A Statistical Refresher PDF
Document Details
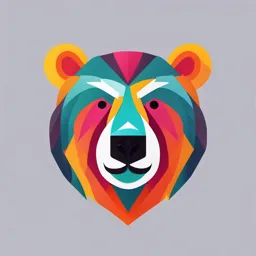
Uploaded by AwedMiami4698
Tags
Related
- A Statistics Refresher PDF
- Group Presentation Chapter 4_ Statistics PDF
- PSY 103 Mod 1 Statistics Notes PDF
- Basic Statistics, Reliability, Validity, and Utility Lecture Notes PDF
- PSY 201 Introduction to Statistics for Psychology I Lecture Slides PDF
- Statistik I: Einführung und Messtheorie/Skalenniveau PDF
Summary
This document provides a refresher on statistical concepts, focusing on measurement scales and variables. It defines key terms and discusses how concepts in psychology are measured. The presentation also details the type of data and associated operations.
Full Transcript
A Statistical Refresher /JMSG We all use numbers as a basic way of communicating. Think about how many times you use numbers in an average day. There is no way to avoid them One advantage of number systems is that they allow us to manipulate information. Through sets of well-defi...
A Statistical Refresher /JMSG We all use numbers as a basic way of communicating. Think about how many times you use numbers in an average day. There is no way to avoid them One advantage of number systems is that they allow us to manipulate information. Through sets of well-defined rules, we can use numbers to learn more about the world. Why We Need Statistics First, statistics are used for purposes of description. Second, we can use statistics to make inferences, which are logical deductions about events that cannot be observed directly. Why We Need Statistics Psychologists rely heavily on statistics to help assess the meaning of the measurements they make. If statistics-related terms look painfully familiar to you, seek your indulgence and you need to remember that overlearning is the key to retention. Measurement In psychology we are interested in either describing the distributions of and/or relationships among abstract concepts: e.g., —Political conservatism —Intelligence —Neuroticism —Aggression Measurement However, in most cases these constructs are abstractions that can often not be directly observed. Concept of Intelligence Operationalization Measure or Operationalization of Intelligence IQ test Measurement Note: that the degree to which the operationalization of the abstract concept actually reflects or mirrors the construct is the degree to which the operationalization can be said to be valid (more later). The value of scientific research is completely dependent upon the degree to which the operationalizations are successful or valid. Concepts and Constructs Concept: —“An abstraction formed by generalization from particulars” —Abstracts are hard to define —E.g. intelligence Construct: —A concept with scientific purpose (i.e. operationalized) —Can be measured and studied. —E.g. IQ Terms Review: Variables and Constants Variable: any condition, event, characteristic or attribute that can take on different values at different times or with different people. —Age of people —Temperature —Intelligence —Xenophobia Constant: —One value in a given context. —Does not change or vary. Terms Review (Experimental): Independent and Dependent Variables Independent (manipulated/treatment) variable —we are referring to a variable that the experimenter has some direct control over and can manipulate —In Experiments IVs are the “cause” —In non-experiments IVs are the “influence —i.e., X → Y Dependent Variables —The variable being influenced/predicted —The outcome variable Terms Review (Nonexperimental): predictor and criterion variables Criterion (outcome) variable —The presumed effect in a nonexperimental study. Predictor Variables —The presumed “cause” on a nonexperimental study. Often used in correlational studies. Terms Review (others) Binary (Dichotomous) variable —Observations (i.e., dependent variables) that occur in one of two possible states, often labelled zero and one. Dummy Variables —Created by recoding categorical variables that have more than two categories into a series of binary variables Endogenous variable —A variable that is an inherent part of the system being studied and that is determined from within the system. —A variable that is caused by other variables in a causal system. Terms Review (others) Confounding variable —A variable that obscures the effects of another variable —Ex. Which is the cause: Effect of the treatment or skills of the experimenter? Control variable —An extraneous variable that an investigator does not wish to examine in a study. Thus the investigator controls this variable. — Also called a covariate. Terms Review (others) Exogenous variable —A variable entering from and determined from outside of the system being studied. —A causal system says nothing about its exogenous variables. Latent variable —An underlying variable that cannot be observed Manifest variable —An observed variable assumed to indicate the presence of a latent variable. Terms Review (others) Mediating variable —A variable that explains a relation or provides a causal link between other variables. Moderating variable —A variable that influences, or moderates, the relation between two other variables and thus produces an interaction effect Polychotomous variables —Variables that can have more than two possible values. —Strictly speaking, this includes all but binary variables. Scales of Measurement measurement as the act of assigning numbers or symbols to characteristics of things (people, events, whatever) according to rules. The rules used in assigning numbers are guidelines for representing the magnitude (or some other characteristic) of the object being measured. Scales of Measurement A scale is a set of numbers (or other symbols) whose properties model empirical properties of the objects to which the numbers are assigned. Categorization —Continuous —Discrete Scales of Measurement Discrete variables: can only take on a finite or restricted set of values. —Can only take on whole values (think digital) —E.g., number of children per family, Number of students taking 100A Continuous variables: can take an infinite number of values —E.g., Temperature (10.3 C, 10.24 C, 15.212 C), Weight (102.2lbs., 116.56 lbs.) The difference often limited only by precision Scales of Measurement Measurement always involves error. —In the language of assessment, error refer to the collective influence of all of the factors on a test score or measurement beyond those specifically measured by the test or measurement. Scales of Measurement Properties of Scales —magnitude, —equal intervals, and —an absolute 0 Scales of Measurement Magnitude is the property of “moreness.” Ex. Labels and ranking Scales of Measurement Equal Intervals —A scale has the property of equal intervals if the difference between two points at any place on the scale has the same meaning as the difference between two other points that differ by the same number of scale units. —When a scale has the property of equal intervals, the relationship between the measured units and some outcome can be described by a straight line or a linear equation in the form Y = a + bX. Scales of Measurement Equal Intervals Ex. the difference between intelligence quotients (IQs) of 45 and 50 does not mean the same thing as the difference between IQs of 105 and 110. Although each of these differences is 5 points (50 – 45 = 5 and 110 – 105 = 5), the 5 points at the first level do not mean the same thing as 5 points at the second. We know that IQ predicts classroom performance. However, the difference in classroom performance associated with differences between IQ scores of 45 and 50 is not the same as the differences in classroom performance associated with IQ score differences of 105 and 110. Scales of Measurement Absolute 0 —obtained when nothing of the property being measured exists. For many psychological qualities, it is extremely difficult, if not impossible, to define an absolute 0 point. —For example, if one measures shyness on a scale from 0 through 10, then it is hard to define what it means for a person to have absolutely no shyness (McCall, 2001). Scales of Measurement Types of Scales —The French word for black is noir (pronounced “n’wa˘re”). —Each letter in noir is the first letter of the succeedingly more rigorous levels: N stands for nominal, o for ordinal, i for interval, and r for ratio scales. Scales of Measurement Nominal scales —simplest form of measurement —are really not scales at all; their only purpose is to name objects. —used when the information is qualitative rather than quantitative. Scales of Measurement Nominal scales —When these numbers have been attached to categories, most statistical procedures are not meaningful. —This is not to say that the sophisticated statistical analysis of nominal data is impossible. Indeed, several new and exciting developments in data analysis allow extensive and detailed use of nominal data —include counting for the purpose of determining how many cases fall into each category and a resulting determination of proportion or percentages. Scales of Measurement Ordinal scales —allows you to rank individuals or objects but not to say anything about the meaning of the differences between the ranks. —imply nothing about how much greater one ranking is than another. —do not indicate units of measurement —For most problems in psychology, the precision to measure the exact differences between intervals does not exist. So, most often one must use ordinal scales of measurement. Scales of Measurement Interval Scales —contain equal intervals between numbers. Each unit on the scale is exactly equal to any other unit on the scale. But like ordinal scales, interval scales contain no absolute zero point. —With interval scales, we have reached a level of measurement at which it is possible to average a set of measurements and obtain a meaningful result. —Because interval scales contain no absolute zero point, a presumption inherent in their use is that no testtaker possesses none of the ability or trait (or whatever) being measured Scales of Measurement Ratio Scales —has a true zero point. —In psychology, ratio-level measurement is employed in some types of tests and test items, perhaps most notably those involving assessment of neurological functioning. —However, it is only theoretical to get an exact zero in behaviors Scales of Measurement Scales of Measurement and Their Properties Property Types of Magnitude Equal Absolute 0 Scale Intervals Nominal No no No Ordinal Yes No No Interval Yes Yes No Ration yes Yes yes Permissible Operations Level of measurement is important because it defines which mathematical operations we can apply to numerical data. For nominal data, each observation can be placed in only one mutually exclusive category. One can use nominal data to create frequency distributions, but no mathematical manipulations of the data are permissible. Permissible Operations Ordinal measurements can be manipulated using arithmetic; however, the result is often difficult to interpret because it reflects neither the magnitudes of the manipulated observations nor the true amounts of the property that have been measured. Permissible Operations With interval data, one can apply any arithmetic operation to the differences between scores. The results can be interpreted in relation to the magnitudes of the underlying property. However, interval data cannot be used to make statements about ratios. Permissible Operations The mathematical operation is reserved for ratio scales, for which any mathematical operation is permissible. Describing Data 1. Frequency distribution 2. Measures of central tendency 3. Measures of Variability 4. Skewness 5. Kurtosis Describing Data distribution —defined as a set of test scores arrayed for recording or study. raw score — is a straightforward, unmodified accounting of performance that is usually numerical. One task at hand is to communicate the test results Frequency distribution Frequency distribution —all scores are listed alongside the number of times each score occurred. —The scores might be listed in tabular or graphic form. Frequency distribution Frequency Distribution —simple frequency distribution to indicate that individual scores have been used and the data have not been grouped. —grouped frequency distribution, test- score intervals, also called class intervals, replace the actual test scores. The number of class intervals used and the size or width of each class interval (i.e., the range of test scores contained in each class interval) are for the test user to decide. Frequency distribution Percentiles are the specific scores or points within a distribution. Percentiles divide the total frequency for a set of observations into hundredths. Instead of indicating what percentage of scores fall below a particular score, as percentile ranks do, percentiles indicate the particular score, below which a defined percentage of scores falls. Frequency distribution Percentile Ranks —replace simple ranks when we want to adjust for the number of scores in a group. —answers the question, “What percent of the scores fall below a particular score (Xi)?” Frequency distribution calculate a percentile rank 1. determine how many cases fall below the score of interest, 2. determine how many cases are in the group, 3. divide the number of cases below the score of interest (Step 1) by the total number of cases in the group (Step 2), and 4. multiply the result of Step 3 by 100. Measures of Central Tendency measure of central tendency is a statistic that indicates the average or midmost score between the extreme scores in a distribution. —Mean —Median —Mode Used to summarize data A variable (X) is a score that can have different values Measures of Central Tendency Mean —arithmetic mean, denoted by the symbol X̄ (pronounced “X bar”), is equal to the sum of the observations (or test scores in this case) divided by the number of observations. —Symbolically written, the formula for the arithmetic mean is X̄ Σ(X/n), where n equals the number of observations or test scores. If grouped, X̄ Σ(fX/n) —Average —appropriate measure of central tendency for interval or ratio data when the distributions are believed to be approximately normal. Measures of Central Tendency Mode —The most frequently occurring score in a distribution of scores —useful in analyses of a qualitative or verbal nature. —Because the mode is not calculated in a true sense, it is a nominal statistic and cannot legitimately be used in further calculations. —On a histogram it represents the highest bar in a bar chart or histogram. Measures of Central Tendency Median —statistic that takes into account the order of scores and is itself ordinal in nature. — basic advantage of the median over the mean in describing data is that it is resilient to extremely large or small values, and may be a better descriptor of a "typical" outcome. Measures of Central Tendency Summary of when to use the mean, median and mode Type of Variable Best measure of central tendency Nominal Mode Ordinal Median Interval/Ratio (not skewed) Mean Interval/Ratio (skewed) Median Measures of Variability Variability is an indication of how scores in a distribution are scattered or dispersed. Statistics that describe the amount of variation in a distribution are referred to as measures of variability. —The range —The interquartile and semi-interquartile ranges —The average deviation —The standard deviation Measures of Variability Range —range of a distribution is equal to the difference between the highest and the lowest scores. —may results to understated or overstated because of extreme scores. —Ex. 1 2 3 4 5 6 7 8 9 10 Range = 10 – 1 =9 Measures of Variability Quartiles and Deciles ranges The two terms refer to divisions of the percentile scale into groups. The quartile system divides the percentage scale into four groups, whereas the decile system divides the scale into 10 groups. Measures of Variability Quartiles and Deciles ranges —dividing points between the four quarters in the distribution are the quartiles Measures of Variability Quartiles and Deciles ranges —interquartile range is a measure of variability equal to the difference between Q3 and Q1. —semi-interquartile range, which is equal to the interquartile range divided by 2. —Knowledge of the relative distances of Q1 and Q3 from Q2 (the median) provides the seasoned test interpreter with immediate information as to the shape of the distribution of scores. Measures of Variability Quartiles and Deciles ranges Deciles are similar to quartiles except that they use points that mark 10% rather than 25% intervals. stanine system —This system converts any set of scores into a transformed scale, which ranges from 1 to 9. —Actually the term stanine comes from “standard nine.” Measures of Variability Average Deviation The average deviation is rarely used. Perhaps this is so because the deletion of algebraic signs renders it a useless measure for purposes of any further operations Measures of Variability standard deviation —measure of variability equal to the square root of the average squared deviations about the mean. —More succinctly, it is equal to the square root of the variance. —The variance is equal to the arithmetic mean of the squares of the differences between the scores in a distribution and their mean. For mean for raw scores Measures of Variability standard deviation —The symbol for standard deviation has variously been represented as s, S, SD, and the lowercase Greek letter sigma (σ). —square root of the variance is the standard deviation (σ), and it is represented by the following formula For population for samples For mean for raw scores The Normal Curve scientists referred to it as the “Laplace- Gaussian curve.” Karl Pearson is credited with being the first to refer to the curve as the normal curve Theoretically, the normal curve is a bell- shaped, smooth, mathematically defined curve that is highest at its center. From the center it tapers on both sides approaching the X -axis asymptotically (meaning that it approaches, but never touches, the axis). The Normal Curve Measures of Shape: Skewness and Kurtosis Skewness —the nature and extent to which symmetry is absent Kurtosis —The term testing professionals use to refer to the steepness of a distribution in its center Skewness positive skew when relatively few of the scores fall at the high end of the distribution. negative skew when relatively few of the scores fall at the low end of the distribution. Skewness how can you interpret the skewness number? Bulmer (1979) — a classic — suggests this rule of thumb: —If skewness is less than −1 or greater than +1, the distribution is highly skewed. —If skewness is between −1 and −0.5 or between + 0.5 and +1, the distribution is moderately skewed. —If skewness is between − 0.5 and + 0.5, the distribution is approximately symmetric. Kurtosis Distributions are generally described —platykurtic (relatively flat), — leptokurtic (relatively peaked), or —somewhere in the middle— mesokurtic. Kurtosis The reference standard is a normal distribution, which has a kurtosis of 3. In token of this, often the excess kurtosis is presented: excess kurtosis is simply kurtosis−3. For example, the “kurtosis” reported by Excel is actually the excess kurtosis. Kurtosis A normal distribution has kurtosis exactly 3 (excess kurtosis exactly 0). Any distribution with kurtosis ≈3 (excess ≈0) is called mesokurtic. A distribution with kurtosis 0) is called leptokurtic. Compared to a normal distribution, its tails are longer and fatter, and often its central peak is higher and sharper.