Chapter 12: The Correlational Research Strategy PDF
Document Details
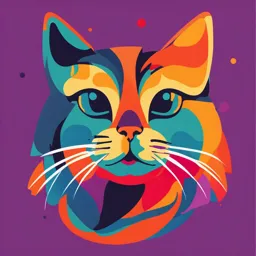
Uploaded by PreciousMossAgate7078
McGill University
Tags
Summary
This document discusses correlational research strategy in psychology, including examples and applications. It emphasizes the importance of understanding the relationship between variables without implying causality.
Full Transcript
PSYC 306 Research Methods in Psychology Chapter 12: The Correlational Research Strategy 1 Outline Correlational strategy The correlation coefficient Measuring correlations Strengths and Weaknesses Timepoint...
PSYC 306 Research Methods in Psychology Chapter 12: The Correlational Research Strategy 1 Outline Correlational strategy The correlation coefficient Measuring correlations Strengths and Weaknesses Timepoint-based Correlations 2 Correlational and experimental research – Correlational research: Intended to demonstrate the existence of a relationship between two variables Does not determine cause-and-effect relationship – Experimental research: Demonstrates a cause-and-effect relationship between two variables 3 Correlational Strategy To study the relationship between two (or more) variables Correlation describes the nature of the relationship: – Direction and degree of the relationship between two or more variables 4 Correlational Strategy The data collection is correlational: – No manipulations – Just measure variables Observations, surveys, physiological 5 Correlational Strategy High external validity, but cannot imply causality – How does the value of one variable change when the value of a second variable changes? 6 Correlational Strategy Examples: – Price of a box of chocolates and its quality (marketing) – Caffeine intake and alertness (basic research) – Movie topics and music preferences (art design) 7 Correlational Strategy Unit of Score on Score on Analysis Variable X Variable Y Time / Person 1 X1 Y1 Time / Person 2 X2 Y2 Time / Person 3 X3 Y3 Time / Person n Xn Yn 8 Correlational Strategy Shoe Size IQ score 5 110 6 112 7 118 7 120 8 122 8 122 9 130 10 140 9 Correlational Strategy The relationship between the variables can be visualized by means of a scatterplot Each point in scatterplot = each measurement Shoe size IQ score 5 110 6 Variable Y 112 7 118 7 120 8 122 8 122 9 130 10 140 Variable X 10 Correlational Strategy Each item/person represented by only one data point: Important assumption: each point in dataset is independent of other points This means no two points can come from same individual Shoe Size IQ Score 5 110 Variable Y 6 112 7 118 7 120 8 122 8 122 9 130 10 140 Variable X 11 Correlational Strategy Each item/person represented by only one data point: Important assumption: each point in dataset is independent of other points Shoe Size IQ Score 150 5 120 140 If several data 5 115 points from 130 IQ Score 5 110 same person, 120 6 112 then breaks 7 110 118 independence 7 120 100 assumptions 8 122 90 8 122 80 9 130 3 5 7 9 11 10 140 Shoe Size 12 Correlational Strategy A line-of-best-fit (regression line) is drawn through the points The closer the points are to the line, the greater the association between variables Knowledge of score on one dimension leads to prediction of other dimension Variable Y Variable X 13 Representing a Correlation A quantitative representation: – Correlation coefficient (r) ranges from -1.0 to +1.0 If one or two variables being correlated are ordinal, we use the Spearman rho (rs) When the two variables are on a ratio or interval scale, the strength is expressed as Pearson r For both Spearman and Pearson correlations, we want to know: Form (linear, non-linear) Direction (sign: - or +) Strength (absolute value between 0 and 1) 14 Nonlinear Correlations Straight Line = Linear Correlation Nonlinear Correlation Change in one variable is Change in one variable is not consistent with change on another consistent with change in another variable variable Region where X changes while Y does not 15 Spearman’s Rank-order correlation Spearman's correlation: determines the strength and direction of the monotonic relationship between two variables What is a monotonic relationship?. The relationship between two variables is monotonic when each of the 2 variables has values that continue in one direction or stay the same (neither variable can reverse direction) 16 Spearman’s Rank-order correlation When should you use the Spearman's rank-order correlation? When the data is ordinal scale (not interval or ratio scale) The data must be monotonic Spearman's correlation coefficient, (ρ, also signified by rs) measures the strength and direction of association between two ranked variables. 17 Calculating a Spearman Correlation 90 Raw Scores, plotted Math Scores 80 70 Raw Scores on Rank-ordered 60 Test: Scores: Not linear 50 English Math Rank(E) Rank(M) 40 56 66 9 4 30 75 70 3 2 30 50 70 90 45 40 10 10 Vocabulary Scores 71 60 4 7 61 71 6.5 5 12 Ranks, plotted 64 56 5 9 10 Math Ranks 58 59 8 8 8 80 77 1 1 6 76 67 2 3 61 63 6.5 6 4 Adjusts for 2 nonlinearities 0 0 2 4 6 8 10 12 18 Vocabulary Ranks Spearman’s Rank-order correlation When to use Spearman's rank-order correlation? With at least 5 pairs of data; preferably > 8 pairs ranks are not meaningful if you have too few pairs ranks are not meaningful if there are too many tied ranks 12 Ranks, plotted 90 Raw Scores, plotted Math Scores 10 Math Ranks 80 8 70 6 60 50 4 40 Adjusts for 2 30 nonlinearities 30 50 70 90 0 0 2 4 6 8 10 12 Vocabulary Scores Vocabulary Ranks 19 Calculating a Pearson Correlation A correlation coefficient describes the relationship between two variables. – It describes three characteristics of a relationship: A) Direction B) Form C) Consistency or strength Most behavioral research uses interval or ratio scale data Assume that the term “correlation” refers to Pearson correlation, r, if not specified 20 Direction of Correlation: Positive r value = when larger values of one variable are associated with larger values of another variable (or smaller with smaller) r > 0 when X increases, Y increases (or when X decreases, Y decreases) Negative r value = when larger values of one variable are associated with smaller values of another variable r < 0 when X increases, Y decreases (or when X decreases, Y increases) (negative) no relationship (positive) -1 0 +1 Negative correlations Positive correlations 21 Direction of Correlation: The direction of the correlation indicates the nature of the change in the variables Positive Negative A B A B A B A B 22 Direction of Correlation: Positive Negative 23 Direction of Correlation: POSITIVE linear correlation (+1) – High scores on one variable matched by high scores on another – Line slants up to the right NEGATIVE linear correlation (-1) – High scores on one variable matched by low scores on another – Line slants down to the right ZERO correlation (0) – No line, straight or otherwise, can be fit to the relationship between the two variables – Two variables are “uncorrelated” 24 Direction of Correlation: Can tell direction by tilt: – Upward from left to right (positive) -Downward from left to right (negative) 7 7 8 6 6 7 6 5 5 5 4 4 4 3 3 3 2 2 2 1 1 1 0 0 0 0 2 4 6 0 2 4 6 0 2 4 6 Perfect positive Moderate positive Weak positive 7 7 7 6 6 6 5 5 5 No correlation 4 4 4 3 3 3 2 2 2 1 1 1 0 0 0 0 2 4 6 0 2 4 6 0 2 4 6 Perfect negative Moderate negative Weak negative 25 Form of Correlation Linear relationship: the data points in the scatter plot tend to cluster around a straight line. – Positive linear relationship: each time the X variable increases by one point, the Y variable increases in a consistently predictable amount. A Pearson correlation describes and measures linear relationships when both variables are numerical scores from interval or ratio scales. Nonlinear relationship: the data points do not cluster around a straight line A Spearman correlation describes and measures monotonic nonlinear relationships when one or more variables are ordinal 26 Form of Correlation Linear Form Nonlinear, Monotonic Form 27 Form of Correlation Pearson (r): Measures linear relationships, where scores cluster around straight line Y changes in a consistent and constant way with X Used most with interval and ratio scale data Range = -1 to 1 Spearman rho (rs): Measures monotonic relationships, where there is a consistent directional relationship between x and y, but no amount of constant change Computed on rank values (smallest to largest) Used most with ordinal scale data Range = -1 to 1 28 Strength of Correlation The degree of association, or consistency, tells us the strength of the relationship (correlation) It is expressed mathematically as a correlation coefficient from -1.0 to +1.0 – The stronger the association, the closer to +/-1.0 -.80 and +.80 are equally strong +.20 and -.20 are equally weak Perfect consistency No consistency Perfect consistency -1.0 0 +1.0 Strength of consistency 29 Strength of Correlation Interpreting the Strength of the correlation in behavioral sciences: Degree of Relationship Value of the Correlation Coefficient No relationship r = -0.10 to 0.10 Weak relationship r =.10 to.30 (and -0.10 to -0.30) Moderate relationship r = 0.30 to 0.70 (and -0.30 to -0.70) Strong relationship r = 0.70 to 1.00 (and -0.70 to -1.00) Pearson and Spearman 30 Comparison of Spearman & Pearson Spearman correlation of 1 results when the two variables are monotonically related, even if their relationship is not linear. This means that all data points with greater x values than that of a given data point will have greater y values as well. 31 Comparison of Spearman & Pearson When the data are roughly elliptically distributed (variance on both factors) and there are no prominent outliers, the Spearman correlation and Pearson correlation give similar values. 32 Comparison of Spearman & Pearson Both Pearson and Spearman fail (yield values close to 0): Nonmonotonic relationships 33 Name that Correlation Which relies on monotonicity? Both Which relies on a linear relationship? Pearson Which is robust to outliers? Spearman For which does -1 mean perfect Both disagreement between the variables? 34 Strength of Correlation Can tell strength by “tightness” of envelope around scores – As relationship increases, circle flattens until flat (line = perfect) 7 7 8 6 6 7 6 5 5 5 4 4 4 3 3 3 2 2 2 1 1 1 0 0 0 0 2 4 6 0 2 4 6 0 2 4 6 Perfect positive Moderate positive Weak positive 7 7 7 6 6 6 5 5 5 No correlation 4 4 4 3 3 3 2 2 2 1 1 1 0 0 0 0 2 4 6 0 2 4 6 0 2 4 6 Perfect negative Moderate negative Weak negative 35 Correlation: Outliers on a Scatterplot A data point that stands apart from the pack Can be an outlier on X variable or on Y variable Outliers can greatly affect the strength of the correlation 36 Correlation: Outliers on a Scatterplot Outlier on Y variable: r =.00003 Outlier removed: r =.59 (significant) 10 10 8 8 6 6 4 4 2 2 0 0 0 5 10 15 0 5 10 15 Outlier = data point that differs significantly from others in set Common to define outliers by the variance in the variable: what is the standard deviation of the set of points are there values > 2-3 standard deviations from the mean 37value Outliers in Spearman & Pearson Correlations The Spearman correlation is less sensitive to (less affected by) outliers than is the Pearson correlation. That is because Spearman's ρ reassigns outliers with a rank, and ranks cannot be outliers. Outlier Example: X values = -2.1, -2, -1.9, -1.8, -1.7, -1.6, -1.5, -1, -.5, 0, 1, 6 X ranks = 1, 2, 3, 4, 5, 6, 7, 8, 9, 10, 11, 12 38 Correlation: Strength & Direction Accuracy of predictive value 7 7 7 6 6 6 5 5 5 4 4 4 3 3 3 2 2 2 1 1 1 0 0 0 0 2 4 6 0 2 4 6 0 2 4 6 Perfect Positive Weak Negative Perfect Negative 8 7 7 7 6 6 6 5 5 5 4 4 4 3 3 3 2 2 2 1 1 1 0 0 0 0 2 4 6 0 2 4 6 0 2 4 6 Weak Positive Moderate Negative Moderate Positive 39 Correlation: Significance Index of believability or meaningfulness of relationship – Is it a fluke? Statistical significance suggests a relationship is unlikely to be the result of chance (typically p <.05) – Probability (alpha) is < 5% that this correlation would have been this large (or larger) due to chance alone – Most likely represents a real relationship that exists in the population 40 Correlation: Significance Small sample sizes are prone to producing large correlations, so the criteria for statistical significance becomes more stringent As n increases, so does the likelihood that the relationships found actually exist Statistical significance is determined by consulting a table – The table takes into account sample size and alpha (p) level 41 Correlation: Significance For a correlation, Level of Significance(p) the degrees of for Two-TailedTest freedom (df) = n.05.01 sample size - 2 1.997.999 2.950.990 (number of 3.878.959 variables) 4.811.917 5.754.874 Ex: sample size (n) 6.707.834 7.666.798 = 10, p =.05. 8.632.765 9.602.735 What size does r 10.576.708 need to be to reach significance? To be significant, r must be equal to or larger than the value corresponding to the df and p level 42 Correlation: Significance Level of Significance (p) for 2-tailed test n p =.05 p =.01 30 0.349 0.449 Ex: sample size (n) = 100, p =.05. 35 0.325 0.418 40 0.304 0.393 What size does r need to be to reach 45 0.288 0.372 significance? 50 0.273 0.354 60 0.250 0.325 70 0.232 0.303 80 0.217 0.283 90 0.205 0.267 100 0.195 0.254 150 0.159 0.208 300 0.113 0.148 43 Correlation: Significance Statistical significance: – Related to p-value associated with n, df, size of correlation – Possible to have a small r but it can be statistically significant if the sample size is big enough Practical significance: – Related to any meaningful, real-world consequences of the observed correlation - A statistically significant difference for an r-value of.11 (n=300) might not be practically significant because it only explains a small portion of actual behavior 44 Examples of Correlations with Small N Easy to obtain strong correlations with small samples when there is no relationship between the variables – When N=2, always get correlation of 1.0 or -1.0 – As sample size increases, it becomes more likely that correlation from the sample reflects real relationship in the population 20 20 n=2 n=6 15 Variable Y 15 Variable Y 10 10 5 r = -1.0 5 r = -0.81 0 0 0 5 10 0 5 10 Variable X Variable X 45 Coefficient of Determination: To determine what percentage of changes in one variable (X) can be accounted for by changes in the other variable (Y), one must calculate the shared variance – The shared variance is the shared common ground between variables X and Y Important: – Correlation values (r) are ordinal Do not increase in equal increments Example: an r of.80 is not twice as strong as r of.40 46 Coefficient of Determination Correlations help explain some part of the variability in X, Y scores: – Other (different) variables can also explain variability in X, Y Venn Diagrams show the proportion of variability shared by two variables (X and Y) The larger the degree of overlap, the greater the strength of the correlation X Y X Y A little relationship More related 47 Coefficient of Determination r2: “Proportion of Shared Variance” r2: “Variance Accounted For” r2: “Coefficient of Determination” X Y X Y r =.0 r =.50 r2 =.0 X Y r2 =.25 25% shared variance 0% shared variance r =.90 r2 =.81 81% shared variance 48 Coefficient of Determination r2 is always positive and does not tell you whether r is positive or negative Positive Correlation Negative Correlation between X, Y between X, Y X Y X Y r = 0.90 r = - 0.90 r 2 = 0.81 r 2 = 0.81 81% shared variance 81% shared variance What is the square root of.81?.9 or -.9 49 Statistical Evaluation of Correlations Interpreting a Correlation – Coefficient of determination: the r-squared value of a correlation – Measures the percentage of variability in one variable that is determined by its relationship with the other variable Value of the Correlation Coefficient, or Degree of Relationship Coefficient of Determination Small r = 0.10 or -0.10; r2 = 0.01 (1%) Medium r = 0.30 or -0.30; r2 = 0.09 (9%) Large r = 0.70 or -0.70; r2 = 0.49 (49%) 50 Interpreting Coefficient of Determination Sometimes 3% of the variance is a lot! – Sometimes it is meaningless In behavioural sciences, it is usual to predict only a small proportion of the variance (< 70% of the variance) 51 Advantages of Correlational Methods Often quick and efficient Often the only method available – For practical reasons (cannot assign or vary personality) – For ethical reasons (cannot get strong levels of some variables) e.g., anxiety High external validity – Reflects natural events being examined 52 Limitations of Correlational Methods Does not tell us why two variables are related – Low internal validity – Very sensitive to outliers – Directionality problem (order of effects) – Third-variable problem – If 2 variables are related, is there a 3rd (unidentified) variable responsible for producing changes in X and Y? 53 Directionality Problem with Correlations Cooking shows on TV Eating behaviors of that individuals choose those individuals to watch Which came first? 54 Third-Variable Problem with Correlations Ice cream sales and crime rate tend to vary together. No direct connection between these two variables. Both are influenced by the outdoor temperature. https://www.nytimes.com/2009/06/19/nyregion/19murder.html?page https://www.lancaster.ac.uk/lums/news wanted=all&_r=0 /archive/explainer-how-does-the- weather-affect-the-economy 55 Assuming Directionality From Japanese study (Ukai et al, 2020, Heart): Correlations 30,076 adults, 40–59 years, no history of Cardiovascular disease Bathing frequency/week: without cardiovascular disease 0-2 times/week, 3-4 times/week, Percentage of individuals Almost every day (7) Baths/week 7 3-4 0-2 Frequency of tub bathing was associated with lower risk of CVD among adults 56 Assuming Directionality From Correlations Media coverage of Japanese bathing-heart correlations: Issues: People with lower-stress lifestyle may have time to take more baths Socioeconomic status may influence bathing rates and eating patterns High-temperature baths can exacerbate cardiovascular disease 57 Timepoint-based Correlations 1) Cross-sectional correlations 2) Cross-lag correlations 3) Autocorrelations All 3 correlation types are computed similarly Same measures of strength, direction, significance 1) Cross-Sectional Correlations Tests of whether 2 variables measured at the same timepoint are related to each other First half of lecture: examples based on time were cross-sectional correlations Example: jumping rope Parent TIME 1 talk TIME 2 talk TIME 3 talk TIME 4 talk Child TIME 1 move TIME 2 move TIME 3 move TIME 4 move 2) Lag Cross-Correlations: Tests whether a variable at an EARLIER timepoint is associated with another variable at a LATER timepoint Called "Temporal precedence" Parent TIME 1 talk TIME 2 talk TIME 3 talk TIME 4 talk Child TIME 1 move TIME 2 move TIME 3 move TIME 4 move Parent's talk at Time X precedes Child's motion at Time Y Difference between Time X and Time Y = “Lag” 2) Lag Cross-Correlations: Example: How do infants learn language? Parent behavior: Parent names an object at time X Infant behavior: Infant turns head to look at object at time (X + Lag) What the parent sees: What the child sees: Measures of Eye Gaze direction used to compute Lag 2) Lag Cross-Correlations: Example: How do infants learn language? Parent-time minus Infant-time (seconds) Negative Lag values = Parent's naming time occurs 5-10 seconds before infant's response Infants' response is slow Sun et al, 2022 (Infancy) 2) Lag Cross-Correlations: Tests of whether a variable at an EARLIER timepoint is associated with another variable at a LATER timepoint This time, change which variable precedes the other Example: parent soothes crying infant Parent TIME 1 talk TIME 2 talk TIME 3 talk TIME 4 talk Child TIME 1 cry TIME 2 cry TIME 3 cry TIME 4 cry Child's behavior precedes Parent's talk 3) Autocorrelations: Test whether a single variable at one timepoint is related to the same variable at another timepoint Example: parental soothing behavior over time Parent TIME 1 talk TIME 2 talk TIME 3 talk TIME 4 talk Lag-1 Lag-2 Lag-3 Positive autocorrelation = parent’s soothing increases over time Negative autocorrelation = parent’s soothing reduces over time 3) Autocorrelations: Examples: Circadian rhythms in cognition and physiology Circadian = repeating pattern over 24 hours Body Temperature Selective Attention Sustained Attention Black et al (2019) Valdez et al (2019) 3) Autocorrelations: Examples: Circadian = repeating pattern over 24 hours will yield Negative auto-correlation at 12-hour lag Positive auto-correlation at 24-hour lag 2 days of clock time (hours) 24-hour lag: 12-hour lag: Positive Autocorr = Large (Negative) Black et al (2019) 3) Autocorrelations: Example: Depressive Symptoms in Bipolar Disorder characterized by alternating periods of low mood, high mood Repeating every 5-6 weeks Depression Symptoms Patients’ weekly 20 depression symptoms 10 0 1 6 11 16 21 26 Time (# weeks) Moore et al (2014) 3) Autocorrelations: A negative autocorrelation A positive autocorrelation 20 Depression 20 Symptoms Depression Symptoms 10 10 0 0 1 6 11 16 21 26 1 6 11 16 21 26 Shifted 3 Shifted 6 events later events later 1 6 11 16 21 26 1 6 11 16 21 26 Autocorrelations 1 0.5 Correlation 0 1 2 3 4 5 6 7 8 9 10 -0.5 -1 Lag Size Lag-3 Negative Autocorrelation: Lag-6 Positive Autocorrelation: Positive value at time X = Positive value at time X = Negative correlation at time X+3 Positive correlation at time X+6 Depression at week 1 = Depression at week 1 = less depressed at week 1+3 more depressed at week 1+6 Correlations and Outcomes What information do these correlations provide? If a cross-sectional correlation is significant: One variable covaries with the other variable If a lagged cross-correlation is significant: One variable has temporal precedence over the other variable If an autocorrelation is significant: One variable shows regular change over time Caveat: none of these correlations completely indicate causality NEXT TIME: CHAPTER 7 EXPERIMENTAL RESEARCH STRATEGY 70