WhatsApp Image 2025-03-23 at 18.03.20 (2).jpeg
Document Details
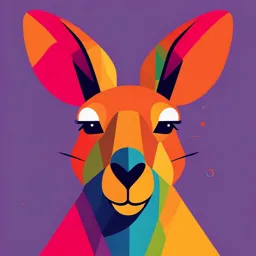
Uploaded by RespectableIridium9066
Full Transcript
## Quantitative Methods ### Correlation and Regression **Correlation**: Correlation is a statistical measure that expresses the extent to which two variables are linearly related, meaning they change together at a constant rate. * The correlation coefficient, denoted by $r$, measures the streng...
## Quantitative Methods ### Correlation and Regression **Correlation**: Correlation is a statistical measure that expresses the extent to which two variables are linearly related, meaning they change together at a constant rate. * The correlation coefficient, denoted by $r$, measures the strength and direction of a linear relationship between two variables. * It takes on values between -1 and +1, inclusive. * $r = +1$: perfect positive correlation * $r = -1$: perfect negative correlation * $r = 0$: no correlation **Regression**: Regression analysis is a statistical process for estimating the relationships among variables. ### Simple Linear Regression Model $y_i = \beta_0 + \beta_1 x_i + \varepsilon_i$ Where: * $y_i$ is the dependent variable. * $x_i$ is the independent variable. * $\beta_0$ is the intercept. * $\beta_1$ is the slope. * $\varepsilon_i$ is the error term. ### Ordinary Least Squares (OLS) Estimation The OLS method is used to estimate the parameters $\beta_0$ and $\beta_1$ by minimizing the sum of the squares of the differences between the observed and predicted values. $\mathop{min} \sum_{i=1}^{n} \varepsilon_i^2 = \mathop{min} \sum_{i=1}^{n} (y_i - \hat{y}_i)^2$ Where: * $\hat{y}_i = \hat{\beta}_0 + \hat{\beta}_1 x_i$ is the predicted value. * $\hat{\beta}_0$ and $\hat{\beta}_1$ are the OLS estimators. ### Key Formulas * Estimated slope: $\hat{\beta}_1 = \frac{\sum_{i=1}^{n} (x_i - \bar{x})(y_i - \bar{y})}{\sum_{i=1}^{n} (x_i - \bar{x})^2}$ * Estimated intercept: $\hat{\beta}_0 = \bar{y} - \hat{\beta}_1 \bar{x}$ ### Goodness of Fit * **Coefficient of Determination ($R^2$)**: $R^2 = \frac{ESS}{TSS} = 1 - \frac{RSS}{TSS}$ Where: * ESS (Explained Sum of Squares) * TSS (Total Sum of Squares) * RSS (Residual Sum of Squares) * $R^2$ represents the proportion of the variance in the dependent variable that is predictable from the independent variable(s). ### Assumptions of OLS 1. The error term has a zero population mean. 2. The independent variables are uncorrelated with the error term. 3. The error term has a constant variance (homoscedasticity). 4. The error term is not correlated across observations. 5. The error term is normally distributed. ### Hypothesis Testing * Null Hypothesis: $H_0: \beta_1 = 0$ (no significant relationship) * Alternative Hypothesis: $H_1: \beta_1 \neq 0$ (significant relationship) * Test Statistic (t-statistic): $t = \frac{\hat{\beta}_1 - 0}{SE(\hat{\beta}_1)}$ Where: * $SE(\hat{\beta}_1)$ is the standard error of $\hat{\beta}_1$ * Decision Rule: Reject $H_0$ if $|t| > t_{\alpha/2, n-2}$ or if p-value $< \alpha$ ### Potential Problems * **Multicollinearity**: High correlation among independent variables. * **Heteroscedasticity**: Non-constant variance of the error term. * **Autocorrelation**: Correlation of error terms across observations. ### Diagrams * **Scatter Plot**: A graph in which the values of two variables are plotted along two axes, the pattern of the resulting points revealing any correlation present. * **Regression Line**: A line of best fit showing the relationship between two variables.