P-Values and NHST PDF
Document Details
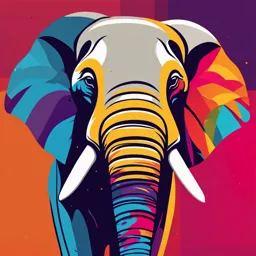
Uploaded by LargeCapacityAntigorite4770
Charles Marks
Tags
Related
- Tema 3: Introducción al Contraste de Hipótesis PDF
- Apuntes - Pruebas de hipótesis PDF
- Sesión 01 PPT - Inferencia Estadística - Universidad Nacional Autónoma de Alto Amazonas - 2024 PDF
- ANOVA PDF
- Hypothesis Testing, P Values and Statistical Inference MD115 PDF
- Data Analysis for Marketing Decisions - Lecture Notes PDF
Summary
This document discusses inferential statistics techniques and null hypothesis significance testing (NHST). It explains the basic principles behind the approach and provides context through the different approaches by Fisher and Neyman-Pearson. The document is primarily focused on providing an overview of the subject, and is not an examination paper.
Full Transcript
P-Values and NHST Charles Marks Scientific Research Questions and Null Hypothesis Significance Testing Framework Introduction In the prior chapter, we discussed how statistics is an art of making probablistic guesses about the nature of phenomena that we observe. At its heart, inferential statistic...
P-Values and NHST Charles Marks Scientific Research Questions and Null Hypothesis Significance Testing Framework Introduction In the prior chapter, we discussed how statistics is an art of making probablistic guesses about the nature of phenomena that we observe. At its heart, inferential statistics techniques make an assumption that a signal does not exist and then ask how probable this assumption is once we observe some sample data. Before diving into learning these statistics techniques, it will be important that we discuss the null hypothesis significance testing (NHST) framework. While null hypothesis significance testing is not the only way to approach inferential statistics, it is the dominant framework and it is important that you understand how it operates. The NHST framework is not without criticism, including publicly by myself (https://www.frontiersin.org/articles/10.3389/fpsyg.2020.00815/full). A big part of this chapter is to introduce null hypothesis testing to you as a necessity, but also to make clear that you should not be basing the quality nor validity of your quantitative research on 𝑝 -values alone. To approach this, in this chapter we will discuss some of the historical developments that have lead to NHST - specifically the works of Ronald Fisher versus that of Jerzy Neyman and Egon Pearson. Modern NHST is a sort of an amalgamation of the works of these two teams and, unfortunately, it is often applied in ways that have resulted in studies of poor scientific quality. You will not need to know the history of statistics in order be a successful applied statistician, BUT, understanding the principles of Fisher’s approach versus Neyman- Pearson’s will provide you context into what NHST is and what some of its flaws are. “Significance” Many of you (all of you?) are likely to recognize the importance of the word “significance”. Significance takes on a sacred quality - many of us have been taught to think of the term “significant” as indicating that your study has found something truly important worth sharing! Results that do not meet this standard are then considered inconsequential and thrown out, never to be looked at again. I really want to challenge you to not care too much about this word. As we will discuss, a result being “significant” does not inherently mean that it is meaningful, useful, or insightful. By the same token, a result being “not significant” does not mean the result is not meaningful, useful, or insightful. Part of the goal in this chapter is to understand what “significance” means and how to think about it in your own studies. An Important Distinction: Scientific Versus Statistical Hypotheses Prior to discussing the null hypothesis framework, I first want to draw the distinction between scientific research questions and hypotheses and statistical hypotheses. While inter-related, we must understand the distinction, else we fail to adequately answer our primary research goals when employing statistical techniques. Scientific Research Questions & Scientific Hypotheses When we undertake a research study, we generally have a research question we are hoping to answer. The purpose of a scientific study is to answer your research question as best as possible. Research questions are presented in plain language, often at the end of the Introduction section of a manuscript. It is common to read a statement in research paper that reads something like: The primary objective of this research study is to address the following research question: among people who smoke cigarettes, is income level associated with likelihood of quitting cigarette use? Often times, a research question is followed by a hypothesis statement. To continue this example, the next sentence may read: We hypothesize that higher income levels will be associated with an elevated likelihood of reporting quitting cigarette use at 6-month follow-up. Assuming we are undertaking a quantitative study, we then take the data available to us and use statistical methods in an effort to best answer our research question. In this case, we need to identify a set of statistical methods that can be applied to answer our research question. The important thing in a research study is to answer our scientific research question and the results of our statistical tests must be understood as being in service to the mission to answer this question. Statistical Hypotheses: The Null and the Alternate We choose our statistical methods in order to answer our scientific research question. However, each inferential statistical method has its own set of hypotheses. The hypotheses that correspond to a statistical method always follow the same form, regardless of the scientific research question being asked. Further, making a decision about your statistical hypotheses is not the same as making a decision about your scientific hypotheses. Within the NHST framework, an inferential statistical method has one inherent hypothesis (the null) and a second that can also be specified (the alternate). We will discuss the purpose and origins of these hypotheses later on in the chapter: 𝐻0 : The null hypothesis, which states that any signal observed within a sample is the result of random chance (i.e., the signal does not exist at the population-level) 𝐻𝐴 : The alternate hypothesis, which states that the observed signal is not the result of random chance and that the signal does exist at the population-level. Within the NHST framework, this is often presented as the negation of the null hypothesis. Remember, when we take a random sample of a population, there is going to be variance (or noise) within the data. Much of the variation in our sample is simply the result of random chance - it wouldn’t be weird to see variations in our sample data from what we may expect. So, statistical tests are designed to assess how confident we are in the signal we have observed, despite the noise within the data. The larger the signal and the smaller the noise, the more confident we are that we can reject the null hypothesis. Answering Our Scientific Research Question with Our Statistical Results Often, when we write a quantitative research report, we string together multiple statistical tests, each with their own set of statistical hypotheses. We take the results of this set of statistical tests and we, as the authors, make an argument about how they answer our scientific research questions and reflect on the plausability of our initial scientific hypotheses. This was a rather long-winded way of saying that if you run a statistical test and find a “significant” result, your job as a researcher is not over. You must always seek to answer your primary research question and “significance” alone is not enough to do so. A Look At History To Understand NHST Okay! Now that that is out of the way, we need to actually discuss the purpose of the null and alternate hypotheses and how we go about evaluating them. NHST is actually a weird mixture of the methods for statistical inference developed by Ronald Fisher and that developed by Jerzy Neyman and Egon Pearson. In fact, their approaches are not compatible and which approach is better has been a topic of debate for nearly a century now! Unfortunately, hypothesis testing is often taught as a series of steps and little time is spent reflecting on what NHST is and what its flaws are. In the following sections, we will discuss the system of statistical inference developed by Fisher and that by Neyman-Pearson. This will help us understand what NHST is and how to use it effectively. Unfortunately, as a social science researcher, you will often be expected to approach science in the “normal” and “customary” ways. For decades, NHST has been misapplied to the point that the “normal” use of NHST is quite often not scientifically rigorous. This has been argued to have resulted in the “replication crisis” in the social sciences. So, first we will discuss the system developed by Fisher, which focuses solely on falsification of the null hypothesis. Then we will discuss the system developed by Neyman-Pearson, which focuses on choosing between a main and alternate hypothesis. Finally, we will discuss how NHST has attempted to marry these two approaches and how this has resulted in a mishmash of ideas that are often misunderstood and misapplied. In a sense, we must know how to navigate NHST so that we can engage with and author quantitative research, but, I want to encourage you to be critical of these practices. Falsifying the Null Hypothesis: Say Hello to the P- Value! Ronald Fisher (1890 - 1962) was a statistician who formally developed the modern idea of falsifying the null hypothesis. Fisher and other statisticians were faced with a challenging philosophical question: how do you prove that something is true (or, how do you prove your scientific hypothesis is correct)? Well, it turned out that proving something is true, especially about human behavior, is basically impossible. There are too many people and too many sources of variability to ever really establish that some “fact” is true about large populations of people (nor is it clear that all people are ruled by some unifying set of constructs that explain humanness). Fisher’s approach was an elegant response to this conundrum. Instead of trying to prove something is true or false, we can actually assume that something is true and then make observations about the world through this assumption. Does what we are observing make sense with our assumption - or, how likely are our observations given our assumption? This is where the idea of the null hypothesis was born. If we want to try to show that some signal exists, Fisher’s method suggests that we assume that (𝐻0 ) a signal does not exist. Then we observe data and ask how likely the observed data if we assume no signal exists, or: 𝑃 (𝑑𝑎𝑡𝑎|𝐻0 ) In other words, what is the probability of observing our sample data, assuming that the null hypothesis is true that no signal exists. If this value approaches 0, we are suggesting that there is a very low probability we could observe our data if the null hypothesis were true. The closer to 0 this value becomes, the more confident we can feel that our assumption of 𝐻0 is wrong. In otherwords, the closer to 0 this value becomes, the more confident we can feel that a signal actually exists at the population level. What does “probability of observing our sample data” mean, though? While we may assume that no signal exists at the population-level (𝐻0 ), we know it is quite unlikely that we won’t observe any signal at all in our sample data. It turns out that when we assume 𝐻0 , we are also assuming that our observations will follow specific patterns, otherwise known as theoretical probability distributions. For example, if I want to run a statistical test to compare anxiety between undergraduate students (𝜇𝑢𝑛𝑑𝑒𝑟 ) and PhD students (𝜇𝑃ℎ𝐷 ), I would start by assuming that 𝐻0 : 𝜇𝑢𝑛𝑑𝑒𝑟 = 𝜇𝑃ℎ𝐷. Now if I recruit 100 undergrads and 100 PhD students and calculate mean anxiety scores 𝑥¯𝑢𝑛𝑑𝑒𝑟 and 𝑥¯𝑃ℎ𝐷 , it seems fairly likely that these values will be slightly different…what we want to know is how likely is what we observe assuming 𝐻0. So, we calculate our signal, in this case we can consider the difference between anxiety scores for the two groups is our signal (i.e., 𝑥¯𝑢𝑛𝑑𝑒𝑟 − 𝑥¯𝑃ℎ𝐷 ). If the null hypothesis is true, it is fairly likely this value will be close to 0! In fact, 0 seems like the most likely value, but values close to 0 also seem fairly likely. Values farther from 0 seem much less likely. This sounds a lot like the definition of a normal distribution! Interesting! In this case, by assuming 𝐻0 , that no signal exists, we are also making an assumption of how our observed variable will behave - in this case that the difference between group anxiety scores should follow a normal distribution. We can then use our signal (i.e., 𝑥¯𝑢𝑛𝑑𝑒𝑟 − 𝑥¯𝑃ℎ𝐷 ) and our noise (here, it may be the standard deviation of anxiety observations) to calculate a test statistic. A test statistic 𝑡 represents a value calculated from the 𝑠𝑖𝑔𝑛𝑎𝑙 and 𝑛𝑜𝑖𝑠𝑒 that corresponds to a standard probability distribution, 𝑇. Once we calculate 𝑡 , we can then ask what the probability is of observing a value of 𝑡 or a greater value, by calculating the corresponding area under the curve from 𝑇. We can denote this probability as: 𝑃 (𝑇 ≥ 𝑡|𝐻0 ) In other words, what is the probability of observing a test statistic 𝑡 from distribution 𝑇 , assuming that 𝐻0 is true! In this case, 𝑡 is a standardized measure of the difference between the two groups’ mean anxiety scores and 𝑇 is the standard normal distribution. We call this value the p-value. The p-value represents the probability of observing a signal of a given strength or stronger, assuming that (𝐻0 ) no signal actually exists. If the p-value is quite small, then that indicates to us that our assumption is probably wrong and that it is quite likely that a signal exists. Notice, this doesn’t prove that 𝐻0 is false, it is just a way of reflecting that it is highly improbable. The term significant is then applied to p-values with values less than some pre-established threshold 𝛼. 𝛼 is typically set to 0.05 in most research practices. Fisher importantly argued that “no isolated experiment, however significant in itself, can suffice for the experimental demonstration of any natural phenomenon.” In other words, a study with significant findings should not be viewed as sufficient alone to determine what is factual. A P-Value is Not… Now, if this description has been confusing, that’s ok. P-values are notoriously confusing and challenging to define in lay terms. As we learn about more statistical tests and how to apply them, we will learn how the p- value is calculated and how it is to be interpreted. The important thing to takeaway is that the p-value represent the probability of observing your data assuming the null hypothesis is true. The smaller a p- value, the less likely we think our data could have happened assuming the null hypothesis is true - as a result, since we a certain in our data (i.e., since its real and not theoretical/assumed), this forces us to question the validity of assuming the null hypothesis. Unfortunately, it is easy to misinterpret a p-value or misunderstand what it is. Cyril Pernet put together a nice list (https://dx.doi.org/10.12688%2Ff1000research.6963.3) of things that a p-value is not: A p-value is not a measure of the strength or magnitude of a signal (i.e., a small p-value does not mean the signal is strong or meaningful) A p-value does not reflect the probability of replicating the signal observed (i.e., getting a small p-value doesn’t mean replication studies are likely to get the same result) A small p-value is not evidence of any alternate hypothesis, it is only a reflection of the relationship between the data and the null The p-value is not the probability that the null hypothesis is true This last one is quite important because one of the biggest mistakes that researchers make is that they interpret the 𝑝 -value to represent how likely it is that the null hypothesis is true, or: 𝑃 (𝐻0 |𝑑𝑎𝑡𝑎). As we have defined, though, the 𝑝 -value represents the probability of observing our data assuming that the null hypothesis is true, or: 𝑃 (𝑑𝑎𝑡𝑎|𝐻0 ). An Interesting Note About This ow, this is a bit of a downer, because, as researchers we are actually far more interested in 𝑃 (𝐻0 |𝑑𝑎𝑡𝑎) than we are in the 𝑝 -value, 𝑃 (𝑑𝑎𝑡𝑎|𝐻0 ). There is a cool formula called Bayes’ Rule that tells us that 𝑃 (𝐻0 |𝑑𝑎𝑡𝑎) and 𝑃 (𝑑𝑎𝑡𝑎|𝐻0 ) are proportional to one another. If one value goes up, so does the other and vice versa. As such, we may understand our 𝑝 -value (𝑃 (𝑑𝑎𝑡𝑎|𝐻0 ) ) being a proxy for the value we actually care about, which is the probability of the hypothesis itself (𝑃 (𝐻0 |𝑑𝑎𝑡𝑎) ). So, this is quite interesting. As scientists, we want to know if our hypothesis is valid or not. But, the 𝑝 -value, one of the most prominent metrics in statistics, is about the probability of our data assuming our hypothesis is true. While these ideas are related, it is important to take note that, using Fisher’s approach we are actually not reflecting directly on the validity of our statistical hypothesis. We have a proxy measure (the 𝑝 -value) which we understand to be inter-related. This issue is the same for the Neyman-Pearson approach we will discuss later! Let’s Do an Example: A P-Value Primer - Big Babies Let’s now run through an example study to get a sense of the general process we undertake when we calculate a p-value. So, we are researching the birth weight of newborn babies in a small town. We had heard some rumors that babies born in this town are all really big! Doctors joke there must be something in the water. So, we want to know, are the babies born in this town actually bigger than normal babies. We happen to know that the weight of newborn babies is normally distributed, that the average weight of a newborn baby is 𝜇 = 7.5 pounds, and that the standard deviation of birth weights is 𝜎 = 1.2 pounds. We start by setting our null hypothesis. Since the null is the assumption that no signal exists, the null would be that birth weights of babies in this small town are the same as babies generally. If we let 𝜇𝑆𝑇 be the weight of newborn babies in this small town, then our null hypothesis would be: 𝐻0 : 𝜇𝑆𝑇 = 𝜇 This is actually the same, mathematically, as saying that: 𝐻0 : 𝜇𝑆𝑇 − 𝜇 = 0 This is quite useful because the difference between the average baby weight in the small town and the average baby weight generally represents our signal. So, let’s say we got birth records from a sample of babies in this small town and we calculated that the average birth weight of the sample of babies is 𝑥¯. If we assume that 𝐻0 is true, then it makes sense that the value of 𝑥¯ − 𝜇 would be normally distributed around the value 0. This is because if we take a random sample of babies from this small town and calculate their average weight 𝑥¯ it is quite probable that 𝑥¯ ≠ 𝜇 because there is always going to be random noise in the data, even if we are assuming that 𝜇𝑆𝑇 = 𝜇. However, if 𝐻0 is actually true, then we can understand that values of 𝑥¯ − 𝜇 close to 0 are more probable than values further from 0. Further, it seems likely that if 𝐻0 were true, that 𝑥¯ has the same probability of being less than 𝜇 as being greater than 𝜇. In other words, it appears that if 𝐻0 is true, that 𝑥¯ − 𝜇 is normally distributed around 0! Last week, we discussed the 𝑍 -distribution (or the standard normal distribution). Further, we talked about how any normal distribution can be “standardized” by dividing the values by the standard deviation. We can actually transform our value 𝑥¯ − 𝜇 so that it corresponds to the 𝑍 -distribution. We use the following equation to do so: 𝑥¯ − 𝜇 𝑧= 𝜎 √𝑛 So, now we have this value 𝑧. This is our test statistic. 𝑧 has several cool properties: 1) it captures the strength of the signal in the dataset, 2) it captures the noise in the dataset, and 3) it corresponds to a 𝑍 - distribution which captures the behavior of the signal assuming that 𝐻0 were true. Now, all we need to do is calculate 𝑃 (𝑍 >= 𝑧|𝐻0 ). So, let’s throw some numbers in. We got the weight of 𝑛 = 16 babies and found that their average weight 𝑥¯ = 8.1 pounds. So we can calculate 𝑧 as follows: 8.1 − 7.5 0.6 𝑧= = =2 1.2 0.3 √16 So, now we can map the value 𝑧 = 2 onto the 𝑧 -distribution like so: x