CAIIB Paper 1 Advance Bank Management Capsule PDF
Document Details
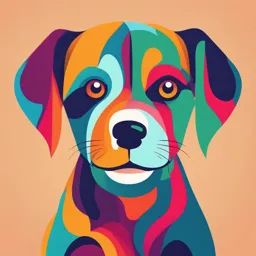
Uploaded by GratifyingDerivative
CAIIB
Ambitious Baba
Tags
Summary
This book provides a capsule summary for CAIIB Paper 1 - Advanced Bank Management. It covers Statistics, Human Resource Management, Credit Management, and Compliance topics, organized into modules for effective learning and exam preparation.
Full Transcript
Ambitiousbaba.com Paid Course B e s t J A I I B C A I I B V i d e o , M o c k T e s t s , C a p s u l e P D F 1 | 257 Ambitiousbaba.com Paid Course CAIIB Paper 1 Advance Bank Management-Capsule Pdf...
Ambitiousbaba.com Paid Course B e s t J A I I B C A I I B V i d e o , M o c k T e s t s , C a p s u l e P D F 1 | 257 Ambitiousbaba.com Paid Course CAIIB Paper 1 Advance Bank Management-Capsule Pdf Index No. of Module Unit Name Page No. Module A Statistics 2 - 89 Module B Human Resource Management 90 - 146 Module C Credit Management 147 – 200 Module d Compliance In Banks & Corporate Governance 201 - 256 CAIIB Paper 1 (ABM) Module A: STATISTICS Index No. of Unit Unit Name Unit 1 Definition of Statistics, Importance & Limitations & Data Collection, Classification & Tabulation Unit 2 Sampling Techniques Unit 3 Measures of Central Tendency & Dispersion, Skewness, Kurtosis Unit 4 Correlation and Regression Unit 5 Time Series Unit 6 Theory of Probability Unit 7 Estimation Unit 8 Linear Programming Unit 9 Simulation CAIIB Paper 1 (ABM) Module A Unit 1: Definition of Statistics, Importance & Limitations & Data Collection, Classification & Tabulation Introduction The word ‘Statistics’ has been derived from the B e s t J A I I B C A I I B V i d e o , M o c k T e s t s , C a p s u l e P D F 2 | 257 Ambitiousbaba.com Paid Course ❑ Latin word ‘statisticum’, ❑ Italian word ‘statistia’ ❑ German word ‘statistik’, ▪ Each of which means a group of numbers or figures that represent some information of human interest. ▪ First used by professor Achen well in 1749 to refer to the subject-matter as a whole. ▪ Achen well defined statistics as the Political Science of many countries. ▪ In the early years statistics is to be used only by the kings to collect facts about the state, revenue of the state or the people in the state of administrative or political purpose. ▪ Gradually the use of statistics which means data or information has increased and widened. ▪ It is now used in almost in all the fields of human knowledge and skills like Business, Commerce, Economics, Social Sciences, Politics, Planning, Medicine and other sciences, physical as well as natural. ▪ In many practical situations in life, we come across different types of data which are needed to be understood, analysed, compared and interpreted correctly. ▪ For example, in a college we need to analyse the data of marks obtained, in a hospital we need to analyse the data of number of patients having different diseases, rate of mortality, Different types of data need to be analysed in Economics, Government and Private organisations, Sports and in many other fields. Statistical analysis of data can be comprised of four distinct phases: Collection of data: In this first stage of investigation, numerical data is collected from different published or unpublished sources, primary or secondary. Classification and Tabulation of data: The raw data collected is to be represented properly for further calculations. The raw data is divided into different groups or classes and represented in a form of a table. Analysis of data: Classified and Tabulated data is analysed using different formulas and methods according to purpose of the study or investigation. Interpretation of data: At the final stage, relevant conclusions are drawn after the data is thoroughly analysed Importance Of Statistics Statistics is the subject that teaches how to deal with data, so statistical knowledge helps to use proper methods for collection of data, properly represent the data, use appropriate formula and methods to analyse correctly and effectively get the results and interpret the data. Applications of Statistics is B e s t J A I I B C A I I B V i d e o , M o c k T e s t s , C a p s u l e P D F 3 | 257 Ambitiousbaba.com Paid Course important in every sphere of field – Business and economics, Medical, Sports, Weather forecast, Stock Market, Quality Testing, Government decisions and policies, Banks, Different educational and research organisations, etc. Business and Economics In Business, the decision maker takes suitable policies and strategies based on information on production, sale, profit, purchase, finance, etc. By using the techniques of time series analysis, the businessman can predict the effect of a large number of variables with a fair degree of accuracy. By using ‘Bayesian Decision Theory’, the businessmen can select the optimal decisions to directly evaluate the payoff for each alternative course of action. In Economics, Statistics is used to analyse demand, cost, price, quantity, different laws of demand like elasticity of demand and consumer’s maximum satisfaction which is determined on the basis of data pertaining to income and expenditure. Medical Statistics have extensive application in clinical research and medical field. Clinical research involves investigating proposed medical treatments, assessing the relative benefits of competing therapies, and establishing optimal treatment combinations. Weather Forecast Statistical methods, like Regression techniques and Time series analysis, are used in weather forecasting. Stock Market Statistical methods, like Correlation and Regression techniques, Time series analysis are used in forecasting stock prices. Return and Risk Analysis is used in calculation of Market and Personal Portfolios and Mutual Funds. Bank In banking industry, credit policies are decided based on statistical analysis of profitability, demand deposits, time deposits, credit ratio, number of customers and many other ratios. The credit policies are based on the application of probability theory. Sports Players use statistics to identify or rectify their mistakes. A proper understanding of the statistics determines the success of a team or a single athlete. B e s t J A I I B C A I I B V i d e o , M o c k T e s t s , C a p s u l e P D F 4 | 257 Ambitiousbaba.com Paid Course Function Of Statistics Statistics present the facts in definite form. Statistics simplify complex data. It provides a techniques of comparison. Statistics study the relationship between two or more variables. It helps in formulating policies. It helps in forecasting outcomes. Limitations Or Demerits Of Statistics Statistics do not deal with Individuals: Statistical methods can’t be applied for individual values of the observations as for individual observation, there is no point of comparing anything or analysing anything. Statistics is the study of mass data or a group of observations and deals with aggregates of facts. Statistics does not study Qualitative Data: Statistical methods can’t be applied for qualitative or non-numerical data. Statistics is the study of only of those facts which are capable of being stated in number or quantity. Statistics give Result only on an Average: Statistical methods are not exact. Generally, when we have large number of observations, it becomes difficult to handle it. A part of the data (sample) is collected for study and draw conclusion from, as a representative for the whole. As a result, the result obtained are not exactly same, had we analysed the whole data. The results are true only on an average in the long run. The results can be biased: The data collection may sometime be biased which will make the whole investigation useless. Generally, this situation arises when data is handled by inexperienced or dishonest person. Definitions Population It is the entire collection of observations (person, animal, plant or things which is actually studied by a researcher) from which we may collect data. It is the entire group we are interested in and from which we need to draw conclusions. Example: If we are studying the weight of adult men in India, the population is the set of weights of all men in India. Data can be classified into two types, based on their characteristics. Variates: A characteristic that varies from one individual to another and can be expressed in numerical terms is called variate. Example: Prices of a given commodity, wages of workers, heights and weights of students in a class, marks of students, etc. B e s t J A I I B C A I I B V i d e o , M o c k T e s t s , C a p s u l e P D F 5 | 257 Ambitiousbaba.com Paid Course Attributes: A characteristic that varies from one individual to another but can’t be expressed in numerical terms is called an attribute. Example: Colour of the ball (black, blue, green, etc.), religion of human, etc. Collection Of Data Researchers or investigators need to collect data from respondents. There are two types of data. Primary Data Primary data is the data which is collected directly or first time by the investigator or researcher from the respondents. Primary data is collected by using the following methods: Direct Interview Method: A face to face contact is made with the informants or respondents (persons from whom the information is to be obtained) under this method of collecting data. The interviewer asks them questions pertaining to the survey and collects the desired information. Questionnaires: Questionnaires are survey instruments containing short closed-ended questions (multiple choice) or broad open-ended questions. Questionnaires are used to collect data from a large group of subjects on a specific topic. Currently, many questionnaires are developed and administered online. Census and sample survey In a census, data about all individual units (e.g., people or households) are collected in the population. In a survey, data are only collected for a sub-part of the population; this part is called a sample. B e s t J A I I B C A I I B V i d e o , M o c k T e s t s , C a p s u l e P D F 6 | 257 Ambitiousbaba.com Paid Course These data are then used to estimate the characteristics of the whole population. In this case, it has to be ensured that the sample is representative of the population in question. For example, the proportion of people below the age of 18 or the proportion of women and men in the selected sample of households has to reflect the reality in the total population. Secondary Data Secondary data are the Second hand information. The data which have already been collected and processed by some agency or persons and is collected for the second time are termed as secondary data. According to M. M. Blair, “Secondary data are those already in existence and which have been collected for some other purpose.” Secondary data may be collected from existing records, different published or unpublished sources, like WHO, UNESCO, LIC, etc., various research and educational organisations, banks and financial places, magazines, internet, etc. Distinction between primary and secondary data The data collected for the first time is called Primary data and data collected through some published or unpublished sources is called Secondary data. The primary data in the hands of one person can become secondary for all others. For example, the population census report is primary for the Registrar General of India and the information from the report is secondary for others. Primary data are original as they are collected first time from the respondents directly or by preparing questionnaires. So they are more accurate than the secondary data. But the collection of primary data requires more money, time and energy than the secondary data. A proper choice between the two forms of information should be made in an enquiry. Classification and Tabulation So, we learned about the different methods of collecting primary and secondary data. The raw data, collected in real situations are arranged randomly, haphazardly and sometimes the data size is very large. Thus, the raw data do not give any clear picture and interpreting and drawing any conclusion becomes very difficult. To make the data understandable, comparable and to locate similarities, the next step is classification of data. The method of arranging data into homogeneous group or classes according to some common characteristics present in the data is called Classification. Example: The process of sorting letters in a post office, the letters are classified according to the cities and further arranged according to the streets. Classification condenses the data by removing unimportant details. It enables us to accommodate large number of observations into few classes and study the relationship between several characteristics. Classified data is presented in a more organised way so it is easier to interpret and compare them, which is known as Tabulation. There are four important bases of classifications: B e s t J A I I B C A I I B V i d e o , M o c k T e s t s , C a p s u l e P D F 7 | 257 Ambitiousbaba.com Paid Course Qualitative Base: Here the data is classified according to some quality or attribute such as sex, religion, literacy, intelligence, etc. Quantitative Base: Here the data is classified according to some quantitative characteristic like height, weight, age, income, marks, etc. Geographical Base: Here the data is classified by geographical regions or location, like states, cities, countries, etc. like population in different states of India. Chronological or Temporal Base: Here the data is classified or arranged by their time of occurrence, such as years, months, weeks, days, etc. This classification is also called Time Series data. Example: Sales of a company for different years. Types of Classification If we classify observed data for a single characteristic, it is known as One-way Classification. Ex: Population can be classified by Religion – Hindu, Muslim, Christians, etc. If we consider two characteristics at a time to classify the observed data, it is known as a Two- way classification. Ex: Population can be classified according to Religion and sex. If we consider more than two characteristics at a time in order to classify the observed data, it is known as Multi-way Classification. Ex: Population can be classified by Religion, sex and literacy. Frequency Distribution Frequency ▪ If the value of a variable (discrete or continuous) e.g., height, weight, income, etc. occurs twice or more in a given series of observations, then the number of occurrences of the value is termed as the “frequency” of that value. ▪ The way of representing a data in a form of a table consisting of the values of the variable with the corresponding frequencies is called “frequency distribution”. ▪ So, in other words, Frequency distribution is a table used to organise the data. ▪ The left column (called classes or groups) includes numerical intervals on a variable under study. ▪ The right column contains the list of frequencies, or number of occurrences of each class/group. ▪ Croxton and Cowden defined frequency distribution as a statistical table which shows the sets of all distinct values of the variable arranged in order of magnitude, either individually or in groups with their corresponding frequencies side by side Intervals are normally of equal size covering the sample observations range. Class-limits or Class Intervals B e s t J A I I B C A I I B V i d e o , M o c k T e s t s , C a p s u l e P D F 8 | 257 Ambitiousbaba.com Paid Course A class is formed within the two values, class-limits or class-intervals. The lower value is called lower class limit or lower-class interval and the upper value is called upper class limit or class interval. Class Length or Class Width The difference between the class’upper and lower class limit is called the length or the width of class. Class Length = Class Width = Upper Class Interval – Lower Class Interval Mid-Value or Class Mark The mid-point of the class is called mid-value or class mark. Class Mark = (Lower class-limit + Upper Class limit)/2 Types of Class Intervals Exclusive type, Inclusive type Exclusive type Class intervals like 0–10, 10–20; 500–1000, 1000–1500 are called exclusive types. Here the upper limits of the classes are excluded from the respective classes and put in the next class while considering the frequency of the respective class. For example, the value 15 is excluded from the class 10–15 and put in the class 15–20. Inclusive type Class intervals 60–69, 70–79, 80–89, etc. are inclusive type. Here both the lower and upper class limits are included in the class-intervals while considering the frequency of the respective class, e.g., 60 and 69 are both included in the class 60–69. B e s t J A I I B C A I I B V i d e o , M o c k T e s t s , C a p s u l e P D F 9 | 257 Ambitiousbaba.com Paid Course Class Boundaries Inclusive classes can be converted to exclusive classes and the new class intervals are called class boundaries. Example : The classes 5–9, 10–14 can be converted to exclusive type of classes using the formula → New UCI = Old UCI + (10 – 9)/2 = 9 + 0.5 = 9.5. New LCI = Old LCI – (10 – 9)/2 = 5 – 0.5 = 4.5. So the class- boundaries are 4.5–9.5, 9.5–14.5, etc. Open-end Class Interval In open-end class interval either the lower limit of the first class or upper limit of the last class or both are missing. Example: Below 10 10–20 20–30 30–40 Above 40 Relative Frequency = frequency /Total frequency Example: Relative frequency of the class interval = 20–30 in Example 2 is 12/32 = 0.375 Percentage Frequency Percentage Frequency = (Class frequency/Total Frequency) × 100 Example: Percentage frequency of the class interval = 20–30 in Example 2 is (12/32) 100 = 37.5. Frequency Density B e s t J A I I B C A I I B V i d e o , M o c k T e s t s , C a p s u l e P D F 10 | 257 Ambitiousbaba.com Paid Course Frequency density of a class interval = Class frequency/Width of Class Continuous Frequency Distribution: ▪ Variable takes values which are expressed in class intervals within certain limits. Problem: Marks obtained by 20 students in an exam for 50 marks are given below–convert the data into continuous frequency distribution form. 18, 23, 28, 29, 44, 28, 48, 33, 32, 43, 24, 29, 32, 39, 49, 42, 27, 33, 28, 29. Problem: Following data reveals information about the number of children per family for 25 families. Prepare frequency distribution of number of children (say variable x, taking distinct values 0, 1, 2, 3, 4). 32112 40123 12042 21232 13401 Solution: B e s t J A I I B C A I I B V i d e o , M o c k T e s t s , C a p s u l e P D F 11 | 257 Ambitiousbaba.com Paid Course CAIIB Paper 1 (ABM) Module A Unit 2: Sampling Methods Sampling Sampling is a process used in statistical analysis in which a predetermined number of observations are taken from a larger population. The methodology used to sample from a larger population depends on the type of analysis being performed. Types of sampling There are two methods of selecting from populations Non- random or judgement sampling Random or probability sampling Random Sampling A probability sampling method is any method of sampling that utilizes some form of random selection. In order to have a random selection method, you must set up some process or procedure that assures that the different units in your population have equal probabilities of being chosen. Type of Random Sampling There are four main type of random sampling i)Simple Random Sampling (SRS) ii)Stratified Sampling iii)Cluster Sampling B e s t J A I I B C A I I B V i d e o , M o c k T e s t s , C a p s u l e P D F 12 | 257 Ambitiousbaba.com Paid Course iv)Systematic Sampling Simple Random Sampling (SRS): Simple Random Sampling selects samples by methods that allow each possible sample to have an equal probability of being picked and each item in the entire population to have an equal chance or being included in the sample. Systematic Sampling: In systematic sampling, elements are selected from the population at a uniform level that is measured in time, order, or space. If we wanted to interview every twentieth student on a college campus, we would choose a random starting point in the first twenty names in the student directory and then pick every twentieth name thereafter. Stratified Sampling: To use stratified sampling, we divide the population into relatively homogenous groups, called strata. Then we use one of two approaches. Either we select at random from each stratum a specified number of elements corresponding to the proportion of that stratum in the population as a whole or we draw an equal number of elements from each stratum and give weight to the results according to the stratum’s proportion of total population. Cluster Sampling: In cluster sampling, we divide the population into groups or clusters and then select a random sample of these clusters. We assume that these individual clusters are representative of the population as a whole. If a market Research team is attempting to determine by sampling the average number of television sets per household in a large city, they could use a city map and divide the territory into blocks and then choose a certain number of blocks (clusters) for interviewing. Every household in each of these blocks would be interviewed. A well designed cluster sampling procedure can produce a more precise sample at considerably less cost than that of simple random sampling. Sampling distribution Sampling Distribution is the distribution of all possible values of a statistic from all possible samples of a particular size drawn from the population. Each sample we draw from a population would have its own means or measure of central tendency and standard deviation. Thus, the statistics we compute for each sample, would vary & be different for each random sample taken. Sample Distribution Table B e s t J A I I B C A I I B V i d e o , M o c k T e s t s , C a p s u l e P D F 13 | 257 Ambitiousbaba.com Paid Course SAMPLES, their DATA & Mean CONCEPT of STANDARD ERROR Standard deviation of the distribution of the sample means is called the standard error of the mean. Similarly standard error of the proportion is the standard deviation of the distribution of the sample proportions. e.g. We take the average height of college girls in India across various samples. We would calculate mean height of each sample. Obviously there is some variability in observed mean. This variability in sampling statistics results from the sampling error due to chance. Thus the standard deviation of the sampling distribution of means measures the extent to which the means vary because of a chance error in the sampling process. Thus the standard deviation of distribution of a sample statistic is known as the Standard error of the statistic. Thus, a standard error indicates not only the size of the chance error but also the accuracy we are likely to get if we use the sample statistic to estimate a population statistic. Sampling From Normal Population B e s t J A I I B C A I I B V i d e o , M o c k T e s t s , C a p s u l e P D F 14 | 257 Ambitiousbaba.com Paid Course Finite Populations: μ= 162.40 x̄ = 162.40 This is not coincidence. The mean of the sample means is the same as the population mean, whenever we use simple random sampling. Example -Bank calculate that its individual saving account have a mean of Rs.2000 and SD of 600. bank takes a sample of 100 account. Calculate the Standard error? What is the probability that the sample lie between 1900 & 2050. σX¯=σ/ √n = 600 /10 =60 Probability associated with a standard normal variable Standard Error Of The Mean For Infinite Populations: Example: Bank calculate that its individual saving account have a mean of Rs.5000 and SD of 600. bank takes a sample of 100 account. Calculate the Standard error? What is the probability that the sample lie between 1900 & 2050. STEP 1 : Standard deviation of error σX¯=σ/ √n = 600 /10 B e s t J A I I B C A I I B V i d e o , M o c k T e s t s , C a p s u l e P D F 15 | 257 Ambitiousbaba.com Paid Course =60 Central Limit Theorem ▪ The mean of the sampling distribution of the mean will equal the population mean regardless of the sample size, even if the population is not normal. ▪ As the sample size increases, the sampling distribution of the mean will approach normality, regardless of the shape of the population distribution. ▪ This relationship between the shape of the population distribution & the shape of sampling distribution of the mean is called the Central Limit Theorem. Actually a sample doesnot have to be very large for the sampling distribution of the mean to approach normal Statistician use the normal distribution as an approximation to the sampling distribution whenever the sample size is atleast 30, but the sampling distribution whenever the sample size is atleast 30. The significance of the CLT is that it permits us to use sample statistics to make interference about population parameters without knowing anything about the shape of the frequency distribution of that population. Example: Bank distribution has a mean of Rs.19000 & standard deviation of Rs.2000. If we draw a random sample of 30 tellers, What is the probability that their earning will average more than Rs.19750 annually? B e s t J A I I B C A I I B V i d e o , M o c k T e s t s , C a p s u l e P D F 16 | 257 Ambitiousbaba.com Paid Course STEP 1 : Calculate Standard error = 2000/ √30 = 2000/ 5.477 = 365.16 STEP 2 : Z value & Standard Normal Probability Distribution X = 19750 = 19750-19000/365.16 = 750/ 365.16 =2.05 Finite Population Multiplier Standard Error Of The Mean For Finite Populations N = size of population N = size of the sample Example: B e s t J A I I B C A I I B V i d e o , M o c k T e s t s , C a p s u l e P D F 17 | 257 Ambitiousbaba.com Paid Course We are interested in a population of 20 textile companies of the same size, all of which are experiencing excessive labour turnover. Standard deviation of the distribution of annual turnover is 75 employees. If we sample 5 of these textile companies, without replacement then compute the standard error of mean? = 75/ √5 [√(20-5/20-1)] = 33.54 * 0.888 = 29.8 Numerical on Sampling Q1. A sack contains 3 pink balls and 7 green balls. What is probability to draw one pink ball and two green balls in one draw? 23 (a) 40 21 (b) 40 27 (c) 40 9 (d) 20 21 (e) 38 Ans(b) Out of (3+ 7) = 10 balls, three (one pink & two green) balls are expected to be drawn 3c 7 1 × c2 So, required probability = 10c 3 3 7 × 1 2 ×1 = 10 3×2×1 3×21 = 120 21 = 40 Q2.A sack contains 4 black balls 5 red balls. What is probability to draw 1 black ball and 2 red balls in one draw? (a) 11/19 (b) 10/21 (c)12/22 B e s t J A I I B C A I I B V i d e o , M o c k T e s t s , C a p s u l e P D F 18 | 257 Ambitiousbaba.com Paid Course (d) 19/11 Ans: B Solution : Out of 9, 3 (1 black & 2 red) are expected to be drawn) Hence sample space n(S) = 9c3 = 9!/(6!×3!) = 362880/4320 = 84 Now out of 4 black ball 1 is expected to be drawn hence n(B) = 4c1 =4 Same way out of 5 red balls 2 are expected be drawn hence n(R) = 5c2 = 5!/(3!×2!) = 120/12 = 10 Then P(B U R) = n(B)×n(R)/n(S) i.e 4×10/84 = 10/21 CAIIB Paper 1 (ABM) Module A Unit 3: Measures of Central Tendency & Dispersion, Skewness, Kurtosis Introduction To Measures Of Central Tendency ▪ Statistical data is first collected (primary or secondary) and then classified into different groups according to common characteristics and presented in a form of a table. ▪ It is easy for us to study the different characteristics of data from a tabular form. ▪ Further, graphs and diagrams can also be drawn to convey a better impression to the mind about the data. B e s t J A I I B C A I I B V i d e o , M o c k T e s t s , C a p s u l e P D F 19 | 257 Ambitiousbaba.com Paid Course ▪ Classified and Tabulated data need to be analysed using different statistical methods and tools and then draw conclusions from it. ▪ Central Tendency and Dispersion are the most common and widely used statistical tool which handles large quantity of data and reduces the data to a single value used for doing comparative studies and draw conclusion with accuracy and clarity. ▪ According to the statistician, Professor Bowley “Measures of Central Tendency (averages) are statistical constants which enable us to comprehend in single effort the significant of the whole”. The main objectives of Measure of Central Tendency are: ✓ To condense data in a single value. ✓ To facilitate comparisons between data. ▪ In other words, the tendency of data to cluster around a central or mid value is called central tendency of data, central tendency is measured by averages. ▪ There are different types of averages, each has its own advantages and disadvantages. Requisites of a Good Measure of Central Tendency ✓ It should be rigidly defined. ✓ It should be simple to understand and easy to calculate. ✓ It should be based on all the observations of the data. ✓ It should be capable of further mathematical treatment. ✓ It should be least affected by the fluctuations of the sampling. ✓ It should not be unduly affected by the extreme values. ✓ It should be easy to interpret. Three types of averages are Mean, Median and Mode. Mean ▪ Mean or average is the most commonly used single descriptive measure of Central Tendency. ▪ Mean is simple to compute, easy to understand and interpret. Mean is of three types: ✓ Arithmetic Mean, ✓ Geometric Mean ✓ Harmonic Mean. Arithmetic Mean ▪ The arithmetic mean is the simplest and most widely used measure of a mean, or average. B e s t J A I I B C A I I B V i d e o , M o c k T e s t s , C a p s u l e P D F 20 | 257 Ambitiousbaba.com Paid Course ▪ It simply involves taking the sum of a group of numbers, then dividing that sum by the count of the numbers used in the series. Arithmetic Mean of Ungrouped or Raw Data Example 1: Consider the marks scored by 10 students in Mathematics in a certain examination 35, 30, 18, 15, 40, 30, 52, x, 47, 10. If the arithmetic mean is 30, find the value of x. X̄ = 35 +30 +18 + 15 + 40 +30 +52 + x + 47 + 10/ 10 30 = 277 + x/ 10 300 = 277 + x X= 23 Arithmetic Mean of Grouped data ▪ If a variate X take values x1, x2, …, xn with corresponding frequencies f 1, f 2, …, f n respectively, then the arithmetic mean of these values is Example 2: Find the Arithmetic mean for following: B e s t J A I I B C A I I B V i d e o , M o c k T e s t s , C a p s u l e P D F 21 | 257 Ambitiousbaba.com Paid Course Combined Arithmetic Mean If X̄ 1 and X̄ 2are the arithmetic mean of two samples of size n1 and n2 respectively then, the Combined arithmetic mean Example: The average marks of a group of 100 students in Mathematics are 60 and for other group of 50 students, the average marks are 90. Find the average marks combined group of 150 students. B e s t J A I I B C A I I B V i d e o , M o c k T e s t s , C a p s u l e P D F 22 | 257 Ambitiousbaba.com Paid Course Example: In private health club, there are 200 members, 100 men, 80 women and 20 children. The average weight of men, women and children are 60 kgs, 50 kgs and 35 kgs respectively. Find the average weight of the combined group. n1 = 100, n2 = 80, n3 = 20 x1 = 60, x2 = 50, x3 = 35 Combined mean = X̄ = n1 x1 + n2 x2 + n3 x3/ n1 + n2 + n3 = 100*60 + 80*50 + 20*35/ 200 = 6000+ 4000 + 700 / 200 = 10700/2 = 53.5 Merits of Arithmetic Mean It is rigidly defined It is easy to calculate and simple to follow It is based on all the observations It is determined for almost every kind of data It is finite and indefinite It is readily put to algebraic treatment It is least affected by fluctuations of sampling. Demerits of Arithmetic Mean It is highly affected by extreme values. It cannot average the ratios and percentages properly. It is not an appropriate average for highly skewed distribution. It cannot be computed accurately if any item is missing. The mean sometimes does not coincide with any of the observed value. Mean cannot be calculated when open-end class intervals are present in the data B e s t J A I I B C A I I B V i d e o , M o c k T e s t s , C a p s u l e P D F 23 | 257 Ambitiousbaba.com Paid Course Geometric Mean The Geometric Mean (GM) is the average value or mean which measures the central tendency of the set of numbers by taking the root of the product of their values. Geometric mean takes into account the compounding effect of the data that occurs from period to period. Geometric mean is always less than Arithmetic Mean and is calculated only for positive values. Applications It is used in stock indexes. It is used to calculate the annual return on the portfolio. It is used in finance to find the average growth rates which are also referred to the compounded annual growth rate. It is also used in studies like cell division and bacterial growth, etc. Geometric Mean of Ungrouped or Raw Data Example: Find the G.M. of the values 10, 24, 15, and 32. Given 10, 24, 15, 32 We know that G.M. = 4√ 10*24*15*32 = (10*24*15*32)^1/4 = 115200 ^1/4 = 18.423 Geometric Mean of Grouped or Raw Data Example: Find the G.M. for the following data B e s t J A I I B C A I I B V i d e o , M o c k T e s t s , C a p s u l e P D F 24 | 257 Ambitiousbaba.com Paid Course Merits of Geometric Mean It is useful in the construction of index numbers. It is not much affected by the fluctuations of sampling. It is based on all the observations. Demerits of Geometric Mean It cannot be easily understood. It is relatively difficult to compute as it requires some special knowledge of logarithms. It cannot be calculated when any item or value is zero or negative. Harmonic Mean Harmonic Mean is defined as the reciprocal of the arithmetic mean of reciprocals of the observations. Arithmetic mean is appropriate measure of central tendency when the values have the same units whereas the Harmonic mean is appropriate measure of central tendency when the values are the ratios of two variables and have different measures. So, generally Harmonic mean is used to calculate the average of ratios or rates. Applications It is used in finance to find average of different rates. It can be used to calculate quantities such as speed. This is because speed is expressed as a ratio of two measuring units such as km/hr. Harmonic Mean of Ungrouped or Raw data: B e s t J A I I B C A I I B V i d e o , M o c k T e s t s , C a p s u l e P D F 25 | 257 Ambitiousbaba.com Paid Course H.M = n / (1/x1 + 1/x2 + 1/x3+ ….. 1/y) Example: Find the HM of the values 10, 24, 15, and 32 = 4/ (1/10+1/24+1/15+1/32) = 4 / 0.1 + 0.042 + 0.067 + 0.031 = 4/.24 = 16.667 Harmonic Mean of Ungrouped or Raw data: N /( F1/X1 + F2/X2 + F3/X3….) Example: Find the H.M. for the following data Comparison between Arithmetic, Geometric and Harmonic Mean ▪ The arithmetic mean is appropriate if the values have the same units, whereas the geometric mean is appropriate if the values have different units and harmonic mean is appropriate if the data values are ratios of two variables with different measures, called rates. ▪ Arithmetic Mean > Harmonic Mean > Geometric Mean B e s t J A I I B C A I I B V i d e o , M o c k T e s t s , C a p s u l e P D F 26 | 257 Ambitiousbaba.com Paid Course ▪ A.M. × H.M. = (G.M.)^2 Example: Find the Harmonic mean of two numbers a and b, if their Arithmetic mean is 16 and Geometric mean is 8. ▪ A.M. = 16 and G.M. = 8 ▪ A.M. × H.M. = G.M^2 ▪ 16 × H.M. = 8^2 ▪ 16 × H.M. = 64 ▪ H.M.= 64/16 = 4 Median And Quartiles ▪ The median is the middle value of a distribution, i.e., median of a distribution is the value of the variable which divides it into two equal parts. ▪ It is the value of the variable such that the number of observations above it is equal to the number of observations below it. ▪ Observations are arranged either in ascending order or descending order of their magnitude. ▪ Median is a position average whereas the arithmetic mean is a calculated average. Median of Ungrouped or Raw data ▪ The formula to calculate the median of the data is different for odd and even number of observations. Median of odd Number of Observations If the total number of given observations is odd, then the formula to calculate the median for a number of n observations is: Median = n +1/ 2 th observation Median of even Number of Observations If the total number of given observations is even, then the median formula to calculate the median for n number of observations is: Median = Median= (n/2)th observation + (n/2+1)th observation / 2 Example: Find Median of 34, 32, 48, 38, 24, 30, 27, 21, 35. Arranging the data in ascending order, 21, 24, 27, 30, 32, 34, 35, 38, 48. B e s t J A I I B C A I I B V i d e o , M o c k T e s t s , C a p s u l e P D F 27 | 257 Ambitiousbaba.com Paid Course n = 9; Median= (n+1/2) th position = (9+1/2) the position = 32 Median of Grouped data: If variable X takes values X1, X2, X3, X4…..X5 and corresponding frequencies f1, f2, f3, f4,….. Fn respectively, then the median value is given by Median class is the class in which the corresponding value of less than cumulative frequency just exceeds the value of N/2. ▪ l1 = lower limit of the median class, ▪ l2 = upper limit of the median class ▪ f = frequency of the median class, ▪ cf = cumulative frequency of the class preceding the median class, ▪ N = total frequency. Example: Find Median for the following data. B e s t J A I I B C A I I B V i d e o , M o c k T e s t s , C a p s u l e P D F 28 | 257 Ambitiousbaba.com Paid Course Quartiles ▪ A quartile represents the division of data into four equal parts. ▪ First, second intervals are based on the data values and third their relationship to the total set of observations. ▪ By dividing the distribution into four groups, the quartile calculates the range of values above and below the mean. A quartile divides data into three points ✓ the lower quartile Q1, ✓ the median Q2, and ✓ the upper quartile Q3, to create four dataset groupings. The interquartile range is a measure of variability around the median, which is calculated using the quartiles are denoted by Q1, Q2 and Q3 B e s t J A I I B C A I I B V i d e o , M o c k T e s t s , C a p s u l e P D F 29 | 257 Ambitiousbaba.com Paid Course Calculate Q1,Q2 & Q3 Q1 = l1 + (q1-CF)/f (l2-l1) where q1 = N/4 Q2 = l1 + (q2- CF)/f (l2-l1) where q2 = 2N/4 Q3 = l1 + (q3- CF)/f (l2-l1) where q3 = 3N/4 Example: Find the quartiles for the following data B e s t J A I I B C A I I B V i d e o , M o c k T e s t s , C a p s u l e P D F 30 | 257 Ambitiousbaba.com Paid Course Q3 = l1 + (q3- CF)/f (l2-l1) = 30 (150-126) / 25 (35-30) = 34.8 MODE ▪ The mode of a set of numbers is that number, which occurs more number of times than any other number in the set. ▪ It is the most frequently occurring value. ▪ If two or more values occur with equal or nearly equal number of times, then the distribution is said to have two or more modes. ▪ In case, there are three or more modes and the distribution or data set is said to be multimodal. Mode of Ungrouped or Raw data Example 22: Find Mode for the data: 23, 25, 20, 23, 26, 21, 27, 28, 30, 27, 23. Value 23 occurs maximum number of times, so Mode = 23. Mode of Grouped data If a variate X take values x1, x2, x3, x4 with corresponding frequencies f1, f2, f3, f4…. respectively, then the mode is B e s t J A I I B C A I I B V i d e o , M o c k T e s t s , C a p s u l e P D F 31 | 257 Ambitiousbaba.com Paid Course Where, l1 = lower limit of the modal class l2= per limit of the modal class f1 = frequency of the modal class f0 = frequency of the class preceding the modal class f2 = frequency of the class succeeding the modal class Example: Find Mode for data Merits of Mode It is easy to calculate and understand. It is not affected much by sampling fluctuations. It is not necessary to know all items. Only the point of maximum concentration is required. Demerits of Mode It is ill defined as it is not based on all observations. It is not capable of further algebraic treatment. It is not a good representative. B e s t J A I I B C A I I B V i d e o , M o c k T e s t s , C a p s u l e P D F 32 | 257 Ambitiousbaba.com Paid Course Relationship among Mean, Media and Mode Mode = 3 Median – 2 Mean Introduction to Measures Of Dispersion A single value that attempts to describe a set of data by identifying the central position within the set of data is called measure of central tendency. Measure of Dispersion is another property of a data which establishes the degree of variability or the spread out or scatter of the individual items and their deviation from (or the difference with) the averages or central tendencies. The process by which data are scattered, stretched, or spread out among a variety of categories is referred to as dispersion. Finding the size of the distribution values that are expected from the collection of data for the particular variable is a part of this process. The dispersion of data is a concept in statistics that lets one understand a dataset more simply by classifying individual pieces of data according their own unique dispersion criteria, such as the variance, the standard deviation, and the range. A collection of measurements known as dispersion can be used to determine the quality of the data in an objective and quantitative manner. Various measures of dispersion are given below: Four Absolute Measures of Dispersion Range Quartile Deviation Mean Deviation Standard Deviation Four Relative Measures of Dispersion Coefficient of Range Coefficient of Quartile Deviation Coefficient of Mean Deviation Coefficient of Variation Characteristics of a Good Measure of Dispersion It should be rigidly defined. It should be based on all observations. It should be easy to calculate and understand. It should be capable of further algebraic treatment. It should not be affected much by sampling fluctuations. B e s t J A I I B C A I I B V i d e o , M o c k T e s t s , C a p s u l e P D F 33 | 257 Ambitiousbaba.com Paid Course Range and Coefficient Of Range Range It is the simplest absolute measure of dispersion. Range (R) = Maximum – Minimum Coefficient of Range = (Max – Min)/(Max + Min) Example 1 Find the range and coefficient of range of the following items: 18, 15, 20, 17, 22, 16. ▪ Range = Max – Min = 22 – 15 = 7. ▪ Coefficient of Range = (Max – Min)/(Max + Min) = 7/37 = 0.19 Note: Range and Coefficient of Range are used to measure the spread in Quality Control, Fluctuations in the Share Prices, in Weather Forecasts: Merits of Range It is easy to understand. It is easy to calculate. Demerits of Range It is not based on all observations. B e s t J A I I B C A I I B V i d e o , M o c k T e s t s , C a p s u l e P D F 34 | 257 Ambitiousbaba.com Paid Course It does not have sampling stability. A single observation may change the value of range. As the amount of data increases, range becomes less satisfactory Quartile Deviation And Coefficient Of Quartile Deviation It is the mid-point of the range between two quartiles. Quartile Deviation is defined as QD = (Q3 – Q1 )/2 Where Q1 = 1st quartile and Q 3 = 3rd quartile. Co-efficient of QD = (Q3 – Q1)/ (Q3 + Q1) Merits of Quartile Deviation It is easy to calculate and understand. It is not affected by extreme values. Demerits of Quartile Deviation It is not based on all observations. It is not capable of further algebraic treatment. It is affected by sampling fluctuations. Mean Deviation and Coefficient of Mean Deviation ▪ Mean deviation of a set of observations of a series is the arithmetic mean of all the deviations. ▪ It is the deviations from mean when calculated considering their absolute values and are averaged. Mean Deviation (MD) ungrouped data MD = [(X1- X̄ ) + (X2- X̄ ) + (X3- X̄ ) + _____ + (Xn - X̄ )] / n Example: Find Mean Deviation and Coefficient of Mean Deviation B e s t J A I I B C A I I B V i d e o , M o c k T e s t s , C a p s u l e P D F 35 | 257 Ambitiousbaba.com Paid Course Merits of Mean Deviation It is based on all observations. It is easy to understand and also easy to calculate. It is not affected by extreme values. Demerits of Mean Deviation Mean deviation ignores algebraic signs; hence it is not capable of further algebraic treatment. It is not very accurate measure of dispersion. Note: Mean deviation and its coefficient are used in studying economic problems such as distribution of income and wealth in a society. Standard Deviation And Coefficient Of Variation ▪ Standard deviation is the most important and commonly used measure of dispersion. ▪ It measures the spread or variability of a distribution. ▪ A small standard deviation means a high degree of consistency in the observations as well as homogeneity of the series. Standard Deviation ungrouped Data Standard Deviation (SD) grouped data B e s t J A I I B C A I I B V i d e o , M o c k T e s t s , C a p s u l e P D F 36 | 257 Ambitiousbaba.com Paid Course Example: Find Standard Deviation and Coefficient of Variation for the following data: 2, 3, 7, 8, 10. Example: Find Standard Deviation? Merits of Standard Deviation B e s t J A I I B C A I I B V i d e o , M o c k T e s t s , C a p s u l e P D F 37 | 257 Ambitiousbaba.com Paid Course It is rigidly defined and has a definite value. It is based on all observations. It is not affected much by sampling fluctuations. Demerits of Standard Deviation It is not easy to calculate. It is not easy to understand. It gives more weight to extreme items. Skewness And Kurtosis Skewness is the degree of distortion from the symmetrical bell curve or the normal distribution. It measures the lack of symmetry in data distribution. There are two types of skewness– positive and negative. If bulk of observations is in the left side of mean and the positive side is longer, it is called positive skewness of the distribution. mean and median > mode. If bulk of observations is in the right side of mean and the negative side is longer, it is called negative skewness of the distribution. mean and median < mode. Karl Pearson’s measure of skewness is B e s t J A I I B C A I I B V i d e o , M o c k T e s t s , C a p s u l e P D F 38 | 257 Ambitiousbaba.com Paid Course µ1 = First central moment = ∑f(x−x̅ )^ 1/ n µ2 = Second central moment = ∑f(x−x̅ )^ 2/ n µ3 = third central moment = ∑f(x−x̅ )^ 3/ n µ4 = Forth central moment = ∑f(x−x̅ )^ 4/ n Kurtosisis all about the tails of the distribution – peakedness or flatness. It is used to describe the extreme values in one versus the other tail. It is actually the measure of outliers present in the distribution. The distributions whose peaks are same as of Normal distribution’s peak, are called Mesokurtic. The distributions whose peaks are higher and sharper than mesokurtic, which meanstails are fatter, are called Leptokurtic distributions. The distributions whose peaks are lower and shorter than mesokurtic, which means tails are thinner, are called Platykurtic distributions. Measure of Kurtosis = β2 = µ4/µ2^2 µ4 = fourth central moment = ∑f(x−x̅ ) 4/ n µ2 = second central moment = ∑f(x−x̅ ) 2/ n β2 = 0 (Mesokurtic distribution), β2 > 0 (Leptokurtic distribution), β2 < 0 (Platykurtic distribution). B e s t J A I I B C A I I B V i d e o , M o c k T e s t s , C a p s u l e P D F 39 | 257 Ambitiousbaba.com Paid Course CAIIB Paper 1 (ABM) Module A Unit 4: Correlation and Regression Introduction Correlation Analysis Correlation analysis is applied in quantifying the association between two continuous variables, for example, an dependent and independent variable or among two independent variables. Regression Analysis Regression analysis refers to assessing the relationship between the outcome variable and one or more variables. The outcome variable is known as the dependent or response variable and the risk elements, and cofounders are known as predictors or independent variables. The dependent variable is shown by “y” and independent variables are shown by “x” in regression analysis. Linear Regression Linear regression is a linear approach to modelling the relationship between the scalar components and one or more independent variables. If the regression has one independent variable, then it is known as a simple linear regression. If it has more than one independent variables, then it is known as multiple linear regression. Linear regression only focuses on the conditional probability distribution of the given values rather than the joint probability distribution. In general, all the real world regressions models B e s t J A I I B C A I I B V i d e o , M o c k T e s t s , C a p s u l e P D F 40 | 257 Ambitiousbaba.com Paid Course involve multiple predictors. So, the term linear regression often describes multivariate linear regression. Correlation and Regression Differences There are some differences between Correlation and regression. Correlation shows the quantity of the degree to which two variables are associated. It does not fix a line through the data points. You compute a correlation that shows how much one variable changes when the other remains constant. When r is 0.0, the relationship does not exist. When r is positive, one variable goes high as the other goes up. When r is negative, one variable goes high as the other goes down. Linear regression finds the best line that predicts y from x, but Correlation does not fit a line. Correlation is used when you measure both variables, while linear regression is mostly applied when x is a variable that is manipulated. Comparison Between Correlation and Regression Basis Correlation Regression Meaning A statistical measure that Describes how an defines co-relationship or independent variable is association of two associated with the variables. dependent variable. Dependent and No difference Both variables are Independent variables different. B e s t J A I I B C A I I B V i d e o , M o c k T e s t s , C a p s u l e P D F 41 | 257 Ambitiousbaba.com Paid Course Usage To describe a linear To fit the best line and relationship between two estimate one variable variables. based on another variable. Objective To find a value expressing To estimate values of a the relationship between random variable based on variables. the values of a fixed variable. Correlation and Regression Statistics The degree of association is measured by “r” after its originator and a measure of linear association. Other complicated measures are used if a curved line is needed to represent the relationship. The above graph represents the correlation. The coefficient of correlation is measured on a scale that varies from +1 to -1 through 0. The complete correlation among two variables is represented by either +1 or -1. The correlation is positive when one variable increases and so does the other; while it is negative when one decreases as the other increases. The absence of correlation is described by 0. B e s t J A I I B C A I I B V i d e o , M o c k T e s t s , C a p s u l e P D F 42 | 257 Ambitiousbaba.com Paid Course Correlation Coefficient Formula B e s t J A I I B C A I I B V i d e o , M o c k T e s t s , C a p s u l e P D F 43 | 257 Ambitiousbaba.com Paid Course Simple Linear Regression Equation As we know, linear regression is used to model the relationship between two variable. Thus, a simple linear regression equation can be written as: Y = a + bX Where, Y = Dependent variable X = Independent variable a = [(∑y)(∑x2) – (∑x)(∑xy)]/ [n(∑x2) – (∑x)2] b = [n(∑xy) – (∑x)(∑y)]/ [n(∑x2) – (∑x)2] CAIIB Paper 1 (ABM) Module A Unit 5: Time Series Time Series B e s t J A I I B C A I I B V i d e o , M o c k T e s t s , C a p s u l e P D F 44 | 257 Ambitiousbaba.com Paid Course Secular trend is caused by basic inherent factors. Business cycle trends are mostly upward. The quality of forecast depends on the information provided by past data and its validity. Data or statistical information accumulated at regular intervals is called TIME SERIES. There are 4 types of variations in time series Secular Trend Cyclical Fluctuation Seasonal Variation Irregular Variation. B e s t J A I I B C A I I B V i d e o , M o c k T e s t s , C a p s u l e P D F 45 | 257 Ambitiousbaba.com Paid Course Secular Trend In this first type of variation the change comes over a long period of time. A steady increase in cost of living recorded by Consumer Price Index is a good example. From year to year there is a fluctuation but there is a steady increase in the trend. Let us see the series given here. Let us try to detect patterns in the information over regular intervals of time. Then let us try to predict to cope with uncertainty. Year 199 199 199 20 200 200 2003 7 8 9 W 1 2 Number 98 105 116 119 135 156 177 Observations There is an increase over time of 7 years. But the increases are not equal. Cyclical Fluctuation Most common example of a cyclical fluctuation is a business cycle. Over time, there are years when business cycle hits peak above the trend line. There are also times when business activity slumps, and hits a point below the trend line. Fluctuations in business activity occur many times, and they have irregular periods and vary widely in amplitude from cycle to cycle. The time between hitting peaks and lows are periods – it B e s t J A I I B C A I I B V i d e o , M o c k T e s t s , C a p s u l e P D F 46 | 257 Ambitiousbaba.com Paid Course can be one or many. The cyclical moves do not follow any regular pattern, they are irregular. Seasonal Variation There is a pattern of change within a year. A doctor can expect the number of flu cases to increase in winter. Hill resorts can expect more tourists during summer. These are regular patterns and can be used for forecasting the amount of flu vaccines required during winter, the doctor's income during winter, the hotel bookings in resorts and availability of air and train bookings. Irregular Variation The value of the variable is unpredictable, changing in a random manner. The effects of earthquakes, floods, wars, etc., cannot be predicted. As a result of flood, the agriculture output suffers. Then the prices go up at an unprecedented rate. This could not be predicted by using time series. Even though we described time series as exhibiting one or another variation, in most instances real time series will contain several of these components. Then the question is how to measure them. Trend Analysis There are three main reasons, why we should study the trends: We will be able to describe historical patterns, which will help us to evaluate the success of previous policies – long-term direction of the time series is given by secular trend. Past trends will help us to project the future – some growth rate of population, GDP. We will be able to separate the trend component and eliminate it from the series, to get an accurate idea of other components like seasonal fluctuations. B e s t J A I I B C A I I B V i d e o , M o c k T e s t s , C a p s u l e P D F 47 | 257 Ambitiousbaba.com Paid Course B e s t J A I I B C A I I B V i d e o , M o c k T e s t s , C a p s u l e P D F 48 | 257 Ambitiousbaba.com Paid Course Ye = a + bx The General equation for annual production Ye = 139.25 + 7.536x Estimate the no of unit ,it may produce during 2019 ‘x’ is coded time = 2(2019-2012.5) = 13 = 139.25 + 7.536 813 = 237.22 B e s t J A I I B C A I I B V i d e o , M o c k T e s t s , C a p s u l e P D F 49 | 257 Ambitiousbaba.com Paid Course = 237 Ships loaded Parabolic Equation: Many series may series can be best described by curves. In these cases, the linear model doesnot adequately describe the change in the change in variable as time changes. To overcome this, we use parabolic curves. Cyclical variation Cyclical variation is a component of the time series, which tends to oscillate above and below the secular trend line for periods longer than a year. Seasonal variation makes a complete regular cycle within each year and does not affect one year any more than another. Once we identify the secular trend, B e s t J A I I B C A I I B V i d e o , M o c k T e s t s , C a p s u l e P D F 50 | 257 Ambitiousbaba.com Paid Course we can isolate the remaining cyclical and irregular components of the trend. Let us assume cyclical component explains most of the variations left unexplained by the trend analysis. Residual Method ▪ Percentage of Trend = y actual / y trend *100 ▪ Relative cycle residual Multiply X by 2 if n is even Ye = a +bx b = ΣXY/ ΣX^2 = 168/ 168 = 1 a = y¯ - b x¯ B e s t J A I I B C A I I B V i d e o , M o c k T e s t s , C a p s u l e P D F 51 | 257 Ambitiousbaba.com Paid Course = 664/8 = 83 Ye= 83 + x Seasonal Variation Time series also includes seasonal variation. Seasonal variation is repetitive and predictable. This can be defined as movements around the trend line in one year or less. In order to measure seasonal variations, time intervals must be measured in small units, like days, weeks, etc. B e s t J A I I B C A I I B V i d e o , M o c k T e s t s , C a p s u l e P D F 52 | 257 Ambitiousbaba.com Paid Course Step 5 For modified mean : discard lowest and highest value Total of Indices = 404.10 STEP 6: Four quarter indices = 400 = 400/404.1 = 0.9899 Spring = 91.25 * 0.9899 = 90.3 B e s t J A I I B C A I I B V i d e o , M o c k T e s t s , C a p s u l e P D F 53 | 257 Ambitiousbaba.com Paid Course Summer = 107.7 *.9899 = 106.6 Fall = 112.1 Winter = 91 Irregular Variation The final component is irregular variation. After we have eliminated trend, cyclical and seasonal variations from the time series, we may still have unpredictable factor left. Irregular variations occur over very short intervals and follow random patterns. We may not be able to isolate them mathematically, but we may isolate the causes for the same. For example, an unusually very cold winter in a region may increase electricity consumption significantly. Wars may increase air and train travel because of the movement of troops. We may not be able to identify all causes. But over time, these random variations tend to correct themselves. STEP 1: First calculate Seasonal Indices Q1 = 95.1 Q2 = 129.9 Q3 = 61.2 Q4 = 113.9 Deseasonlised B e s t J A I I B C A I I B V i d e o , M o c k T e s t s , C a p s u l e P D F 54 | 257 Ambitiousbaba.com Paid Course STEP 2: TREND LINE (Ye = a + bx) a= y b = xy/x^2 STEP 3 : Trend Value Percent of Trend B e s t J A I I B C A I I B V i d e o , M o c k T e s t s , C a p s u l e P D F 55 | 257 Ambitiousbaba.com Paid Course Suppose management wants to determine the sales value for the 3rd qt of 6th year 23- 10.5 = 12.5 (coded X value) 12.5 * 2 = 25 Ye = a +bx = 18 + 0.16*25 = 22 Means 22000 units CAIIB Paper 1 (ABM) Module A Unit 6: Theory of Probability Introduction To Probability Probability means chance/s or possibility of happening of an event. For example, suppose we want to plan for a picnic in a weekend. Before planning we may check the weather forecast and see what is the chance that there will be rain at that time, accordingly we may do the planning. Probability gives a numerical measure of this chance or possibility. Suppose it says that there is a 60% chance that rain may occur in this weekend, 60% or 0.6 is called the probability of raining. To understand the concept of probability first we have to understand the concepts of Factorial, Permutations and Combinations. Factorial: B e s t J A I I B C A I I B V i d e o , M o c k T e s t s , C a p s u l e P D F 56 | 257 Ambitiousbaba.com Paid Course In mathematics, Factorial is equal to the product of all positive integers which are less than or equal to a given positive integer. The Factorial of an integer is denoted by that integer and an exclamation point. ▪ Thus, factorial five is written as 5! which is equal to 1 × 2 × 3 × 4 × 5 = 120 ▪ The product of the first n natural numbers is called factorial n and is denoted by n! = n × (n – 1) × (n – 2) × … × 2 × 1 ▪ The above formula can also be represented as n! = n × (n – 1) … (n – r + 1) × (n – r)! ▪ Where r < n It may be noted that: 0! = 1, 1! = 1 Permutations and Combinations ▪ A permutation is the arrangement of objects in which order is the priority. The fundamental difference between permutation and combination is the order of objects, in permutation, the order of objects is very important, i.e., the arrangement must be in the stipulated order of the number of objects, taken only some or all at a time. ▪ The combination is the arrangement of objects in which order is irrelevant. The notation for permutation is P (n, r) or nPr, denoting the number of permutations of n things when r things are selected at a time. ▪ If there are three things a,b, and c then permutations of three things taken two at a time is denoted by P (3, 2) or 3P2. ▪ It is given by (a, b), (a, c), (b, c), (b, a), (c, a), (c, b) = 6 ▪ In general, P (n, r) is the number of permutations when r things are selected at a time from n items. The notation for combination is C(n, r) or nCr which is the number of combinations or selections of n things if only r things are selected. If there are three things a, b and c then combination of these three things taken two at a time is denoted by 3C2 and is given by (a, b), (a, c), (b, c) = 3 B e s t J A I I B C A I I B V i d e o , M o c k T e s t s , C a p s u l e P D F 57 | 257 Ambitiousbaba.com Paid Course Example: Using 5 letter of word SHYAM, how many distinct word can be formed? N= 5 R= 5 5P5 = 5!/ (5-5)! = 5*4*3*2/0! = 5*4*3*2/1 = 120 Note: Permutation and Combination are related to each other by formula P(n,r) = r! * C(n,r). Example: In how many ways 3 pencils can be selected from 5 pencils? 3 pens can be selected from 5 pens in 5C3 ways 5C3 = 5! / 3! 2! × = 10 ways Example: From a group of 7 boys and 6 girls, 3 boys and 4 girls is to be selected. In how many ways this can be done? 3 boys can be selected from 7 boys in 7C3 ways = 7C3 = 7! /3! 4! × = 7* 6 *5 *4! /3*2*4! = 35 4 girls can be selected from 6 girls in 6 C4 ways = 6! 4! 2! = 6 *5 *4! /4! *2 = 15 3 boys and 4 girls can be selected in 7C3 × 6C4 = 35 × 15 = 525 ways. Random Experiment or Trial An operation or experiment conducted under identical conditions and which has a number of possible outcomes is called Random Experiment or Trial. Example: 1. Tossing a coin 2. Throwing a dice 3. Selecting a card form a pack of cards Sample Space and Sample Points The set of all possible outcomes of a random experiment is called sample space. The elements of the sample space are called sample points. Sample space is denoted by S. Example: 1. In an experiment of throwing a coin, S = {H,T] B e s t J A I I B C A I I B V i d e o , M o c k T e s t s , C a p s u l e P D F 58 | 257 Ambitiousbaba.com Paid Course 2. In an experiment of throwing a dice, S = {1, 2, 3, 4, 5, 6} The number of sample points in a sample space of random experiment is denoted by n (s). For example, (1) n (S) = 2, and example (2) n (S) = 6 Event Any subset of the sample space S is called an event. If S is a sample space and A is a subset of S (i.e., A⊂ S), then A is called an event. Example: In an experiment of throwing dice where S = {1, 2, 3, 4, 5, 6}, the event of getting odd numbers is A = {1, 3, 5} Types of Events Certain Event If sample points in an event are same as sample points in sample space of that random experiment, then the event is called a certain event. Example: Getting any number between 1 to 6 on a dice is a certain event. Impossible Events An event which never occurs or which has no favourable outcomes is called an impossible event. In other words, the event corresponding to the set φ (null set) is called an impossible event. Example: Getting a number 7 on a dice is an impossible event. Mutually Exclusive Events Events are said to mutually exclusive if the happening of any of them restricts the happening of the others i.e., if no two or more of them can happen together or simultaneously in the same trial. Example: In tossing a coin event head and tail are mutually exclusive. Note: If A & B are mutually exclusive events of sample space S, then A ∩ B = φ. Example: In tossing a coin event head and tail are mutually exclusive. Note: If A & B are mutually exclusive events of sample space S, then A ∩ B = φ. Equally Likely Events Events are said to be equally likely if they have equal choice to occur. In other words, outcomes of a trial are said to be equally likely if taking into consideration all relevant evidences, there is no reason to prefer one with respect to other. Example: In throwing a dice all the six faces are equally likely to occur. Exhaustive Events B e s t J A I I B C A I I B V i d e o , M o c k T e s t s , C a p s u l e P D F 59 | 257 Ambitiousbaba.com Paid Course ▪ If the sample points of the events taken together ▪ Note: If A & B are exhaustive events of sample space S, then A U B = S. ▪ Example: Random Experiment: ▪ Throwing a dice S = {1,2,3,4,5,6}, ▪ A= Event of odd numbers = {1, 3, 5} ▪ B= Event of odd numbers = {2, 4, 6}, ▪ C= Event of multiple of 3 = {3, 6} ▪ Here A U B = {1, 2, 3, 4, 5, 6} = S, ▪ Here A and B are called exhaustive events ▪ But A U C = {1, 3, 5, 6} ≠ S, ▪ so A and C are not exhaustive events. Complementary Event If A is an event in sample space S, then the non-occurrence event of A is called Complementary event of A. Two events A and B are called complementary events, if A and B exhaustive as well as mutually exclusive events. In other words, A and B are called complementary events if A U B = S and A ∩ B = φ. Example: Random Experiment: Throwing a dice, S = {1, 2, 3, 4, 5, 6}, A = {1, 2}, B = {3, 4, 5, 6} As A U B = S and A ∩ B = φ, A and b are complementary events. Complementary event of A is denoted by Ac, A/ or A. Mathematical Definition Of Probability If the sample space S of a random experiment consists of n equally likely, exhaustive and mutually exclusive sample points and m of them are favourable to an event A, then the probability of event A is given by B e s t J A I I B C A I I B V i d e o , M o c k T e s t s , C a p s u l e P D F 60 | 257 Ambitiousbaba.com Paid Course Number of favourable items/ Total number of outcomes Example: Two unbiased dice are thrown. Find the probability that: Both the dice show same number. First dice shows 6. The total of the numbers on the dice is 8. Solution In a random throw of two dice, the total number of cases is given below: A: Both the dice show same number n(A)/n(S) = 6/36 = 1/6 B: First die show 6 n(B)/n(S) = 6/36 = 1/6 C: Total of the number on the dice is 8 n(C)/n(S) = 5/36 Example: Two unbiased coins are tossed simultaneously. Find the probability of getting – at least one tail, B e s t J A I I B C A I I B V i d e o , M o c k T e s t s , C a p s u l e P D F 61 | 257 Ambitiousbaba.com Paid Course majority of heads S = {(H, H), (H, T), (T, H), (T, T)} n (S) = 4 (i)A: At least one tail, P (A) = n (A)/ n (S) = 3 /4 (ii)B: Majority of heads P (B) = n (B)/ n( S) = 1/ 4 Addition Theorem Let A and B are two events (subsets of sample space S) and are not disjoint, then the probability of the occurrence of A or B or A and B both, in other words probability of occurrence of at least one of them is given by, Example: Find the probability that a card drawn from a pack of cards will be a red or a picture card. Probability of selecting a red card = 26 = Event A P(A) = 26/52 = 1/2 Probability of getting picture card = 6 = Event B P(B) = 12/52 = 3/13 There are 6 red cards which are picture cards, P (A∩B) = 6/52 P (A U B) = P (A) + P (B) – P (A∩B) ½ + 3/13 – 6/52 = 8/13 B e s t J A I I B C A I I B V i d e o , M o c k T e s t s , C a p s u l e P D F 62 | 257 Ambitiousbaba.com Paid Course Conditional Probability ▪ The conditional probability of an event A is the probability that the event will occur given the knowledge that an event B has already occurred. P (A/B). ▪ If the events A and B are such that the occurrence of A doesn’t depend upon occurrence of event B, (A and B are independent event), the conditional probability of event A given event B is simply the probability of event A, that is P (A). B e s t J A I I B C A I I B V i d e o , M o c k T e s t s , C a p s u l e P D F 63 | 257 Ambitiousbaba.com Paid Course ▪ Similarly, probability of event B given that event A has already occurred is denoted by P (B/A). P (B/A) = P (A ∩ B) / P(A) Example: Consider a fair coin is tossed 3 times S = (HHH, HHT, HTH, TTT, TTH, THT, THH, HTT) = 8 Event A = Atleast two tail appear Event B – First coin show Head P(A) = (TTT, TTH, THT, HTT) = 4/8 = ½ P(B) = (HHH, HHT, HTH, HTT) = 4/8 = ½ P (A ∩ B) = 1/8 P(A/B) = 1/ 8 / ½ = 1/4 Multiplication Theorem ▪ If A and B are two events of a sample space S associated with an experiment, then the probability of simultaneous occurrence of events A and B is given by P (A ∩ B) = P(A) P(B/A) = P(B) P(A/B) Independent Events Two events A and B are independent of each other if the occurrence or non-occurrence of one does not affect the occurrence of the other. P (A ∩ B) = P(A) P (B) Example: Two balls are drawn from a bag one by one with 2 white and 3 black balls. What is the probability that the second ball is white? Event W1 = first ball - White Ball Event B1 = First Ball – Black Ball Event W2 = Second Ball – White Ball 1st White Ball = 2/5 + ¼ 2nd Black Ball = 3/5 + 2/4 P(W2) = P(W1) + P(W2/W1) + P(B1) + P(W2/B1) 2/5 + ¼ + 3/5 + 2/4= 2/5 B e s t J A I I B C A I I B V i d e o , M o c k T e s t s , C a p s u l e P D F 64 | 257 Ambitiousbaba.com Paid Course Random Variable ▪ A random variable is a function that associates a real number with each element in the sample space. ▪ In other words, a random variable is a function X: S → R, ▪ where S is the sample space of the random experiment under consideration and R is the real number line. Example. Consider the random experiment of tossing a coin two times and observing the result (a Head or a Tail) for each toss. Let X denote the total number of heads obtained in the two tosses of the coin. Example: Suppose that you play a certain lottery by buying one ticket per week. Let X be the number of weeks until you win a prize. X is a random variable. Discrete Random Variable: ▪ If a random variable takes a finite number or countable infinite number of possibilities, it is called a discrete random variable. ▪ Example: 1. Age in years 2. Number of arrivals in a clinic 3. Number of accidents Continuous Random Variable: ▪ If a random variable takes infinite number of possibilities, it is called a continuous random variable. ▪ Example 1. Percentage of marks 2. Weight 6.5 PROBABILITY Binomial Distribution ▪ Consider a random experiment consisting of n repeated independent trials with p the probability of success at each individual trial. Let the random variable X represent the number of successes in the n repeated trials. ▪ Then X follows a Binomial distribution. The definition of this distribution is: ▪ A random variable X has a binomial distribution, X ~ Binomial (n, p), if the discrete density of X is given by: P[X=x] = f(x) = nCx px (1 – p)n–x, x = 0, 1, 2..., n = 0 otherwise B e s t J A I I B C A I I B V i d e o , M o c k T e s t s , C a p s u l e P D F 65 | 257 Ambitiousbaba.com Paid Course f(x) = nCx px qn–x; x = 0, 1, 2..., n = 0 otherwise where p + q = 1 P = the probability of success n is the total number of trials. Example: Toss a coin for 10 times and you wan to get head 4 times & probability of coming head is 0.5 calculate f(x)? n = 10, x= 4 & p = 0.5 q = 1- 0.5 = 0.5 f(x) = nCx px qn–x = 10C4* 0.510 o.510 Binomial Distribution Real Life Examples Many instances of binomial distributions can be found in real life. If a new drug is introduced to cure a disease, it either cures the disease (it’s successful) or it doesn’t cure the disease (it’s a failure). If you purchase a lottery ticket, you’re either going to win money, or you aren’t. Basically, anything you can think of that can only be a success or a failure can be represented by a binomial distribution. Mean = np Variance = np (1 – p) = npq SD = √ variance Measure of Skewness = â1 = (1-2p)2/npq Measure of Kurtosis = â2 = 3 + [1 – 6pq /npq] Binomial Distribution is symmetric if p = q = 0.5 If p < 0.5, distribution is positively skewed and if p > 0.5, distribution is negatively skewed. Mode M= (n+1) p ▪ If M is not an integer, mode is the integral part lying between M – 2 and M. B e s t J A I I B C A I I B V i d e o , M o c k T e s t s , C a p s u l e P D F 66 | 257 Ambitiousbaba.com Paid Course ▪ If M is an integer, there are two modes and thus the distribution is bimodal, and two modes are M – 1 and M. Problem: If X follows Binomial distribution with n = 8, p = 1/2, then Find P [IX-4I ≤ 2] Solution : P[-2