Certified Analytics Professional Examination Study Guide PDF
Document Details
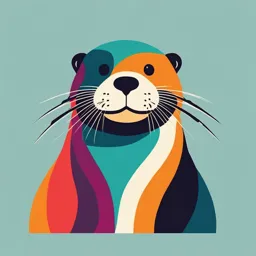
Uploaded by GoodlyTiger
Scott Nestler
Tags
Summary
This study guide is for the Certified Analytics Professional (CAP®) and Associate Certified Analytics Professional (aCAP™) examination. It provides information on central concepts in the CAP® program, and details of the examination.
Full Transcript
Certified Analytics Professional EXAMINATION STUDY GUIDE ® aCAP ™ CE RT I F I E D A N A LY T I C S PR O F E S S I O N AL ( C A P®) & AS S O C I AT E C E RT I...
Certified Analytics Professional EXAMINATION STUDY GUIDE ® aCAP ™ CE RT I F I E D A N A LY T I C S PR O F E S S I O N AL ( C A P®) & AS S O C I AT E C E RT I F I E D A NALYT ICS P RO F E S S I O N A L (aC A P™) EXA M I N AT I O N S T U D Y GUID E 5521 Research Park Drive, Suite 200, Catonsville, MD 21228 USA 855-249-2589 ACKN OW L ED G E M E NT S We are pleased to publish this first study guide for the CAP program. It would not have been possible without the work of the Study Committee (listed) and the comments from the many reviewers of the draft guide. STUDY GUIDE MEMBERS: Alan Taber, CAP (Lockheed Martin) – Co-chair Subhashish Samaddar, CAP (Georgia State University) – Co-chair Robert Bordley, CAP (Booz Allen Hamilton) Rami Musa, CAP (Dupont) Mike Smith, CAP (ICFI) Frank Stein, CAP (IBM) Cat Truxillo, CAP (SAS) Zachary Waltz, CAP (IBM) 2 FOR E W OR D As chair of the Analytics Certification Board, I congratulate the Study Guide committee on having assembled in short order such a comprehensive study guide for the Certified Analytics Professional (CAP®) program. I know the guide is not going to satisfy everyone or directly provide them with answers for the test. It isn’t designed to do so. It is designed to provide some information on central concepts embedded in the CAP program. It is up to the individual to determine his/her familiarity with the concept and decide whether more review or study on that topic is warranted. The examination has 100 multiple choice test questions for each of which there is only one correct answer. The questions are both vendor and software neutral, designed to confirm that the test taker has the underlying knowledge necessary to know which steps to follow in an analytics process and to select the correct tools. The exam covers seven domains or areas of analytics practice: business problem framing, analytics problem framing, data, methodology (approach) selection, model building, deployment, and model life cycle management. A sample of the type of questions is available with this guide and can also be accessed through the Candidate Handbook. These sample questions will never appear on an exam. Each sample gives not only the correct answer but also provides rationale for why each is (in)correct. What are individual benefits of the Certified Analytics Professional program? Advances your career by setting you apart from the competition Promotes personal satisfaction from accomplishing a key career goal Helps improve your overall job performance by setting you on a course for continual professional development Recognizes the investment you have made in your career Demonstrates commitment to the field What are employer benefits of the Certified Analytics Professional program? Helps with identifying and developing qualified analytics professionals Proves to stakeholders that your organization follows industry-standard analytics practice Provides a career path to encourage employees Useful as positive factor in responding to proposals Indicates a company willing to invest in its employees Indicates a willingness to maintain up to date knowledge You are to be applauded for seeking certification. While the exam is the most pressing hurdle to achieving the CAP, it is not the only criterion. The Certified Analytics Professional program depends on each of the Five E's: They are adherence to the Code of Ethics, Effective mastering of soft skills, acceptable levels of Experience and Education and finally, successfully passing the Exam. The result of this program is a well-rounded analytics professional who can work in many fields to provide analytic leadership and support. The Analytics Certification Board wishes all candidates complete success in their certification process. If I, or they, can be of help, feel free to contact me at [email protected] or email our Certification Manager at [email protected]. Scott Nestler, US Army Chair Analytics Certification Board 3 TAB LE OF C O NT E NT S CHAPTER 1: INTRODUCTION TO THE CAP® PROGRAM......................................................... 7 About the Professional Job Task Analysis............................................................................................... 9 The Five E’s.............................................................................................................................................. 14 CHAPTER 2: DOMAIN I – BUSINESS PROBLEM FRAMING................................................... 17 What will you learn in this chapter?....................................................................................................... 17 Learning Objectives................................................................................................................................ 17 Key Concepts/Fundamentals................................................................................................................. 17 Objective 1. Receive and refine the business problem.................................................................. 17 Objective 2. Identify stakeholders.................................................................................................... 18 Objective 3. Determine whether the problem is amenable to an analytics solution................... 19 Objective 4. Refine problem statement and delineate constraints............................................... 19 Objective 5. Define an initial set of business benefits.................................................................... 20 Objective 6. Obtain stakeholder agreement on the problem statement..................................... 20 Summary.................................................................................................................................................. 20 Further reading........................................................................................................................................ 21 CHAPTER 3: DOMAIN II – ANALYTICS PROBLEM FRAMING............................................... 23 What will you learn in this chapter?....................................................................................................... 23 Learning Objectives................................................................................................................................ 23 Key Concepts/Fundamentals................................................................................................................. 23 Objective 1. Reformulating the business problem statement as an analytics problem............... 23 Objective 2. Develop a proposed set of drivers and relationships to outputs............................. 25 Objective 3. State the set of assumptions related to the problem............................................... 26 Objective 4. Define the key metrics of success............................................................................... 27 Objective 5. Obtain stakeholder agreement................................................................................... 27 Summary of key terms....................................................................................................................... 28 Summary.................................................................................................................................................. 28 Further reading........................................................................................................................................ 28 CHAPTER 4: DOMAIN III – DATA...................................................................................................... 31 What will you learn in this chapter?....................................................................................................... 31 Learning Objectives................................................................................................................................ 31 Key Concepts/Fundamentals................................................................................................................. 31 Objective 1. Identify and prioritize data needs and resources...................................................... 31 Objective 2. Identify means of data collection and acquisition..................................................... 33 4 Objective 3. Determine how and why to harmonize, rescale, clean and share data................... 41 Objective 4. Identify ways of discovering relationships in the data............................................... 46 Objective 5. Determine the documentation and reporting of findings........................................ 49 Objective 6. Use data analysis results to refine business and analytics problem statements..... 49 Summary.................................................................................................................................................. 49 Further Reading....................................................................................................................................... 50 CHAPTER 5: DOMAIN IV – METHODOLOGY (APPROACH) SELECTION......................... 51 What will you learn in this chapter?....................................................................................................... 51 Learning Objectives................................................................................................................................ 51 Objective 1. Identify available problem solving approaches......................................................... 51 Objective 2. Select software tools.................................................................................................... 54 Objective 3. Model testing approaches*......................................................................................... 59 Objective 4. Select approaches*...................................................................................................... 60 Summary.................................................................................................................................................. 60 Further Reading....................................................................................................................................... 60 CHAPTER 6: DOMAIN V – MODEL BUILDING........................................................................... 61 What will you learn in this chapter?....................................................................................................... 61 Learning Objectives................................................................................................................................ 61 Objective 1. Identify model structures............................................................................................. 61 Objective 2. Evaluate and calibrate models and data.................................................................... 63 Objective 3. Calibrate models and data*........................................................................................ 64 Objective 4. Integrate the models*.................................................................................................. 65 Summary.................................................................................................................................................. 65 Further Reading....................................................................................................................................... 65 CHAPTER 7: DOMAIN VI – SOLUTION DEPLOYMENT........................................................... 67 What will you learn in this chapter?....................................................................................................... 67 Learning Objectives................................................................................................................................ 67 Objective 1. Perform business validation of the model.................................................................. 68 Objective 2. Deliver report with the findings................................................................................... 68 Objective 3. Create model, usability, and system requirements for production.......................... 68 Objective 5. Support Deployment................................................................................................... 69 Summary.................................................................................................................................................. 70 Further reading........................................................................................................................................ 70 * Tasks performed by analytics professionals beyond CAP® 5 TAB LE OF C O NT E NT S CHAPTER 8: DOMAIN VII – MODEL LIFECYCLE........................................................................ 71 Learning Objectives................................................................................................................................ 71 Objective 1. Document initial structure........................................................................................... 71 Objective 2. Track model quality...................................................................................................... 72 Objective 3. Recalibrate and maintain the model*......................................................................... 72 Objective 4. Support training activities............................................................................................ 73 Objective 5. Evaluate the business benefit of the model over time.............................................. 73 Summary.................................................................................................................................................. 73 Further reading........................................................................................................................................ 74 APPENDIX A: SOFT SKILLS FOR THE ANALYTICS PROFESSIONAL.................................. 75 Introduction............................................................................................................................................. 75 Learning Objectives................................................................................................................................ 75 Task 1: Talking intelligibly with stakeholders who are not fluent in analytics..................................... 75 Task 2: Client/employer background & focus....................................................................................... 77 Task 3: Clarifying the analytics process.................................................................................................. 78 Summary.................................................................................................................................................. 79 Further reading........................................................................................................................................ 79 APPENDIX B: USING THE STUDY GUIDE TO HELP PREPARE FOR THE CAP® EXAM... 81 GLOSSARY................................................................................................................................................. 83 REVIEW QUESTIONS........................................................................................................................... 115 Answers to Review Questions.............................................................................................................. 130 Study Guide References for Specific Domains................................................................................... 136 Further reading...................................................................................................................................... 137 List of Figures Figure 1: Possible shapes of analytics knowledge (OR/MS Today, June 2013) Figure 2: Kano’s Requirements Model (used under Creative Commons, http://creativecommons.org/ licenses/by-nc-nd/3.0/us/) Figure 3: Input Table by Alan Taber, CAP® (used with permission) Figure 4: Black Box Sketch by Alan Taber, CAP® (used with permission) Figure 5: INFORMS CAP® Methodology Classification (used with permission) Figure 6: Sample software application characteristics by Rami Musa, CAP® (used with permission) 6 * Tasks performed by analytics professionals beyond CAP® CHA PTE R 1 INTRODUCTION TO THE CAP® PROGRAM The Institute for Operations Research and the Management Sciences (INFORMS) is an international scientific society with more than 11,000 members, including Nobel Prize laureates, dedicated to applying scientific methods to help improve decision making, management, and operations. Members of INFORMS work in business, government, and academia. INFORMS serves the scientific and professional needs of operations research analysts, experts in analytics, consultants, scientists, students, educators, and managers, as well as their institutions, by publishing a variety of journals that describe the latest research in operations research. INFORMS commissioned a study in 2010 by Capgemini Consulting to evaluate the need for embracing the analytics community as a key membership strategy for the Institute. The Capgemini study concluded that INFORMS should embrace the analytics community and that one of the first initiatives should be a detailed study on the development of a certification and training program to meet the needs of this market. Further market research corroborated this finding. Mike Hamm of Michael Hamm and Associates wrote of his market research—done in 2011 on behalf of INFORMS—that “I have never seen a candidate audience where [there is such] a high degree of political interest regarding the potential composition and architecture of a future certification program. Everybody wants a piece of this....” INFORMS defines analytics as the scientific process of transforming data into insight for making better decisions. It is seen as an end-to-end process beginning with identifying the business problem to evaluating and drawing conclusions about the prescribed solution arrived at through the use of analytics. Analytics professionals are skilled at this process. INFORMS established the analytics certification program to advance the use of analytics by setting agreed upon standards for the profession. The program also advances the analytics profession by providing a means for organizations to identify and develop qualified analytics professionals, by contributing to the career success and continued competence for analytics professionals, and by improving the credibility and visibility of the analytics profession. INFORMS vision for the Certified Analytics Professional (CAP®) program is to advance the use of analytics to transform the world by setting agreed-upon standards for the profession. The INFORMS mission for the CAP® program is 7 to advance the analytics profession by providing a high-quality program of certification and by promoting continuing competence for practitioners. Once INFORMS decided to pursue a certification program, the practicalities of creating the program and its accompanying exam were addressed. According to an article in Analytics Magazine, September/October 2012 by Scott Nestler, Jack Levis, and Bill Klimack, the CAP® program is appropriate for the analytical semi- professionals as well as the analytical professionals. However, it will not be a suitable certification for the “analytical amateurs” as depicted in the following graphic (Nestler et al. 2012, Figure 2). The assessment instrument—the exam—contains 100 multiple choice test items and is being administered via paper and pencil for the first year. INFORMS is investigating the possibility of moving to computer-based testing for subsequent years to facilitate serving its international membership. CAP® (a) (b) (c) (d) (e) Analyst Type Semi- Amateur Professional Professional Professional (Davenport) professional Shape "bar-shaped" "bar-shaped" "I-shaped" "T-shaped" "Pi-shaped" Figure 1: Possible shapes of analytics knowledge For more information on the development of the CAP program, read “Steering Toward Analytics” in OR/MS Today, June 2013 (p. 30) by Gary Bennett and Jack Levis. 8 ABOUT THE PROFESSIONAL JOB TASK ANALYSIS A job task analysis (JTA) is a comprehensive description of the duties and responsibilities of a profession, occupation, or specialty area; our approach consists of four elements: 1) domains of practice, 2) tasks performed, 3) knowledge required for effective performance on the job, and 4) domain weights that account for the importance of and frequency with which the tasks are performed. More specifically, the JTA for the CAP® program can be viewed as an outline of a partial body of knowledge, as it represents a delineation of common or typical tasks performed and knowledge applied by analytics professionals, grouped together in a hierarchical domain structure. In the course of analytics work, these tasks may be performed multiple times with modifications based on data, findings, and results, as part of ongoing feedback loops that are routinely a part of practice. The JTA serves as the test blueprint for exam development and links what is done on the job to what is measured by the certification examination. This linkage is necessary to establish a valid, practice-related examination. It is important to realize that the JTA is a dynamic document that will change in the future to reflect best practices and changes in the analytics profession. The JTA study defines the current knowledge, skills, and abilities (KSAs) that must be demonstrated by analytics professionals to effectively and successfully provide these services. KSAs are validated according to their frequency of use and importance. The JTA also serves as a “blueprint” for the content (performance domains) of the INFORMS CAP® examination. INFORMS upholds stringent guidelines for the construction and implementation of the examination development and administration process. An 11-member panel of subject matter experts (SMEs) was selected to develop the first JTA for the CAP® credential. This group was called the Analytics Certification Job Task Analysis Working Group. The following leaders in the analytics profession were selected to participate in this important project: Arnold Greenland (IBM Global Business Scott Nestler (Naval Postgraduate School) Services) Jerry Oglesby (SAS) Bill Klimack (Chevron) Michael Rappa (North Carolina State/ Jack Levis (UPS) Institute for Advanced Analytics) Daymond Ling (Canadian Imperial Bank of Tim Rey (Dow Chemical) Commerce) Rita Sallam (Gartner) Freeman Marvin (Innovative Decisions, Inc.) Sam Savage (Stanford/Vector Economics) 9 The findings of this working group were then validated by a random sample of practicing analytics professionals. Feedback from this survey resulted in slight modifications of the performance domains, tasks, and knowledge that comprise the test blueprint that determines the content of the CAP® examination. In developing the JTA, members of the working group relied on their knowledge of practice gained from years of experience, academic program content, corporate job descriptions in analytics, and articles from professional and scholarly publications. The following table includes the final domains and weights derived from the JTA and a review of validation survey recommendations. Domain Approximate Weight I. Business Problem (Question) Framing 12%–18% II. Analytics Problem Framing 14%–20% III. Data 18%–26% IV. Methodology (Approach) Selection 12%–18% V. Model Building 13%–19% VI. Deployment 7%–11% VII. Model Life Cycle Management 4%–8% The INFORMS CAP® examination is based on the following test blueprint derived from the JTA process. The final agreed-upon weights reflect the percentage of questions from each domain that will be included in each test form. The JTA and the test blueprint resulting from this process will be reviewed periodically and updated as needed to reflect current practices in analytics. The list of domains and key tasks follows: (12%–18%) Domain I Business Problem (Question) Framing (The ability to understand a business problem and determine whether the problem is amenable to an analytics solution.) T-1 Obtain or receive problem statement and usability requirements T-2 Identify stakeholders T-3 Determine whether the problem is amenable to an analytics solution T-4 Refine the problem statement and delineate constraints 10 T-5 Define an initial set of business benefits T-6 Obtain stakeholder agreement on the business problem statement (14%–20%) Domain II Analytics Problem Framing (The ability to reformulate a business problem into an analytics problem with a potential analytics solution.) T-1 Reformulate problem statement as an analytics problem T-2 Develop a proposed set of drivers and relationships to outputs T-3 State the set of assumptions related to the problem T-4 Define key metrics of success T-5 Obtain stakeholder agreement on the approach (18%–26%) Domain III Data (The ability to work effectively with data to help identify potential relationships that will lead to refinement of the business and analytics problem.) T-1 Identify and prioritize data needs and sources T-2 Acquire data T-3 Harmonize, rescale, clean, and share data T-4 Identify relationships in the data T-5 Document and report findings (e.g., insights, results, business performance) T-6 Refine the business and analytics problem statements (12%–18%) Domain IV Methodology (Approach) Selection (The ability to identify and select potential approaches for solving the business problem.) T-1 Identify available problem solving approaches (methods) T-2 Select software tools T-3 Test approaches (methods)* T-4 Select approaches (methods)* (13%–19%) Domain V Model Building (The ability to identify and build effective model structures to help solve the business problem.) T-1 Identify model structures* T-2 Run and evaluate the models T-3 Calibrate models and data* T-4 Integrate the models* T-5 Document and communicate findings (including assumptions, limitations, and constraints) *Tasks that are beyond the scope of the CAP® 11 (7%–11%) Domain VI Deployment (The ability to deploy the selected model to help solve the business problem.) T-1 Perform business validation of the model T-2 Deliver report with findings; or T-3 Create model, usability, and system requirements for production T-4 Deliver production model/system* T-5 Support deployment (4%–8%) Domain VII Model Life Cycle Management (The ability to manage the model life cycle to evaluate business benefit of the model over time.) T-1 Document initial structure T-2 Track model quality T-3 Recalibrate and maintain the model* T-4 Support training activities T-5 Evaluate the business benefit of the model over time The knowledge statements for the CAP® program have been identified but not individually assigned to each task. The knowledge statements appropriate to a given task have been used. Not all statements are appropriate for all tasks, although there may appear to be some blanks in coverage this is not the case. K-1 Characteristics of a business problem statement (i.e., a clear and concise statement of the problem describing the situation and stating the desired end state or goal) K-2 Interviewing (questioning) techniques (i.e., the process by which a practitioner elicits information and understanding from business experts, including strategies for the success of the project) K-3 Client business processes (i.e., the processes used by the client or project sponsor that are related to the problem) K-4 Client and client-related organizational structures K-5 Modeling options (i.e., the analytic approaches available for seeking a solution to the problem or answer to the question including optimization, simulation, forecasting, statistical analysis, data mining, machine learning, etc.) K-6 Resources necessary for analytics solutions (e.g., human, data, computing, software) 12 *Tasks that are beyond the scope of the CAP® K-7 Performance measurement (i.e., the technical and business metrics by which the client and the analyst measure the success of the project) K-8 Risk/return (i.e., trade-offs between prioritizing the primary objective and minimizing the likelihood of significant penalty taking into account therisk attitude of the decision maker) K-9 Presentation techniques (i.e., strategies for communicating analytics problems and solutions to a broad audience of business clients) K-10 Structure of decisions (e.g., influence diagrams, decision trees, system structures) K-11 Negotiation techniques (i.e., strategies and methods that allow the analytics professional to reach a shared understanding with the client) K-12 Data rules (e.g., privacy, intellectual property, security, governance, copyright, sharing) K-13 Data architecture (i.e., a description of how data are processed, stored, and used in organizational systems including conceptual, logical, and physical aspects) K-14 Data extraction technologies (e.g., scripting, spreadsheets/ databases,connection tools, standards-based connectivity options, unstructured data extraction tools) K-15 Visualization techniques (i.e., any technique for creating images, diagrams or animations to communicate a message including data visualization, information visualization, statistical graphics, presentation graphics, etc.) K-16 Statistics (descriptive, correlation, regression, etc.) K-17 Software tools 13 THE FIVE E’S The five E’s are ethics, education, experience, examination, and effectiveness. These are the five pillars of the Certified Analytics Professional. The CAP® credentialed person will have read, agreed to, and signed the code of ethics that governs behavior of a professional analyst. This code was created by the Task Force who are among the originators of the program (see Figure 1). The code is intended to describe the accepted behavior of an analytics professional. All candidates for the CAP® must agree to the code of ethics as part of the application process. Actions that are opposed to the code of ethics may be reason to rescind the CAP® credential. Education is considered essential for the analytics professional. Candidates must have at least a bachelor’s degree from a regionally accredited college or university. Experience goes hand in hand with education as part of the prerequisites for application. The higher and more appropriate the education earned, the less experience is required. Examination is the fourth leg or pillar of the CAP® program. Through examination we seek confirmation that the applicant has knowledge of those areas of the job/ task analysis that are considered essential for practice. Because the examination is based on a broad spectrum of practice rather than the content of a course or series of courses, it must be constructed with due care. Each test item or question has been created carefully so as to ensure a fair, valid, and reliable examination that discriminates against no one except for those who do not have the knowledge to earn the CAP® credential. Each item is reviewed and refined numerous times by a committee of subject matter experts in the field of analytics. The sole reason to use a test item is as a tool to determine who is knowledgeable. Because there may be a lot riding on the successful completion of the exam, the test items must be carefully crafted. All test items are written with reference to the specific domain, task, and knowledge statements outlined earlier. Test items are also sourced to ensure that all items are readily available and should be known to everyone who is an analytics professional. No items are written based on proprietary data or sources that are known only to a select few. For examples, see the Candidate Handbook that contains 24 questions or items that are indicative of the style of test item but that do not themselves appear on the exam. In the future, there may be additional items that we will release from the item bank and use as practice test questions. The CAP® program is so new that INFORMS does not yet have items that have outlasted their usefulness as a discriminatory tool to distinguish between the knowledgeable and those who do not yet possess the knowledge. 14 The rules for item writing are specific and few: Avoid negative stems or questions as much as possible Do not use ‘All of the above’ or ‘None of the above’ as answer options Avoid excess verbiage Avoid disadvantaging any part of the test population but the unknowing Ask only one question at a time Ensure that the incorrect answers are incorrect for a specific reason Effectiveness is the art of applying your knowledge and skill in a way that enables achievement of your organization’s goals. The soft skills required are dealt with more fully in Appendix A: Soft Skills. Nevertheless, the skilled analyst must be diplomatic and aware enough to understand the context of the business problem and the stakeholder agendas involved while not allowing that understanding to bias the process or the truth thereby developed. The Certified Analytics Professional (CAP®) program is not the work of one person or one department: it would not have been possible without the support of professionals in the field. You can see a long list of those professionals on the INFORMS website under Contributors (www.informs.org/Certification-Continuing- Ed/Analytics-Certification/Contributors). This study guide is the culmination of a massive collaborative effort among concerned professionals to develop a guide that will assist future CAPs. The guide is not intended to give the answer to each and every test question. Rather, it is intended to guide the individual toward the knowledge of an analytics professional and to let the individual use his or her discretion as to areas that warrant further study. The guide includes reference materials for this further study. The guide is also intended to be a comprehensive outline for those who are working in, intend to work in, or are preparing to work in an analytics area. Because being an effective analytics professional is as much of an art as a science, the study guide relies heavily on case studies, examples, and stories. If you have comments on the guide, the certification program, or wish to assist with the further development and dissemination of the CAP® program, please feel free to e-mail [email protected]. 15 TH I S PA GE IS DE LIBE RATELY LEF T BLANK CHA PTE R 2 DOMAIN I – BUSINESS PROBLEM FRAMING WHAT WILL YOU LEARN IN THIS CHAPTER? In this chapter, you will learn about the first step of an analytics project: framing the business problem. You will learn, as a part of these processes, how to determine the business problem, identify and enlist stakeholders, determine if the problem has an analytics solution, refine the problem statement as necessary, and define the set of business benefits. Learning Objectives 1. Obtain or receive the problem statement and usability requirements 2. Identify stakeholders 3. Determine whether the problem is amenable to an analytics solution 4. Refine the problem statement and delineate constraints 5. Define an initial set of business benefits 6. Obtain stakeholder agreement on the business problem statement Key Concepts/Fundamentals OBJECTIVE 1. RECEIVE & REFINE THE BUSINESS PROBLEM A business problem statement generally starts by describing a business opportunity or threat, or an issue in broad terms. For example, it could simply start by saying 'our growth has been stagnant for the last two years' or a bit less broad 'our Seattle plant is experiencing production problems and is missing deadlines.' Most client firms in their early meetings with you (the analytics professional) will tend to report what they are experiencing as problems. As they do that, they will use their own language and key terms. Do get definitions of all terms, as meanings change between organizations. Another factor to consider is that the client firm representatives in these meetings also play an important role in what is reported and how it is reported. It is natural that each representative (of the firm) uses their own lenses and contexts to report (and thus frame) the way they see the problem. These views are all very important on their own merits because they inform the analyst in some useful way. However, - - 17 because of the individual lenses used to report these observations, sometimes these views can have a good degree of variance regarding causes and effects, and thus may obscure the real issues. One popular way to frame a business opportunity or problem is to obtain reliable information on the five W’s: who, what, where, when, and why. Who: are the stakeholders who satisfy one or more of the following with ⑳ respect to the project: funding, using, creating, or affected by the project’s - outcome. - - What: problem/function is the project meant to solve/perform? - & Where: does the problem occur? Or where does the function need to be performed? Are the physical and spatial characteristics articulated? - - When: does the- problem occur, or function need to be performed? When does the project need to be completed? -- Why: does the problem occur, or function need to occur? OBJECTIVE 2. IDENTIFY STAKEHOLDERS Of the five W’s, who (the stakeholders are) is probably the most critical to the long term success of the project. Stakeholders are anyone affected by the project, not - just those in the initial meetings, and they may have different levels of input or - involvement during the project. A stakeholder analysis helps identify the following: The interests of all stakeholders, who may affect or be affected by the - project, along with their constraints. Potential issues that could disrupt the project. - Key people for information distribution during execution phase. - Groups that should be encouraged to participate in different stages of - the project. Communication planning and stakeholder management strategies during - the project planning phase. Ways to reduce potential negative impacts and manage negative - stakeholders. 18 OBJECTIVE 3. DETERMINE WHETHER THE PROBLEM IS AMENABLE TO AN ANALYTICS SOLUTION Before more time and money is spent on solving the problem, it is time to figure out if this problem is likely to have an analytics solution. First of all, does the answer - and the change process to get there lie within the organization’s control? Second, - does the requisite data exist or can it be obtained? Third, can the likely problem be - solved and/or modeled? Last, but perhaps most importantly, can the organization - accept and deploy the answer? The problem may not be amenable to an analytics - solution because of the characteristics of the problem or the limitations of the analytic tools/methods available. The problem statement could be reassessed to - make it amenable to the available analytic tools/methods, or if this is not possible, the project deemed not feasible. If there isn’t a feasible way forward, the ethical analyst will say so to the key stakeholders. For the Seattle plant example, it may be decided to use mathematical optimization software to improve the plant’s process. This will work as long as data exist on inputs and outputs for each step in the plant process, and as long as the stakeholders are willing to accept new ways of operating that won’t necessarily match current work policies and procedures. OBJECTIVE 4. REFINE PROBLEM STATEMENT & DELINEATE CONSTRAINTS After the initial analysis, it may be necessary to refine the problem statement to - make it more accurate, more appropriate to the stakeholders, or more amenable to available analytic tools/methods. As part of this process, it will become necessary to define what constraints the project will operate under. These constraints could be analytical, financial, or political in nature. - - - For the Seattle plant example, an optimization problem with a large number of constraints or a complex objective function may not be solvable within the capability of the available software/hardware combination. In this case the problem may need to be restated with fewer constraints and/or a less complex objective function. This may cause the problem statement to be updated to make sure that the approach will satisfy—just to name a few of the potential constraints—desired accuracy and repeatability, program cost, timeframe, and number of stakeholders impacted, either positively or negatively. 19 OBJECTIVE 5. DEFINE AN INITIAL SET OF BUSINESS BENEFITS With the problem statement set, it is now possible to define the initial set of business benefits. These benefits may be determined quantitatively or qualitatively. If quantitative, it may be financial (e.g., net present value) or contractual (e.g., service level agreements). This is also known as the business case. For the Seattle plant example, an initial determination of the financial benefit due to optimal use of resources should be determined along with an initial view of the required project goals determined, e.g., plant is currently losing money at the rate of 3% of gross sales with current performance and needs to come to 5% margin on gross sales. The key profit driver is on-time performance, which is currently 68% and needs to get to 98%. How will it get there? At this stage we think it is because there is plant capacity being wasted, so we’re going to look at optimizing our scheduling and manufacturing processes to reduce overall time by reducing queue and wait time. You’ll note that we haven’t said, yet, that we’re going to simulate incoming orders with one distribution and performance of each machine on the floor with their own distributions, even though we may be thinking about doing just that. At this stage, the problem is a business problem and the objectives are business objectives. OBJECTIVE 6. OBTAIN STAKEHOLDER AGREEMENT ON THE PROBLEM STATEMENT With the problem statement refined and the initial business benefits determined, it is necessary to obtain stakeholder agreement before proceeding further with the project. It may be necessary to repeat this cycle several times until stakeholder concurrence with the particulars of the project are achieved and permission to proceed is granted. At the end of this process, you will have agreement on the project’s objectives, initial approach, and resources to get there. SUMMARY Although business problem framing is not the analytical heart of an analytics project, it is probably the most important because it sets the expectations and limitations of the project. 20 FURTHER READING Davenport T, Kim J (2013) Keeping up with the Quants: Your Guide to Understanding and Using Analytics (Harvard Business Review Press, Boston). Framing the problem at https://www.boundless.com/business/management/ decision-making/observation-framing-the-problem/. Kirkwood CW (1997) with Spreadsheets (Duxbury Press, Pacific Grove, CA). Lindstrom C (2009) How to write a problem statement, March 18, http://www. ceptara.com/blog/how-to-write-problem-statement. Nixon NW (2013) Focus first on framing, not solving, the problem, April 18, http:// philadelphia.regionsbusiness.com/print-edition-commentary/focus-first-on- framing-not-solving-the-problem/. Seelig T (2013) Shift your lens: The power of re-framing problems. Seelig T, ed. (HarperOne, New York), http://stvp. stanford.edu/blog/?p=6435. Spradlin D (2012) The power of defining the problem, September 25, http:// blogs.hbr.org/cs/2012/09/the_power_of_defining_the_prob.html. 21 TH I S PA GE IS DE LIBE RATELY LEF T BLANK CHA PTE R 3 DOMAIN II – ANALYTICS PROBLEM FRAMING WHAT WILL YOU LEARN IN THIS CHAPTER? This chapter is all about the dialogue between the business people who have a problem that they need to solve and the analytics folks who will give them the information required to solve the problem. This dialogue is mediated by the analytics professional (YOU) who is trusted by both sides because you are fluent in the language and culture of each side. As with any translation effort between two different groups, much of what follows are simple precepts to keep the sense of the business problem while decomposing it into actionable analytics pieces. Learning Objectives 1. Reformulate a problem statement as an analytics problem 2. Develop a proposed set of drivers and relationships to inputs 3. State the set of assumptions related to the problem 4. Define key metrics of success 5. Obtain stakeholder agreement on the approach Key Concepts/Fundamentals OBJECTIVE 1. REFORMULATING THE BUSINESS PROBLEM STATEMENT AS AN ANALYTICS PROBLEM There’s an apocryphal story of a Black & Decker sales convention. The VP of sales gets up to the dais, and says, “Folks, I have some bad news for you. We’ve done some detailed customer surveys to find out what our customers care about. They couldn’t care less about our carbide tips, or the voltage rating of our drills. In fact, they’d rather not think about drills at all! What our customers want is to hang a picture, or put up drywall, or do any number of other jobs. Our job is to help them do just that.” Similarly, your business and operational stakeholders likely could not care less about how you and your team are going to solve their problem. They just want it to be solved reliably and deliver the results. The first step is to decode the business problem statement to get to the analytics problem. There are many ways to do this, some more formal than others. In simple 23 terms, you are translating the “what” of the business problem into the “how” of the analytics problem. 1. What result do we want? 2. Who will act? 3. What will they do? 4. What will change in the organization as a result of the new information generated? For example, a company wishes to increase market share, but what is the underlying problem they need to address? Are they, for instance, emphasizing carbide-tipped drills to someone who only wants to hang a picture? One formal method of decomposition is quality function deployment (QFD) (http:// www.ieee.li/tmc/quality_function_deployment.pdf). This is a rigorous process that maps the translation of requirements from one level to the next, e.g., from the business level to the first analytics level, from the first analytics level to the second level, etc. Whether you are formally decomposing and parsing a complex business statement, or you are less formally brainstorming with a project sponsor, it is critically important to account for tacit as well as formal requirements. The best known model in this area is Kano’s requirements model (Figure 2). It distinguishes between unexpected customer delights, known customer requirements, and customer must-haves that are not explicitly stated. CUSTOMER SATISFACTION Often there are business or operational requirements that are taken for granted by those exciting requirements stakeholders that if not surfaced will normal requirements result in customer dissatisfaction, particularly items that come DON'T DO under the heading of “that’s FULFILL FULFILL the way we always do things.” EXPECTATIONS EXPECTATIONS Now, there are times when those expected requirements or assumptions need requirements to be challenged, but they can’t be challenged until they are brought to light. When you ask your CUSTOMER DISSATISFACTION business stakeholders for a list of what requirements they have, they will tend to focus on the “normal 24 requirements,” not the “expected requirements.” As the analytics professional charged with translating business requirements into the problem statement, you really need to probe to make sure that you have the entire appropriate context as well, including the expected requirements. OBJECTIVE 2. DEVELOP A PROPOSED SET OF DRIVERS & RELATIONSHIPS TO OUTPUTS These next three items are related. Your input/output functions are strongly related to your assumptions about what is important about this problem as well as the key metrics by which you’ll measure the organizational response to the problem. We’ll start by defining the input/output functions of the problem at hand. As with any of these areas, you can be as formal or informal as you like, but sketches and diagrams certainly help communicate with your stakeholders and help get everyone on the same page. Here’s a very simple example: An organization wants to predict the number of detected software defects over the next six months. That’s the output. The inputs would be elicited from stakeholder interviews, using questions like, “What future activities will add to our rate from where we are today?”, “What will decrease our rate from where we are today?”, “Will we add interfaces or components to the testing?”, “Will we materially change the size of the test team?”, etc. Bear in mind that you aren’t looking for causation at this stage, just ideas around which you’ll form some hypotheses against which you’ll test your model later. Once you have these inputs and a general sense of their predicted effects, you have a choice of how to communicate them to the team at large. A simple table (Figure 3) is one approach. A black box sketch (Figure 4) is another approach. How you do it isn’t nearly as important as doing it in a way that the people you’re working with will understand. INCREASING FACTORS DECREASING FACTORS NAME SCALE NAME SCALE NEW INTERFACES LESS THAN 1 TEST TEAM SIZE LESS THAN 1 CUSTOMER SITE TIME SINCE LAST 1-10 LESS THAN 1 DEPLOYMENT NEW FUNCTIONALITY … … … … 25 Test Team Size Test Intensity Test Level Rate of software Remaining Defects defect detection Interface Changes Location Changes Even these simple examples help illustrate the concept. The idea here is to make the inputs visible and start getting agreement among the team on the direction and scale of the relationships to bound the problem and to create the related hypotheses that you’ll use later to attack the data. A point you’ll want to emphasize to the team is that these are preliminary assumptions and while your best estimate is needed, it is still just an estimate and is subject to change depending on what reality turns out to be. The danger we’re trying to avoid here is what Kahneman calls “anchoring.” People have a tendency to hang on to views that they’ve seen and held before, even if they are incorrect. Reminding them that these are initial and preliminary, rather than finalized views, helps mitigate the anchoring effect. OBJECTIVE 3. STATE THE SET OF ASSUMPTIONS RELATED TO THE PROBLEM This is where you set the boundaries of the problem. As you look at your input drivers, each likely has one or more assumptions embedded in it that needs to be surfaced and listed. Additionally, some complexities can be trimmed away if their presumed effect on the answer is less than the effort required to handle them. As Stephen R. Covey (2004, p. 24) said, “We simply assume that the way we see things is the way they really are or the way they should be. And our attitudes and behaviors grow out of these assumptions.” Common practice assumptions in your organization also need to be listed and questioned regularly to ensure that they are either still valid or that the problem statement needs to change to incorporate changes to them. 26 OBJECTIVE 4. DEFINE THE KEY METRICS OF SUCCESS There is a truism quoted by many people that “what is measured, improves” (cf. Drucker, Pearson’s Law, Hawthorne effect). This ties directly to the business problem statement, but goes down one level further to the items that comprise the key success metric. For example, if the business problem is that the organization wants to increase return on sales from 10% to 12%, you might decompose that a few different ways. One way is to give each business group that goal, or even to give each group the objective of reaching 13%, figuring that some won’t make it and on average we’ll be okay. Another way is to look at your value chain and give each group a target: cost of goods reduction of five percentage points, general and administrative reduction of three percentage points, etc. These metrics need to be negotiated, published, committed to, and tracked so that your team knows where you are and what to do next. As is the case throughout this chapter, you have to make sure that all facets of the business problem are incorporated in the metrics. After all, if you don’t say how something will be measured, you don’t know how you’re doing, and you can’t succeed. OBJECTIVE 5. OBTAIN STAKEHOLDER AGREEMENT Although you’ve been in touch with your business stakeholders at some level all along, this is when you come back to them to walk them through your assumptions and approach and what the final answer will look like to be sure that you really are answering the business problem. Whether in the form of a formal presentation, you want your assumptions acknowledged along with the reframing you did from the business problem, and the key metrics you will be using to mark progress toward the solution. Many people tend to think of stakeholders as people in positions “above” the analytics team. It is true that there is a group of stakeholders that are the ones with the business need and who are paying for the effort. But just as importantly, you must also have an agreement with the people executing the analytics work that your methods and hypotheses are workable in the time and budget allotted to get the work done. The output of this stakeholder agreement will vary by organization, but should include the budget, timeline, interim milestones (if any), goals, and any known effort that is excluded as out of scope. The key is to get all the pieces we’ve noted in this chapter verbally discussed, documented, and visibly agreed to by all parties. It can be tempting to settle for e-mails or written documents only and desk-side reviews. For all but the simplest problems, this is a mistake. Translation of problems from the business domain to the analytics domain, or truly from any 27 given domain to another domain, requires that all parties agree to definitions and terms, which really does require full and frank discussion. Otherwise, errors will creep in and what was delivered will miss critical unstated requirements. If you allow your project to rely on written communication only, you’ve missed the opportunity to correct misapprehensions when it is still cheap to do so. SUMMARY OF KEY TERMS : the act of breaking down a higher-level requirement to multiple lower-level requirements (http://www.hq.nasa.gov/office/codeq/software/ ComplexElectronics/l_requirements2.htm). : a requirement should be unitary (no conjunctions such as and, but, or or), positive, and testable. SUMMARY Faithful translation of the business problem statement into an analytics problem statement requires the following: Understanding the business case for solving this particular problem Framing the business case as an actionable analytics problem by: Defining the key input and output drivers Surfacing and understanding individual and organizational assumptions Assigning goals to each sub-group affected by the problem Full and frank review of the approach with the business stakeholders and the analysts to ensure that the problem can be attacked as planned and that a successful attack will yield the desired business result. FURTHER READING Albright SC, Winston W, Zappe C (2011) Data Analysis and Decision Making, 4th ed. (South-Western Cengage Learning, Mason, OH). Covey S (2004) (Simon & Schuster, New York). 28 Crow KA (1992) Quality Function Deployment, http://www.ieee.li/tmc/quality_ function_deployment.pdf. National Aeronautics and Space Administration (2009), Assurance Process for Complex Electronics, http://www.hq.nasa.gov/office/codeq/software/ ComplexElectronics/l_requirements2.htm. Tversky A, Kahneman D (1974) Judgment under uncertainty: Heuristics and biases. Science 185(4157):1124–1131. 29 TH I S PA GE IS DE LIBE RATELY LEF T BLANK CHA PTE R 4 DOMAIN III - DATA WHAT WILL YOU LEARN IN THIS CHAPTER? Analytics is defined by INFORMS as the scientific process of transforming data into insight for making better decisions. In this section we will see how data collection, manipulation and analysis support the analytic framework from problem identification to model building and management. Data transformation starts with data element definition and potential source identification. Once sources are identified, collection of new data and extraction and transformation of existing data can begin. Often data will need to be cleaned to address incorrect and/or missing data points. Finally, the data must be properly formatted for use in the common database and loaded into it. Learning Objectives By the end of this chapter, you should be able to 1. Identify and prioritize data needs and resources 2. Identify means of data collection and acquisition 3. Determine how and why to harmonize, rescale, clean and share data 4. Identify ways of discovering relationships in the data 5. Determine the documentation and reporting of findings 6. Use data analysis results to refine business and analytics problem statements Key Concepts/Fundamentals OBJECTIVE 1. IDENTIFY & PRIORITIZE DATA NEEDS & RESOURCES Data reduces our uncertainty about the values assigned to variables of interest in the analysis. Analysis typically uses `hard data’, i.e., data that is obtained by scientific observation and measurement (e.g., experimentation). But much of our information is frequently soft, e.g., gleaned from interviews and reflective opinions and preferences. Hence it will be important to convert this soft information into scientific data. The 31 traditional way in which soft data is converted into hard data is to hypothesize an artificial individual whose preferences and beliefs can be completely described with hard data. (In economics, this artificial individual is called the `economic man’ and is viewed as totally rational.) We then determine what hard data would be required so that this artificial individual’s behavior coincides with that of the actual individual with soft data. We then solve the analytical problem as if our actual individual could be described by this artificial individual. Probably the most successful example of this approach is conjoint measurement or analysis which posits that the behavior of the actual individual can be described by an artificial individual whose preferences are described by a utility function. The utility function for various outcomes is first specified as a parametric function of observable attributes of that item. If this utility function were known, then it is, in theory, straightforward to specify which of several items an individual would choose or how an individual would rank several items. To determine the parameters of this utility function, individuals are then asked to either specify which, of several hypothetical alternatives, they prefer or how they would rank different items in order of preferability. The parameters are then calibrated so as to minimize the disparity between what the individual actual prefers and what the model predicts the individual should prefer. Other methods analogous to conjoint have been developed when uncertainty is involved. For example, it may be necessary to develop a `subjective probability’ as a summary measure of an individual’s beliefs about whether an event occurs. To assess an individual’s subjective probability, consider a random mechanism (e.g., a roulette wheel in a casino or a table of random numbers). For any frequency f between zero and one, this random mechanism can be used to define an uncertain event A(f) which occurs with probability f. An individual’s belief in whether an event E occurs can be specified by asking an individual to choose between betting on event E occurring or A(f) occurring. For some frequencies f, the individual will prefer E to E(f) and for others, they will prefer A(f) to E. The point at which the individual is indifferent is called the individual’s `subjective probability’ for event E. While conjoint is focused on assessing utility functions for known outcomes, the decisions which will be informed by analysis typically are gambles which do not have guaranteed outcomes. As a result, it becomes important to extend the concept of utility to gambles with uncertain outcomes. To construct these utilities, define an experiment where M is some best possible outcome and m is a worst possible outcome. Consider a gamble which leads to M with frequency f and m otherwise. Again consider a carefully designed laboratory environment where the individual must decide between the consequence and the gamble. Then there will be some maximum value of f for which the individual still prefers the consequence to the gamble. This maximum value measures the individual’s preference in the consequence. 32 In gathering data, it is usually important to have some measure of the confidence which is placed on each of the various data points. To translate this notion of confidence into something tangible, consider two individuals, both whose measure of belief in event E is described by the subjective probability p. Consider a carefully designed laboratory experiment in which each individual observes one success in one trial. Each individual’s new belief in the event is then measured. Suppose the resulting value for both individuals is U. A parallel experiment is then run in which the individual’s belief in event E is measured after the individual, instead of observing success in the one trial, observes a failure. Let L be the measure of belief which both individuals now have in the event. Now suppose that one of the individual’s original assessment of p is based only on observing n trials. (More Then it can be shown that n=1/(U-L). Suppose that the other individuaI’s beliefs are based on soft data. Then for analytical purposes, it still is legitimate to use 1/(U-L) as a measure of confidence in p. These examples assumed a carefully designed laboratory experiment. Just as a physical experiment presumes that the physical environment has been prepared to eliminate contaminating influences, so these laboratory experiments must be designed to eliminate contaminating influences like ambiguity, reference point effects, etc. OBJECTIVE 2. IDENTIFY MEANS OF DATA COLLECTION & ACQUISITION The focus of this stage is on identifying which kinds of data collection will have the most favorable impact on the quality of the actions and recommendations supported by the analysis. An especially useful tool for doing this analysis is the decision tree. (While the decision tree as applied to uncertainty was formalized in the mid-twentieth century, it can be argued that the Pythagorean Y might have been the first decision tree.) Consider the following very simple decision tree where there are two choices : continue the present course or make a specific change. If a change is made, the outcome of the change could be favorable or unfavorable. We can write this decision tree in outline form as 1. Continue present course a. Get an average outcome b. Implement a change 2. Get a good outcome a. Get a poor outcome 33 There are two possible outcomes of making the change. If the chance of getting a good outcome is high enough, then it will be better to implement the change. Otherwise implementing the change will be unwise. For example, suppose that we attach a probability p to getting a good outcome if we make a change. Suppose we believe that U is the value (utility) of making a change with the good outcome, L is the value (utility) of making a change if the poor outcome occurs and u is the value (utility) of continuing the present source. Then we will only make a change if p U + (1 - p) L > u. Suppose we find that the best decision (i.e., the one with highest utility to the customer) is to continue the present course. Then we will get utility score u. But instead of simply making a decision, we could have chosen to gather data and then make our decision based on the results of the data gathering exercise. If we chose to gather data, then our decision tree becomes 1. Gather data and get favorable information a. Continue present course i. Get an average outcome b. Implement a change i. Get a good outcome ii. Get a poor outcome 2. Gather data and get unfavorable information a. Continue present course i. Get an average outcome b. Implement a change i. Get a good outcome ii. Get a poor outcome Now suppose we gather data and get favorable information. This increases the probability of getting a good outcome given we implement a change. Suppose the change in probability is not enough to justify implementing the change. So our conditional decision is Now suppose that instead of getting favorable information, our data gathering led us to collect unfavorable information. This lowers the probability 34 of getting a good outcome given we implement a change. As a result, our other conditional decision is Thus our two conditional decisions are Hence regardless of the outcome of the information, we continue our present course. This simple example demonstrates an important principle: Of course, sometimes people collect information—even though they know what decision they will make—in order to defend themselves against criticisms from others. And sometimes people collect information to postpone making the decision. When would information be valuable? Suppose that the favorable information led to a substantial change in the probability of getting a good outcome. Suppose that this change in probability was enough to justify implementing the change. Then our two conditional decisions would be We can assign a value (or utility) to these two conditional decisions. Let u* be the utility of implementing a change, given that we get favorable information. Let u be the utility of continuing the present course, given that we can unfavorable information. Let q be the probability of our getting favorable information if we collect data. Then the utility if we decide to gather data will be q u* + (1 - q) u. Since the utility if we did not gather data was u, this tells us that our overall utility has increase from u to q u* + (1 - q) u. Since u* >u, collecting the information can only improve our utility. This demonstrates a well-established principle: i.e., it can never make you worse off if you behave rationally. But in reality, there is a cost to collecting this information. Suppose that paying this cost would reduce our utility by some factor d. Thus our utility if we collect information is d (q u* + (1 - q )u) , while it is u if we do not collect information. So if we knew q and d, the decision on whether to buy information would depend on (u* - u)/u. What determines u*? Before making a decision, the chance of getting a good outcome after making a decision was p. Suppose that if we get favorable information, this probability changes to p* while if we get unfavorable information, 35 it changes to p**. Then if q is the chance of getting favorable information, the rules of probability require that p=qp*+(1-q)p**. Thus while the utility of making the change was originally p U + (1 - the utility now changes—given a favorable outcomes—to u* where u*=p* U + (1 - p*) L = p* + L. So the critical value u* depends upon p* and, in particular, on how much p* differs from p. The degree to which the new information can change the value of p depends upon the confidence in the original value of p as well as in the impact of the data. One key question is But given that they do change, we need to know what the potential payoff might be. In this example, H was the maximum payoff if we knew for certain that there would be a good outcome. If the potential payoff, H, were small, then gathering more information would also be pointless. The final consideration is cost. Since analysts often collect information from the client’s subject matter experts, it is important to treat the time of these subject matter experts as precious. If they feel their time is being wasted, then they will complain to the client who will eventually begin to wonder about the value of doing your analysis. There are many cases in which an organization chooses a flawed heuristic over a more sophisticated procedure just because the flawed heuristic seems to require less painful information collection. There are also privacy issues. Invasion of privacy can lead to a loss of customer good will and, in some cases, legal repercussions. And if we are gathering information that is potentially proprietary intellectual property issues become paramount. The fact that information technology has made it easier to collect information does not mean that information collection is costless. Once you identify the variables on which you should collect data, the next step is collecting that data. Data collection is analogous to asking certain subjects certain close-ended questions under certain circumstances. Hence there are five steps involved in data collection: 1. Determining how to identify subjects (the sample design) 2. Determining how many subjects to identify (the sampling plan) 36 3. Determining the questions to be asked 4. Determining the possible answers to the question (the granularity of the experiment) 5. Determining a control group SAMPLE DESIGN The population of subjects that could be recruited should be identified. It is common to require random sampling, i.e., to conduct sampling so as to give each subject an equal chance of being part of the sample. This reflects the fact that convenience sampling, e.g., asking those subjects that happen to be easy to identify, has been shown to lead to significant biases. But if the event of interest is highly unlikely, it may be advantageous to bias the sampling toward sampling those individuals most likely to have experienced the event of interest. The analysis will, however, have to take into account this systematic deviation, called stratified random sampling, from conventional random sampling. Typically each subject has different characteristics (or covariates). To determine how these covariates affect the results of the experimenter, it is tempting—but inefficient—to change one factor at a time and record the change in response from the factor as the impact of that factor. Design of experiments has been shown to be a much more efficient way of assessing the impact of changing factors. This typically involves changing several factors simultaneously. If a full factorial design is used, it is possible to identify the impact of each factor as well as the impact of all possible two-way, three-way, etc. interactions between factors. When it is not necessary to know these higher-order interactions, the less time-consuming fractional factorial designs are used. It is common to use response surface modeling (and especially regression) to specify the value of interest as a function of the covariates. The independent variable reflects the covariates and are commonly represented using dummy variables for categorical and interval data. When the variable is ratio scale, Box- Cox Transformations are often used to achieve normality. When the dependent variable is categorical, the regression model is typically logistic. When the dependent variable is ordinal, the regression model is typically ordered logit. When the dependent variable is ratio, standard regression is often used. If Y is the dependent variable and X1,…Xn represent the independent (or explanatory variables), then the typical regression model has the form y=E[Y] + e where e is a normally distributed error term and E[Y], the expected value of Y is some parameterized function of (X1,…Xn). In the interests of making this function linear, it is common to write 37 E[Y] = g(a1 X1’+… an Xn’ ) where g is a `link’ function and X1’,…Xn’ are monotonic transformations of X1,… Xn. This becomes a generalized linear model if we generalized the error term to be a member of the exponential family of distributions (which includes the normal distribution, the exponential distribution and a remarkably large number of other distributions.) Because time is often an important dimension, there are a separate body of time- series methods when observations are collected over time. Time-series analysis typically corrects for seasonal patterns (e.g., unusually high sales during holiday seasons) and provides a natural way of identifying trends. SAMPLING PLAN How many individuals should be sampled? This is typically determined by the existing amount of uncertainty in the quantity of interest, how much that uncertainty needs to be reduced to facilitate the making of a decision and the degree to which an individual’s responses is contaminated with random error. A simple rule of thumb is that quadrupling the number of individuals sampled reduces the uncertainty by half. While uncertainty is commonly measured by the standard deviation, there are situations in which the standard deviation does not exist and the difference between the third and first fractile of the uncertainty distribution is more appropriate. If we are willing to describe our uncertainty using the previously mentioned exponential family of distributions, the rule for updating uncertainties based on sample information has a very simple form. If our uncertainty is described by an exponential family distribution, it will have two parameters. (In some cases, the parameters may be vectors.) The data is described by the number of observations and the sum of a score for each individual observation. This score for each individual observation will depend upon the exponential family distribution being assumed (If the data consists of coin flips, the score might be one for successes and zero otherwise.) Based on this observed data, the original distribution of the uncertainty is updated. The updated distribution will have the same form as the original distribution with two changes. The first parameter is increased by the summed score while the second parameter is increased by the number of observations. In effect, the original uncertainty about the variable—which reflects soft data—can be treated as if it were generated by a hypothetical set of observations. Pooling this hypothetical data with the actual data then generates a new set of hypothetical data. 38 DETERMINING THE QUESTIONS TO BE ASKED A key issue in designing the experiment is determined the nature of the variable being assessed. Is the variable categorical (e.g., values of the variable are blue, red, white) where there is no natural ordering between the values of the variable? When we have categorical scales, the data can be summarized by the proportion of observations which assumed each of the possible values of the categorical variables (e.g., the proportion of blue responses, red responses, etc.) One can ask YES/NO questions or multiple-choice questions for nominal scales. One extension (likert-type questions) asks subjects to indicate whether they fully agree, partially agree, are neutral, partially disagree or fully disagree with the statement.) Alternatively the variable might be ordinal (e.g., short, medium, tall) where there is a natural ordering between the values of the variables. When we have ordinal sales, it is possible to define the normalized quantity for each response x by the fraction of responses less than or equal to x (e.g., the fraction of people who are either short or medium.) A second approach, semantic differential, has the form: `what is your experience navigating our web-site’ with answers like `very hard, somewhat hard, OKAY, somewhat easy, very easy’ where the two ends of the scale represented opposites. In this case, the response is ordinal. In both Likert and semantic differential scales, the response scales may be improved by providing concrete examples of what would have to be true for a `fully agree’ or a `fully disagree’ response to be true. A third approach, rank-order, asks individuals to rate various factors in order of importance. Alternatively the variable might be interval (e.g., thirty degrees centrigrade, forty degrees centrigrade, fifty degrees centigrade) where the differences between values (e.g., forty degrees minus thirty degrees) are meaningful. Note that when we have interval scales, it is possible to define a normalized quantity for each response x by subtracting the lowest possible value from x and dividing the result by the difference between the highest and lowest value. A fourth approach is the simple multiple-choice question. It is important to remember that subjects will often answer a question even when they have no idea about what question is being asked or about what their answer means. (For example, individuals will generally answer the question which is more important diamonds or water even though the answer clearly depends upon 39 whether the individual feels that the choice is between having no water at all for a week (and dying of dehydration) or simply go without an added glass of water for an hour or two.) Questions must be designed with care. DETERMINING A CONTROL GROUP Measurements are typically only meaningful if there is reference to some kind of underlying standard. Thus in extensive measurement, there is some base unit of measure. The score of an item is the number of multiples this basic unit required to create an object that is comparable to the item of interest. By for many non- physical cases, there is no meaningful unit of measurement. In these cases, one creates a benchmark group of units, some smaller than the item of interest and some larger. The score of an item is the proportion of items in a benchmark group which the item outranks. When the item is an uncertain quantity, the score of an item is the probability of the item outranking a randomly chosen item from the benchmark group. This benchmark group is commonly referred to as a control with the item’s score being called its effect size. While some data needs to be created and collected, some data already exists. The purpose of extraction is to collect all this data from the many sources in which it appears so that it can eventually be loaded into a common database. In extracting this data, it is critical to know the data source from which each data element was taken, If the results of an analysis depend critically on the data element, then understanding the validity of this data element becomes critical. In addition, if there is some change in the clients for the analysis, it will be important to transition the database to reflect the data sources which these new clients consider important. This requirement is called traceability and typically requires careful documentation. Another increasingly important issue in using existing data sources is privacy. It is now fairly easy to get personal information on customers by buying such information from vendors. But the customers who provided this information often had an expectation that the information was to be used for a specific purpose, e.g., for enabling them to buy a product over the internet. When these customers discover that their information is being used for another purpose, some customers feel that their privacy is being violated. On top of the privacy issue are intellectual property issues. Even though it may be easy to access the information, there may be copyright or other issues which limit your ability to use it without permission or without compensating the owner of the information. 40 OBJECTIVE 3. DETERMINE HOW AND WHY TO HARMONIZE, RESCALE, CLEAN & SHARE DATA Data cleaning, while often the least glamorous phase of analysis, is often the most necessary. This is especially the case with pre-existing databases. Because pre- existing databases were collected for other purposes, the quality of the data will be driven by what was important in the original use of this data and hence need not satisfy the quality requirements for the analysis at hand. For example, vendors often have to fill in various forms in order to get reimbursed for their services. Sometimes third parties successfully get their own questions added to these surveys. But both vendor and buyer are primarily interested in the fields which determine how much the vendor gets compensated for their services. As a result, these decision-relevant fields get scrutinized carefully and the rest do not. There are many other reasons why survey quality may be deficient: 1. Individuals asked to fill out a lengthy survey will get fatigued and simply put in default values so that they can finish the survey. If there are five possible answers to a survey, they may also check a neutral response. Or in a survey of satisfaction, they may either indicate that they are satisfied with everything or satisfied with nothing. 2. Individuals may also be offended by questions about their age, income, marital status, ethnicity and—if the survey forces them to fill in an answer— will often deliberately fill in a false answer. This is especially true given increasing concerns about privacy 3. Biases can often arise because most people, when asked to fill out a survey, simply refuse. Those who did fill out the survey are often people with more leisure time or with more emotional commitment to the organization asking that the survey be filled out. As a result, data cleaning includes: 1. Identifying the range of valid responses for each question and labeling the data field 2. Identifying invalid data responses (e.g., where letters are used where numbers are required) 3. Identifying inconsistent data encodings (e.g., different abbreviations might be used for state) 41 4. Identifying suspicious data responses (e.g., when physically questionable numbers are put in for a response) Are there outliers that don’t seem to make sense? 5. Identify suspicious distribution of values (e.g., when one finds that 99% of the respondents in a survey of poor neighborhoods have incomes of more than a million dollars.) Descriptive statistics can be very helpful in identifying suspicious distributions. For example, histograms specify the frequency with which various data response are used. Box and Whisker charts as well as stem and leaf plots provide compact descriptions of the variation in the data within a field and help identify outliers. Scatterplots show how the value of one set of variables depends on another. Summary statistics like the mean, median, upper and lower fractiles can also be useful 6. Identify suspicious interrelationships between fields. We first identify whether there is any correlation between data fields—possibly using factor analysis or principle component analysis. The creator of the database may have created a new variable—by combining existing fields—which was useful for their analysis but is no longer useful for your analysis So a key part of data cleaning is determining whether the data makes sense. It also involves handling null or missing values. There are several possible solutions: 1. Deletion: Dropping the observation containing the missing value 2. Deletion when necessary: Not using the observation in analyses requiring a valid response for the missing item. This approach means that one might have a sample of 1000 people for one kind of analysis and a sample of 950 people for a second kind of analysis. 3. Imputing a value: In other words, we use regression to attempt to predict what the answer to this question would have been—based on the answers the subject gave to other questions. 4. Randomly imputing a value: The problem with imputing a value is that it pretends that we do know the value that the subject filled it for this question. Thus understates our uncertainty about the value (and thus overstates our sample size) which can lead to biases in the analysis. Random imputation in theory reruns the analysis for all possible responses the subject might have given to the question, weighted by the regression-based probability of the subjective giving that response. Efficient algorithms have been developed for doing multiple imputation. 42 It is important to determine whether important observations (e.g., observations from a specific group of sub-users) is missing. A field should be created with the data of each observation (a date stamp.) A field should also be created identifying the data source from which this information is collection. This field will be important in the next step where information from different data sources is combined into a single database While the individual responses come from different data sources, they need to be placed into a common database (which typically is organized into rows representing observations and columns representing observed characteristics of that observation). This requires that all of the data be summarized at a common level of granularity. For example, we might have 1000 observations of one product, 5000 observations of a product and its location and 3 observations of a product, its option content and its location. If details about a product’s location are not relevant for the analysis, then we can sum up our observations so that all data is at this less granular level. In other cases, we need to go to this more granular level. If we simply dropped all the observations that did not have this information, there could be insufficient sample size to support a meaningful analysis. Alternatively we may rewrite all of our 9000 records at this more granular level with fields for the product, its location and its option content. We now must treat many of our records as if they had missing values for location and option content. Sometimes data is aggregated in different ways. Thus some information on vehicles is stored with certain vehicles being called two-door Chevrolets. Other information is stored with certain vehicles called Chevy Cruzes. Still other information is stored as General Motors compacts. In this case, there is no a single categorization that is more granular than any other categorization. As a result, we may simply need to define a record which has enough fields to contain the information from each of these observations. Thus there might be a field indicating whether the vehicle was two-door or not, a field for the vehicle’s model name (Cruz), a field indicating the vehicle’s body-type (compact) and a field reflecting the vehicle’s division and manufacturer. In some cases, the model may require information on a variable which is not in the database but can be computed from items in the database. This may require the creation of a new field in the database for this derived variable. In some cases, a single observation may reflect the responses of 10,000 people while another observation may reflect the responses of 100 people. As opposed to creating a database with 10,100 rows for these two observations, it may be useful to introduce a weighting field that identifies the number of respondents associated with the observation. 43 Because different datasets are typically generated with different data architectures and different programming languages, these languages may use different standards for encoding information. Thus missing values can be represented by spaces, the words NA, the words Not/Available, etc. Some decisions may be required in how to handle textual fields. This could be handled by creating numeric columns describing the textual field and—without deleting the textual field—using the columns to classify the field. For example, the textual field might contain verbatim user expressions of satisfaction. A column might be created which expresses the encoder’s interpretation of that field as expressing satisfaction, dissatisfaction or neutrality. Before loading the database, it is useful to assess whether certain fields have the same value across all datasets. If this is the case, then it may be worth deleting those fields. The data is then loaded into the common database. Information is typically normalized so that any given item of information only occurs in the database exactly once. This is the place to do some final checks on the quality of the data: 1. Completeness: Are all the fields of the data complete? 2. Correctness: Is the data accurate? 3. Consistency: Is the data provided under a given field and for a given concept consistent with the definition of that field and concept? 4. Currency: Is the data obsolete? 5. Collaborative: Is the data based on one opinion or on a consensus of experts in the relative area? 6. Confidential: Is the data secure from unauthorized use by individuals other than the decision maker? 7. Clarity: Is the data legible and comprehensible? 8. Common Format: Is the data in a format easily used in the application for which it is intended? 9. Convenient: Can the data be conveniently and quickly accessed by the intended user in a time-frame that allows for it to be effectively used? 10. Cost-effective: Is the cost of collecting and using the data commensurate with its value? 44 The term data warehouse is generally used to describe: 1. A staging area, i.e, the operational data sets from which the information is extracted 2. Data integration which is the centralized source where the data is conveniently stored 3. Access layers, i.e., multiple OLAP (on-line analytical processing) data marts which store the data in a form which will be easy for the analysis to retrieve The data mart is organized along a single point of view (e.g., time, product type, geography) for efficient data retrieval. It allows analysts to 1. slice data, i.e., filtering data by picking a specific subset of the data-cube and choosing a single value for one of its dimensions; 2. dice data, i.e., grouping data by picking specific values for multiple dimensions; 3. drill-down/up, i.e., allow the user to navigate from the most summarized (high-level) to the most detailed (drill-down); 4. roll-up, i.e., summarize the data along a dimension (e.g., computing totals or using some other formula); 5. pivot, i.e., interchange rows and columns (`rotate the cube’). Fact tables are used to record measurements or metrics for specific events at a fairly granular level of detail. Transaction fact details record facts about specific events (like sales events), snapshot fact tables record facts at