Module 2.2 Data Analysis PDF
Document Details
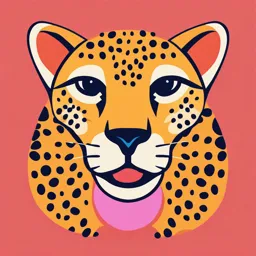
Uploaded by WellConnectedPanPipes
Tags
Summary
This document discusses descriptive and inferential statistics, including measures of central tendency, variability, and skewness. It also examines the concept of distributions using examples.
Full Transcript
MO DULE 2.2 Data Analysis Descriptive and Inferential Statistics Descriptive Statistics descriptive statistics Sta- In our discussion of research, we have considered...
MO DULE 2.2 Data Analysis Descriptive and Inferential Statistics Descriptive Statistics descriptive statistics Sta- In our discussion of research, we have considered two issues thus far: how to design a tistics that summarize, study to collect data and how to collect those data. Assuming we have been successful organize, and describe a at both of those tasks, we now need to analyze those data to determine what they may sample of data. tell us about our initial theory, hypothesis, or speculation. We can analyze the data measure of central we have gathered for two purposes. The first is simply to describe the distribution of tendency Statistic that scores or numbers we have collected. A distribution of numbers simply means that the indicates where the center numbers are arrayed along two axes. The horizontal axis is the score or number axis of a distribution is located. running from low to high scores. The vertical axis is usually the frequency axis, which Mean, median, and mode indicates how many individuals achieved each score on the horizontal axis. The statisti- are measures of cen- cal methods to accomplish such a description are referred to as descriptive statistics. tral tendency. You have probably encountered this type of statistical analysis in other courses, so we variability The extent to will simply summarize the more important characteristics for you. Consider the two which scores in a distri- distributions of test scores in Figure 2.2. Look at the overall shapes of those distributions. bution vary. One distribution is high and narrow; the other is lower and wider. In the left graph, skew The extent to which the distribution’s center (48) is easy to determine; in the right graph, the distribution’s scores in a distribution are center is not as clear unless we specify the central tendency measure of interest. One lopsided or tend to fall on distribution is bell shaped or symmetric, while the other is lopsided. Three measures the left or right side of the or characteristics can be used to describe any score distribution: measures of central distribution. tendency, variability, and skew. Positive skew means that the scores or observations are bunched at the bottom of the score range; negative skew means that scores or observa- mean The arithmetic tions are bunched at the top of the score range. As examples, if the next test you take average of the scores in a distribution; obtained by in this course is very easy, there will be a negative skew to score distribution; if the test is summing all of the scores very hard, the scores are likely to be positively skewed. in a distribution and divid- Measures of central tendency include the mean, the mode, and the median. The ing by the sample size. mean is the arithmetic average of the scores, the mode is the most frequently occur- ring score, and the median is the middle score (the score that 50 percent of the mode The most common remaining scores fall above and the other 50 percent of the remaining scores fall or frequently occurring below). As you can see, the two distributions in Figure 2.2 have different means, score in a distribution. modes, and medians. In addition, the two distributions vary on their lopsidedness, median The middle score or skewness. The left distribution has no skew; the right distribution is positively in a distribution. skewed, with some high scores pulling the mean to the positive (right) side. 62 Descriptive and Inferential Statistics 63 10 10 9 Mean, Median, Mode 9 Mode 8 8 Median 7 7 Mean 6 6 Frequency Frequency 5 5 4 4 3 3 2 2 1 1 0 0 0 48 100 0 45 55 59 100 Test Scores Test Scores FIGURE 2.2 Two Score Distributions (N = 30) 10 10 9 9 8 8 7 7 6 6 Frequency Frequency 5 5 4 4 Mean 3 3 2 2 1 1 0 0 0 48 100 0 50 59 100 Test Scores Test Scores FIGURE 2.3 Two Score Distributions (N = 10) Another common descriptive statistic is the standard deviation, or the variance of a distribution. In Figure 2.3, you can see that one distribution covers a larger score range and is wider than the other. We can characterize a distribution by looking at the extent to which the scores deviate from the mean score. The typical amount of deviation from a mean score is the standard deviation. Since distributions often vary from each other simply as a result of the units of measure (e.g., one distribution is a measure of inches, while another is a measure of loudness), sometimes it is desir- able to standardize the distributions so that they all have means of.00 and standard (or average) deviations of 1.00. The variance of a distribution is simply the squared standard deviation. 64 Chapter 2 Research Methods and Statistics in I-O Psychology Inferential Statistics In the studies that you will encounter in the rest of this text, the types of analyses used are not descriptive, but inferential. When we conduct a research study, we do it for a reason. We have a theory or hypothesis to examine. It may be a hypothesis that accidents are related to personality characteristics, or that people with higher scores on a test of mental ability perform their jobs better than those with lower scores, or that team members in small teams are happier with their work than team members in large teams. In each of these cases, we design a study and collect data in order to come to some conclusion, to draw an inference about a relationship. In research, we use findings from the sample we collected to make inferences to a larger popula- tion. Once again, in other courses, you have likely been introduced to some basic inferential statistics Sta- inferential statistics. Statistical tests such as the t-test, analysis of variance or F-test, tistics used to aid the or chi-square test can be used to see whether two or more groups of participants researcher in testing (e.g., an experimental and a control group) tend to differ on some variable of inter- hypotheses and making est. For example, we can examine the means of the two groups of scores in Figure 2.3 inferences from sample to see if they are different beyond what we might expect as a result of chance. If I tell data to a larger sample or you that the group with the lower mean score represents high school graduates and population. the group with the higher mean score represents college graduates, and I further tell you that the means are statistically significantly different from what would be found with simple random or chance variation, you might draw the inference that educa- tion is associated with higher test scores. The statistical test used to support that con- clusion (e.g., a t-test of mean differences) would be considered an inferential test. Box 2.1 provides a sampling of thoughts on statistics and data analysis. Statistical Significance Two scores, derived from two different groups, might be different, even at the third decimal place. How can we be sure that the difference is a “real” one—that it exceeds a difference we might expect as a function of chance alone? If we examined the Box 2.1 | A Sampling of Thoughts on Statistics and Data It is easy to lie with statistics, but it is even easier Without data, you’re just another person with to lie without them—statistician Frederick an opinion—W. Edwards Deming (1900–1993), Mosteller (1916–2006). American statistician, professor, and author If it isn’t scientific, it’s not good practice, In God we trust; all others must bring data— and if it isn’t practical, it’s not good science— W. Edwards Deming (1900–1993), American Morris Viteles (1898–1996), author of early I-O statistician, professor, and author psychology textbook If we have data, let’s look at data. If all we There are 3 kinds of lies: lies, damned lies, and have are opinions, let’s go with mine—Jim statistics—Benjamin Disraeli (1804–1881), former Barksdale (1943- ), American business executive British Prime Minister at Netscape Statistical thinking will one day be as Torture the data, and it will confess to necessary for efficient citizenship as the ability to anything—Ronald Coase (1910–2013), British read and write—H. G. Wells (1866–1946), English economist, author, and Nobel Prize winner writer and historian Descriptive and Inferential Statistics 65 mean scores of many different test groups, such as the two displayed in Figure 2.3, we would almost Mick Stevens/The New Yorker Collection/The Cartoon Bank never find that the means were exactly the same. A convention has been adopted to define when a difference or an inferential statistic is signifi- cant. Statistical significance is defined in terms of a probability statement. To say that a finding of difference between two groups is significant at the 5 percent level, or a probability of.05, is to say that a difference that large would be expected to occur only 5 times out of 100 as a result of chance alone. If the difference between the means was even larger, we might conclude that a difference this large might be expected to occur only 1 time out of 100 as a result of chance alone. This latter result would be reported as a difference at the 1 percent level, or a probability of.01. As the probability goes down (e.g., from.05 to.01), we become more confident that the difference is a real difference. It is impor- tant to keep in mind that the significance level addresses only the confidence that statistical significance we can have that a result is not due to chance. It says nothing about the strength of Indicates that the prob- an association or the practical importance of the result. The standard, or threshold, ability of the observed for significance has been set at.05 or lower as a rule of thumb. Thus, unless a result statistic is less than the would occur only 5 or fewer times out of 100 as a result of chance alone, we do not stated significance level adopted by the researcher label the difference as statistically significant. (commonly p <.05). A sta- tistically significant finding The Concept of Statistical Power indicates that the results found are unlikely to have Many studies have a very small number of participants in them. This makes it very occurred by chance, and difficult to find statistical significance even when there is a “true” relationship thus the null hypothesis (i.e., hypothesis of no among variables. In Figure 2.3, we have reduced our two samples in Figure 2.2 effect) is rejected. from 30 to 10 by randomly dropping 20 participants from each group. The dif- ferences are no longer statistically significant. But from our original study with 30 participants, we know that the differences between means are not due to chance. Nevertheless, the convention we have adopted for defining significance prevents us from considering the new difference to be significant, even though the mean values and the differences between those means are identical to what they were in Figure 2.2. The concept of statistical power deals with the likelihood of finding a statistically statistical power The significant difference when a true difference exists. The smaller the sample size, likelihood of finding a the lower the power to detect a true or real difference. In practice, this means that statistically significant researchers may be drawing the wrong inferences (e.g., that there is no association) difference when a true when sample sizes are too small. The issue of power is often used by the critics of difference exists. significance testing to illustrate what is wrong with such conventions. Schmidt and Hunter (2002b) argued that the typical power of a psychological study is low enough that more than 50 percent of the studies in the literature do not detect a difference between groups or the effect of an independent variable on a dependent variable when one exists. Thus, adopting a convention that requires an effect to be “statisti- cally significant” at the.05 level greatly distorts what we read in journals and how we interpret what we do read. Power calculations can be done before a study is ever initiated, informing the researcher of the number of participants that should be included in the study in 66 Chapter 2 Research Methods and Statistics in I-O Psychology order to have a reasonable chance of detecting an association (Cohen, 1988, 1994; Murphy & Myors, 2004). Research studies can be time-consuming and expensive. It would be silly to conduct a study that could not detect an association even if one were there. The power concept also provides a warning against casually dismissing studies that do not achieve “statistical significance” before looking at sample sizes. If the sample sizes are small, we may never know whether or not there is a real effect or difference between groups. Correlation and Regression As we saw in the discussion about research design, there are many situations in which experiments are not feasible. This is particularly true in I-O psychology. It would be unethical, for example, to manipulate a variable that would influence well-being at work, with some conditions expected to reduce well-being and others to enhance measurement Assigning numbers to characteristics well-being. The most common form of research is to observe and measure natural of individuals or objects variation in the variables of interest and look for associations among those variables. according to rules. Through the process of measurement, we can assign numbers to individuals. These numbers represent the person’s standing on a variable of interest. Examples of these correlation coefficient numbers are a test score, an index of stress or job satisfaction, a performance rating, Statistic assessing the or a grade in a training program. We may wish to examine the relationship between bivariate, linear associa- two of these variables to predict one variable from the other. For example, if we are tion between two variables. Provides information interested in the association between an individual’s cognitive ability and training about both the magnitude success, we can calculate the association between those two variables for a group of (numerical value) and the participants. If the association is statistically significant, then we can predict training direction (1 or 2) of the success from cognitive ability. The stronger the association between the two variables, relationship between two the better the prediction we are able to make from one variable to another. The sta- variables. tistic or measure of association most commonly used is the correlation coefficient. The Concept of Correlation The best way to appreciate the concept of correlation is graphically. Examine the hypothetical data in Figure 2.4. The vertical axis of that figure represents training grades. The horizontal axis represents a score on a test of cognitive ability. For both scatterplot Graph used to axes, higher numbers represent higher scores. This graph is called a scatterplot plot the scatter of scores because it plots the scatter of the scores. Each dot represents the two scores achieved on two variables; used to by an individual. The 40 dots represent 40 people. Notice the association between test display the correlational scores and training grades. As test scores increase, training grades tend to increase as relationship between two well. In high school algebra, this association would have been noted as the slope, or variables. “rise over run,” meaning how much rise (increase on the vertical axis) is associated with one unit of run (increase on the horizontal axis). In statistics, the name for this regression line Straight form of association is correlation, and the index of correlation or association is called line that best “fits” the the correlation coefficient. You will also notice that there is a solid straight line that scatterplot and describes goes through the scatterplot. This line (technically known as the regression line) is the relationship between the straight line that best “fits” the scatterplot. The line can also be presented as an the variables in the graph; equation that specifies where the line intersects the vertical axis and what the slope can also be presented as an equation that specifies of the line is. where the line intersects As you can see from Figure 2.4, the actual slope of the line that depicts the asso- the vertical axis and ciation is influenced by the units of measurement. If we plotted training grades what the angle or slope against years of formal education, the slope of the line might look quite different, as of the line is. is depicted in Figure 2.5, where the slope of the line is much less steep or severe. For Correlation and Regression 67 practical purposes, the regression line can be quite use- 100 ful. It can be used to predict what value on the Y variable (in Figure 2.4, training grades) might be expected for someone with a particular score on the X variable (here, 75 cognitive test scores). Using the scatterplot that appears 60 Training Grade in Figure 2.4, we might predict that an individual who achieved a test score of 75 could be expected to also get 50 a training grade of 75 percent. We might use that predic- tion to make decisions about whom to enroll in a training program. Since we would not want to enroll someone who 25 might be expected to fail the training program (in our case, receive a training grade of less than 60 percent), we might limit enrollment to only those applicants who 0 0 25 50 54 75 100 achieve a score of 54 or better on the cognitive ability test. Cognitive Test Score FIGURE 2.4 Scatterplot of Test Scores and Training Grades The Correlation Coefficient 100 For ease of communication and for purposes of further analysis, the correlation coefficient is calculated in such 75 a way that it always permits the same inference, regard- less of the variables that are used. Its absolute value Training Grade 50 will always range between.00 and 1.00. A high value (e.g.,.85) represents a strong association, and a lower 25 value (e.g.,.15) represents a weaker association. A value of.00 means that there is no association between two 0 variables. Generally speaking, in I-O psychology, cor- 10 11 12 13 14 15 16 17+ relations in the range of.10 are considered close to Years of Education trivial, while correlations of.40 or above are considered FIGURE 2.5 Scatterplot of Years of Education and Training Grades substantial. Correlation coefficients have two distinct parts. The first part is the actual value or magnitude of the correlation (ranging from.00 to 1.00). The second part is the sign (+ or −) that precedes the numerical value. A positive (+) correlation means that there is a positive association between the variables. In our examples, as test scores and years of education go up, so do training grades. A negative (−) corre- lation means that as one variable goes up, the other variable tends to go down. An example of a negative correlation would be the association between age and visual acuity. As people get older, their uncorrected vision tends to get worse. In I-O psychology, we often find negative correlations between measures of commit- ment and absence from work. As commitment goes up, absence tends to go down, and vice versa. Figure 2.6 presents examples of the scatterplots that represent various degrees of positive and negative correlation. You will notice that we have again drawn straight lines to indicate the best-fit straight line that represents the data points. By examining the scatterplots and the corresponding regression lines, you will notice something else about correlation. As the data points more closely approach the straight line, the correlation coefficients get higher. If all of the data points fell exactly on the line, the correlation coefficient would be 1.00 and there would be a “perfect” correlation between the two variables. We would be able to perfectly pre- dict one variable from another. As the data points depart more from the straight line, the correlation coefficient gets lower until it reaches.00, indicating no relationship at all between the two variables. 68 Chapter 2 Research Methods and Statistics in I-O Psychology Y Y High High rxy = +.95 rxy = –.95 Low X Low X Low High Low High Y Y Y High High High rxy =.00 rxy = +.50 rxy = –.50 Low X Low X Low X Low High Low High Low High FIGURE 2.6 Scatterplots Representing Various Degrees of Correlation linear Relationship Up to this point, we have been assuming that the relationship between two between two variables variables is linear (i.e., it can be depicted by a straight line). But the relation- that can be depicted by a ship might be nonlinear (sometimes called “curvilinear”). Consider the scatter- straight line. plot depicted in Figure 2.7. In this case, a straight line does not represent the nonlinear Relationship shape of the scatterplot at all. But a curved line does an excellent job. In this case, between two variables that although the correlation coefficient might be.00, one cannot conclude that there cannot be depicted by a is no association between the variables. We can conclude only that there is no linear straight line; sometimes association. called “curvilinear” and In this figure, we have identified the two variables in question as “stimulation” most easily identified by and “performance.” This scatterplot would tell us that stimulation and performance examining a scatterplot. are related to each other, but in a unique way. Up to a point, stimulation aids in successful performance by keeping the employee alert, High awake, and engaged. But beyond that point, stimulation makes performance more difficult by turning into infor- mation overload, which makes it difficult to keep track of relevant information and to choose appropriate actions. Performance Most statistics texts that deal with correlation offer Average detailed descriptions of the methods for calculating the strength of a nonlinear correlation or association. But for the purposes of the present discussion, you merely need to know that one of the best ways to detect non- Low linear relationships is to look at the scatterplots. As in Low Average High Figure 2.7, this nonlinear trend will be very apparent if it Stimulation is a strong one. In I-O psychology, many, if not most, of FIGURE 2.7 An Example of a Curvilinear Relationship the associations that interest us are linear. Correlation and Causation 69 Multiple Correlation As we will see in later chapters, there are many situations in which more than one variable is associated with a particular aspect of behavior. For example, you will see that although cognitive ability is an important predictor of job performance, it is not the only predictor. Other variables that might play a role are personality, experi- ence, and motivation. If we were trying to predict job performance, we would want to examine the correlation between performance and all of those variables simul- taneously, allowing for the fact that each variable might make an independent con- tribution to understanding job performance. Statistically, we could accomplish this through an analysis known as multiple correlation. The multiple correlation coef- multiple correlation ficient would represent the overall linear association between several variables (e.g., coefficient Statistic that cognitive ability, personality, experience, motivation) on the one hand and a single represents the overall variable (e.g., job performance) on the other hand. As you can imagine, these cal- linear association between culations are so complex that their study is appropriate for an advanced course several variables (e.g., cog- nitive ability, personality, in prediction or statistics. For our purposes in this text, you will simply want to be experience) on the one aware that techniques are available for examining relationships involving multiple hand and a single variable predictor variables. (e.g., job performance) on the other hand. Correlation and Causation Correlation coefficients simply represent the extent to which two variables are asso- ciated. They do not signal any cause–effect relationship. Consider the example of height and weight. They are positively correlated. The taller you are, the heavier you tend to be. But you would hardly conclude that weight causes height. If that were the case, we could all be as tall as we wish simply by gaining weight. Box 2.2 provides a cautionary note on making conclusions about causation. In an earlier section of this chapter that dealt with the context of research results, we described the anomalous finding that better-functioning medical teams appeared to be associated with more medical errors. Would it make sense, then, to retain only poorer-functioning teams? Similarly, we gave the example of less friendly sales per- sonnel in convenience stores being associated with higher sales. Would it make sense Box 2.2 | Experimental Design and Causation It is not always easy to separate causes and youngest group) and heard a heavy Italian effects. The experimental design that you use accent. The researcher concluded that as you often determines what conclusions you can grow older, you develop an Italian accent. It is a draw. A story is told of the researcher who safe bet that had the researcher studied a group interviewed the inhabitants of a particular of people as they aged, he would have come neighborhood. He noted that the young people to a very different conclusion, perhaps even an spoke fluent English. In speaking with the opposite one. middle-aged people who would be the parent Source: Adapted from Charness, N. (Ed.). (1985). generation of the younger people, he found that Aging and human performance, p. xvii. New York: they spoke English with a slight Italian accent. John Wiley & Sons. Reproduced by permission of John Finally, he spoke with older people (who would Wiley & Sons. represent the grandparent generation of the 70 Chapter 2 Research Methods and Statistics in I-O Psychology to fire pleasant sales reps? In both cases, it was eventually discovered that a third variable intervened to help us understand the surprising correlation. It became clear that the initial association uncovered was not a causal one. The question of correlation and causality has an important bearing on many of the topics we will consider in this book. For example, there are many studies that show a positive correlation between the extent to which a leader acts in a considerate manner and the satisfaction of the subordinates of that leader. Because of this corre- lation, we might be tempted to conclude that consideration causes satisfaction. But we might be wrong. Consider two possible alternative explanations for the positive correlation: 1. Do we know that considerate behavior on the part of a business leader causes worker satisfaction rather than the other way around? It is possible that satisfied subordinates actually elicit considerate behavior on the part of a leader (and conversely, that a leader might “crack down” on dissatisfied work group members). 2. Can we be sure that the positive correlation is not due to a third variable? What if work group productivity was high because of a particularly able and motivated group? High levels of productivity are likely to be associated with satisfaction in workers, and high levels of productivity are likely to allow a leader to concentrate on considerate behaviors instead of pressuring workers for higher production. Thus, a third variable might actually be responsible for the positive correlation between two other variables. Big Data Big Data is a term that describes using large data sets to examine relationships among variables and to make organizational decisions based on such data. Big Data has been on the list of SIOP’s Top 10 Workplace Trends for every year from 2014 to 2018. Even though this Big Data trend has become quite popular in recent years in I-O psychology and many other domains, I-O psychologists have been using large data sets to make informed organizational decisions for many decades (Guzzo, Fink, King, Tonidandel, & Landis, 2015). In other domains, big data and data science have received a great deal of attention for their use in making predictions about politi- cal elections (e.g., Silver, 2012). In the sports world, the use of statistics and analyt- ics in making organizational decisions was described and popularized in the book Moneyball by Michael Lewis (2003) and later showcased by the film (2011) of the same name. In the film, Brad Pitt portrays Oakland A’s general manager Billy Beane who used analytics and big data to help his low payroll baseball team identify and acquire undervalued players and compete with much higher payroll teams. A recent book in the SIOP Organizational Frontiers Series is entitled Big data at work: The data science revolution and organizational psychology. This book shows “how advances in data science have the ability to fundamentally influence and improve organizational science and practice” (Tonidandel, King, & Cortina, 2015). Based on their strong statistics background and training, I-O psychologists are well-prepared to help companies make good decisions with their large data sets. In addition, I-O psychologists are well-prepared to help companies avoid making mistakes with their data. Marcus and Davis (2014) discuss how some companies are making broad pre- dictions from their big data sets, but many of these predictions need to be viewed with skepticism. As I-O psychologists have noted for a long time, large data sets are good at identifying significant correlations, but such data sets don’t indicate which Meta-Analysis 71 ones are meaningful or important. After reading predictions and proclamations of proponents of Big Data, Marcus and Davis sarcastically noted that “correlations never sounded so good” (recall the previous section’s discussion about correlation and causation). This Big Data trend (which goes by several names including Pre- dictive Analytics and Data Science) aligns well with evidence-based I-O psychology, which involves making organizational decisions using data and which was discussed in Chapter 1. Finally, an article in Harvard Business Review called data scientist “the sexiest job of the 21st Century” (Davenport & Patil, 2012). Of course, one needs to be at least a little skeptical of this proclamation given that both authors are data sci- entists, but nevertheless, they make a good case that those who are expert at working with big data will be in great demand over the coming years. Meta-Analysis Cancer researchers, clinicians, and patient advocates have engaged in a vigorous debate about whether women aged 40 to 70 can decrease their chances of dying from breast cancer by having an annual mammogram. One expert asserts that the earlier cancer can be detected, the greater the chance of a cure, and that an annual mammogram is the only reliable means of early detection. Another argues that this is not necessarily true and, furthermore, because mammograms deliver potentially harmful radiation, they should be used only every two or three years unless a patient has significant risk factors for the disease. Still another says that mammograms give a false sense of security and may discourage patients from monitoring their own health. Experts on all sides cite multiple studies to support their position. And women are left with an agonizing dilemma: Who is right? What is the “truth”? Similar confusion exists over the interpretation of study results in psychology topics. You may find hundreds of studies on the same topic. Each study is done with a different sample, a different sample size, and a different observational or experi- mental environment. It is not uncommon for individual studies to come to different conclusions. For example, one study of the relationship between age and job satis- faction may have administered a locally developed satisfaction questionnaire to 96 engineers between the ages of 45 and 57 who were employed by Company X. The study might have found a very slight positive correlation (e.g., +.12) between age and meta-analysis Statistical satisfaction. Another study might have distributed a commercially available satisfac- method for combining tion questionnaire to 855 managerial-level employees between the ages of 27 and 64 and analyzing the results who worked for Company Y. The second study might have concluded that there was from many studies to a strong positive correlation (e.g., +.56) between age and satisfaction. A third study of draw a general conclusion 44 outside sales representatives for Company Z between the ages of 22 and 37 using about relationships among the same commercially available satisfaction questionnaire might have found a slight variables. negative correlation between age and satisfaction (e.g., −.15). Which study is “right”? How can we choose among them? statistical artifacts Charac- Meta-analysis is a statistical method for combining results from many studies to teristics (e.g., small sample draw a general conclusion (Ones, Viswesvaran, & Schmidt, 2017; Schmidt & Hunter, size, unreliable measures) of a particular study that 2002a). Meta-analysis is based on the premise that observed values (like the three distort the observed correlations shown above) are influenced by statistical artifacts (characteristics of results. Researchers can the particular study that distort the results). The most influential of these artifacts is correct for artifacts to sample size. Others include the spread of scores and the reliability of the measures arrive at a statistic that rep- used (“reliability” is a technical term that refers to the consistency or repeatability resents the “true” relation- of a measurement; we will discuss it in the next module of this chapter). Consider ship between the variables the three hypothetical studies we presented above. One had a sample size of 96, the of interest. 72 Chapter 2 Research Methods and Statistics in I-O Psychology second of 855, and the third of 44. Consider also the range of scores on age for the three studies. The first had an age range from 45 to 57 (12 years). The second study had participants who ranged in age from 27 to 64 (37 years). The participants in the third study ranged from 22 to 37 years of age (15 years, with no “older” employees). Finally, two of the studies used commercially available satisfaction questionnaires, which very likely had high reliability, and the third study used a “locally developed” questionnaire, which may have been less reliable. Using these three studies as exam- ples, we would probably have greater confidence in the study with 855 participants, with an age range of 37 years that used a more reliable questionnaire. Neverthe- less, the other studies tell us something. We’re just not sure what that something is because of the influences of the restricted age ranges, the sample sizes, and the reli- abilities of the questionnaires. In its most basic form, meta-analysis is a complex statistical procedure that includes information about these statistical artifacts (sample size, reliability, and range restric- tion) and corrects for their influences, producing an estimate of what the actual relationship is across the studies available. The results of a meta-analysis can provide accurate estimates (i.e., population estimates) of the relationships among constructs (e.g., intelligence, job performance) in the meta-analysis, and these estimates do not rely on significance tests. In addition, it is possible to consider variables beyond these statistical artifacts that might also influence results. A good example of such a variable is the nature of the participants in the study. Some studies might conclude that racial or gender stereotypes influence performance ratings, while other studies conclude that there are no such effects. If we separate the studies into those done with student participants and those done with employees of companies, we might discover that stereotypes have a strong influence on student ratings of hypotheti- cal subordinates but have no influence on the ratings of real subordinates by real supervisors. Meta-analysis can be a very powerful research tool. It combines individual studies that have already been completed and, by virtue of the number and diversity of these studies, has the potential to “liberate” conclusions that were obscure or confusing at the level of the individual study. Meta-analyses are appearing with great regu- larity in I-O journals and represent a real step forward in I-O research. The actual statistical issues involved in meta-analysis are incredibly complex, and they are well beyond what you need to know for this course. Nevertheless, because meta-analysis is becoming so common, you at least need to be familiar with the term. As an example, we will examine the application of meta-analysis to the relationship between tests and job performance in Chapter 3. Micro-, Macro-, and Meso-Research In the same spirit in which we introduced you to the term meta-analysis, we need to prepare you for several other terms you may encounter while reading the research literature, particularly the literature associated with organizational topics in the last few chapters of this book. Over the 100-plus years of the development of I-O psy- chology as a science and area of practice, there has been an evolution of areas of interest from individual differences characteristics to much broader issues related to teams, groups, and entire organizations. In later chapters, you will encounter topics such as team training, group cohesiveness, and organizational culture and climate. In our discussion of Hofstede (2001), Chao and Moon (2005), and others in Chapter 1, we have already introduced you to a very broad level of influence Micro-, Macro-, and Meso-Research 73 called national culture. As a way of characterizing the research focus of those who are more interested in individual behavior as opposed to those more interested in the behavior of collections of individuals (e.g., teams, departments, organiza- tions), the terms micro-research and macro-research were introduced, with micro micro-research The study being applied to individual behavior and macro being applied to collective behavior of individual behavior. (Smith, Schneider, & Dickson, 2005). But it is obvious that even individual behavior macro-research The study (e.g., job satisfaction) can be influenced by collective variables (e.g., group or team of collective behavior. cohesion, reputation of the employer, an organizational culture of openness). As a result, a third term—meso-research (meso literally means “middle” or “between”)— meso-research The was introduced to both describe and encourage research intended to integrate study of the interaction micro- and macro-studies (Buckley, Riaz Hamdani, Klotz, & Valcea, 2011; Rousseau & of individual and collec- House, 1994). tive behavior. In practice, meso-research is accomplished by including both individual differ- ences data (e.g., cognitive ability test scores) and collective data (the technological emphasis of the company, the team culture, etc.) in the same analysis. This type of analysis, known as multi-level or cross-level analysis (Klein & Kozlowski, 2000), is too complex for a discussion in an introductory text such as this. Nevertheless, you need to be aware that meso-research is becoming much more common for many of the same reasons we described in the consideration of “context” earlier in the chapter. Behavior in organizations cannot be neatly compartmentalized into either micro or macro levels. There are many influences that cut across levels of analysis. Many important questions about the experience of work require such a multi- level consideration (Drenth & Heller, 2004). Even though we don’t expect you to master the analytic techniques of multi-level research, you should at least be able to recognize these terms and understand at a basic level what they are meant to convey. As we will see in the final chapter of this book, the value of multi-level considerations can be seen when studying safety in the workplace. Safe behavior results from an intricate combination of individual worker characteristics (e.g., knowledge of how to work safely and abilities to work safely), work team influences (the extent to which team members reinforce safe work behavior in one another), leader behavior (the extent to which the work group leader adopts and reinforces safe work behavior), and the extent to which senior leaders of the organization acknowledge the impor- tance of safe work behavior (Wallace & Chen, 2006). Module 2.2 Summary Descriptive statistics are expressed in terms of absolute values without inter- pretation. Inferential statistics allow a researcher to identify a relationship between variables. The threshold for statistical significance is.05, or 5 occur- rences out of 100. Statistical power comes from using a large enough sample to make results reliable. A statistical index that can be used to estimate the strength of a linear relation- ship between two variables is called a correlation coefficient. The relationship can also be described graphically, in which case a regression line can be drawn to illustrate the relationship. A multiple correlation coefficient indicates the strength of the relationship between one variable and the composite of several other variables. Correlation is a means of describing a relationship between two variables. When examining any observed relationship and before drawing any causal inferences, the researcher must consider whether the relationship is due to a 74 Chapter 2 Research Methods and Statistics in I-O Psychology third variable or whether the second variable is causing the first rather than vice versa. Meta-analysis, the statistical analysis of multiple studies, is a powerful means of estimating relationships in those studies. It is a complex statistical procedure that includes information about statistical artifacts and other variables, and corrects for their influences. Key Terms descriptive statistics median scatterplot meta-analysis measure of central tendency inferential statistics regression line statistical artifacts variability statistical significance linear micro-research skew statistical power nonlinear macro-research mean measurement multiple correlation meso-research mode correlation coefficient coefficient M ODULE 2.3 Interpretation through Reliability and Validity So far, we have considered the scientific method, the design of research studies, the collection of data, and the statistical analyses of data. All of these procedures prepare us for the most important part of research and application: the interpretation of the data based on the statistical analyses. The job of the psychologist is to make sense out of what he or she sees. Data collection and analysis are certainly the foundations of making sense, but data do not make sense of themselves; instead, they require someone to interpret them. Any measurement that we take is a sample of some behavioral domain. A test of reasoning ability, a questionnaire related to satisfaction or stress, and a training grade are all samples of some larger behavioral domain. We hope that these samples are consistent, accurate, and representative of the domains of interest. If they are, then we can make accurate inferences based on these measurements. If they are not, our inferences, and ultimately our decisions, will be flawed, regardless of whether the decision is to hire someone, institute a new motivation program, or initiate a stress reduction program. We use measurement to assist in decision making. Because a sample of behavior is just that—an example of a type of behavior but not a complete assessment samples, by definition, are incomplete or imperfect. So we are always in a position of having to draw inferences or make decisions based on incomplete or imperfect measurements. The challenge is to make sure that the measurements are reliability Consistency or “complete enough” or “perfect enough” for our purposes. stability of a measure. The technical terms for these characteristics of measurement are reliability and validity The accuracy of validity. If a measure is unreliable, we would get different values each time we sam- inferences made based on pled the behavior. If a measure is not valid, we are gathering incomplete or inaccurate test or performance data; information. Although the terms “reliability” and “validity” are most often applied to also addresses whether a test scores, they could be applied to any measure. We must expect reliability and measure accurately and validity from any measure that we will use to infer something about the behavior of completely represents an individual. This includes surveys or questionnaires, interview responses, perfor- what was intended to mance evaluation ratings, and test scores. be measured. Reliability When we say that someone is “reliable,” we mean that he or she is someone we can count on, someone predictable and consistent, and someone we can depend on for 75 76 Chapter 2 Research Methods and Statistics in I-O Psychology help if we ask for it. The same is true of measures. We need to feel confident that if we took the measure again, at a different time, or if someone else took the measure- ment, the value would remain the same. Suppose that you went for a physical and before you saw the doctor, the nurse took your temperature and found it to be 98.6°. If the doctor came in five minutes later and retook your temperature and reported that it was 101.5°, you would be surprised. You would have expected those readings to agree, given the short time span between measurements. With a discrepancy this large, you would wonder about the skill of the nurse, the skill of the doctor, or the adequacy of the thermometer. In technical terms, you would wonder about the reli- ability of that measure. Test–Retest Reliability There are several different aspects to measurement reliability. One aspect is sim- ply the temporal consistency—the consistency over time—of a measure. Would we have gotten the same value had we taken the measurement next week as opposed to this week, or next month rather than this month? If we set out to measure some- one’s memory skills and this week find that they are quite good, but upon retesting the same person next week we find that they are quite poor, what do we conclude? Does the participant have a good memory or not? Generally speaking, we want our measures to produce the same value over a reasonable time period. This type of test–retest reliability A reliability, known as test–retest reliability, is often calculated as a correlation coef- type of reliability calcu- ficient between measurements taken at time 1 and measurements taken at time 2. lated by correlating meas- Consider Figure 2.8. On the left, you see high agreement between measures of the urements taken at time 1 same people taken at two different points in time. On the right, you find low levels with measurements taken of agreement between the two measurements. The measurements on the left would at time 2. be considered to have high test–retest reliability, while those on the right would be considered to have low test–retest reliability. P 70 P P 70 P N O 60 N N O 60 O N O K L M 50 K K L M 50 L M K L M Occasion 1 Occasion 1 G H I J 40 G G H I J 40 G H J I H I D E F 30 D D E F 30 E D F J E B C 20 B F B C 20 C B C A 10 A A 10 A Score Score Score 10 20 30 40 50 60 70 Score 10 20 30 40 50 60 70 A B D G K N P G A C B E D F C E H L O H J I K N F I M L M O J P A Occasion 2 B Occasion 2 FIGURE 2.8 Examples of High and Low Test–Retest Reliability: Score Distributions of Individuals Tested on Two Different Occasions Reliability 77 Equivalent Forms Reliability Remember when you took the SAT®? The SAT has been administered to millions of high school students over the decades since its introduction. But the same SAT items have not been administered to those millions of students. If that were the case, the answers to those items would have long since been circulated among dishonest test takers. For many students, the test would simply be a test of the extent to which they could memorize the right answers. Instead, the test developers have devised many different forms of the examination that are assumed to cover the same general content, but with items unique to each form. Assume that you take the test in Ames, Iowa, and another student takes a different form of the test in Philadelphia. How do we know that these two forms reliably measure your knowledge and abilities, that you would have gotten roughly the same score had you switched seats (and tests) with the other student? Just as is the case in test–retest reliability, you can have many people take two different forms of the test and see if they get similar scores. By correlating the two test scores, you would be calculating the equivalent forms reliability of that equivalent forms test. Look at Figure 2.8 again. Simply substitute the term “Form A” for “Occasion 1” reliability A type of reli- and “Form B” for “Occasion 2” and you will see that the left part of the figure would ability calculated by corre- describe a test with high equivalent forms reliability, while the test on the right would lating measurements from demonstrate low equivalent forms reliability. a sample of individuals who complete two differ- ent forms of the same test. Internal Consistency As you can see from the examples above, to calculate either test–retest or equiva- lent forms reliability, you would need to have two separate testing sessions (with either the same form or different forms). Another way of estimating the reliability of a test is to pretend that instead of one test, you really have two or more. A simple example would be to take a 100-item test and break it into two 50-item tests by col- lecting all of the even-numbered items together and all of the odd-numbered items together. You could then correlate the total score for all even-numbered items that were answered correctly with the total score for all of the odd-numbered items answered correctly. If the subtest scores correlated highly, you would consider the test reliable from an internal consistency standpoint. If we are trying to measure internal consistency Form a homogeneous attribute (e.g., extraversion, stress), all of the items on the test of reliability that assesses should give us an equally good measure of that attribute. There are more sophisti- how consistently the items cated ways of estimating internal consistency reliability based on the average corre- of a test measure a single lation between every pair of test items. A common statistic used to estimate internal construct; affected by the number of items in the consistency reliability using such averages is known as Cronbach’s alpha (Cho & Kim, test and the correlations 2015; Cortina, 1993). among the test items. Inter-Rater Reliability Often several different individuals make judgments about a person. These judg- ments might be ratings of a worker’s performance made by several different super- visors, assessments of the same candidate by multiple interviewers, or evaluations made by several employees about the relative importance of a task in a particular job. In each of these cases, we would expect the raters to agree regarding what they have observed. We can calculate various statistical indices to show the level of agree- ment among the raters. These statistics would be considered estimates of inter-rater reliability. 78 Chapter 2 Research Methods and Statistics in I-O Psychology As you can see from our discussion of reliability, there are different ways to cal- culate the reliability index, and each may describe a different aspect of reliability. To the extent that any of the reliability coefficients are less than 1.00 (the ideal coeffi- cient denoting perfect reliability), we assume that there is some error in the observed score and that it is not a perfectly consistent measure. Nevertheless, measures are not expected to be perfectly reliable; they are simply expected to be reasonably reli- able. The convention is that values in the range of.70 to.80 represent reasonable reliability. Although we have considered each of these methods of estimating reli- ability separately, they all address the same general issue that we covered earlier in generalizability theory A the chapter: generalizability. The question is: To what extent can we generalize the sophisticated approach to meaning of a measure taken with one measurement device at one point in time? the question of reliabil- A more sophisticated approach to the question of reliability is based in generaliz- ity that simultaneously considers all types of error ability theory (Guion, 2011), which considers all different types of error (e.g., test– in reliability estimates retest, equivalent forms, and internal consistency) simultaneously, but a description (e.g., test–retest, equiva- of this technique is beyond the scope of this text. For the interested reader, Putka lent forms, and internal and Sackett (2010) present an excellent conceptual and historical treatment of the consistency). evolution of reliability theory and generalizability theory. Validity The second characteristic of good measurement is validity, which addresses the issue of whether the measurements we have taken accurately and completely represent what we had hoped to measure. For example, consider the job of a physician in general practice. Suppose that we wanted to develop a measure of the performance of general practitioners and that we decided to use malpractice insurance rates over the years as a measure of performance. We note that these rates have gone up every year for a particular physician, and we conclude that the physician must not be very good. If he or she were good, we would have expected such malpractice premiums to have gone down. In the physician example, the measure we have chosen to represent performance would be neither accurate nor complete. Malpractice rates have much less to do with a particular doctor than they do with claims in general and with amounts awarded by juries in malpractice lawsuits. Both the number of malpractice suits and the jury awards for those suits have climbed steadily over the past few decades. As a result, you would note that malpractice premiums (like car insurance premiums) have climbed steadily every year for almost every physician. Furthermore, a physician in general prac- tice has a wide variety of duties, including diagnosis, treatment, follow-up, education, referral, record keeping, continuing education, and so forth. Even if malpractice premium rates were accurate representations of performance in certain areas such as diagnosis and treatment, many other areas of performance would have been ignored by this one measure. For both reliability and validity, the question is whether what we have mea- sured allows us to make predictions or decisions, or take actions, based on what we assume to be the content of those measures. In our physician example, if we were deciding whether to allow a physician to keep a medical license or to be added to the staff of a hospital, and we based that decision on our chosen “performance” measure (malpractice premiums), our decision (or inference that physicians with a history of increasing premiums are poor performers) would not be a valid deci- sion or inference. Note that the reliability of a measure puts a ceiling, or limit, on the validity of that measure. That is, if the reliability of a measure is low, then it will Validity 79 be difficult to find a valid relationship or correlation between that measure and another measure. You will remember that we concluded our discussion of reliability by introducing the concept of generalizability. What we said was that reliability was really a unitary phenomenon and that the various estimates of reliability (e.g., test–retest) were really just different ways to get at a single issue: consistency of measurement. The important concept to keep in mind was generalizability. The same is true of validity. Like reliability, there are several different ways to gather information about the accu- racy and completeness of a measure. Also like reliability, validity is a unitary concept; you should not think that one type of validity tells you anything different about the completeness and accuracy of a measure than any other type of validity (Binning & Barrett, 1989; Guion, 2011; Landy, 1986). Like reliability, validity concerns the confi- dence with which you can make a prediction or draw an inference based on the mea- surements you have collected. There are three common ways of gathering validity evidence. We will describe each of these three ways below. Although validity is relevant to discussions of any measurement, most validity studies address the issue of whether an assessment permits confident decisions about hiring or promotion. Although most validity studies revolve around tests (e.g., tests of personality or cognitive ability), other assessments (e.g., interviews, application blanks, or even tests of aerobic endurance) might form the basis of a validity study. For the purposes of this chapter, we will use hiring and promo- tion as the examples of the decisions that we have to make. For such purposes, we have a general hypothesis that people who score higher or better on a particular measure will be more productive and/or satisfied employees (Landy, 1986). Our validity investiga- C D tion will be focused on gathering information that Job Analysis will make us more confident that this hypothesis can be supported. If we are able to gather such confirming information, we can make decisions Conceptual Job-Related Job about individual applicants with confidence—our Level Attributes Demands inference about a person from a test score will be valid. Remember, validity is not about tests, it is B B about decisions or inferences. I-O psychologists usually gather validity evidence using one of three common designs. We will con- Operational Predictors Criteria Level A sider each of these designs in turn. All three fit into the same general framework shown in Figure 2.9. FIGURE 2.9 Validation Process from Conceptual and Operational Levels The box on the top is labeled “Job Analysis.” Job analysis is a complex and time-consuming process predictor The test chosen that we will describe in detail in Chapter 4. For the purposes of the current discus- or developed to assess sion, you simply need to think of job analysis as a way of identifying the important attributes (e.g., abilities) demands (e.g., tasks, duties) of a job and the human attributes necessary to carry out identified as impor- those demands successfully. Once the attributes (e.g., abilities) are identified, the tant for successful job test that is chosen or developed to assess those abilities is called a predictor, which is performance. used to forecast another variable. Similarly, when the demands of the job are iden- criterion An outcome tified, the definition of an individual’s performance in meeting those demands is variable that describes called a criterion, which is the variable that we want to predict. In Figure 2.9, a line important aspects or with an arrow connects predictors and criteria. This line represents the hypothesis demands of the job; the we outlined above. It is hypothesized that people who do better on the predictor will variable that we predict also do better on the criterion—people who score higher will be better employees. when evaluating the valid- We gather validity evidence to test that hypothesis. ity of a predictor. 80 Chapter 2 Research Methods and Statistics in I-O Psychology Criterion-Related Validity The most direct way to support the hypothesis (i.e., to connect the predictor and criteria boxes) is to actually gather data and compute a correlation coefficient. In criterion-related validity this design, technically referred to as a criterion-related validity design, you would Validity approach that is correlate test scores with performance measures. If the correlation was positive and demonstrated by cor- statistically significant, you would now have evidence improving your confidence in relating a test score with the inference that people with higher test scores have higher performance. By corre- a performance measure; lating these test scores with the performance data, you would be calculating what is improves researcher’s con- known as a validity coefficient. The test might be a test of intelligence and the perfor- fidence in the inference mance measure might be a supervisor’s rating. Since we mentioned a “supervisor’s that people with higher test scores have higher rating,” something becomes immediately obvious about this design: We are using the performance. test scores of people who are employed by the organization. This can be done in two different ways. validity coefficient Corre- lation coefficient between Predictive Validity The first method of conducting a criterion-related study is to a test score (predictor) administer a particular test to all applicants and then hire applicants without using and a performance meas- ure (criterion). scores from that particular test to make the hiring decision. You would then go back to the organization after some time period had passed (e.g., six or nine months) and collect performance data. This design, where there is a time lag between the collec- predictive validity tion of the test data and the criterion data, is known as a predictive validity design design Criterion-related because it enables you to predict what would have happened had you actually used validity design in which the test scores to make the hiring decisions. If the test scores were related to perfor- there is a time lag between mance scores, you might conclude that you should not have hired some people. collection of the test data Their performance was poor, as were their test scores. From the point at which the and the criterion data. employer knows that the validity coefficient is positive and significant, test scores can be used for making future hiring decisions. The validity coefficient does not, by itself, tell you what score to designate as a passing score. We will deal with this issue in Chapter 6, where we consider the actual staffing process. The predictive validity design we have described above is only one of many different predictive designs you might employ. Concurrent Validity In research on many diseases, such as cancer and coronary heart disease, researchers carry out a process known as a clinical trial. The clinical trial design assigns some patients to a treatment group and others to a control group. The treatment group actually gets the treatment under study (e.g., a pill), whereas the control group does not. Instead, the control group gets a placebo (e.g., a pill with neutral ingredients). It is difficult to recruit patients for many clinical trials because they want to be in the treatment group and don’t want to take the chance of being assigned to a control group (although they would not typically know to which group they had been assigned). If the treatment is actually effective, it will benefit the treatment group patients, but the control group patients will not experience the ben- efits. Many employers and I-O researchers are like the prospective patients for the control group—they don’t want to wait months or even years to see if the “treatment” (e.g., an ability test) is effective. While they are waiting for the results, they may be concurrent validity hiring ineffective performers. design Criterion-related There is a criterion-related validity design that directly addresses that concern. It validity design in which there is no time lag is called the concurrent validity design. This design has no time lag between gath- between gathering the test ering the test scores and the performance data because the test in question is admin- scores and the perfor- istered to current employees rather than applicants, and performance measures mance data. can be collected on those employees simultaneously, or concurrently (thus the term Validity 81 “concurrent design”). Since the employees are actually working for the organization, the assumption is made that they must be at least minimally effective, alleviating any concern about adding new employees who are not minimally effective. As in the case of the predictive design, test scores are correlated with performance scores to yield a validity coefficient. If it is positive and significant, the test is then made part of the process by which new employees are hired. There is a potential disadvantage in using the concurrent design, however. We have no information about those who are not employed by the organization. This has both technical and practical implications. The technical implication is that you have range restriction—only the scores of those who scored highly on the predictor—so the correlation coefficient may be artificially depressed and may not be statistically significant. There are statistical corrections that can offset that problem. The practical problem is that there might have been applicants who did less well than the employees did on the test yet might have been successful per- formers. Since they were never hired, the employer will never know. I-O psycholo- gists have conducted a good deal of research comparing concurrent and predictive designs, and their general conclusion has been that, even though the concurrent design might underestimate validity coefficients, in practice this does not usually happen (Schmitt, Gooding, Noe, & Kirsch, 1984). One final problem with concur- rent designs is that the test-taking motivation may not be as high for those who are already employed. It is also useful to remember that both concurrent and predic- tive designs are only two variations on many different ways to assemble validity data (Guion, 2011; Landy, 1986, 2007). We will now consider two additional methods for collecting validity data. Content-Related Validity The SIOP Principles define a content-related validation design as “a study that dem- content-related validation onstrates that the content of the selection procedure represents an adequate sample design A design that dem- of important work behaviors and activities and/or worker knowledge, skills, abilities, onstrates that the content or other characteristics (KSAOs) defined by the analysis of work” (SIOP, 2003). The of the selection procedure job analysis in Figure 2.9 is an example of this strategy. As another example, assume represents an adequate sample of important work that you are the director of a temporary employment agency and want to hire appli- behaviors and activities cants who can be assigned to word-processing tasks for companies. You know that and/or worker KSAOs these companies typically use either WordPerfect or Microsoft Word and use either defined by the job analysis. a Macintosh or a PC system. So you ask the job applicants to demonstrate their pro- ficiency with both of these word-processing packages on both PCs and Macs. Since not all employers have the latest hardware or software, you also ask the applicants to perform sample word-processing tasks on various versions of the software and differ- ent vintages of hardware. By doing this, you have taken the essence of the work for which you are hiring individuals—word processing on any of a number of hardware and software configurations—and turned it into a test. There can be little argument that, at least conceptually, there is a clear link in our example between test scores and probable performance. Of course, you would also need to demonstrate that the test you had assembled fairly represented the types of word-processing projects that the temporary employees would encounter. If you were using only the word-processing test, you would also need to show that actual word-processing (e.g., as opposed to developing financial spreadsheets with Excel) is the most important part of the work for which these temps are hired. If, for example, the temps were hired to answer phones or manually file records, the test of word processing would be largely irrelevant. But assuming that the job the temps will be 82 Chapter 2 Research Methods and Statistics in I-O Psychology asked to do is word processing, you can infer that applicants who do better on your test will tend to do better at the actual word-processing tasks in the jobs to which they are assigned. The validity of the inference is based not on a correlation but on a logical comparison of the test and the work. To return to Figure 2.9, although the focus of the study is the association between a predictor and a criterion (in this case, the speed and accuracy of word processing), no criterion information from the work setting is collected. The example of the word-processing test was simple and straightforward. Many jobs are not quite as simple as that of a word processor. Consider the position of a manager of a cellular telephone store with 5 inside and 15 outside sales and techni- cal representatives. Suppose that the company opened a companion store in the next town and needed to hire a manager for that store. How could we employ a content-related design to gather data that would give us confidence in making the hiring decision? The job of manager is complex, involving many varied tasks, as well as a wide variety of knowledge, skills, abilities, and interpersonal attributes. Using Figure 2.9 as our model, we would analyze the job to determine the most important tasks or duties, as well as the abilities needed to perform those tasks. We would do this by asking experienced employees and supervisors in other cellular phone stores to give us the benefit of their observations and personal experience. We would ask them to complete one or more questionnaires that covered tasks and their impor- tance and necessary abilities. Based on an analysis of their answers, we could then identify or develop possible predictors for testing manager candidates. We would then choose the set of predictors that measured abilities that had been judged to be most closely related to various performance demands for managers. Through the use of knowledgeable employees and supervisors, we would have been able to make the logical connection between the predictors and anticipated performance. Although content-related validation designs for jobs can become rather complex, we have described the “basic” model so you can get a feel for how the content-related strategy differs from the criterion-related strategy. But remem- ber, both strategies are addressing the same basic hypothesis: People who do better on our tests will do better on the job. Construct Validity construct validity Validity Calling construct validity a “type” of validity is a historical accident and not really approach in which inves- correct (Landy, 1986). In the 1950s, a task force outlined several ways to gather valid- tigators gather evidence ity evidence and labeled three of them: criterion, content, and construct (Cronbach to support decisions or & Meehl, 1955). The labels have stuck. Modern I-O psychology, however, does not inferences about psycho- recognize that distinction—referred to sarcastically by Guion (1980) as the “holy logical constructs; often trinity.” Instead, as we have described above, validity is considered “unitarian.” There begins with investigators are literally hundreds of ways of gathering evidence that will increase the confidence demonstrating that a test designed to measure a of our decisions or inferences. Criterion-related designs and content-related designs particular construct cor- are two of the many available approaches (Guion, 2011; Landy, 1986). Every study relates with other tests in could have a different design, even though some may be more popular than others. the predicted manner. The same is true with validity designs. Every validity study could have a different design, but criterion- and content-related designs are among the most popular, for reasons we will describe below. Construct validity represents “the integration of evidence that bears on the interpretation or meaning of test scores—including content and criterion-related evidence—which are subsumed as part of construct validity” (Messick, 1995, p. 742). Validity 83 A construct can be defined as a psychological concept or characteristic that a predictor is intended to mea- Job Analysis sure (SIOP, 2003). A construct is a broad representa- C D tion of a human characteristic. Intelligence, personality, and leadership are all examples of constructs. Memory, Conceptual Job-Related Job assertiveness, and supportive leader behavior are all Level Attributes Demands examples of these broader entities. Examine Figure 2.10. As you can see by comparing this with Figure 2.9, we have simply added the term “con- struct” to our generic validation model. The modified Construct Construct figure demonstrates that constructs are related to both attributes and job demands. Let’s consider the job of a financial consultant for an investment banking firm. As B B a result of a job analysis, we were able to determine that memory and reasoning were important parts of the job of Operational Predictors Criteria a financial consultant because the job required the con- Level A sultant to remember data about various stocks and bonds and to use that information to develop an investment FIGURE 2.10 A Model for Construct Validity strategy for an individual client. What is one of the broad and essential attributes necessary both to do well on a test of reasoning and memory and to be effective in advising clients on how they should construct Psychological invest their money? It is intelligence, or cognitive ability. In this case, the construct concept or character- is intelligence, and we see it as underlying both performance on the test and perfor- istic that a predictor is mance on the job. In other words, doing well on the job requires the same construct intended to measure; as doing well on the test. examples are intelli- gence, personality, and The