Research Methods PDF
Document Details
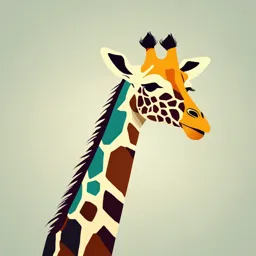
Uploaded by UnselfishForethought4915
University of Zambia
Dr Bornwell Sikateyo
Tags
Related
- Research Methodology in Dentistry. The Scientific Method (2023-2024) PDF
- Research Methods Notes PDF
- DENT 1007 Biostatistics Introduction PDF
- Descriptive Studies PDF - Biomedical Research & Biostatistics
- Summary Research Methods (PDF)
- MED 106 Research Methods and Medical Statistics: Introduction to Biomedical Research (PDF)
Summary
This document provides details of a research methods course at the University of Zambia, covering topics such as epidemiology, biostatistics, and research methodology. It outlines course objectives, content, and assessment criteria.
Full Transcript
RESEARCH METHODS DME 4115 DR BORNWELL SIKATEYO CBE COORDINATOR – UNZA, SoM RATIONALE In order for students to develop critical thinking in their education, they need to be exposed early to scientific approach to issues and to be provided with tools for evid...
RESEARCH METHODS DME 4115 DR BORNWELL SIKATEYO CBE COORDINATOR – UNZA, SoM RATIONALE In order for students to develop critical thinking in their education, they need to be exposed early to scientific approach to issues and to be provided with tools for evidence-based practice. Evidence-Based Practice (EBP) is necessary for improving the quality of health care as well as patient outcomes AIM The aim of the Research Methods course is to provide the student with knowledge of the principles of epidemiology, biostatistics, research methodology and evidence-based practice and to be able to disseminate research findings using various means and in various fora. Objectives At the end of the course, the student should be able to: 1. Describe and apply basic epidemiological techniques 2. Outline the process of research proposal development 3. Formulate a research proposal 4. Demonstrate skills in scientific writing 5. Recognize the importance of evidence-based practice in health outcomes COMPENTENCES 1. Describes and applies basic epidemiology techniques 2. Outlines the process of research proposal development 3. Formulates a community health research proposal development 4. Illustrates the process of research proposal development 5. Formulates a research proposal 6. Demonstrates skills in scientific writing 7. Recognize the importance of evidence-based practice in health outcomes 8. Demonstrate skills in literature search and referencing Course Contents Part I: Epidemiology Part II: Biostatistics Part III: Research Methodology PART I: Epidemiology Unit 1: Introduction to Epidemiology Unit 2: Implementation Science/Evidence-based Practice Unit 3: Screening for Disease Unit 4: Epidemiology of Communicable and Non-Communicable Diseases PART II: Biostatistics Unit 5: Principles of Statistics 5.1 Methods of data collection 5.2 Sampling techniques 5.3 Tests of significance 5.4 Presentation of data and use of statistical terms 5.5 Interpretation of data 5.6 Implement intervention measures PART III: RESEARCH METHODOLOGY Unit 6: Introduction to Research 6.1 Definition of concepts in research 6.2 Importance and significance of research PART III: RESEARCH METHODOLOGY Unit 7: Types of Research 7.1 Basic research 7.2 Applied/Translational research 7.3 Research approaches 7.3.1 Qualitative 7.3.2 Quantitative 7.4 Research Ethics PART III: RESEARCH METHODOLOGY Unit 8 Research Proposal Development 8.1 Problem Identification and Analysis 8.2 Statement of the problem 8.3 Rationale/Justification 8.4 Specific and general objectives 8.5 Hypothesis Testing 8.6 Literature review PART III: RESEARCH METHODOLOGY Unit 8.7 Research methods 8.7.1 Study design 8.7.2 Variables 8.7.3 Study site and population 8.7.4 Sample size 8.7.5 Sampling techniques 8.7.6 Data collection techniques PART III: RESEARCH METHODOLOGY Unit 8.7 Research methods 8.7.7 Strategies for data analysis 8.7.8 Research project management 8.7.9 Work plan 8.7.10 Budget 8.7.11 References 8.7.12 Ethical considerations PART III: RESEARCH METHODOLOGY Unit 9 Scientific Research Writing 9.1 Research proposal writing 9.2 Research report/manuscript writing 9.3 Research finding dissemination ASSESSMENT i) Continuous Assessment - 40% Written test - 20% Assignments - 15% Quizzes - 5% (ii) Final Examination - 60% Written Examination - 50% Viva Voce -10% Total 100% End Thank you very much for your attention UNIVERSITY OF ZAMBIA SCHOOL OF MEDICINE DEPARTMENT OF MEDICAL EDUCATION INTRODUCTION TO BIOSTATISTICS @2023 ISAAC FWEMBA, PhD BSc(ZAM), MPH(UK), PhD(GH) LECTURER SCHOOL OF MEDICINE UNIVERSITY OF ZAMBIA CENTRAL LIMIT THEOREM, SAMPLING,ESTIMATION FOR MEAN & PROPORTION OBJECTIVES The concept of the sampling distribution To compute probabilities related to the sample mean and the sample proportion The importance of the Central Limit Theorem To construct and interpret confidence interval estimates for the population mean and the population proportion 8/21/2023 1 2 SAMPLING DISTRIBUTIONS A sampling distribution is a distribution of all of the possible values of a sample statistic for a given sample size selected from a population. For example, suppose you sample 50 students from MED4 class regarding their mean GPA. If you obtained many different samples of size 50, you will compute a different mean for each sample. We are interested in the distribution of all potential mean GPAs we might calculate for any sample of 50 students. 8/21/2023 1 3 2 PROVING THAT 𝑆 IS UNBIAS 2 ESTIMATOR OF 𝜎 𝑛 2 𝑖=1 𝑋𝑖 − 𝑋 𝐸(𝑆 2 ) =𝐸 = 𝜎2 𝑛−1 SOME IMPORTANT PROVES 𝐸( 𝑋𝑖 ) = 𝐸(𝑋𝑖 ) 𝐸(𝑋𝑖 ) = 𝜇 𝑉𝑎𝑟(𝑋𝑖 ) = 𝜎 2 𝐸(𝑐𝑋) = 𝑐𝐸(𝑋) 8/21/2023 1 4 2 PROVING THAT 𝑆 IS UNBIAS 2 ESTIMATOR OF 𝜎 𝑛 2 𝑖=1 𝑋𝑖 − 𝑋 𝐸(𝑆 2 ) =𝐸 = 𝜎2 𝑛−1 𝑛 𝑛 𝑛 = 𝐸( 𝑥𝑖2 − 𝑛𝑥 2 = 𝐸( 𝑥𝑖 − 𝑥 2 ) = 𝐸( 𝑥𝑖2 − 2𝑥𝑖 𝑥 + 𝑥 2 𝑛 𝑖=1 𝑖=1 𝑖=1 𝑛 = 𝐸( 𝑥𝑖2 ) − 𝐸(𝑛𝑥 2 𝑛 𝑛 𝑖=1 𝑛 = 𝐸( 𝑥𝑖2 − 2𝑥𝑖 𝑥 + 𝑥2 = 𝐸(𝑥𝑖2 )) − 𝑛𝐸(𝑥 2 𝑖=1 𝑖=1 𝑖=1 𝑖=1 𝑛 𝑛 𝑛 𝜎2 = 𝜎 + 𝜇 ) − 𝑛( + 𝜇2 2 2 = 𝐸( 𝑥𝑖2 − 2𝑥 𝑥𝑖 + 𝑛𝑥 2 𝑛 𝑖=1 𝑖=1 𝑖=1 𝑛 𝑛 𝜎2 = 𝑛𝜎 + 𝑛𝜇 − 𝑛( ) + 𝑛(𝜇2 2 2 = 𝐸( 𝑥𝑖2 − 2𝑥 𝑥𝑖 + 𝑛𝑥 2 𝑛 𝑖=1 𝑖=1 𝑛 = 𝐸( 𝑥𝑖2 − 2𝑥. 𝑛𝑥 + 𝑛𝑥 2 = 𝑛𝜎 2 − 𝜎 2 = (𝑛 − 1)𝜎 2 𝑛 2 𝑖=1 𝑖=1 𝑥𝑖 − 𝑥 𝑛 − 1)𝜎 2 = 𝐸( )= ≈ 𝜎2 𝑛−1 𝑛−1 8/21/2023 1 5 DEVELOPING A SAMPLING DISTRIBUTION Assume there is a population … Population size N=4 Random variable, X, is age of individuals Values of X: 18, 20,22, 24 (years) 8/21/2023 1 6 DEVELOPING A SAMPLING DISTRIBUTION 8/21/2023 1 7 DEVELOPING A SAMPLING DISTRIBUTION 8/21/2023 1 8 DEVELOPING A SAMPLING DISTRIBUTION 8/21/2023 1 9 DEVELOPING A SAMPLING DISTRIBUTION 8/21/2023 1 10 COMPARING THE POPULATION DISTRIBUTION TO THE SAMPLE MEANS DISTRIBUTION 8/21/2023 1 11 SAMPLE MEAN SAMPLING DISTRIBUTION: STANDARD ERROR OF THE MEAN Different samples of the same size from the same population will yield different sample means A measure of the variability in the mean from sample to sample is given by the Standard Error of the Mean: (This assumes that sampling is with replacement or sampling is without replacement from an infinite population) σ σX n Note that the standard error of the mean decreases as the sample size increases 8/21/2023 1 12 SAMPLE MEAN SAMPLING DISTRIBUTION: IF THE POPULATION IS NORMAL If a population is normal with mean μ and _ standard deviation σ, the sampling distribution X of is also normally distributed with σ μX μ and σX n 8/21/2023 1 13 Z-VALUE FOR SAMPLING DISTRIBUTION OF THE MEAN _ Z-value for the sampling distribution of X : ( X μX ) ( X μ) Z σX σ n _ where: X = sample mean = population mean = population standard deviation n = sample size 8/21/2023 1 14 SAMPLING DISTRIBUTION PROPERTIES 8/21/2023 1 15 SAMPLING DISTRIBUTION PROPERTIES 8/21/2023 1 16 DETERMINING AN INTERVAL INCLUDING A FIXED PROPORTION OF THE SAMPLE MEANS Find a symmetrically distributed interval around µ that will include 95% of the sample means when µ = 368, σ = 15, and n = 25. Since the interval contains 95% of the sample means 5% of the sample means will be outside the interval Since the interval is symmetric 2.5% will be above the upper limit and 2.5% will be below the lower limit. From the standardized normal table, the Z score with 2.5% (0.0250) below it is -1.96 and the Z score with 2.5% (0.0250) above it is 1.96. 8/21/2023 1 17 DETERMINING AN INTERVAL INCLUDING A FIXED PROPORTION OF THE SAMPLE MEANS 8/21/2023 1 18 DETERMINING AN INTERVAL INCLUDING A FIXED PROPORTION OF THE SAMPLE MEANS Calculating the upper limit of the interval σ 15 XU μZ 368 (1.96) 373.88 n 25 Calculating the lower limit of the interval 95% of all sample means of sample size 25 are between 362.12 and 373.88 8/21/2023 1 19 SAMPLE MEAN SAMPLING DISTRIBUTION: IF THE POPULATION IS NOT NORMAL 8/21/2023 1 20 CENTRAL LIMIT THEOREM 8/21/2023 1 21 SAMPLE MEAN SAMPLING DISTRIBUTION: IF THE POPULATION IS NOT NORMAL 8/21/2023 1 22 HOW LARGE IS LARGE ENOUGH? For most distributions, n > 30 will give a sampling distribution that is nearly normal For fairly symmetric distributions, n > 15 For a normal population distribution, the sampling distribution of the mean is always normally distributed 8/21/2023 1 23 EXAMPLE Suppose a population has mean μ = 8 and standard deviation σ = 3. Suppose a random sample of size n = 36 is selected. What is the probability that the sample mean is between 7.8 and 8.2? 8/21/2023 1 24 EXAMPLE 8/21/2023 1 25 EXAMPLE 8/21/2023 1 26 Table positive values 8/21/2023 1 27 Negative values 8/21/2023 1 28 POPULATION PROPORTIONS π = the proportion of the population having some characteristic Sample proportion (p) provides an estimate of π: X number of items in the sample having the characteristic of interest p n sample size 0≤ p≤1 p is approximately distributed as a normal distribution when n is large (assuming sampling with replacement from a finite population or without replacement from an infinite population) 8/21/2023 1 29 Sampling Distribution of p 8/21/2023 1 30 Z-VALUE FOR PROPORTIONS Standardize p to a Z value with the formula: p p Z σp (1 ) n 8/21/2023 1 31 Example If the true proportion of voters who support Proposition A is π = 0.4, what is the probability that a sample of size 200 yields a sample proportion between 0.40 and 0.45? i.e.: if π = 0.4 and n = 200, what is P(0.40 ≤ p ≤ 0.45) ? 8/21/2023 1 32 Example if π = 0.4 and n = 200, what is P(0.40 ≤ p ≤ 0.45) ? (1 ) 0.4(1 0.4) Find p : σp 0.03464 n 200 Convert to 0.40 0.40 0.45 0.40 P(0.40 p 0.45) P Z standardized 0.03464 0.03464 normal: P(0 Z 1.44) 8/21/2023 1 33 Example if π = 0.4 and n = 200, what is P(0.40 ≤ p ≤ 0.45) ? Utilize the cumulative normal table: P(0 ≤ Z ≤ 1.44) = 0.9251 – 0.5000 = 0.4251 Standardized Sampling Distribution Normal Distribution 0.4251 Standardize 0.40 0.45 0 1.44 p Z 8/21/2023 1 34 8/21/2023 1 35 CHAPTER SUMMARY In this chapter we discussed: The concept of a sampling distribution Computing probabilities related to the sample mean and the sample proportion The importance of the Central Limit Theorem 8/21/2023 1 36 ESTIMATION 8/21/2023 1 37 POINT AND INTERVAL ESTIMATES A point estimate is a single number, a confidence interval provides additional information about the variability of the estimate Lower Upper Confidence Confidence Limit Limit Width of confidence interval 8/21/2023 1 38 POINT ESTIMATES 8/21/2023 1 39 CONFIDENCE INTERVALS How much uncertainty is associated with a point estimate of a population parameter? An interval estimate provides more information about a population characteristic than does a point estimate Such interval estimates are called confidence intervals 8/21/2023 1 40 CONFIDENCE INTERVAL ESTIMATE An interval gives a range of values: – Takes into consideration variation in sample statistics from sample to sample – Based on observations from 1 sample – Gives information about closeness to unknown population parameters – Stated in terms of level of confidence e.g. 95% confident, 99% confident Can never be 100% confident 8/21/2023 1 41 CONFIDENCE INTERVAL EXAMPLE Cereal fill example Population has µ = 368 and σ = 15. If you take a sample of size n = 25 you know – 368 ± 1.96 * 15/5 = (362.12, 373.88). 95% of the intervals formed in this manner will contain µ. – When you don’t know µ, you use 𝑥 to estimate µ If 𝑥 = 362.3 the interval is 362.3 ± 1.96 * 15/5 = (356.42, 368.18) Since 356.42 ≤ µ ≤ 368.18 the interval based on this sample makes a correct statement about µ. But what about the intervals from other possible samples of size 25? 8/21/2023 1 42 CONFIDENCE INTERVAL EXAMPLE Lower Upper Contain Sample # 𝑥 Limit Limit µ=368? 1 362.30 356.42 368.18 Yes 2 369.50 363.62 375.38 Yes 3 360.00 354.12 365.88 No 4 362.12 356.24 368.00 Yes 5 373.88 368.00 379.76 Yes 8/21/2023 1 43 CONFIDENCE INTERVAL EXAMPLE In practice you only take one sample of size n In practice you do not know µ so you do not know if the interval actually contains µ However, you do know that 95% of the intervals formed in this manner will contain µ. Thus, based on the one sample, you actually selected you can be 95% confident your interval will contain µ (this is a 95% confidence interval) Note: 95% confidence is based on the fact that we used Z = 1.96. 8/21/2023 1 44 ESTIMATION PROCESS 8/21/2023 1 45 ESTIMATION PROCESs 8/21/2023 1 46 CONFIDENCE LEVEL, (1-) Suppose confidence level = 95% Also written (1 - ) = 0.95, (so = 0.05) A relative frequency interpretation: – 95% of all the confidence intervals that can be constructed will contain the unknown true parameter A specific interval either will contain or will not contain the true parameter – No probability involved in a specific interval 8/21/2023 1 47 CONFIDENCE INTERVALS Confidence Intervals Population Population Mean Proportion σ Known σ Unknown 8/21/2023 1 48 CONFIDENCE INTERVALS Assumptions – Population standard deviation σ is known – Population is normally distributed – If population is not normal, use large sample (n > 30) Confidence interval estimate: 8/21/2023 1 49 FINDING THE CRITICAL VALUE, ZΑ/2 8/21/2023 1 50 COMMON LEVELS OF CONFIDENCE Commonly used confidence levels are 90%, 95%, and 99% 8/21/2023 1 51 INTERVALS AND LEVEL OF CONFIDENCE 8/21/2023 1 52 EXAMPLE A sample of 11 babies from a large normal population has a mean weight of 2.20 kgs. We know from past testing that the population standard deviation is 0.35 kgs. Determine a 95% confidence interval for the true mean weight of the population. 8/21/2023 1 53 EXAMPLE A sample of 11 babies from a large normal population has a mean weight of 2.20 kgs. We know from past testing that the population standard deviation is 0.35kgs. Solution: X Z α/2 σ n 2.20 1.96 (0.35/ 11) 2.20 0.2068 1.9932 μ 2.4068 8/21/2023 1 54 INTERPRETATION We are 95% confident that the true mean weight is between 1.9932 and 2.4068kgs Although the true mean may or may not be in this interval, 95% of intervals formed in this manner will contain the true mean 8/21/2023 1 55 CONFIDENCE INTERVALS 8/21/2023 1 56 DO YOU EVER TRULY KNOW µ? Probably not! In virtually all real world health situations, σ is not known. If there is a situation where σ is known then µ is also known (since to calculate σ you need to know µ.) If you truly know µ there would be no need to gather a sample to estimate it. 8/21/2023 1 57 Confidence Interval for μ (σ Unknown)? If the population standard deviation σ is unknown, we can substitute the sample standard deviation, S This introduces extra uncertainty, since S is variable from sample to sample So we use the t distribution instead of the normal distribution 8/21/2023 1 58 Confidence Interval for μ (σ Unknown) Assumptions – Population standard deviation is unknown – Population is normally distributed – If population is not normal, use large sample (n > 30) Use Student’s t Distribution S X tα / 2 Confidence Interval Estimate: n (where tα/2 is the critical value of the t distribution with n -1 degrees of freedom and an area of α/2 in each tail) 8/21/2023 1 59 STUDENT’S T DISTRIBUTION The t is a family of distributions The tα/2 value depends on degrees of freedom (d.f.) – Number of observations that are free to vary after sample mean has been calculated d.f. = n - 1 8/21/2023 1 60 DEGREES OF FREEDOM (DF) Idea: Number of observations that are free to vary after sample mean has been calculated Example: Suppose the mean of 3 numbers is 8.0 Here, n = 3, so degrees of freedom = n – 1 = 3 – 1 = 2 (2 values can be any numbers, but the third is not free to vary for a given mean) 8/21/2023 1 61 STUDENT’S T DISTRIBUTION 8/21/2023 1 62 STUDENT’S T TABLE Upper Tail Area Let: n = 3 df = n - 1 = 2 df.10.05.025 = 0.10 /2 = 0.05 1 3.078 6.314 12.706 2 1.886 2.920 4.303 3 1.638 2.353 3.182 /2 = 0.05 The body of the table contains t values, not 0 2.920 t probabilities 8/21/2023 1 63 Selected t distribution values 8/21/2023 1 64 EXAMPLE OF T DISTRIBUTION CONFIDENCE INTERVAL 8/21/2023 1 65 READING Student’s t Table 8/21/2023 1 66 Example of t distribution confidence interval Interpreting this interval requires the assumption that the population you are sampling from is approximately a normal distribution (especially since n is only 25). This condition can be checked by creating a: – Normal probability plot or – Boxplot 8/21/2023 1 67 Confidence Intervals 8/21/2023 1 68 CONFIDENCE INTERVALS FOR THE POPULATION PROPORTION, Π Recall that the distribution of the sample proportion is approximately normal if the sample size is large, with standard deviation (1 ) σp n We will estimate this with sample data: p(1 p) n 8/21/2023 1 69 CONFIDENCE INTERVAL ENDPOINTs Upper and lower confidence limits for the population proportion are calculated with the formula p(1 p) p Z α/2 n where – Zα/2 is the standard normal value for the level of confidence desired – p is the sample proportion – n is the sample size Note: must have np > 5 and n(1-p) > 5 8/21/2023 1 70 EXAMPLE A random sample of 100 people shows that 25 are left-handed. Form a 95% confidence interval for the true proportion of left-handers 8/21/2023 1 71 Example A random sample of 100 people shows that 25 are left-handed. Form a 95% confidence interval for the true proportion of left-handers. p Z /2 p(1 p)/n 25/100 1.96 0.25(0.75)/100 0.25 1.96(0.0433) 0.1651 0.3349 8/21/2023 1 72 Example We are 95% confident that the true percentage of left-handers in the population is between 16.51% and 33.49%. Although the interval from 0.1651 to 0.3349 may or may not contain the true proportion, 95% of intervals formed from samples of size 100 in this manner will contain the true proportion. 8/21/2023 1 73 REVISION 8/21/2023 1 74 Applications of the Central Limit Theorem Consider the distribution of serum cholesterol levels for all 20- to 74-year-old males living in the Zambia. The mean of this population is 𝜇 = 211 mg , and the standard deviation is a= 46 mg. Question 1.0: If we select repeated samples of size from the population, what proportion of the samples will have a mean value of 230 mg or above? 8/21/2023 1 75 Applications of the Central Limit Theorem Assuming that a sample of size 25 is large enough, the central limit theorem states that the distribution of means of samples of size 25 is approximately normal with mean 𝜇 = 211 mg and standard error / n = 9.2 mg. This sampling distribution and the underlying population distribution are shown in the figure on the next slides. Example: If𝑥 = 230, then Z score can be computed using Z X- n 8/21/2023 1 76 Applications of the Central Limit Theorem 230− 211 𝑧= 9.2 = 2.07. Consulting the z score table, we find that the area to the right of z = 2.07 is 0.019. Only about 1.9% of the samples will have a mean greater than 230 mg. Interpretation: Equivalently, if we select a single sample of size 25 from the population of 20 to 74-year-old males, the probability that the mean serum cholesterol level for this sample 8/21/2023 1 is 230 mg or higher is 0.019.77 Applications of the Central Limit Theorem Question 2: What mean value of serum cholesterol level cuts off the lower 10% of the sampling distribution of means? Locating 0.100 in the body of Table A., we see that it corresponds to the value z = -1.28. Solving for 𝑋, 𝑋−211 𝑧= 9.2 z = -1.28 𝑋 = 211 + ( -1.28)(9.2)= 199.2. Therefore, approximately 10% of the samples of size 25 have means that are less than or equal to 199.2 mg. 8/21/2023 1 78 Applications of the Central Limit Theorem Let us now calculate the upper and lower limits that enclose 95 % of the means of samples of size 25 drawn from the population. Since 2.5 % of the area under the standard normal curve lies above z = 1.96 and another 2.5 % lies below z = -1.96, Z = 1.96 P( −1.96 ≤ Z ≤ 1.96) = 0.95. 8/21/2023 1 79 Applications of the Central Limit Theorem Thus, we are interested in outcomes of Z for which −1.96 ≤ Z ≤ 1.96 𝑋− 211 −1.96 ≤ ≤ 1.96 9.2 211−1.96(9.2) ≤ 𝑋 ≤ 211+1.96(9.2). 8/21/2023 1 80 Applications of the Central Limit Theorem This tells us that approximately 95 % of the means of samples of size 25 lie between 193.0 mg and 229.0 mg. Implication: However, if we select a random sample size 25 that is reported to be from the population of serum cholesterol levels for all 20 to 74year old males, and the sample has a mean that is either greater than 229.0 or less than 193.0 mg, we should be suspicious of this claim. 8/21/2023 1 81 Applications of the Central Limit Theorem Either the random sample was actually drawn from a different population or a rare event has taken place. For the purposes of this discussion, a "rare event" is defined as an outcome that occurs less than 5% of the time. Question 3: Suppose we had selected samples of size 10 from the population rather than samples of size 46 𝑙𝑂 standard error of 𝑋 would be 25. In this case, the 8/21/2023 = 14.5mg , 1 82 Applications of the Central Limit Theorem 𝑋− 211 −1.96 ≤ ≤ 1.96 14.5 The upper and lower limits that enclose 95 % of the means would be 182.5 ≤ 𝑋 ≤ 239.5. Interpretation: Note that this interval is wider than the calculated for samples of size 25. We expect the amount of sampling variation to increase as the size decreases. 8/21/2023 83 Applications of the Central Limit Theorem Drawing samples of size 50 would result in upper and lower limits 198.2 ≤ 𝑋 ≤ 223.8 Not surprisingly, this interval is narrower than the one constructed for samples of size 25. Samples of size 100 produce the limits shown below 8/21/2023 84 Applications of the Central Limit Theorem Implication: We note that ;as the size of the samples increases, the amount of variability among the sample means-quantified by the standard error a decreases; 𝑛 consequently, the limits encompassing 95% of these means means move closer together. The length of an interval is simply the upper limit minus the lower limit. Note that all the intervals we have constructed have been symmetric about the population mean 211 mg. 8/21/2023 85 Applications of the Central Limit Theorem Question4.0: Suppose that we again wish to construct an interval that contains 95% of the means of samples of size 25. Since 1% of the area under the standard normal curve lies above z = 2.32 and 4% lies below z = -1.75, P(−1.75 ≤ 𝑋 ≤ 2.32)=0.95 8/21/2023 86 Applications of the Central Limit Theorem Similarly, substituting 𝑋 − 211 for Z, we find the 9.2 interval to be 194.9 X 232.3 Interpretation: Therefore, we are able to say that approximately 95% of the means of samples of size 25 lie between 194.9 mg and 232.3 mg. 8/21/2023 87 Applications of the Central Limit Theorem An exception to this rule is the one-sided interval; we return to this special case below.) We now move on to a slightly more complicated question : Question5.0: How large would the samples need to be for 95 % of their means to lie within :±:5 mg of the population mean 𝜇.To answer this, it is not necessary to know the value of the parameter𝜇 · 8/21/2023 88 Applications of the Central Limit Theorem We simply find the sample size n for which P(𝜇− 5 ≤ 𝑋 ≤ 𝜇+ 5) = 0.95, or P(- 5 X - 5) = 0.95 To begin, we divide all three terms of the inequality by 𝜎 the standard error 𝑛 = 46𝑛; this results in Since Z is equal to 8/21/2023 89 Applications of the Central Limit Theorem Recall that 95% of the area under the standard normal curve lies between z = −1.96 and z = 1.96 Therefore, to find the sample size n, we could use the upper bound of the interval and solve the equation z = 1.96 5 or 46 𝑛 equivalently, we could use the lower bound and solve −5 z = -1.96 using simple algebra 46 𝑛 can be rearranged to 5 𝑛 1.96 = 46 8/21/2023 90 Applications of the Central Limit Theorem 1.96(46) 𝑛= 5 When we deal with sample sizes, it is conventional to round up. Therefore, samples of size 326 would be require for 95% of the sample means to lie within±5 mg of the population mean 𝜇. Interpretation: Another way to state this is that if we a sample of size 326 from the population and calculate its mean, the probability that the sample mean is±5 mg of the true population mean 𝜇 is 0.95. 8/21/2023 91 Applications of the Central Limit Theorem Up to this point, we have focused on two-sided intervals: we have found the upper and lower limits that enclose a specified proportion of the sample means. In some situations, however, we are interested in a one-sided interval instead. Question 6.0: We might wish to find the upper bound for 95 % of the mean serum cholesterol levels of samples of size 25. Since 95 % of the area under the standard normal curve lies below z = 1.645 8/21/2023 92 Applications of the Central Limit Theorem consequently, we are interested in P(Z ≤ 1.645) = 0.95. outcomes of Z for which 𝑍 ≤ 1.645 𝑥 − 211 Substituting 𝑥 − 211 for Z produces 9.2 ≤ 1.645 9.2 Approximately 95 % of the means of samples of size 25 lie below 226.1 mg. If we want to construct a lower bound for 95 % of the mean serum cholesterol levels, we focus on values of Z that lie above -1.645 ; we𝑥solve − 211 9.2 ≥ −1.645 8/21/2023 93 TABLE APPLICATION 8/21/2023 94 INTRODUCTION TO EPIDEMIOLOGY S H Nzala SOM, UNZA OBJECTIVES 1) Describe the origin of epidemiology 2) Define the term epidemiology 3) Outline the scope, uses and objectives of epidemiology 4) Give the broad classification of epidemiology Introduction to Epidemiology Health A state of complete physical, mental, and social well-being and not merely the absence of disease or infirmity - World Health Organization, 1948 Public Health Is an effort organized by society to protect, promote, and restore the health of the population Disciplines of Medicine Basic sciences: anatomy, physiology, pathology etc. Clinical sciences: paediatrics, obstetrics etc - concerned with care for individual patient Public health: community replaces the individual Background of Epidemiology Epidemiology draws upon the medical, biological, and behavioral sciences (anthropology, psychology, sociology, and education), as well as statistics, demographics health and medical care services and computer sciences. We can study health and disease by -Observing effects on individuals Laboratory investigation of experimental animals Measuring the distribution of health problems in the population Background We can study health and disease by Observing effects on individuals Laboratory investigation of experimental animals Measuring the distribution of health problems in the population Origin “Epidemiology” from Greek : Epi = upon; Demos = people ‘epidemic’ = “upon the people” , Logos = study Epidemiologists first concern was to investigate, control and prevent epidemics Two basic assumptions about disease: Disease does not occur at random Disease has causal and preventive factors Definition Study of the distribution and determinants of health- related states and events in specified populations and the application of this study to the control of health problems. Analytical tool for assessing the effectiveness of medical intervention and health care delivery Basic science of public health that focuses on the population at large. Epidemiology Definition (cont’d) Epidemiology Disease deviation from physical, mental or emotional health expands to include conditions such as injuries, birth defects, health outcome etc. Population group of people often geographically defined Epidemiology Definition (cont’d) Epidemiology Distribution characterizing the distribution of health status in terms of age, gender, race etc. Determinants any factor that brings about a change in a health condition or other defined outcomes. Meaning of the definition Study - includes: surveillance, observation, hypothesis testing, analytic research and experiments. Distribution - refers to analysis of: times, persons, places and classes of people affected. Determinants - include factors that influence health: biological, chemical, physical, social, cultural, economic, genetic and behavioural. Meaning of the definition Health-related states and events - refer to: diseases, causes of death, behaviours such as use of tobacco, positive health states, reactions to preventive regimes and provision and use of health services. Specified populations - include those with identifiable characteristics, such as occupational groups Application to prevention and control - the aims of public health—to promote, protect, and restore health. Determinants of disease Determinants of disease occurrence includes both causes and factors that influence the risk of disease Disease is as a result of the epidemiologic triad (Agent, Host and Environment) Infection occurs only when the AGENT is encountered by a susceptible Host in an ENVIRONMENT that is favourable Two basic assumptions about disease: Disease does not occur at random Disease has causal and preventive factors It results from an interaction of the host (e.g. a person), the agent (e.g. a bacterium) and the environment (e.g. contaminated water supply) Epidemiologic Triad Disease is the result of forces within a dynamic system consisting of: agent of infection host environment Factors Influencing Disease Transmission Agent Environment Weather Infectivity Housing Pathogenicity Geography Virulence Occupational Immunogenicity setting Antigenic stability Age Air quality Survival Sex Food Host Genotype Behaviour Nutritional (www) status Distribution of disease Frequencies of values or categories of measurement with respect to time, place and persons Frequency Involves measuring disease distribution Requires information - count (quantification) - size of population - time period Requires mathematical calculation - ratio - proportion - rate Specialty disciplines: Pharmacoepidemiology, Clinical Epi, Psycho-Social Epi, Nutritional Epi, Molecular Epi, Genetic Epi, Cancer Epi, Environmental Epi, Occupational Epi, etc., Scope Shift from infectious diseases initially to chronic diseases of later life and now applied to other areas e.g. -injuries - adverse drug reactions -mental illness -family planning -health services research etc. Scope Shift also to evaluation of certain exposures (chemicals, ionising radiation) Evaluation of effects of new approaches to prevention Evaluation of treatment effectiveness etc Organization of health care Epidemiology not only concerned with epidemics but also with inter-epidemic periods and with sporadic and endemic occurrences of diseases Classification Two broad categories: a) Descriptive epidemiology: the study of the frequency (amount) and distribution of health related state within a population by person, place and time b) Analytic epidemiology: more focused study of health related problems or reasons for relatively high or low frequency in specific groups. Epidemiological questions To describe the occurrence of disease fully, some broad questions must be asked: a) What health events are occurring? b) Who is affected? c) When do the cases occur? d) Where do the cases occur? Other questions Why is it occurring How can it be influenced Uses of Epidemiology: Historical study - is community health getting better or worse? We can only decide by comparing experiences over time. Community diagnosis Working of health services - availability, accessibility, utilization, effectiveness, efficacy, efficiency Uses of Epidemiology: Individual risks and chances of getting disease Completing the clinical picture - constructing a model Identification of syndromes “lumping and splitting” Search for causes Evaluation of presenting s/s of disease - by analysing data in hospital charts Clinical decision making - involves use of decision trees Specific objectives of Epidemiology: to identify aetiology of disease and risk factors to determine extent of disease found in the community (disease burden) to study the natural history and prognosis of disease to evaluate new preventive and therapeutic measures and new modes of health care delivery to provide foundation for developing public policy and regulatory decisions relating to environmental problems. Epidemiology: a scientific tool To describe To understand To propose and test hypotheses To validate or challenge public policy DESCRIPTIVE AND ANALYTIC EPIDEMIOLOGY OBJECTIVES To describe the two broad categories of epidemiology To lay the foundation for understanding study designs Kinds of Epidemiology Study of the occurrence Descriptive and distribution of disease Further studies to determine the Analytic validity of a hypothesis concerning the occurrence of disease. Experimental Deliberate manipulation of the cause is predictably followed by an alteration in the effect not due to chance Descriptive vs. Analytic Epidemiology Descriptive Analytic Used when little is Used when insight known about the about various aspects disease of disease is available Rely on preexisting Rely on development data of new data Who, where, when Why Illustrates Evaluates the causality potential associations of associations Both are important! DESCRIPTIVE EPIDEMIOLOGY PERSON, PLACE AND TIME Key questions Why now? time Why here? place Why in this group? person Descriptive Epidemiology Study of the occurrence and distribution of disease in terms of: Time Place Person What are the three categories of descriptive epidemiologic clues? □ Person: Who is getting sick? □ Place: Where is the sickness occurring? □ Time: When is the sickness occurring? PPT = person, place, time Time Secular Periodic Seasonal Epidemic DESCRIPTIVE EPIDEMIOLOGY In order to describe the occurrence of disease fully, it is necessary to specify Person, Place and Time Person, Place and Time Examining the distribution of disease in a population in terms of descriptive characteristics in order to: 1) Identify subgroups at highest risk 2) Find clues about possible causes (hypothesis generating studies) DESCRIPTIVE EPIDEMIOLOGY Descriptive epidemiology identifies nonrandom variations in the distribution of disease to enable an investigator to generate testable hypotheses regarding aetiology. Population at Risk: What to ask for? Who gets the disease ? PERSON Where does the disease occur ? PLACE When does the disease occur ? TIME Descriptive data answer question: who, when, where about a disease or condition PERSON: Three characteristics are of vital importance: Age, Sex and Ethnic group or race. e.g. death rates fairly high in infancy, decreasing to reach lowest point between ages 5 - 14 and climbs gradually up to age 40. Thereafter - exponential. PERSON: Demographics (Age) Describe the pattern of infection distribution by the age groups in terms of population exposure and susceptibility? 700 600 500 400 300 200 100 0 >M Person – Race/ethnicity Race/ethnicity Major differences exist between culture, behavior, health events and related activities with subsequent impact on disease and mortality patterns It has to be considered in all analysis of studies and its effect controlled for PERSON Other variables would include: a) social class (education, area of residence, income, life style etc) b) Blood Type- e.g ‘A’ - increased risk of gastric cancer ‘O’ - increased risk of duodenal ulcer etc. PERSON: Other variables c) Environmental exposures chemicals (tobacco, asbestos), infectious diseases (specific immunity) d) Occupation, e) Marital status- death rates vary from lowest to highest in the order: married, single, widowed, and divorced PERSON-Family variables: a) Family size - associated with social class, b) Birth order - first borns at higher risk of asthma, schizophrenia, peptic ulcer and pyloric stenosis PERSON-Family variables: c) Maternal age - e.g congenital abnormalities d) Parental deprivation - e.g psychiatric and psychosomatic disorders, attempted suicide PLACE May be Geographical, urban-rural differences etc. TIME Occurrence usually expressed on a monthly or annual basis Major types of variation over time are: secular, cyclic, and short term fluctuations. TIME Secular trends: these are long term variations- years or decades. Cyclic trends : recurrent alterations in the frequency of disease. Cycles may be annual (seasonal) or have other periodicity. Descriptive Epidemiology Correlational studies Case reports Case series Cross sectional studies Analytic Epidemiology Determining the Etiology of Disease Types of Studies Cross-sectional – prevalence rates that may suggest association (good for developing theory, but no causal association) Retrospective (Case-control) – good for rare diseases and initial etiologic studies Prospective (cohort, longitudinal, follow-up) – yields incidence rates and estimates for risk. Better for causal association. Experimental (intervention studies) – strongest evidence for etiology Cross-Sectional Studies Single point in time (snapshot studies) Risk factors and disease measured at the same time Determines prevalence ratios Experimental Studies Uses an intervention in which the investigator manipulates a factor and measures the outcome Elements of a complete experiment Manipulation of data Use of a control group Ability to randomize subjects to treatment groups Classification of Epidemiology INTRODUCTION TO RESEARCH AND PROPOSAL DEVELOPMENT LECTURE 3 RESEARCH QUESTIONS, OBJECTIVES, HYPOTHESES AND VARIABLES Formulating research question OBJECTIVES Define research question Formulate research question Describe the characteristics of research questions Formulating a research question Before you begin writing a research proposal, take some time to map out your research strategy. A necessary first step is to formulate a research question. A Research Question is a statement that identifies the phenomenon to be studied. For example, “Does monetary policy affect the economy?” Formulating a research question A valid and clear research question is: well justified; original; feasible; focused; Identifying a research question flow - chart Criteria for developing a good research question Feasible Interesting Novel Ethical Relevant Cummings et al. 2001 7 Good research question? Feasible Ethical Participants Social or scientific Resources value Manageable Safe Data available? Relevant Advance scientific Interesting knowledge? Novel Influence clinical In relation to previous practice? findings Impact health policy? Confirm or refute? Guide future research? New setting, new population 8 A Research Question Must Identify 1. The variables under study 2. The population being studied 3. The testability of the question 9 Formulation of Research Questions Problems are questions about relationships among variables/factors Examples: -What causes attrition in the medical school? -Why are health professionals migrating to other countries? -What factors determine urbanization? -What causes political violence? -Why is immunization low during rain season? -Does racial school integration enhance educational attainment? Research Questions Strategies for Developing the Research Question (s): Know the Area or field of interest- helps to familiarize with the area on which your research focuses. Widen the Base of Your Experience- You should not be limited by the research in the specific field you are researching, but know researchers in other fields and from other disciplines. Contact and discussing with practitioners may give a different perspective on what the questions are. Consider Using Techniques for Enhancing Creativity- There is literature on creativity relevant to the process of generating research questions. Research Questions Avoid the Pitfalls of: - Posing research questions that can’t be answered. - Asking questions that have already been answered satisfactorily. - Developing a research question simply on the basis that they allow the use of a particular statistical package that is available. HYPOTHESES Objectives: Hypothesis Statement about the relationship between 2 or more variables Converts the research question into a statement that predicts an expected outcome A unit or subset of the research problem 63 Hypotheses A hypothesis is a tentative guess or intuitive hunch of a situation Tentative hypotheses can provide a useful bridge between the research question and the design of the enquiry. Examples of hypotheses are: - The degree of urbanization in a society varies directly with the division of labour. -The division of labour in a society varies directly with technological development Hypotheses Hypotheses are tentative answers to research problems. Hypotheses are predictions of relationships between one or more factors and the problem under study, which can be tested. They are expressed in the form of a relationship between independent (factor) and dependent (core problem) variables When a researcher suggests a hypothesis, he/she has no assurance that it will be verified. When a hypothesis is constructed, and if it is rejected, another one is put forward- If it is accepted, it is incorporated into the scientific body of knowledge. Hypotheses In interpreting the results, if there is statistically significant difference between the two means of variables, we then reject the null hypothesis (Ho)-i.e. it is a true hypothesis. If we conclude that the observed difference is not statistically significant, we then accept the null hypothesis. Hypotheses This is determined by application of a significant level statistics, such as Chi-Square test (x²) to estimate a p-value, which takes the value of 0.05, expressing the likelihood of finding a difference by chance when there is no real difference. For a statistically significant difference between the two means, the p-value is smaller than 0.05-0.000, but if no statistically differences observed, the p-value is larger or greater than 0.05, e.g. 0.06, 0.07,0.5, 0.1,0.3 and so on. Sources of Hypotheses and Research Questions: Hypotheses can derive from theories, directly from observations, intuitively or combination of these. Research questions and hypotheses have common characteristics: - Must be Clear -Value free- no introduction of researcher’s biases - They are specific (measurable). - Amenable to empirical testing Characteristics of hypotheses Declarative statement that identifies the predicted relationship between 2 or more variables Testability Based on sound scientific theory/rationale 20 Directional vs. Non-Directional Hypotheses Directional hypothesis Specifies the direction of the relationship between independent and dependent variables Non-directional hypothesis Shows the existence of a relationship between variables but no direction is specified 21 OBJECTIVES Describe what objectives are Identify types of objectives in research Formulate study objectives Research Objectives Objectives of research summarise what is to be achieved by the study. Objectives should be closely related to the statement of the problem. Objectives are required to answers questions pertaining to: Why do we want to carry out the research? What do we hope to achieve? Two Types of Research Objective are Formulated 1. General Objective: States what is expected to be achieved by the study in general terms. 2. Specific Objectives: are the breakdown of a general objective into smaller and logically connected parts. Specific Objectives should systematically address the various aspects of the problem as defined under “statement of the problem” and the “key factors” that are assumed to influence or cause the problem. Research Objectives Specific Objectives specify what you will do in your study, where and for what purpose. Examples: If the general objective is, “ To identify the reasons for low utilization of [c/(c+d)] or [a/(a+b)] < [c/(c+d)] H=Hypotheses: Persons with cancer have significantly lower level of serum vitamin C than healthy persons of the same age and sex. Cross-sectional Design factor present No Disease factor absent Study population factor present Disease factor absent time Study only exists at this point in time Cross-sectional Studies Often used to study conditions that are relatively frequent with long duration of expression (nonfatal, chronic conditions) It measures prevalence, not incidence of disease Example: community surveys Not suitable for studying rare or highly fatal diseases or a disease with short duration of expression Cross-sectional studies Disadvantages Weakest observational design, (it measures prevalence, not incidence of disease). Prevalent cases are survivors The temporal sequence of exposure and effect may be difficult or impossible to determine Usually don’t know when disease occurred Rare events a problem. Quickly emerging diseases a problem Epidemiologic Study Designs Case-Control Studies – an “observational” design comparing exposures in disease cases vs. healthy controls from same population – exposure data collected retrospectively – most feasible design where disease outcomes are rare Case-Control Studies Cases: Disease Controls: No disease factor present Cases factor absent (disease) Study population factor present Controls (no disease) factor absent present past time Study begins here Case-Control Study Strengths – Less expensive and time consuming – Efficient for studying rare diseases Limitations – Inappropriate when disease outcome for a specific exposure is not known at start of study – Exposure measurements taken after disease occurrence – Disease status can influence selection of subjects Epidemiologic Study Designs Cohort Studies – an “observational” design comparing individuals with a known risk factor or exposure with others without the risk factor or exposure – looking for a difference in the risk (incidence) of a disease over time – best observational design – data usually collected prospectively (some retrospective) disease Factor Study present no disease population free of disease Factor disease absent no disease present future time Study begins here Timeframe of Studies Prospective Study - looks forward, looks to the future, examines future events, follows a condition, concern or disease into the future time Study begins here Prospective Cohort study Exposed Outcome Measure exposure and confounder variables Baseline Non-exposed Outcome time Study begins here Timeframe of Studies Retrospective Study - “to look back”, looks back in time to study events that have already occurred time Study begins here Retrospective Cohort study Exposed Outcome Measure exposure and confounder variables Baseline Non-exposed Outcome time Study begins here Cohort Study Strengths – Exposure status determined before disease detection – Subjects selected before disease detection – Can study several outcomes for each exposure Limitations – Expensive and time-consuming – Inefficient for rare diseases or diseases with long latency – Loss to follow-up Experimental Studies investigator can “control” the exposure akin to laboratory experiments except living populations are the subjects generally involves random assignment to groups clinical trials are the most well known experimental design the ultimate step in testing causal hypotheses Experimental Studies In an experiment, we are interested in the consequences of some treatment on some outcome. The subjects in the study who actually receive the treatment of interest are called the treatment group. The subjects in the study who receive no treatment or a different treatment are called the comparison group. Epidemiologic Study Designs Randomized Controlled Trials (RCTs) – a design with subjects randomly assigned to “treatment” and “comparison” groups – provides most convincing evidence of relationship between exposure and effect – not possible to use RCTs to test effects of exposures that are expected to be harmful, for ethical reasons RANDOMIZATION outcome Intervention no outcome Study population outcome Control no outcome baseline future time Study begins here (baseline point) Epidemiologic Study Designs Randomized Controlled Trials (RCTs) – the “gold standard” of research designs – provides most convincing evidence of relationship between exposure and effect trials of hormone replacement therapy in menopausal women found no protection for heart disease, contradicting findings of prior observational studies Randomized Controlled Trials Disadvantages – Very expensive – Not appropriate to answer certain types of questions it may be unethical, for example, to assign persons to certain treatment or comparison groups