COQT111 Slides - Quantitative Techniques - PDF
Document Details
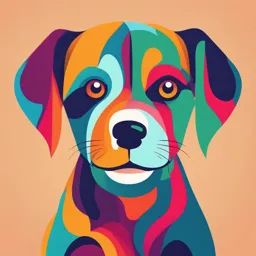
Uploaded by AdoringPentagon
Eduvos
Robbie Stewart
Tags
Summary
These slides provide an overview of the basic concepts related to quantitative techniques in management.
Full Transcript
lOMoARcPSD|14819275 COQT111 Slides Quantitative Techniques (Eduvos) Scan to open on Studocu Studocu is not sponsored or endorsed by any college or university Downloaded by Manyoni Amahle ([email protected]) lOMoARcPSD|14...
lOMoARcPSD|14819275 COQT111 Slides Quantitative Techniques (Eduvos) Scan to open on Studocu Studocu is not sponsored or endorsed by any college or university Downloaded by Manyoni Amahle ([email protected]) lOMoARcPSD|14819275 QUANTITATIVE TECHNIQUES A COQT111 Robbie Stewart 1 Chapter 1 Statistics in Management Prescribed sections 1.1 – 1.9 not 1.4 p 2 - 17 2 Downloaded by Manyoni Amahle ([email protected]) 1 lOMoARcPSD|14819275 1.1 Introduction Management Decision Making Statistics is used by managers to assist them in their decision making. Statistics forms a “management decision support system”. Information versus Data Information must be: Data is: Timely; Readily available; Accurate; Consist of individual values; Relevant; Of little use in its raw form. Adequate; Easily accessible 3 What is Statistics? Statistics can be defined as the science of collecting, organising, analysing and interpreting data in order to make decisions The Statistical Cycle The three key components in identifying problems are: Think systematically; Look for connections and relationships; Understand why data values differ from one another 4 Downloaded by Manyoni Amahle ([email protected]) 2 lOMoARcPSD|14819275 5 1.2 The language of statistics A random variable is an item of interest on which data is collected and analysed Data is the values or outcomes recorded on a random variable A sampling unit is the object being measured, counted or observed with respect to the random variable A population is a set of all objects or individuals of interest from which a sample can be drawn A population parameter describes a characteristic of a population A sample is a subset of data values drawn from a population 6 Downloaded by Manyoni Amahle ([email protected]) 3 lOMoARcPSD|14819275 The language of statistics continued A sample statistic describes a characteristic of a sample 7 1.3 Components of Statistics Descriptive statistics summarises sample data into a few measures Inferential statistics generalises sample findings to the broader population Statistical modelling builds models of relationships between random variables 8 Downloaded by Manyoni Amahle ([email protected]) 4 lOMoARcPSD|14819275 1.5 Statistical Applications in Management Finance Marketing Human Resources Operations and Logistics 1.6 Data and Data Quality Data is the raw material of statistical analysis Data quality is influenced by: ✓ Data quality, data source, the method of data collection and appropriate data preparation Selection of the Statistical Method depends on: ✓ The management problem to be addressed ✓ The type of data available 9 1.7 Data Types and Measurement Scales Qualitative random variables ✓ Data has categorical responses that have no numerical value ✓ Only the number of responses per category can be counted Quanitative random variables ✓ Data has numerical values ✓ Numeric data can be further classified as discrete or continuous 10 Downloaded by Manyoni Amahle ([email protected]) 5 lOMoARcPSD|14819275 Types of Data 11 Quantitative Random Data Discrete data ✓ Data is whole numbers (integers) ✓ Cannot be fractions or decimals ✓ e.g. - Number of students in a class Continuous data ✓ Data can take on any numerical value ✓ Can be fractions or decimals ✓ e.g. – The distanced a student travels to campus in kilometres 12 Downloaded by Manyoni Amahle ([email protected]) 6 lOMoARcPSD|14819275 Measurement scales Nominal data is the weakest form of data to analyse, has no numerical properties, no specific order and is associated with categorical data e.g. male, female Ordinal data is also associated with categorical data but has an implied ranking, is a stronger than nominal data and each consecutive category possesses either more or less than the previous category e.g. small, medium, large Interval data is also associated with numeric data and quantitative random variables Ratio data consists of real numbers associated with quantitative random variables, has all the properties of numbers and is the strongest data for statistical analysis 13 14 Downloaded by Manyoni Amahle ([email protected]) 7 lOMoARcPSD|14819275 15 1.8 Data sources Internal data is sourced from within a company External data is sourced from outside an organisation Primary data is data that is recorded for the first time at source and with a specific purpose in mind. The main advantage is it is high quality data with both relevance and accuracy. The main disadvantage is that it can be time consuming and expensive to collect Secondary data is data that already exists in a processed format. The main advantages are that it is less time consuming and cheaper to collect. The main disadvantages are that it may be less relevant, out of date, difficult to assess data accuracy, it may not be possible to manipulate the data further and by using different data sources the data may become distorted and biased 16 Downloaded by Manyoni Amahle ([email protected]) 8 lOMoARcPSD|14819275 1.9 Data Collection Methods Data collection includes observation, surveys and experimentation Observation is a method to collect primary data. ✓ The advantage of observation is that the respondent is unaware they are being observed and thus behaves more naturally thus reducing the likelihood of collecting biased data. ✓ The disadvantage of observation is that as this is a passive form of data collection there is no opportunity to investigate further. 17 Data Collection Methods (cont) Surveys are a method to collect primary data through direct questioning using questionnaires. Surveys capture mainly attitudinal-type data Surveys are conducted through personal interviews, telephone surveys or e-surveys 18 Downloaded by Manyoni Amahle ([email protected]) 9 lOMoARcPSD|14819275 A personal interview is a face to face questionnaire: ✓ Advantages of a personal interviews include: ❖ Higher response rates ❖ Allows for reasons to be identified ❖ Data is current and more accurate ❖ Allows for questioning of a technical nature ❖ Non-verbal responses can be noted ❖ More questions can be asked ❖ The use of aided–recall questions and other prompts is possible ✓ Disadvantages of a personal interviews include: ❖ Time consuming ❖ Expensive 19 Telephone interviews are often used for snap (straw) surveys: ✓ Advantages of a telephone interviews include: ❖ Data is current and more geographically dispersed ❖ Call backs are possible ❖ Relatively low cost ❖ People are often more willing to talk ❖ Interviewer probing is possible ❖ Questions can be clarified ❖ The use of aided–recall questions is possible ❖ A larger sample of respondents can be reached 20 Downloaded by Manyoni Amahle ([email protected]) 10 lOMoARcPSD|14819275 ✓ Disadvantages of a telephone interviews include: ❖ Lack of anonymity ❖ Non-verbal responses cannot be observed ❖ Trained interviewers are required which increases costs ❖ Interviewer bias can occur ❖ Data can be lost if the respondent puts down the phone ❖ Sampling bias can occur as only people with phones can be interviewed 21 E-surveys are increasingly popular because: ✓ The process is automated eliminating data capture errors ✓ Cheaper and faster ✓ Local, national and international respondents can be reached ✓ Data is current and accurate ✓ Advantages of e-surveys over personal interviews include: ❖ Interviewer bias is eliminated ❖ Respondents have more time ❖ Respondent anonymity is assured 22 Downloaded by Manyoni Amahle ([email protected]) 11 lOMoARcPSD|14819275 ✓ Disadvantages of e-surveys over personal interviews include: ❖ Limited sampling frames ❖ Sampling bias can occur as only people with email, internet access or mobile phones can respond ✓ Disadvantages of e-surveys over traditional postal surveys include: ❖ Lack of personal communication leads to less control over the data collection process ❖ Low response rates ❖ The respondent cannot clarify questions ❖ Survey questions need to be short and simple ❖ Limited opportunity to investigate further ❖ Bias can occur as there is no way to control who answers the questions 23 Experimentation is used to obtain primary data Examples of experimentation include: ✓ Price manipulation ✓ Altering machine settings to examine the effects on product quality ✓ Advantages of experimentation include: ❖ High quality ❖ Data is likely to be accurate and “noise-free” ❖ Data is more reliable and valid than surveys ✓ Disadvantages of experimentation include: ❖ Costly and time consuming ❖ Controlling external factors may confound the results 24 Downloaded by Manyoni Amahle ([email protected]) 12 lOMoARcPSD|14819275 Chapter 2 Summarising Data Prescribed sections 2.1 – 2.3 p 26 – 42 Do not study Stacked Bar Charts, Multiple Bar Charts, Histograms, Box Plot, Trendline Graphs, Lorenz Curve and Pareto Curve. 25 2.1 Introduction p27 26 Downloaded by Manyoni Amahle ([email protected]) 13 lOMoARcPSD|14819275 2.2 Summarising Categorical Data Single Categorical Variable – To construct a categorical frequency table List the categorical variables (column 1) Count the number of occurrences (column 2) For a percentage frequency table convert the count to a percentage (column 3) 27 – To construct a bar chart List the categorical variables on the horizontal axis Scale the vertical axis to the counts (or percentage) Draw the bars to the height of the count (or percentage) 28 Downloaded by Manyoni Amahle ([email protected]) 14 lOMoARcPSD|14819275 – To construct a pie chart Divide a circle into categorical segments The size of each segment must be equal to of the count (or percentage) of its category The sum of the segments must be equal to the sample size (or 100%) 29 2.3 Summarising Numeric Data (p35) – Frequency tabulated (grouped) data 30 Downloaded by Manyoni Amahle ([email protected]) 15 lOMoARcPSD|14819275 2.3 Summarising Numeric Data (p35) – Numeric frequency distribution summarises data into intervals of equal width. (Grouped data) Step 1: Determine the data range Step 2: Choose the number of intervals (between 5 and 8) Step 3: Determine the interval width Step 4: Set the interval limits – The lower limit should be lower than the minimum value. The lower limit for each interval is found by adding the interval width to each preceding lower limit. 31 Step 5: Draw a table and assign to ONE of the intervals. If data is continuous the upper limit of each interval should be < the lower limit of the next intervals lower limit. If data is discrete the whole number one less than the next intervals lower limit must be used 70 −20 – Interval width = = 10 5 32 Downloaded by Manyoni Amahle ([email protected]) 16 lOMoARcPSD|14819275 Cumulative Frequency Distribution (p38) – A cumulative frequency distribution is a summary table of cumulative frequency counts and is used to answer questions of a less than or greater than nature Step 1: Using the numeric frequency distribution add an extra interval below the first interval (the frequency for this interval should be zero (0)) Step 2: Count the number of variables that fall below (or equal to) the maximum value of each interval Alternatively add the frequencies of each interval below the maximum value of the current interval together or the current intervals frequency to the cumulative frequency of the previous interval. Step 3: Check that the value of the cumulative frequency of the last interval is equal to the sample size. 33 Cummulative frequency distribution table 34 Downloaded by Manyoni Amahle ([email protected]) 17 lOMoARcPSD|14819275 Ogive (p39) – An Ogive is a graph of the cumulative frequency distribution. To construct an Ogive: Step 1: On the x axis mark the interval limits. Step 2: On the y axis plot the cumulative frequency against the upper limit of the interval. Step 3: Join the cumulative frequency points with a line graph 35 Ogive – a graph of a cummulative frequency distribution 36 Downloaded by Manyoni Amahle ([email protected]) 18 lOMoARcPSD|14819275 Chapter 3 Describing Data: Numeric Descriptive Statistics Prescribed sections 3.1 – 3.5, 3.8 & 3.10 p 66 – 87, 91 & 93 - 94 Do not study Geometric mean on p73. 37 3.1 Introduction (p66) Three characteristics are commonly used ✓ Measures of central and non-central location ✓ Measures of dispersion ✓ Measures of skewness 38 Downloaded by Manyoni Amahle ([email protected]) 19 lOMoARcPSD|14819275 Using your Casio fx82 to calculate measures Ungrouped Data is data that is given as individual data points Ungrouped data has no frequency distribution On your Casio fx82 calculator you must setup the Stats Mode to frequency OFF for ungrouped data ✓ Press Shift ; Setup ; down arrow ; 3 (STAT) ; 2 (OFF) ✓ Press Mode ; 2 (STAT) ; 1 (1-VAR) Grouped Data is numeric data that is summarized into intervals of equal width showing how many data values (frequency (f)) fall within that interval. On your Casio fx82 calculator you must setup the Stats Mode to frequency ON for grouped data ✓ Press Shift ; Setup ; down arrow ; 3 (STAT) ; 1 (ON) ✓ Press Mode ; 2 (STAT) ; 1 (1-VAR) 39 3.2 Central Location Measures p67 – Arithmetic Mean (Average) ALWAYS SHOW ALL YOUR WORKINGS ✓On your calculator choose Option 3:Sum ; Option 2:Σx (Σx = 376) ✓ n = Option 4:Var ; Option 1:n (n = 20) UNGROUPED DATA 376 ✓ x̄ = = 18.8 20 ✓ Check your answer x̄ = Option 4:Var ; Option 2: x̄ = 18.8 40 Downloaded by Manyoni Amahle ([email protected]) 20 lOMoARcPSD|14819275 Central Location Measures GROUPED DATA – Arithmetic Mean (Average) σ 𝑥 = 1130 𝑛 = 30 1130 𝑥ҧ = = 37.67 minutes 30 41 Central Location Measures UNGROUPED DATA – Median (middle number) p68 sequence in ascending order 13 15 15 16 18 18 18 18 18 19 20 20 20 20 20 20 21 21 22 24 select average of 2 middle numbers if n is an even number (median = (19+20)÷2 = 19.5) select middle number if n is an odd number – Mode (Most frequently occurring) p70 Mode = 20 as it occurs six times 42 Downloaded by Manyoni Amahle ([email protected]) 21 lOMoARcPSD|14819275 Central Location Measures GROUPED DATA – Median (Me) Ome is the opening value of the class in which the median occurs c is the class width f( 1.96 reject H0 – It is concluded with 95% confidence that the mean spending of grocery shoppers is not R175. – The claim by the Grocery Shoppers Association is therefore rejected at the 5% level of significance. 142 Downloaded by Manyoni Amahle ([email protected]) 71 lOMoARcPSD|14819275 8.4 Hypothesis Test for a Single Population Mean (μ) – Population Standard Deviation (σ) is Unknown Example 8.3 page 212 SARS claim it takes, on average, less than 45 minutes to complete a tax return via eFiling Time taken to complete tax return on eFiling 42 56 29 35 47 37 39 29 45 35 51 53 𝑠 𝑆𝑡𝑎𝑛𝑑𝑎𝑟𝑑 𝑒𝑟𝑟𝑜𝑟 = 𝑛 = 12 (df = 11) 𝑛 𝑥 = 41.5 𝑥−𝜇 𝑠 = 9.04 𝑡 − 𝑠𝑡𝑎𝑡 = 𝑠 𝛼 = 0.05 𝑛 𝑡 − 𝑐𝑟𝑖𝑡 = ±1.796 143 Hypothesis Testing – Step 1: Define the Statistical Hypotheses (Null and Alternative) One-sided Lower-tailed Hypothesis Test 𝐻0 : 𝜇 ≥ 45 (It takes 45 minutes or more to complete a tax return) 𝐻1 : 𝜇 < 45 (It takes less than 45 minutes to complete a tax return) 144 Downloaded by Manyoni Amahle ([email protected]) 72 lOMoARcPSD|14819275 Step 2: Determine the Region of Acceptance of the Null Hypothesis Accept H0 if t-stat ≥ -1.796 Accept H1 (reject H0) if t-stat < -1.796 Step 3: Calculate the Sample Test Statistic 𝑠 9.04 𝑆𝑡𝑎𝑛𝑑𝑎𝑟𝑑 𝑒𝑟𝑟𝑜𝑟 = = = 2.6096 𝑛 12 x − 41.5 − 45 − 3.5 t − stat = = = = −1.341 s 9.04 2.6096 n 12 145 – Step 4: Compare the Sample Test Statistic to the Area of Acceptance Critical t = -1.796 t-crit = -1.796 146 Downloaded by Manyoni Amahle ([email protected]) 73 lOMoARcPSD|14819275 – Step 5: Draw Statistical and Management Conclusions t-stat = -1.796 ≤ -1.341 Accept H0 : µ ≥ 45 It can be concluded with 95% confidence that the mean time to complete a tax return via eFiling is no less than 45 minutes. The claim by SARS is therefore rejected at the 5% level of significance. 147 When to use the Student t -statistic instead of the z –stat (p214) – If σ is unknown and n ≤ 40 use the t stat (with appropriate degrees of freedom) – If σ is unknown and n > 40 use the z stat as a good approximation of the t stat and use s instead of σ 148 Downloaded by Manyoni Amahle ([email protected]) 74 lOMoARcPSD|14819275 8.5 Hypothesis Test for a Single Population Proportion (π) (p215) Example 8.4 A Cell phone company claims it has 15% market share. If a survey of 360 users shows 42 use the cell company test the claim at the 1% level of significance. n = 360 42 z − stat = (p − ) p= = 0.1167 360 (1 − ) = 0.15 n 1 = 0.01 z − crit = 2.58 149 Hypothesis Testing – Step 1: Define the Statistical Hypotheses (Null and Alternative) Two-sided Hypothesis Test H 0 : = 0.15 H1 : 0.15 150 Downloaded by Manyoni Amahle ([email protected]) 75 lOMoARcPSD|14819275 Step 2: Determine the Region of Acceptance of the Null Hypothesis α = 0.01/2 = 0.005 z-crit = ±2.58 Accept H0 if -2.58 ≤ z ≤2.58 Accept H1 (reject H0) if z < -2.58 or z > 2.58 Step 3: Calculate the Sample Test Statistic 42 𝑝−𝜋 − 0.15 𝑧 − 𝑠𝑡𝑎𝑡 = = 360 𝜋 1−𝜋 0.15 1 − 0.15 𝑛 360 0.1167 − 0.15 = = −1.771 0.0188 151 – Step 4: Compare the Sample Test Statistic to the Area of Acceptance 152 Downloaded by Manyoni Amahle ([email protected]) 76 lOMoARcPSD|14819275 – Step 5: Draw Statistical and Management Conclusions – z-stat = -1.771 ≥ critical z -2.58, the z-stat lies within the area of acceptance of H0 : µ = 0.15 – We can say with 99% confidence that the claim by the cell phone company that they have a 15% market share is true. 153 Errors encountered in Hypothesis Testing (p203) – Type I errors – Reject H0 when it is if fact true – Type II errors – Accept H0 when it is if fact false 154 Downloaded by Manyoni Amahle ([email protected]) 77 lOMoARcPSD|14819275 Chapter 9 Hypothesis Testing: Comparison between Two Populations (Means and Proportions) Prescribed sections 9.1 – 9.3 and 9.5 p 235 – 242 and 247 - 251 155 – The z standardization formula is: – Where: (𝑥1ҧ − 𝑥ҧ2 ) is the difference in sample means – Where: (μ1 − μ2 ) is the difference in population means 156 Downloaded by Manyoni Amahle ([email protected]) 78 lOMoARcPSD|14819275 Hypothesis Testing (Difference between two means where σ is known) – Example 9.1 (p236) Courier A used 60 times with an average delivery time of 42 minutes and a population standard deviation of 14 minutes. Courier B used 48 times with an average delivery time of 38 minutes and a population standard deviation of 10 minutes. Test the claim that there is no statistically significant difference between the courier companies at a 5% level of significance 157 Hypothesis Testing (Difference between two means where σ is known) – Step 1: Define the Statistical Hypotheses (Null and Alternative) Two-sided Hypothesis Test 𝐻0 : 𝜇1 − 𝜇2 = 0 (no significant difference in courier delivery times) 𝐻1 : 𝜇1 − 𝜇2 ≠ 0 (there is a significant difference in courier delivery times) 158 Downloaded by Manyoni Amahle ([email protected]) 79 lOMoARcPSD|14819275 Step 2: Determine the Region of Acceptance of the Null Hypothesis α = 0.05/2 = 0.025 z-crit = ±1.96 Accept H0 if -1.96 ≤ z ≤ 1.96 Accept H1 (reject H0) if z < -1.96 or z > 1.96 Step 3: Calculate the Sample Test Statistic 𝑥ҧ1 − 𝑥ҧ2 − 0 42 − 38 − 0 𝑧 − 𝑠𝑡𝑎𝑡 = = = 1.73 𝜎12 𝜎22 142 102 + + 60 48 𝑛1 𝑛2 159 Step 4: Compare sample statistic to critical value Sample test z stat is within the critical z value limits z-stat = 1.73 Step 5: Statistical and management conclusion At a 5% level of significance we accept H0 There is no statistically significant difference between the courier companies 95% of the time. 160 Downloaded by Manyoni Amahle ([email protected]) 80 lOMoARcPSD|14819275 161 Hypothesis Testing (Difference between two means where σ is unknown) – Example 9.2 (p241) A financial analyst proposes the % return on investment (ROI%) for financial companies is greater than the ROI% for manufacturing firms. A sample of 28 financial firms provides a mean ROI% of 18.714% with a sample standard deviation of 9.645% and a sample of 24 manufacturing firms provides a mean ROI% of 15.125% with a sample standard deviation of 8.823%. Conduct a hypothesis test at a 5% level of significance. 162 Downloaded by Manyoni Amahle ([email protected]) 81 lOMoARcPSD|14819275 Hypothesis Testing (Difference between two means where σ is unknown) – Step 1: Define the Statistical Hypotheses (Null and Alternative) One-sided upper tailed Hypothesis Test 𝐻0 : 𝜇1 − 𝜇2 ≤ 0 (ROI% of financial firms is not significantly greater than ROI% of manufacturing firms) 𝐻1 : 𝜇1 − 𝜇2 > 0 (ROI% of financial firms is greater than ROI% of manufacturing firms as per the analysts claim) 163 Step 2: Determine the Region of Acceptance of the Null Hypothesis α = 0.05 where degrees of freedom (df) = 𝑛1 + 𝑛2 − 2 = 28 + 24 – 2 = 50 t-crit = +1.676 Accept H0 if t ≤ 1.676 Accept H1 (reject H0) if t > 1.676 164 Downloaded by Manyoni Amahle ([email protected]) 82 lOMoARcPSD|14819275 Step 3: Calculate the Sample Test Statistic 𝑛1 − 1 𝑠12 + 𝑛2 − 1 𝑠22 𝑠𝑝2 = 𝑛1 + 𝑛2 − 2 28 − 1 × 9.6452 + 24 − 1 × 8.8232 = = 86.0468 28 + 24 − 2 𝑥ҧ1 − 𝑥ҧ2 − 𝜇1 − 𝜇2 18.714 − 15.125 − 0 𝑡 − 𝑠𝑡𝑎𝑡 = = 1 1 1 1 𝑠𝑝2 + 86.0468 × 28 + 24 𝑛1 𝑛2 = 1.391 165 Step 4: Compare sample statistic to critical value Sample test t stat is within the critical t value limits t-stat = 1.391 < t-crit = 1.676 166 Downloaded by Manyoni Amahle ([email protected]) 83 lOMoARcPSD|14819275 Step 5: Statistical and management conclusion At a 5% level of significance we accept H0 There is no statistically significant difference between the ROI% of financial and manufacturing firms at the 5% level of significance. The claim by the financial analyst that the ROI% of financial firms is greater than the ROI% of manufacturing firms is rejected at the 5% level of significance 167 ( p1 − p2 ) → ( 1 − 2 ) 168 Downloaded by Manyoni Amahle ([email protected]) 84 lOMoARcPSD|14819275 Hypothesis Testing (Difference between two proportions) – Example 9.4 (p248) A research company is required to establish if the recall rate of teenagers is different from the recall rate for young adults of a recent AIDS awareness campaign. A sample of 640 teenagers and 420 young adults were interviewed. 362 teenagers and 260 young adults were able to recall the AIDS awareness campaign. Conduct a hypothesis test at a 5% level of significance to test the claim that there is an equal recall rate for both groups. 169 Hypothesis Testing (Difference between two proportions) – Step 1: Define the Statistical Hypotheses (Null and Alternative) Two tailed Hypothesis Test 𝐻0 : 𝜋1 − 𝜋2 = 0 (as per the claim of equal recall rates) 𝐻1 : 𝜋1 − 𝜋2 ≠ 0 170 Downloaded by Manyoni Amahle ([email protected]) 85 lOMoARcPSD|14819275 Step 2: Determine the Region of Acceptance of the Null Hypothesis α = 0.05/2 = 0.025 where degrees of freedom (df) = 𝑛1 + 𝑛2 = 640 + 420 = 1060 z-crit = ±1.96 Accept H0 if -1.96 ≤ z ≤ 1.96 Accept H1 (reject H0) if z < -1.96 or z > 1.96 171 Step 3: Calculate the Sample Test Statistic 362 𝑝1 = 640 = 0.5656 where 𝑛1 = 640 260 𝑝2 = = 0.619 where 𝑛2 = 420 420 362 + 260 𝜋ො = = 0.5868 640 + 420 1 1 Standard error = 𝜋ො × 1 − 𝜋ො × 𝑛1 + 𝑛2 = 1 1 0.5868 × 1 − 0.5868 × + = 0.0309 640 420 172 Downloaded by Manyoni Amahle ([email protected]) 86 lOMoARcPSD|14819275 Standard error = 0.0309 𝑝1 − 𝑝2 − 𝜋1 − 𝜋2 𝑧 − 𝑠𝑡𝑎𝑡 = 𝑠𝑡𝑎𝑛𝑑𝑎𝑟𝑑 𝑒𝑟𝑟𝑜𝑟 0.5656 − 0.619 − 0 = 0.0309 = −1.728 173 Step 4: Compare sample statistic to critical value Sample test t stat is within the critical t value limits z-crit = − 1.96 < z-stat = −1.728 < z-crit = + 1.96 174 Downloaded by Manyoni Amahle ([email protected]) 87 lOMoARcPSD|14819275 Step 5: Statistical and management conclusion At a 5% level of significance we accept H0 We can say with 95% confidence that there is no statistically significant difference in the recall rate of the AIDS awareness campaign between teenagers and young adults. 175 Chapter 10 Chi-Square Hypothesis Tests Prescribed sections 10.1 – 10.3 p 271 – 281 176 Downloaded by Manyoni Amahle ([email protected]) 88 lOMoARcPSD|14819275 10.1 Introduction and Rationale The chi-squared statistic, written as χ2, is used to test hypothesis on patterns of outcomes for categorical random variables. The chi-squared test can be used in three different contexts, of which we study two: to test for independence of association between two categorical variables, e.g. ‘Is the choice of magazine read associated with the reader’s gender? 177 to test for equality of proportions across two or more populations, e.g. ‘Is the percentage of unionised workers per firm the same across four construction firms? as a goodness-of-fit test, (is not within the scope of this course) e.g. ‘Is the completion time (in minutes) for a task normally distributed? 178 Downloaded by Manyoni Amahle ([email protected]) 89 lOMoARcPSD|14819275 The Chi-Squared Statistic The chi-squared test is based on frequency count data. It always compares a set of observed frequencies obtained from a random sample to a set of expected frequencies that describes the null hypothesis. 179 10.2 The Chi-Square test for Independence of Association – Example 10.1 (p273) A company publishes 3 magazines, Beat, Youth and Grow. Management would like to know if teenage readership preference is independent of gender. A survey of 200 randomly selected teenagers (80 girls and 120 boys) was conducted and their magazine preference and gender recorded. 180 Downloaded by Manyoni Amahle ([email protected]) 90 lOMoARcPSD|14819275 Table of gender by magazine preference Conduct a chi-squared hypothesis test, at a 5% level of significance, to determine if there is a statistical association between gender and magazine preference. 181 Exploratory Data Analysis By converting the frequency counts to percentages any likely association between gender and magazine preference may be identifiable. Females seem to prefer Grow magazine, males seem to prefer Beat magazine and the preference for Youth magazine seems evenly spread between genders. 182 Downloaded by Manyoni Amahle ([email protected]) 91 lOMoARcPSD|14819275 Hypothesis test for independence of association Step 1: Define the null and alternative hypotheses 𝐻0: There is no association between gender and magazine preference. 𝐻1: There is an association between gender and magazine preference. 183 184 Downloaded by Manyoni Amahle ([email protected]) 92 lOMoARcPSD|14819275 Step 2: Determine the region of acceptance of the null hypothesis α = 0.05 𝑑𝑓 = (𝑟 − 1)(𝑐 − 1) df = (2-1)x(3-1) = 2 Critical χ2 = 5.991. Accept H0 if χ2 ≤ 5.991 185 Step 3: Calculate the sample test statistic (χ2 -stat) To calculate the expected frequencies: 𝑟𝑜𝑤 𝑡𝑜𝑡𝑎𝑙 × 𝑐𝑜𝑙𝑢𝑚𝑛 𝑡𝑜𝑡𝑎𝑙 𝑓𝑒 = 𝑛 186 Downloaded by Manyoni Amahle ([email protected]) 93 lOMoARcPSD|14819275 Construct the χ2 table for observed (fo) and expected (fe) frequencies 187 Step 4: Compare the sample statistic to the region of acceptance Sample χ2 = 4.964 < Critical χ2 = 5.991 Sample χ2 is within the critical χ2 limits 188 Downloaded by Manyoni Amahle ([email protected]) 94 lOMoARcPSD|14819275 Step 5: Statistical and management conclusion At a 5% level of significance we accept H0 There is no statistically significant difference in the preference for magazines based on the readers gender. 189 10.3 Hypothesis test for equality of multiple proportions – Example 10.2 (p278) A retail company would like to know if the proportion of customers with loyalty cards is the same in three different stores at the 10% significance level. A random sample of 180 customers across the three different stores was selected and the number of loyalty card holders recorded. 190 Downloaded by Manyoni Amahle ([email protected]) 95 lOMoARcPSD|14819275 Hypothesis test for equality of multiple proportions Step 1: Define the null and alternative hypotheses 𝐻0: π1 = π2 = π3 (Proportions are equal across all stores). 𝐻1: Proportions are not equal in at least one store. 191 Step 2: Determine the region of acceptance of the null hypothesis α = 0.1 𝑑𝑓 = (𝑟 − 1)(𝑐 − 1) df = (2-1)x(3-1) = 2 Critical χ2 = 4.605 Accept H0 if χ2 ≤ 4.605 192 Downloaded by Manyoni Amahle ([email protected]) 96 lOMoARcPSD|14819275 Step 3: Calculate the sample test statistic (χ2 -stat) To calculate the expected frequencies: 𝑟𝑜𝑤 𝑡𝑜𝑡𝑎𝑙 × 𝑐𝑜𝑙𝑢𝑚𝑛 𝑡𝑜𝑡𝑎𝑙 𝑓𝑒 = 𝑛 193 Construct the χ2 table for observed (fo) and expected (fe) frequencies 194 Downloaded by Manyoni Amahle ([email protected]) 97 lOMoARcPSD|14819275 Step 4: Compare the sample statistic to the region of acceptance Sample χ2 = 3.805 < Critical χ2 = 4.605 Sample χ2 is within the critical χ2 limits 195 Step 5: Statistical and management conclusion At a 10% level of significance we accept H0 There is no statistically significant difference in the proportion of loyalty card holders in the three different stores. 196 Downloaded by Manyoni Amahle ([email protected]) 98 lOMoARcPSD|14819275 Chapter 12 Linear Regression and Correlation Analysis Prescribed sections 12.1 – 12.5 p 328 – 342 197 12.1 Introduction In management, many numeric measures are related (either strongly or loosely) to one another. For example: advertising expenditure is assumed to influence sales volumes; a company’s share price is likely to be influenced by its return on investment; the number of hours of operator training is believed to impact positively on productivity. 198 Downloaded by Manyoni Amahle ([email protected]) 99 lOMoARcPSD|14819275 Scatter plot between pairs of x and y 199 12.2 Simple Linear Regression Analysis Simple linear regression analysis finds a straight- line equation that represents the relationship between the values of two numeric variables. Where: y = a + bx y = dependent variable a = intercept on the vertical axis (value of y when x = 0) y b = gradient (b = x ) x = independent variable 200 Downloaded by Manyoni Amahle ([email protected]) 100 lOMoARcPSD|14819275 Example 12.1 Flat screen TV Sales p330 Step1: Identify the dependent and independent variables Step 2: Construct a scatter plot 201 Step3: Calculate the linear regression equation using the STAT mode in your calculator Press MODE choose STAT Select 2:A+BX enter the x and y variables Press AC 𝑛 σ 𝑥𝑦 − σ 𝑥 σ 𝑦 To determine b use the formula 𝑏 = σ 2 σ 2 𝑛 𝑥 − 𝑥 σ 𝑦 −𝑏 σ 𝑥 To determine a use the formula 𝑎 = 𝑛 From the 5:Reg option retrieve the values for 1:A and 2:B to confirm your answers Plot the regression line Note: In formula 12.1 on page 333 the text book refers to b0 instead of a and b1 instead of b 202 Downloaded by Manyoni Amahle ([email protected]) 101 lOMoARcPSD|14819275 𝑦 = 12.817 + 4.368𝑥 203 12.3 Correlation Analysis The reliability of the estimate of y depends on the strength of the relationship between the x and y variables. A strong relationship implies a more accurate and reliable estimate of y. Correlation analysis measures the strength of the linear association between two numeric (ratio-scaled) variables, x and y. 204 Downloaded by Manyoni Amahle ([email protected]) 102 lOMoARcPSD|14819275 Correlation Analysis (cont) This measure is called Pearson’s correlation coefficient. It is represented by the symbol r When r is calculated from sample data the following formula is used : The value or r can be confirmed by retrieving the r value with your calculator Press Shift 1 Press 5:Reg Retrieve the value for 5:r r2 can be calculated by pressing the x2 button 205 Correlation Analysis (cont) Pearson’s correlation coefficient can be calculated using the formula’s provided in the formula sheet Press Shift 1 Press 3:Sum Retrieve the values for 1:∑x2; 2:∑x ; 3:∑y2; 4:∑y; 5:∑xy Press Shift 1 Press 4:Var Retrieve the values for 1:n 206 Downloaded by Manyoni Amahle ([email protected]) 103 lOMoARcPSD|14819275 Predicting the value of the dependent variable for a given independent variable Once the regression equation has been estimated it is possible to predict the value of the dependent variable (y) for any given dependent variable (x) by substitution. Flat-screen TV sales and adverts placed Adverts 4 4 3 2 5 2 4 3 5 5 3 4 Sales 26 28 24 18 35 24 36 25 31 37 30 32 a = 12.816; b = 4.368 y = 12.816 + 4.368x Estimate the sales level if the firm places 6 advertisements y = 12.816 + 4.368(6) = 39 Thus if 6 adverts are placed we predict the firm will sell 39 TV’s 207 How to interpret a Correlation Coefficient 208 Downloaded by Manyoni Amahle ([email protected]) 104 lOMoARcPSD|14819275 Perfect associations 209 Strong associations 210 Downloaded by Manyoni Amahle ([email protected]) 105 lOMoARcPSD|14819275 Moderate to weak associations 211 No association 212 Downloaded by Manyoni Amahle ([email protected]) 106 lOMoARcPSD|14819275 12.4 The Coefficient of Determination (r2) The coefficient of determination can be calculated by squaring the correlation coefficient (r) 0 ≤ r2 ≤ 1 which can be interpreted as 0% ≤ r2 ≤ 100% 213 12.5 Testing the Regression Model for Significance Example 12.4 page 341 Flat-screen TV sales and adverts placed Adverts 4 4 3 2 5 2 4 3 5 5 3 4 Sales 26 28 24 18 35 24 36 25 31 37 30 32 Use the same 5 steps as Hypothesis Testing – Step 1: Define the Statistical Hypotheses (Null and Alternative) Two tailed Hypothesis Test 𝐻0 : 𝜌 = 0 (No relationship between adverts and sales) 𝐻1 : 𝜌 ≠ 0 (Adverts and sales are related) 214 Downloaded by Manyoni Amahle ([email protected]) 107 lOMoARcPSD|14819275 Step 2: Determine the Region of Acceptance of the Null Hypothesis At a 5% level of significance α = 0.025 Degrees of freedom (df) = n – 2 = 12 – 2 = 10 Accept H0 if -2.228 ≤ t-stat ≤ 2.228 Accept H1 (reject H0) if t-stat < -2.228 or > 2.228 Step 3: Calculate the Sample Test Statistic 𝑛−2 12 − 2 10 𝑡 − 𝑠𝑡𝑎𝑡 = 𝑟 = 0.8198 = 0.8198 × = 4.527 1 − 𝑟2 1 − 0.81982 0.3279 215 – Step 4: Compare the Sample Test Statistic to the Area of Acceptance t stat = 4.527 > critical t = 2.228 Reject H0 216 Downloaded by Manyoni Amahle ([email protected]) 108 lOMoARcPSD|14819275 – Step 5: Draw Statistical and Management Conclusions t-stat = 4.527 > critical t = 2.228 Reject H0 : 𝜌 = 0 Accept 𝐻1 : 𝜌 ≠ 0 It can be concluded with 95% confidence that there is a strong positive correlation between the number of advertisements placed and the sales of flat-screen TV’s. 217 Chapter 14 Index Numbers Prescribed sections 14.1 – 14.3 p 375 – 390 218 Downloaded by Manyoni Amahle ([email protected]) 109 lOMoARcPSD|14819275 14.1 Introduction Classification of Index Numbers There are two major categories of index numbers. Within each category, an index can be calculated for either a single item or a basket of related items. These categories are: price indexes – single price index – composite price index quantity indexes – single quantity index – composite quantity index 219 14.2 Price Indexes Simple Price Index (Price Relative) The simple price index is the change in price from a base period to another time period for a single item. It is also called a price relative. Example 14.1 p377 In January 2008 petrol cost R6.87 per litre, in January 2009 the price was R7.18 per litre, in January 2010 the price was R7.58 per litre and in January 2011 the price was R8.44 per litre. Using 2008 as the base year calculate the price relatives for 2009, 2010 and 2011 220 Downloaded by Manyoni Amahle ([email protected]) 110 lOMoARcPSD|14819275 Example 14.1 p377 6.87 𝑃𝑟𝑖𝑐𝑒 𝑅𝑒𝑙𝑎𝑡𝑖𝑣𝑒(2008) = × 100 = 100 (Base year) 6.87 7.18 𝑃𝑟𝑖𝑐𝑒 𝑅𝑒𝑙𝑎𝑡𝑖𝑣𝑒(2009) = 6.87 × 100 = 104.5 (4.5% increase since 2008) 7.58 𝑃𝑟𝑖𝑐𝑒 𝑅𝑒𝑙𝑎𝑡𝑖𝑣𝑒(2010) = × 100 = 110.3 (10.3% increase 6.87 since 2008) 8.44 𝑃𝑟𝑖𝑐𝑒 𝑅𝑒𝑙𝑎𝑡𝑖𝑣𝑒(2011) = 6.87 × 100 = 122.9 (22.9% increase since 2008) 221 Composite Price Index for a Basket of Items The composite price index measures the average price change for a basket of related items from one time period (the base period) to another time period (the current period) ✓ To calculate the composite price index each item is weighted according to it’s importance ✓ Importance is determined by the value of each item (price x quantity) ✓ To determine weighting quantities consumed must be held constant 222 Downloaded by Manyoni Amahle ([email protected]) 111 lOMoARcPSD|14819275 Laspeyres vs Paasche weighting methods The Laspeyres approach holds quantities constant at base period values The Paasche approach holds quantities constant at current period values 223 Example 14.2 p379 Using 2010 as the base year calculate the Laspeyres weighted aggregate composite price index for 2011. Base Year (2010) Current Year (2011) Unit Price Quantity Unit Price Quantity Toiletry Items p0 q0 p1 q1 Soap R1.95 37 R2.10 40 Deodorant R14.65 24 R15.95 18 Toothpaste R6.29 14 R6.74 16 Step 1: Find the base year σ 𝑝0 × 𝑞0. Base Year Value Toiletry Items p0 q0 p0 x q0 Soap R1.95 37 72.15 Deodorant R14.65 24 351.60 Toothpaste R6.29 14 88.06 ∑(p0 x q0) 511.80 224 Downloaded by Manyoni Amahle ([email protected]) 112 lOMoARcPSD|14819275 Step 2: Find the current year σ 𝑝1 × 𝑞0. Current Year Value Toiletry Items p1 q0 p1 x q0 Soap R2.10 37 77.70 Deodorant R15.95 24 382.80 Toothpaste R6.74 14 94.36 ∑(p1 x q0) 554.86 σ 𝑝1 ×𝑞0 Step 3: Calculate the composite price index σ × 100 𝑝0 ×𝑞0 554.86. 511.81 × 100 = 108.84 Step 4: Management interpretation Between 2010 and 2011 the prices of the basket of toiletry items increased by 8.84% using the Laspeyres weighted aggregates method. 225 Example 14.2 p379 Using 2010 as the base year calculate the Paasche weighted aggregate composite price index for 2011. Base Year (2010) Current Year (2011) Unit Price Quantity Unit Price Quantity Toiletry Items p0 q0 p1 q1 Soap R1.95 37 R2.10 40 Deodorant R14.65 24 R15.95 18 Toothpaste R6.29 14 R6.74 16 Step 1: Find the base year σ 𝑝0 × 𝑞1. Base Year Value Toiletry Items p0 q1 p0 x q1 Soap R1.95 40 78.00 Deodorant R14.65 18 263.70 Toothpaste R6.29 16 100.64 ∑(p0 x q1) 442.34 226 Downloaded by Manyoni Amahle ([email protected]) 113 lOMoARcPSD|14819275 Step 2: Find the current year σ 𝑝1 × 𝑞1. Current Year Value Toiletry Items p1 q1 p1 x q1 Soap R2.10 40 84.00 Deodorant R15.95 18 287.10 Toothpaste R6.74 16 107.84 ∑(p1 x q1) 478.94 σ 𝑝1 ×𝑞1 Step 3: Calculate the composite price index σ × 100 𝑝0 ×𝑞1 478.94. × 100 = 108.3 442.34 Step 4: Management interpretation Between 2010 and 2011 the prices of the basket of toiletry items increased by 8.3% using the Paasche weighted aggregates method. 227 14.3 Quantity Indexes A quantity index measures the percentage change in consumption level, either for a single item (e.g. milk) or a basket of items (e.g. hardware tools), from one time period to another. Simple Quantity Index (Quantity Relative) The simple quantity index is the change in quantity from a base period to another time period for a single item. It is also called a quantity relative. 𝑞1 𝑄𝑢𝑎𝑛𝑡𝑖𝑡𝑦 𝑟𝑒𝑙𝑎𝑡𝑖𝑣𝑒 = × 100% 𝑞0 228 Downloaded by Manyoni Amahle ([email protected]) 114 lOMoARcPSD|14819275 Example 14.5 p385 In 2009 a hardware store sold 143 doors. In 2010 they sold 122 doors and in 2011 they sold 174 doors. Using 2009 as the base year calculate the quantity relatives for 2010 and 2011 143 𝑄𝑢𝑎𝑛𝑡𝑖𝑡𝑦 𝑅𝑒𝑙𝑎𝑡𝑖𝑣𝑒(2009) = × 100 = 100 (Base year) 143 122 𝑄𝑢𝑎𝑛𝑡𝑖𝑡𝑦 𝑅𝑒𝑙𝑎𝑡𝑖𝑣𝑒(2010) = × 100 = 85.3 (14.7% 143 decrease since 2009) 174 𝑄𝑢𝑎𝑛𝑡𝑖𝑡𝑦 𝑅𝑒𝑙𝑎𝑡𝑖𝑣𝑒(2011) = 143 × 100 = 121.7 (21.7% increase since 2009) 229 Composite Quantity Index for a basket of items The composite quantity index measures the average quantity change for a basket of related items from one time period (the base period) to another time period (the current period) ✓ To calculate the composite quantity index each item is weighted according to it’s importance ✓ Importance is determined by the value of each item (price x quantity) ✓ To determine weighting prices must be held constant 230 Downloaded by Manyoni Amahle ([email protected]) 115 lOMoARcPSD|14819275 Laspeyres vs Paasche weighting methods The Laspeyres approach holds prices constant at base period values The Paasche approach holds prices constant at current period values Σ 𝑝0 𝑞1 𝐿𝑎𝑠𝑝𝑒𝑦𝑟𝑒𝑠 𝑞𝑢𝑎𝑛𝑡𝑖𝑡𝑦 𝑖𝑛𝑑𝑒𝑥 = Σ(𝑝0 𝑞0 ) Σ 𝑝1 𝑞1 𝑃𝑎𝑎𝑠𝑐ℎ𝑒 𝑞𝑢𝑎𝑛𝑡𝑖𝑡𝑦 𝑖𝑛𝑑𝑒𝑥 = Σ(𝑝1 𝑞0 ) 231 Example 14.6 p386 Using 2010 as the base year calculate the Laspeyres weighted aggregate composite quantity index for 2011. Base Year (2010) Current Year (2011) Unit Price Quantity Unit Price Quantity Carpentry Items p0 q0 p1 q1 Cold glue R13.00 45 R15.00 52 Boards R63.00 122 R77.00 110 Paint R122.00 16 R125.00 20 Step 1: Find the base year σ 𝑝0 × 𝑞0. Base Year Value Carpentry Items p0 q0 p0 x q0 Cold glue R13.00 45 585.00 Boards R63.00 122 7686.00 Paint R122.00 16 1952.00 ∑(p0 x q0) 10223.00 232 Downloaded by Manyoni Amahle ([email protected]) 116 lOMoARcPSD|14819275 Step 2: Find the current year σ 𝑝0 × 𝑞1. Current Year Value Carpentry p0 q1 p0 x q1 Items Cold glue R13.00 52 676.00 Boards R63.00 110 6930.00 Paint R122.00 20 2440.00 ∑(p0 x q1) 10046.00 σ 𝑝0 ×𝑞1 Step 3: Calculate the composite quantity index σ × 100 𝑝0 ×𝑞0 10046 × 100 = 98.27 10223 Step 4: Management interpretation Between 2010 and 2011 the quantities of the basket of carpentry items decreased by 1.73% using the Laspeyres weighted aggregates method. 233 Example 14.6 p386 Using 2010 as the base year calculate the Paasche weighted aggregate composite quantity index for 2011. Base Year (2010) Current Year (2011) Unit Price Quantity Unit Price Quantity Carpentry Items p0 q0 p1 q1 Cold glue R13.00 45 R15.00 52 Boards R63.00 122 R77.00 110 Paint R122.00 16 R125.00 20 Step 1: Find the base year σ 𝑝1 × 𝑞0. Base Year Value Carpentry Items p1 q0 p1 x q0 Cold glue R15.00 45 675.00 Boards R77.00 122 9394.00 Paint R125.00 16 2000.00 ∑(p0 x q1) 12069.00 234 Downloaded by Manyoni Amahle ([email protected]) 117 lOMoARcPSD|14819275 Step 2: Find the current year σ 𝑝1 × 𝑞1. Current Year Value Carpentry Items p1 q1 p1 x q1 Cold glue R15.00 52 780.00 Boards R77.00 110 8470.00 Paint R125.00 20 2500.00 ∑(p1 x q1) 11750.00 σ 𝑝1 ×𝑞1 Step 3: Calculate the composite quantity index σ × 100 𝑝0 ×𝑞1 11750 × 100 = 97.36 12069 Step 4: Management interpretation Between 2010 and 2011 the quantities of the basket of carpentry items decreased by 2.64% using the Paasche weighted aggregates method. 235 THE END 236 Downloaded by Manyoni Amahle ([email protected]) 118