 e e of a hyypothesiis test A stud dent investigatees wheth her pren natal alcohol afffects birtth weighht. 19 Step 1: state two opposing hypothesis about the unknown population. : 18 : 18 Step 2: set the criteria for a decision. 0.05 Step 3: collect data and compute sample statistics. 4 18 1 √ √16 15 18 3.00 1 20 Step 4: make a decision. With an alpha level of.05, 3 is located in the critical region. We reject the null hypothesis and conclude that the prenatal alcohol did have an effect on birth weight. Factors that influence a hypothesis test The size of mean difference The variability of the scores The number of scores in the sample The alpha level 21 Assumptions for hypothesis tests with z‐scores Random sampling Independent observations The value of is unchanged by the treatment Normal sampling distribution 8.4 The hypothesis for a directional test When a researcher begins an experiment with a specific prediction about the direction of the treatment effect, it is possible to state the statistical hypothesis in a manner that incorporate a directional prediction into and. In this way, the hypothesis test is a directional test, called one‐ tailed test. 22 Suppose a research is using a sample of 16 rates to examine the effect of a new diet drug. Under regular circumstances, the distribution of food consumption for rats is normal with 10 and 4 The expected effect of the drug is to reduce food consumption. Because a specific direction is expected for the treatment effect, the researcher can perform a directional test. : 10 (The drug has no effect) : 10 (The drug reduces food consumption) For one‐tailed test, the critical region is located entirely in one tail of the distribution. 23 24 Consider the research study that investigated the effect of stimulation during infancy on human development. It is hypothesized that if parents give their children extra handling and stimulation, children should be able to grow faster. A one‐tail test could be used. Step 1: state two opposing hypothesis about the unknown population. : 26 : 26 Step 2: set the criteria for a decision. 0.05 25 26 Step 3: obtain the sample data. Suppose 4 and 29.5. 4 26 2 √ √4 29.5 26 1.75 2 Step 4: make a statistical decision. For a one‐tail test, with an alpha level of.05, 1.75 is located in the critical region. We reject the null hypothesis and conclude that extra handling did have an effect on infants’ growth. What if we used a two‐tailed test? Can we still reject the null hypothesis? 27 A researcher administers a treatment to a sample of n = 25 participants and uses a hypothesis test to evaluate the effect of the treatment. The hypothesis test produces a z‐score of z = 2.37. Assuming that the researcher is using a two‐tailed test: a) the researcher should reject the null hypothesis with either α =.05 or α =.01 b) the researcher should fail to reject H0 with either α =.05 or α =.01 c) Cannot answer without additional information d) the researcher should reject the null hypothesis with α =.05 but not with α =.01 28 8.5 The general elements of hypothesis testing: a review Hypothesized population parameter Sample statistic Estimate of error The test statistic The alpha level 29 8.6 Concerns about hypothesis testing Two concerns with using a hypothesis test to establish the significance of a treatment effect The focus of a hypothesis test is on the data rather than the hypothesis When you reject a null hypothesis, you can conclude that “this specific sample mean is very unlikely (p 1.96 d. 1 12 The estimated standard error, sM, provides a measure of: a) how much difference is reasonable to expect between the t statistic and the corresponding z‐score. b) how spread out the scores are in the population. c) how spread out the scores are in the sample. d) how much difference is reasonable to expect between the sample mean and the population mean. 13 9.2 Hypothesis tests with the t statistic Population standard deviation is known z statistic Population standard deviation is unknown t statistic 14 For hypothesis tests with a t statistic, we use the same steps that we used with z‐scores. The major differences are We compute a t statistic rather than a z statistic We consult the t distribution table rather than the unit normal table to find the critical region. Consider a research study that tests the effectiveness of eye‐ spot patterns to frighten away moth‐eating birds. 15 Step 1: state two opposing hypothesis about the unknown population. : 30 : 30 Step 2: set 0.05. 8 16 17 Step 3: obtain the sample data and calculate the test statistic. 30 √ 72 3 1 9 1 1 √9 36 30 6.00 1 Step 4: make a statistical decision. With an alpha level of.05, 6.00 is located in the critical region. We reject the null hypothesis and conclude that the presence of eye‐spot patterns does influence behavior. 18 Two basic assumptions of the t test: 1. The values in the sample must consist of independent observations. 2. The population sampled must be normal. With very small samples, this assumption is important. With larger samples, this assumption can be violated without affecting the validity of the hypothesis test. 19 A sample of n = 25 individuals is selected from a population with μ = 80, and a treatment is administered to the sample. Which set of sample characteristics is most likely to lead to a decision that there is a significant treatment effect? a. M = 85 and small sample variance b. M = 85 and large sample variance c. M = 90 and small sample variance d. M = 90 and large sample variance 20 What is the sample variance and the estimated standard error for a sample of n = 4 scores with SS = 300? a. s2 = 100 and sM = 5 b. s2 = 10 and sM = 5 c. s2 = 100 and sM = 20 d. s2 = 10 and sM = 20 21 With α =.01, what is the critical t value for a one‐tailed test with n = 30? a. t = 2.457 b. t = 2.756 c. t = 2.462 d. t = 2.750 22 A sample has a mean of M = 39.5 and a standard deviation of s = 4.3. In a two‐tailed hypothesis test with α =.05, this sample produces a t statistic of t = 2.14. Based on this information, the correct statistical decision is: a) it is impossible to make a decision about H0 without more information. b) the researcher can reject the null hypothesis with α =.05 but not with α =.01. c) the researcher must fail to reject the null hypothesis with either α =.05 or α =.01. d) the researcher can reject the null hypothesis with either α =.05 or α =.01. 23 9.3 Measuring effect size for the t statistic Recall one criticism of a hypothesis test is that it simply determines whether the treatment effect is greater than chance but does not really evaluate the size of the treatment effect. Effect size measures must also be reported, such as Cohen’s : mean difference Cohen’s standard deviation For hypothesis tests with statistic, the population standard deviation is not known. We can use the sample standard deviation in its place. mean difference estimated Cohen’s sample standard deviation 24 An alternative method for measuring effect size is to determine how much of the variability in the scores is explained by the treatment effect. 25 Total variability=396 Variability due to chance=72 Variability due to treatment effect=396‐72=324 26 The proportion of the variability in the scores that is explained by the treatment effect is given by: variability due to treatment 324 0.8182 total variability 396 The value of can also be calculated based on the outcome of the t test: 6 36 0.8182 6 8 44 27 28 9.4 Directional hypothesis and one‐tailed tests The t statistic can also use with a one‐tailed test. Again, consider the research study that tests the effectiveness of eye‐spot patterns to frighten away moth‐eating birds. 9 36 72 Step 1: state two opposing hypothesis about the unknown population. : 30 : 30 Step 2: set 0.05. 8 29 30 Step 3: obtain the sample data and calculate the test statistic. 30 √ 72 3 1 9 1 1 √9 36 30 6.00 1 Step 4: make a statistical decision. With an alpha level of.05, 6.00 is located in the critical region. We reject the null hypothesis and conclude that the presence of eye‐spot patterns does influence behavior. 31 Chapter 10: t Test for Two Independent Samples 10.1 Overview 10.2 The t statistic for an independent‐measures research design 10.3 Hypothesis tests and effect size with the independent‐ measures t statistic 10.4 Assumptions underlying the independent measures 1 10.1 Overview We have learned how to use one sample as the basis for drawing conclusions about one population through the use of or statistic. However, most research studies require the comparison of two (or more) sets of data. For example, An educational psychologist may want to compare two methods of teaching mathematics. A clinical psychologist may want to evaluate a therapy technique by comparing depression scores for patients before therapy with their scores after therapy. 2 For the first example, the two sets of data come from two completely separate samples. The researcher compares the math achievement between two groups of individuals, each receiving a different teaching method. We call this type of design an independent‐measures research design or a between‐subject design. 3 For the second example, the two sets of data come from the same sample. The researcher obtain one set of scores by measuring depression for a sample of patients before they begin therapy and then obtain a second set of data by measuring the same individuals after 6 weeks of therapy. We call this type of design a repeated‐measures research design or a within‐subject design. 4 5 10.2 The t statistic for an independent‐measures research design The goal of an independent‐measures research study is to evaluate the mean difference between two populations (or between two treatment conditions). As always, the null hypothesis states that there is no change, no effect, or, in this case, no difference. : 0 The alternative hypothesis states that there is a mean difference between the two populations. : 0 6 10.2 The t statistic for an independent‐measures research design The basic structure of the t statistic sample statistic hypothesized population parameter estimated standard error of sample statistic For single‐sample design, sample mean hypothesized population mean estimated standard error of sample mean 7 For independent‐measures design, sample mean hypothesized population difference mean difference estimated standard error of sample mean difference 8 Because the hypothesized population mean difference is zero, sample mean difference estimated standard error of sample mean difference 0 The statistic is a simple ratio comparing the actual mean difference with the difference that is expected by chance. 9 We need to know before we can calculate. represents how accurately the difference between two sample means represents the difference between the two population means. sample statistic Population parameter The difference between and are due to two sources of error approximates with some error approximates with some error 10 The typical amount of error when we use to approximate is The typical amount of error when we use to approximate is The formula for calculating the typical amount of error when we use to approximate is 11 In , is an estimate of the variance for population 1,. is an estimate of the variance for population 2,. 12 If we assume that , then we have two estimates of. That is, both and estimate. So we can combine these two to produce a more accurate estimate of. We call this combined estimate as the pooled variance,. Now the formula of becomes 13 The pooled variance is actually an average of the two sample variances taking account of the degrees of freedom. Given that a larger sample presents a better representation of the population, the corresponding sample variance should provide a more accurate estimate of. Therefore, the sample variance for the larger sample should carries more weight in determining. 14 Suppose 50, 30, 6. 50 10 6 1 30 6 6 1 50 30 8 5 5 Suppose 20, 3, 48, 9. 20 10 3 1 48 6 9 1 20 48 6.8 2 8 15 The degrees of freedom for single‐sample t statistic: The degrees of freedom for independent‐measures t statistic: 16 10.3 Hypothesis tests and effect size with the independent‐ measures t statistic Suppose a study that investigates whether the use of mental images can improve memory. A list of 40 pairs of nouns; two groups; only one group taught to form mental image for each pair of nouns; group 1 images : 10 26 200 group 2 no images : 10 18 160 Step 1: state two opposing hypothesis about the unknown population. : 0 : 0 Step 2: set 0.05. 2 18 17 18 Step 3: obtain the sample data and calculate the test statistic. 200 160 20 9 9 20 20 2 10 10 26 18 4.00 2 Step 4: make a statistical decision. With an alpha level of.05, 4.00 is located in the critical region. We reject the null hypothesis and conclude that the use of mental images can improve memory. 19 Effect size: mean difference Cohen’s standard deviation The denominator of Cohen’s is population standard deviation. Although we have two populations, we make the assumption that the two population standard deviations are equal,. Because can be used as an estimate of , 26 18 estimated Cohen’s 1.79, √20 indicating a very larger treatment effect. 20 We can also calculate the percentage of variance accounted for by treatment effect,. 4 0.47 4 18 also indicating a large effect. It is also possible to calculate by using the definitional formula directly. variability due to treatment total variability 21 22 Total variability 680 Variability due to chance 360 Variability due to treatment effect 680 360 320 variability due to treatment 320 0.47 total variability 680 23 10.4 Assumptions underlying the independent measures The independent‐measures t requires three assumptions: 1. The observations within each sample must be independent 2. The two populations from which the samples are selected must be normal 3. The two populations from which the samples are selected must be equal variance. The first two assumptions are also made with single‐sample t tests. The third assumption is referred to as homogeneity of variance. This assumption requires that the two populations being compared have the same variance. 24 The homogeneity of variance assumption is very important. If this assumption is violated, then it does not make sense to use to estimate the same population variance. It is very important to evaluate whether the homogeneity assumption is satisfied before you actually use it. You can visually examine the two sample variances to see whether they are reasonably close. If one sample variance is three or four times larger than the other one, then there is a reason for concern. 25 You can also conduct a statistical test to evaluate the assumption. F‐max test max Compare the calculated F‐max to a critical value from Table B.3 (Appendix B). If the value of F‐max is greater than the critical value, then conclude the homogeneity assumption has been violated. 26 To locate the critical value, you need to know number of separate samples 1 for each sample variance (Note the ‐max test assumes that all samples are the same size) the alpha level. For example, group 1 images : 10 200 group 2 no images : 10 160 200 9 1.25 160 9 With 2, 9, and 0.05, the critical value 4.03. 1.25 4.03, the assumption is satisfied. 27 What if the homogeneity assumption is not satisfied? We cannot use. An alternative procedure is to calculate the t statistic by the following formula, The degrees of freedom is adjusted by , where and 1 2 28 Chapter 16: Correlation 16.1 Overview 16.2 The Pearson Correlation 16.3 Understanding and Interpreting the Pearson Correlation 16.4 Hypothesis Tests with the Pearson Correlation 16.5 The Spearman Correlation 16.6 Other Measures of Relationship 1 16.1 Introduction Correlation is a statistical technique that is used to measure and describe the relationship between two variables. Variables are simply observed. 2 The direction of the relationship A positive correlation: a high score on one variable tends to go together with a high score on the other variable; a low score on one variable tends to be associated with a low score on the other variable. A negative correlation: a high score on one variable tends to be accompanied with a low score on the other variable, and vice versa. 3 4 The form of the relationship. 5 The degree of the relationship A linear correlation measures how well the data points fit on a straight line. The degree of relationship is measured by the numerical value of the correlation. A value of 1 indicates a perfect correlation. A value of 0 indicates no fit at all. 6 16.2 The Pearson Correlation The most common correlation coefficient is the Pearson correlation (r), which measures the degree and direction of linear relationship between two variables. The sum of products of deviations ( ) measures degree of the linear relationship between two variables. To calculate : Find the X deviation and Y deviation for each individual Multiply the deviations to obtain the product for each individual Add up the products 7 8 9 10 One problem with the covariance is that it is not a standardized measure in the sense that it depends on the units of measurement. A standardized measure: the Pearson correlation. X Y 0 2 10 6 4 2 8 4 8 6 11 scores Deviations Squared deviations Products 0 2 ‐6 ‐2 36 4 12 10 6 4 2 16 4 8 4 2 ‐2 ‐2 4 4 4 8 4 2 0 4 0 0 8 6 2 2 4 4 4 6 4 64 16 28 28 0.875 √64 16 12 16.3 Understanding and interpreting the Pearson Correlation Correlation and Causation: a correlation does not imply a cause‐and‐effect relationship between the two variables. Correlation and restricted range 13 Correlation and outliers 14 r2 is called the coefficient of determination. It measures the proportion of variability in one variable that can be determined from the relationship with the other variable. In the similar manner, we use r2 as a measure of effect size in earlier chapters where r2 indicates how much of the variability in the dependent variable in accounted for by the independent variable. We can use the same guideline to interpret the magnitude of r2 here. 15 16.4 Hypothesis tests with the Pearson Correlation 16 Test the significance of the Pearson correlation The null and alternative hypotheses : 0 : 0 statistic can be used to test the null hypothesis: sample statistic hypothesized population parameter estimated standard error of sample statistic The degrees of freedom for the statistic is 2 17 An easier way to find the significance of the Pearson correlation is to compare the sample to a critical value from Table B.6 in Appendix B using the degrees of freedom of 2. For example, when 0.875 and 5, using a two‐tailed test of.05, the critical value is. 878. Therefore, we fail to reject the null hypothesis. 18 16.5 The Spearman correlation The Spearman correlation is an alternative correlation measure. It can be used with ordinal variables It can be used to measure other non‐linear forms of correlation. To calculate the Spearman correlation, First, rank observations for each variable. If tied ranks occur, assign the average of the ranks involved to each score. Then simply using the Pearson correlation formula for the ranks of and. 19 X Y X‐Rank Y‐Rank 3 12 1 5 4 10 2 3 8 11 3 4 10 9 4 2 13 3 5 1 9 0.9 √10 10 Testing a hypothesis for the Spearman correlation is the same as the procedure for testing the Pearson correlation. For the previous example, 0.9 and 5, using a two‐ tailed test of.05, the critical value is 1.00. Therefore, we fail to reject the null hypothesis and conclude that no correlation exists in the population. 20 16.6 Other measures of relationship The point‐biserial correlation is used to measure the relationship between two variables in situations where one dichotomous variable is correlated with one variable with regular, numerical scores. To compute the point‐biserial correlation, First, the dichotomous variable is converted to numerical values by assigning 0 to one category and 1 to the other category Then the regular Pearson correlation formula is used with converted data. 21 22 When both variables to be correlated are dichotomous, the correlation between the two variables is called the phi‐ coefficient. To compute the phi‐coefficient, First, convert each of the dichotomous variables to numerical values by assigning 0 to one category and 1 to the other category. Then the regular Pearson correlation formula is used with converted data. 23 Original data Converted Scores Birth order X Personality Y Birth order X Personality Y 1st Introvert 0 0 3rd Extrovert 1 1 Only Extrovert 0 1 2nd Extrovert 1 1 4th Extrovert 1 1 2nd Introvert 1 0 Only Introvert 0 0 3rd Extrovert 1 1 24 A negative value for a correlation indicates: a. A much weaker relationship than if the correlation were positive. b. Increases in X tend to be accompanied by decreases in Y. c. A much stronger relationship than if the correlation were positive. d. Increases in X tend to be accompanied by increases in Y. 25 Suppose the correlation between height and weight for adults is +0.80. What proportion (or percent) of the variability in weight can be explained by the relationship with height? a. 64% b. 40% c. 80% d. 100 ‐ 80 = 20% 26 For a hypothesis test for the Pearson correlation, the null hypothesis states that: a. there is a non‐zero correlation for the general population. b. the population correlation is zero. c. there is a non‐zero correlation for the sample. d. the sample correlation is zero. 27 Under what circumstances should the Spearman correlation be used? a. All of the other options are appropriate circumstances for the Spearman correlation. b. The Pearson is too difficult to compute. c. The original data are measured on an ordinal scale of measurement. d. The researcher's primary interest is the linearity of the relationship. 28 In what situations can the point‐biserial correlation be used? a. When both X and Y are ranks. b. When an independent‐measures t test would also be appropriate. c. When a single‐sample t test would also be appropriate. d. When both X and Y are dichotomous. 29 UNIVERSITY OF GHANA (All rights reserved) DEPARTMENT OF TEACHER EDUCATION SCHOOL OF EDUCATION AND LEADERSHIP FIRST SEMESTER - 2020/2021 ACADEMIC YEAR COURSE SYLLABUS Course Code and Title: EDTE 301: Quantitative Research Methods in Education Credits: 3 Instructor Lecture Time: Wednesday 9:30 – 11:20 & Dr. Paul Kwame Butakor 1:30 – 3:20 Email: [email protected] Venue: Online Introduction/Course Description This course provides an introduction to quantitative methods in education. It emphasizes how educationists use simple quantitative techniques to investigate research questions coming from education theory, prior research and applied problems. The purpose of this course is to present students with an introduction to descriptive and inferential univariate statistics commonly used in social science research. It will also emphasize three different aspects of statistical reasoning: (1.) computational formulas and assumptions, (2.) computer applications, and (3.) appropriate uses of univariate statistics in educational research. A thorough understanding of the topics covered in this course will prepare students for more advanced graduate work in educational statistics and ensure that students can conduct their own data analyses. The course also introduces statistical software for simple quantitative analysis. Topics include scale and sample types, inferential statistics, normal curve, relations, and interpreting test scores. Learning Outcomes By the end of this course, the student should be able to: Define educational research, give its characteristics and explain why we conduct educational research Recognize and understand various research designs, methods, concepts, and terminology Search for published research articles and develop a literature review focused on a topic of the student’s choice relevant to education especially educational leadership and management issues Explain what is sampling and mention the difference between the two major sampling techniques Identify sources for research data and develop skills in evaluating data gathering instruments such as questionnaires or interviews Identify the various data collection procedures and mention their advantages and disadvantages Demonstrate how to appropriately choose techniques to analyze data and the steps involved in doing so Perform basic analysis using SPSS Demonstrate how to summarize, draw conclusions/inferences from data EDTE 301: Quantitative Research Methods in Education Instructor: Dr. Paul K. Butakor Page 1 Course Delivery: Tutorial sessions Lectures Seminar presentations by students Group discussions Plagiarism policy Plagiarism in any form is unacceptable and shall be treated as a serious offence. Appropriate sanctions, as stipulated in the Plagiarism Policy, will be applied when students are found to have violated the Plagiarism policy. The policy is available at http://www.ug.edu.gh/aqau/policies-guidelines. ALL students are expected to familiarize themselves with the contents of the Policy. Assessment and Grading A combination of formative and summative assessment, including individual presentations in class and group work will be used. Assessment weighting End-of-semester examination 70% Test/Assignment 1 (Individual) 15% Assignment 2 (Group) 15% Grading Scale: You will be graded as follows in line with the university’s grading system: A=80-100%; B+=75-79%; B =70-74%, C+ =65-69%, C= 60-64%, D+ = 55 -59%, D = 50 – 54%, E = 45-49%; F = 0-44% Required Textbook Gravetter, F. J., & Wallnau, L. B. (2009). Statistics for the behavioral sciences (8th Ed). Belmont, CA: Thomson Wadsworth. Creswell, J. W. (2012). Educational research: planning, conducting, and evaluating quantitative and qualitative research. Pearson Education, Inc. Additional Reading List Creswell, J.W. (2013). Qualitative, quantitative, and mixed methods approaches (4 th ed.). Thousand Oaks, CA: Sage Publications, Inc. Mertens, D.M. (2009). Research and evaluation in education and psychology: Integrating diversity with quantitative, qualitative, and mixed methods. Thousand Oaks, CA: Sage Publications, Inc. Ravid, R. (2010). Practical statistics for educators (4th ed.). Lanham, MD: Rowman & Littlefield Publishers. Vogt, W.P. (2006).Quantitative research methods for professionals in education and other fields. Boston, MA: Allyn & Bacon. Wiersma, W. & Jurs, S.G. (2008). Research methods in education: An introduction, (9th ed.). Boston, MA: Pearson. EDTE 301: Quantitative Research Methods in Education Instructor: Dr. Paul K. Butakor Page 2 Tentative Syllabus Week Task/Readings Week Topics Beginning Introduction and course overview Course expectations Attendance policy and, Assessment 1 . Read Creswell chapters 1 Overview of educational research &2 Collecting quantitative data Read Creswell chapter 3 o Variables o Sampling terminologies and techniques o Data collection tools o Reliability and validity o Analyzing and interpretating 2 quantitative data Quantitative research designs Read Creswell chapters 7 o Experimental &8 o Quasi-experimental o Correlation o Surveys Introduction to Statistics Frequency distributions 3 Central tendency Measures of dispersion or variability Z-scores Distribution of sample means Presentation 2 Introduction to hypothesis testing 4 Introduction to the t-statistics T-test for two independent samples 5 T-test for two repeated samples Correlation 6 Introduction to regression Presentation 3 REVISION 7 EDTE 301: Quantitative Research Methods in Education Instructor: Dr. Paul K. Butakor Page 3