Performance Attribution History and Progress PDF
Document Details
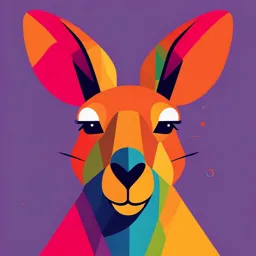
Uploaded by EasedOrangutan
Université du Québec en Abitibi-Témiscamingue (UQAT)
2019
Carl Bacon, CIPM
Tags
Summary
This document, from the CFA Institute Research Foundation, examines the history and progress of performance attribution. It explores different aspects including early development, foundations, multiperiod analysis, multicurrency attribution, types of attribution, risk-adjusted attribution, fixed-income attribution, and other key issues related to the subject.
Full Transcript
CFA INSTITUTE RESEARCH FOUNDATION / LITERATURE REVIEW PERFORMANCE ATTRIBUTION HISTORY AND PROGRESS CARL BACON, CIPM Research Foundation Literature Review PERFORMANCE ATTRIBUTION History and Progress Carl Bacon, CIPM Statement of Purpose The CFA Institute Research Foundation is a notfor-profit...
CFA INSTITUTE RESEARCH FOUNDATION / LITERATURE REVIEW PERFORMANCE ATTRIBUTION HISTORY AND PROGRESS CARL BACON, CIPM Research Foundation Literature Review PERFORMANCE ATTRIBUTION History and Progress Carl Bacon, CIPM Statement of Purpose The CFA Institute Research Foundation is a notfor-profit organization established to promote the development and dissemination of relevant research for investment practitioners worldwide. Neither CFA Institute Research Foundation, CFA Institute, nor the publication’s editorial staff is responsible for facts and opinions presented in this publication. This publication reflects the views of the author(s) and does not represent the official views of CFA Institute Research Foundation. CFA®, Chartered Financial Analyst®, and GIPS® are just a few of the trademarks owned by CFA Institute. To view a list of CFA Institute trademarks and the Guide for the Use of CFA Institute Marks, please visit our website at www.cfainstitute.org. © 2019 CFA Institute Research Foundation. All rights reserved. No part of this publication may be reproduced, stored in a retrieval system, or transmitted, in any form or by any means, electronic, mechanical, photocopying, recording, or otherwise, without the prior written permission of the copyright holder. This publication is designed to provide accurate and authoritative information in regard to the subject matter covered. It is sold with the understanding that the publisher is not engaged in rendering legal, accounting, or other professional service. If legal advice or other expert assistance is required, the services of a competent professional should be sought. Cover photo credit: egon69 / E+ / Getty Images ISBN 978-1-944960-89-6 Contents Introduction............................................................................................ Early Development. . ................................................................................ Foundations............................................................................................ Multiperiod Analysis. . .............................................................................. Multicurrency Attribution....................................................................... Types of Attribution................................................................................ Risk-Adjusted Attribution........................................................................ Fixed-Income Attribution. . ...................................................................... Other Attribution Issues.......................................................................... Conclusion: The Evolution of Attribution Methodologies. . ..................... Bibliography............................................................................................ 1 3 10 15 20 23 26 27 30 33 35 This publication qualifies for 2.5 CE credits under the guidelines of the CFA Institute Continuing Education Program. © 2019 CFA Institute Research Foundation. All rights reserved. iii Performance Attribution History and Progress Carl Bacon, CIPM Chief Adviser, StatPro Introduction The objective of performance attribution, as stated by Menchero (2000), is to explain portfolio performance relative to a benchmark, identify the sources of excess return, and relate them to active decisions by the portfolio manager. Hensel, Ezra, and Ilkiw (1991) defined attribution as the “mathematical process of explaining an investment return by relating it to the different risk-taking decisions implicit in the portfolio, and the extent to which each of those risks was rewarded or penalized in the capital markets” (p. 66). Colin (2014) said that the purpose of attribution is to disentangle the fund’s overall return into the component returns generated by each risk. In other words, attribution measures which of your investment decisions about the portfolio’s underlying risks worked and which did not. This information is critical business intelligence for anyone involved in selecting, managing, or marketing investments. As the authors noted above, Fischer and Wermers (2013) considered attribution analysis to be the “description and the quantification of key factors on the returns of investment portfolios” (p. 395). DiBartolomeo (2003) considered performance attribution to be the process of disentangling component portions of the observed returns to draw conclusions about the strengths and weaknesses of the investment process. Note that these definitions are similar. The central question of an attribution analysis is thus the following: To which investment decisions can the active return of a portfolio relative to its benchmark be traced over a given period? Formally, Bacon (2008) stated that “performance attribution is a technique used to quantify the excess return of a portfolio against its benchmark into the active decisions of the investment decision process” (p. 117). Murira and Sierra (2006) considered performance attribution the ex post complement to the ex ante risk decomposition provided by the risk measurement process. “Performance attribution” is, therefore, perhaps a misleading term: As these descriptions suggest, the excess, active, or relative return is examined, not the whole of the return (or absolute return). “Relative return attribution” © 2019 CFA Institute Research Foundation. All rights reserved. 1 Performance Attribution or simply “attribution analysis” is perhaps better terminology. Absolute attribution, or simply contribution analysis, would explain the sources of return of a portfolio in isolation rather than in comparison with a benchmark. As described in Bacon (2008), attribution analysis is a critical management tool for several key stakeholders in the asset management process. Above all, it is the main tool for performance analysts because it allows them to ensure that the return is correct. It allows them to participate in the investment decision process by enabling them to demonstrably add value and thus justify their salary and effort. Return attribution, together with risk analysis, is the tool that allows the analyst to understand the sources of return in the portfolio and to communicate that understanding to portfolio managers, senior managers, and asset owners. Investment management firms themselves, and the portfolio managers they employ, are also major users of attribution analysis. Attribution analysis provides a good starting point for a dialogue with asset owners on the positive and negative aspects of recent performance. Attribution analysis can be used aggressively to identify underperformance early and provide clients with a thorough explanation of underperformance and, hopefully, a rationale for improving performance. Attribution analysis is also crucial in gaining the confidence of asset owners by demonstrating a good understanding of the drivers of performance. Portfolio managers inhabit the front office of asset management firms; typically, performance analysts inhabit the middle office. The back office is also able to use daily, security-level attribution, however, as a diagnostic tool for identifying pricing and transaction errors. Bacon (2008) also stated that “senior management take an active interest in attribution analysis to provide them with a tool to monitor their portfolio managers. They will be keen to identify performance outliers—good or bad— and to ensure that value is added consistently across the firm” (p. 117). Hence, attribution analysis has numerous uses across a wide range of asset management stakeholders. Lord (1997) suggested that the characteristics of a good attribution system would include the following: •• a consistent approach for analysing returns, •• a solid theoretical framework for valuation, •• the ability to analyse portfolios and indexes consistently, •• attribution factors consistent with decision variables of the portfolio strategy process, 2 © 2019 CFA Institute Research Foundation. All rights reserved. Performance Attribution •• summary attribution analysis constructed from security details, which themselves should be available for inspection, •• transactions, and •• robust analytic systems and high-quality data to provide meaningful attribution results. Murira and Sierra (2006) suggested that there is general agreement that an attribution framework must meet two key requirements: 1. It should be consistent with the investment decision-making process of the portfolio. Performance attribution is most useful if it mirrors the investment management process because, in that case, it enables the identification and explanation of the sources of excess return. 2. It should be compatible with the organization’s performance measurement and risk measurement systems. Managers would have difficulty making effective use of the information provided by the performance attribution process if the data being explained by this process were inconsistent with the data originating from the internal risk and performance systems. As in many literature reviews, here the approach to the task is historical— beginning with the first attempts at performance measurement, about a halfcentury ago, and progressing to the present. Early Development Arguably, comparative performance measurement began with Dietz’s (1966) Pension Funds: Measuring Investment Performance and the Bank Administration Institute (BAI 1968) report Measuring the Investment Performance of Pension Funds for the Purpose of Inter-Fund Comparison. The purpose of the BAI study was to develop methods of comparing the performance of pension funds to differentiate between the abilities of their respective managers. The main conclusions of their study are still relevant: 1. Performance measurement returns should be based on market value, not cost. 2. Returns should be total returns. 3. Returns should be time weighted. 4. Performance should include risk as well as return. 5. Funds should be classified according to their investment objectives. © 2019 CFA Institute Research Foundation. All rights reserved. 3 Performance Attribution Although the report did not recommend the use of attribution analysis— instead, emphasizing the importance of the total return of the portfolio—it did suggest that comparison of various sector returns within the portfolio might be useful. Interestingly, Eugene Fama was later invited to write and wrote the chapter on risk for this report. Fama Decomposition. Fama was the first to fully delve into the subject of attribution analysis, which he did in “Components of Investment Performance” (Fama 1972). In this seminal paper, Fama suggested breaking down observed return into the part resulting from ability to pick the best securities at a given level of risk (which he at the time called “selectivity”; now, it is often referred to as “selection return”) and the part that is the result of predictions of general market price movements—that is, the return from systematic risk—as follows: r − rF = r − β × (b − rF ) − rF + β × (b − rF ), Excess return Selectivity (1) Systematic risk where: r = average portfolio return b = average market or benchmark return β = portfolio systematic risk rF = average risk-free rate If a portfolio is fully diversified, it incurs no specific risk and the total portfolio risk will equal the systematic risk. Portfolio managers will give up diversification, however, to seek additional return. Therefore, the return from selectivity can be broken down into (1) the additional return required to justify sacrificing a given amount of diversification and (2) the net return from selectivity (the remainder of the manager’s value added). The return from selectivity is equivalent to Jensen’s alpha, α. The term “diversification” (used in a different context from the one we would understand today) “is the return required to justify moving away from the benchmark and taking on specific risk” (Bacon 2013, p. 88). To calculate this diversification return, you first have to calculate the effective beta required such that systematic risk alone is equivalent to the total portfolio risk. This beta is the Fama beta, calculated as follows: βF = 4 σ , σb (2) © 2019 CFA Institute Research Foundation. All rights reserved. Performance Attribution where: σ = portfolio risk σ b = benchmark risk Therefore, the return required to justify not being fully diversified is calculated by using the difference between the Fama beta and the portfolio beta as follows: d = (β F − β) × (b − rF ). (3) Note that the “Fama beta will always be greater than or equal to the portfolio beta since total risk is greater than or equal to the systematic risk of the portfolio” (Bacon 2013, p. 89). The net return from selectivity (“net selectivity,” for short) is the remaining return from selectivity after deducting the amount of return required to justify not being fully diversified: Net selectivity = Selectivity – Diversification. Net selectivity = SNet = α − d . If net selectivity is negative, the portfolio manager has failed to justify the loss of diversification. Timing is the return from the manager’s variations in systematic risk (beta) around some policy or target amount: β × (b − rF ) = (β − β I ) × (b − rF ) + β I × (b − rF ), Systematic Risk Manager ’ s Systematic Risk or Timing (4) Investor ’ s Systematic Risk where β I = the investor’s systematic risk or target risk. Figure 1 illustrates Fama’s decomposition for portfolio A. A ′ represents the return from systematic risk (conventional beta) plus the risk-free rate, and A ′′ represents the return from the Fama beta plus the risk-free rate. Fama’s (1972) “Components of Investment Performance” is the first paper to seriously analyse the sources of return of an investment portfolio and, therefore, represents the birth of attribution analysis. Notional Funds. In response to the BAI (1968) paper, a working group was set up towards the end of 1970 by the Society of Investment Analysts (SIA) in the United Kingdom to make recommendations on the subject © 2019 CFA Institute Research Foundation. All rights reserved. 5 Performance Attribution Return from Systematic Return from Risk Selectivity Figure 1. Fama Decomposition r– A Net Selectivity A” Diversification A’ r–F β βF Systematic Risk (β) Source: Redrawn from a diagram in Fama (1972). of portfolio performance measurement for pension funds. Its approach, eventually published in 1972 (SIA 1972), had two aims: (1) the determination of a standard method of calculating a portfolio’s return, so that funds could be fairly compared, and (2) the decomposition of the performance of the fund into two components—selection of individual stocks and selection of stock sectors. The first aim is achieved by calculating the time-weighted rate of return using a simple approximation (which later became known as the analyst’s test method and ultimately became redundant as more accurate time-weighted returns became available). The second aim, the analysis of performance, is produced by constructing “notional funds” based on market indexes and comparing them with actual funds. This early work dealt with equity-market sectors as the macro level of selection and securities within the sectors as the micro level, but common practice now is to regard asset classes (stocks, bonds, etc.) as the macro level and securities within the asset class as the micro level. Thus, our discussion will proceed from this latter starting point. The working group established two notional funds as follows: •• a fully restrained fund (index returns, benchmark weights) and •• a partly restrained fund (index returns, actual weights). Note that the actual fund consists of actual returns and actual weights. The fully restrained fund (sometimes called the “fully notional fund”) represents the return on the policy portfolio—that is, the strategic benchmark or policy weights set by the asset owner (represented by the pension 6 © 2019 CFA Institute Research Foundation. All rights reserved. Performance Attribution fund trustee) multiplied by the market index returns for each asset class. In mathematical notation, i =n b = ∑Wi × bi , (5) i =1 where: i =n Wi = weight of the benchmark in the ith asset class (note ∑Wi = 1) i =1 bi = return of the index in the ith asset class The partly restrained fund (sometimes called the “semi-notional fund”) represents the intermediate return resulting from the actual weights determined by the manager multiplied by the market index returns. Again, in mathematical notation, i =n bS = ∑ wi × bi , (6) i =1 i =n where wi is the weight of the portfolio in the ith asset class (note ∑ wi = 1). i =1 The actual portfolio return in mathematical notation is i =n r = ∑ wi × ri , (7) i =1 where ri is the return of the portfolio assets in the ith asset class. The performance of a fund—in this case, a pension fund—thus depends on two factors: the selection of asset classes (typically, now described as asset allocation) and the selection of securities within an asset class. (As noted earlier, this analysis can also be applied to sector allocation and stock selection within sectors in an exactly parallel way.) The contribution of each of these components can be found by breaking down the transition from the actual fund to the fully restrained fund as shown in Table 1. Table 1. Source of Profit/Loss Selection of asset classes (asset allocation) Partly restrained fund minus fully restrained fund Selection of securities Actual fund minus partly restrained fund Total Actual fund minus fully restrained fund © 2019 CFA Institute Research Foundation. All rights reserved. 7 Performance Attribution The success of the manager’s asset allocation is measured by comparing the performance of the partly restrained fund with that of the fully restrained fund. The success of the manager’s security selection is measured by comparing the performance of the actual fund with that of the partly restrained fund. The SIA (1972) report is thus crucial because it introduces the concepts of macro and micro levels of manager decision making and intermediate notional portfolios to calculate these effects. In “Investment Performance of Pension Funds” (Holbrook 1977), the British actuary J.P. Holbrook, in effect, pulled together the BAI (1968) report, Fama’s (1972) decomposition, and the SIA (1972) report. This virtually unknown paper provides the foundation for much of the development in performance analysis that followed. Directing his advice to pension fund trustees, Holbrook defined the decision-making process as follows: The practical management of a pension fund investment portfolio involves decisions at three levels. i) Policy, i.e. the proportions of the assets which, as a long-term aim, the trustees wish to hold in the different investment markets. The fundamental element of policy is the split between equity-type and fixed-interest investments. Definition of policy may extend to a split of equity-type investments between UK equities, overseas equities and properties, specific proportions being set for each. If the trustees are not experts in long-term investment, they will tend to formulate policy in discussion with specialist advisers; it is gratifying to record that actuaries who advise on the financing of pension schemes are increasingly becoming involved in such discussions. ii) Strategy, i.e. decisions to depart from the policy proportions in the light of current market conditions, including decisions to hold part of the fund on short-term deposit. The trustees will usually seek advice from experts in the various markets regarding the short-term outlook, up to the time of the next investment meeting and over the next year or so. Except where trustees delegate strategic decisions to managers and advisers, investment meetings are usually held at short regular intervals, and arrangements are made for special meetings whenever it is thought that there has been a material change in market prospects. Often trustees authorize the managers concerned to determine the timing of purchases; thus, a manager is able to retain part of the assets entrusted to him in the form of shortterm deposits. 8 © 2019 CFA Institute Research Foundation. All rights reserved. Performance Attribution iii) Selection, i.e. the choice of the particular investments to hold, buy or sell within the various markets. Again, practice varies; many trustees give their managers carte blanche in this area— some keep close control over day-to-day transactions. (p. 20) Holbrook went on to describe the trustees’ needs. First, they need to know whether the fund performed well, moderately, or badly. This knowledge requires the calculation of return, comparisons with market returns, and consideration of risk: The performance of the fund as a whole, and within the different markets and sectors, must be examined separately. Secondly, the trustees need to be advised on the reasons for their fund’s performance; this may be investigated by analysing the results to show contributions to total performance made by policy, strategy, and selection decisions. Finally, the trustees need help in interpreting the results as a guide to future action. Identification of points of strength and weakness in past performance may lead to reconsideration of policy and practice, of the decision-making process, and possibly to a change of manager. (p. 21) To facilitate the breakdown of returns into policy, strategic, and selection decisions, Holbrook suggested the return calculation of three notional funds: rm = based on the model, r p = based on the trustees’ policy proportions, and rA = based on actual allocations. Then, the geometric excess return, g, for the whole fund is given by (1 + g ) = (1 + r ) (1 + r p ) (1 + rA ) (1 + r ) = × × . (1 + rm ) (1 + rm ) (1 + r p ) (1 + rA ) (8) The first factor represents the contribution to total performance resulting from the decision to adopt a policy that differs from the equity and fixedinterest proportions in the model [the industry standard allocation between equity and fixed income at the time]. The second, the contribution from the strategic decision to depart from policy proportions. The third, the contribution from selection decisions for the fixed-interest and equity sectors taken together. (Holbrook 1977, p. 31) This passage is the first articulation of successive notional portfolios each of which isolates one step in the investment decision process. The fact that it was written in 1977 shows how far ahead of his time Holbrook was. © 2019 CFA Institute Research Foundation. All rights reserved. 9 Performance Attribution Holbrook (1977) linked this successive notional portfolio approach to Fama’s decomposition by equating the first factor to the investor’s return from systematic risk, the second factor to the manager’s return from systematic risk, and the third factor to selectivity. Foundations The Brinson Model. The next major development in attribution analysis stems from a series of papers written in the 1980s that collectively describe Brinson attribution. Brinson, Hood, and Beebower’s (1986) “Determinants of Portfolio Performance” is perhaps the most well-known paper on attribution. It provided the name for the most common type of equity attribution model used today: the Brinson model. Despite being a relatively short paper, it made two contributions: 1. The general framework used to decompose total portfolio returns. Brinson et al. (1986) suggested a model for breaking down the arithmetic return in excess of the benchmark (r – b) under the assumption of a standard top-down investment decision process in which the portfolio manager seeks to add value through both timing and security selection. Figure 2 illustrates their framework for analysing portfolio returns. Quadrant I represents the policy benchmark return (b ) for a period—that is, the return on a hypothetical portfolio wherein the asset-class weights are those in long-term investment policy (Wi ) and the returns are those of the asset-class benchmarks (bi ). i =n Quadrant I = b = ∑Wi × bi . i =1 (9) Quadrant II represents the return effects of policy and timing. In timing, the portfolio manager seeks to add value by holding assetclass (or sector) weights in the portfolio that are different from the asset-class benchmark weights. An asset-class weight in the portfolio that is greater than the benchmark weight for that asset class would be described as overweight and a lesser weight would be described as underweight. Clearly, the portfolio manager will aim to overweight well-performing asset classes (or sectors) and underweight poorly performing asset classes. Quadrant II minus Quadrant I, therefore, describes the added or subtracted value from timing only. 10 © 2019 CFA Institute Research Foundation. All rights reserved. Performance Attribution Figure 2. The Brinson Quadrants Selection Passive Allocation Actual Actual Passive Quadrant IV Quadrant II Portfolio Return Policy & Timing r= i=n Σ wi × ri i=1 bS = i=n Σ wi × bi i=1 Quadrant III Quadrant I Policy & Selection Policy rS = i=n Σ Wi × ri i=1 b= i=n Σ Wi × bi i=1 Excess returns due to: Timing Security Selection Other Total II – I III – I IV – III – II + I IV – I Source: Brinson et al. (1986). Quadrant III represents returns resulting from policy and security selection combined. In security selection, the portfolio manager seeks to add value by selecting individual securities within the asset class or sector. Quadrant III minus Quadrant I, therefore, describes the added or subtracted value from selection only. Quadrant IV represents the actual return of the managed portfolio. Brinson et al. (1986) called the remaining term “other” or the “crossproduct” term. It is now more commonly considered to be “interaction.” Quadrant IV minus Quadrant III minus Quadrant II plus Quadrant I represents the added or subtracted value from interaction. 2. In a more controversial practice, the framework is used on a sample of pension funds to demonstrate that most of the difference in returns among pension funds is the result of policy decisions, not timing or selection. This use of the framework is responsible for much of its fame. © 2019 CFA Institute Research Foundation. All rights reserved. 11 Performance Attribution Attribution Formulas. Brinson et al. (1986) may be the better known of the “Brinson papers,” but the more important paper in terms of the development of attribution is “Measuring Non-US Equity Portfolio Performance” by Brinson and Fachler (1985). Again, it is a short paper, but it documented for the first time the formulas required to calculate individual attribution effects: nn Allocation (Brinson and Fachler [1985] used the term “market selection”). The contribution to excess return from asset allocation in the ith sector is Ai = (wi − Wi ) × (bi − b ). (10) nn Selection. The contribution to excess return from stock selection in sector i is Si = Wi × (ri − bi ). (11) nn Interaction (Brinson and Fachler used the term “cross-product”). The contribution to excess return from interaction in sector i is I i = (wi − Wi ) × (ri − bi ). (12) With a few modifications, these formulas provide the basic foundations for most of the attribution analysis used today. The terms “asset allocation,” “security selection,” and “interaction” are now universal and familiar to all. At the time, however, allocation might have been described as “timing,” “market selection,” or “weighting.” To establish when the term “allocation” was first used is difficult, but Cocks (1972) was among the first. He defined it as “all those decisions by which the portfolio manager allocates his funds among the selected securities (or broad classes of securities)” (p. 3). Asset allocation specifically locates the decision process in what asset classes to hold and in what weights, and this terminology is associated with Ibbotson and Sinquefield (1982), although no one knows when the term was first used. Use of the term “interaction” (or “other” or “cross-product”) is difficult to establish, but certainly, by the early 1990s, “asset allocation,” “security selection,” and “interaction” had become the most commonly used terms. The Brinson et al. (1986) paper did not provide a formula for individual attribution effects, but the following variation of the allocation formula is associated with that paper: 12 © 2019 CFA Institute Research Foundation. All rights reserved. Performance Attribution The contribution to excess return from asset allocation in the ith sector is Ai = (wi − Wi ) × bi . (13) It seems unlikely that Brinson et al. (1986) intended this interpretation, and the Brinson and Fachler (1985) variant for asset allocation is far more common and better aligned with most investment decision processes. If the Brinson et al. definition is used, the contribution from asset allocation will be positive for any overweight sector in which the sector return is positive, but it will be positive when using the Brinson–Fachler definition only if the sector return exceeds the overall benchmark return. Graphically, the Brinson et al. (1986) method is easier to illustrate for each sector i than the Brinson and Fachler (1985) method, as shown in Figure 3. The contribution to portfolio return from sector i is the total area ri × wi, and the contribution from the benchmark alone is area bi × Wi. In this example the total contribution from the portfolio in sector i is greater than the contribution from the benchmark. The attribution to excess return in sector i is the sum of the areas representing selection [Wi × (ri − bi )], allocation [(wi − Wi ) × bi ], and interaction [(wi − Wi ) × (ri − bi )]. In this particular example, ri > bi, bi > 0 and wi > Wi; therefore, all three contributions are positive. The Brinson and Fachler (1985) method illustrated in Figure 4 is a little less intuitive. As stated in Bacon (2008), “We observe no change to the areas representing selection and interaction but allocation is now described by the area (wi − Wi ) × (bi − b )” (p. 125). Figure 3. Brinson, Hood, and Beebower (1986) Attribution ri Selection Interaction Wi × (ri – bi) (wi – Wi) × (ri – bi) bi Benchmark Contribution Allocation Wi × bi (wi – Wi) × bi Wi wi Source: Bacon (2008). © 2019 CFA Institute Research Foundation. All rights reserved. 13 Performance Attribution Figure 4. Brinson and Fachler Attribution ri Selection Interaction (ri – bi) × Wi (ri – bi) × (wi – Wi) bi Allocation Wi × (bi – b) (wi – Wi) × (bi – b) b (wi – Wi) × b Wi × b Wi wi Source: Bacon (2008). The traditional formulas described in Brinson and Fachler (1985) were clearly in use well before 1985. The same formulas as those suggested by Brinson–Fachler and associated with Brinson et al. (1986) can be found in a formal discussion response to a paper by Kingston (1973) presented to the Faculty of Actuaries on 14 October 1974, more than 10 years before the Brinson–Fachler paper was published. The formulas may well have been developed prior to the publication of the Brinson and Fachler (1985), Brinson, Hood, and Beebower (1986), Brinson, Singer, and Beebower (1991), and Singer (1996) papers, but collectively these papers established attribution analysis as an important aid in the critical review of active strategies and decision-making processes for asset managers, asset consultants, and asset owners. Interaction. Interaction is a controversial effect. Most authors—for example, Laker (2001), Spaulding (2003/2004), Campisi (2004b), and Bacon (2008)—would agree that the interaction effect is a directly calculated term, not a residual, but what does it mean? . . . It is certainly not intuitive. As noted, Brinson and Fachler (1985) and Brinson, Hood, and Beebower (1986), arguably the first definers of the interaction effect, rather unhelpfully described this effect as “other” or a “cross-product”; they did not use the term “interaction.” By the early 1990s, the term had become fairly universal 14 © 2019 CFA Institute Research Foundation. All rights reserved. Performance Attribution in the literature, however, probably popularised by the software providers of the time. The interaction effect is clearly a cross-product, the combination of allocation and selection decisions. Laker (2000) suggested “that when calculated properly, the interaction term plays a valuable role in explaining a portfolio’s active performance, and indeed that sometimes it is clearly more explanatory than the other attributes” (p. 43). Spaulding (2003/2004) was more nuanced in his comments, despite pointing out potentially misleading results (from mispriced securities or investing in sectors not in the benchmark). On balance, Spaulding suggested, it is best to calculate interaction, take the time to do some analysis, and then decide where to assign it, or better yet, show the interaction as a separate item and prepare to explain it. Campisi (2004b) and Bacon (2008) were less supportive of using the interaction effect; they suggested that it is not an active part of the investment decision process and, therefore, need not be calculated. Like many asset managers in practice, they suggested including interaction in the selection effect. The decision to include interaction with stock selection is not arbitrary. If you assume that asset allocation decisions are taken first in a top-down investment process, then the attribution from stock selection can be derived from Quadrant IV minus Quadrant II in Figure 2 and interaction need not be calculated at all. The decision to include interaction with stock selection is, therefore, the direct result of the investment decision process. The contribution to stock selection in the ith sector is now Si = wi × (ri − bi ), (14) and Figure 4 can now be redrawn as shown in Figure 5 with selection represented by the area wi × (ri − bi ). Multiperiod Analysis The attribution models described so far work well for single periods with static data. No obvious way exists, however, to combine attribution effects over time. Because of the multiplicative (geometric) nature of returns, arithmetic excess returns fail to add up over multiple periods. Carino (1999, p. 5) eloquently explained the problem this way: Over multiple periods, the natural way to combine returns is to compound them. The compounded return R over t periods is R = (1 + ri ) × (1 + r2 ) × × (1 + rt ) − 1. [15] © 2019 CFA Institute Research Foundation. All rights reserved. 15 Performance Attribution Figure 5. Selection including Interaction ri Selection wi × (ri – bi) bi Allocation Wi × (bi – b) (wi – Wi) × (bi – b) b (wi – Wi) × b Wi × b Wi wi Source: Bacon (2008). And for the benchmark, B = (1 + bi ) × (1 + b2 ) × × (1 + bt ) − 1. [16] It is clearly unsatisfactory to simply add effects over time, because the sum of return differences does not equal the difference between compounded returns. Singer (1996, p. 54) made the same point: While it is tempting to simply add the individual periods to obtain multiperiod attribution results, it is wrong: R − B ≠ (ri − bi ) + (r2 − b2 ) + + (rt − bt ). [17] Nor is it satisfactory to compound the single period return differences because that result does not equal the difference in compounded returns either. Laker (2002) made the point through this equation: R − B ≠ (1 + r1 − b1 ) × (1 + r2 − b2 ) × × (1 + rt − bt ) − 1. 16 (18) © 2019 CFA Institute Research Foundation. All rights reserved. Performance Attribution Linking and Smoothing. Obviously, if multiperiod arithmetic excess returns do not add up, then clearly arithmetic attribution that adds up for single periods will not add up for multiple periods. Value added through active management decisions compounds over time, a fact that should be accounted for in multiperiod frameworks. Naturally, the end users of attribution analyses expect their analyses to add up without residuals. Solutions can be categorized into two types, smoothing algorithms, such as those described by Carino (1999) and Menchero (2000), and linking algorithms (sometimes described as “dollar attribution”), such as those in GRAP (1997), Frongello (2002a, 2002b), and Bonafede, Foresti, and Matheos (2002).1 The authors of each paper suggested that their method was the most appropriate to implement. Smoothing algorithms, in effect, take the resulting residual and methodically redistribute it across other factors. Carino (1999) used logarithms, and Menchero (2000) redistributed the residual in an optimised way that minimised the change in any single factor. GRAP (1997), Frongello (2002a, 2002b), and Bonafede et al. (2002) compounded attribution effects through time and achieved identical results, but crucially, their methods are order dependent: If the time periods are reversed, the attribution result will change. Frongello acknowledged the order dependence but regarded it as a strength; others, including Laker (2002), would describe it as a weakness. Davies and Laker (2001) suggested an “exact” method for using the Brinson model to calculate attributes over multiple periods at a portfolio level, but not sector level. Because of the so-called exact nature of their model, they suggested it would be helpful to evaluate the accuracy of other proposed methods by comparison with their method at the total portfolio level. Many articles have been written critiquing and defending existing methods and offering refinements and new methods, including Banchik (2004/2005), Carino (2002), David (2012/2013), Frongello (2002a, 2002b), Jiang and Saenz (2014/2015), Kirievsky and Kirievsky (2000, 2004), Laker (2002, 2005), Menchero (2003), Mirabelli (2000/2001), Reztsov (2011/2012), Spaulding (2002), and Wong (2003/2004, 2007). By and large, however, the end user has proven to be unconcerned about these black box approaches, provided that the multiperiod arithmetic attribution adds up without residuals. Frongello (2006) concluded that “although the mathematics can differ greatly from one method to another, the story told by the resulting attribution is the same regardless of the method” (p. 366). Software providers have typically found solutions but often keep their methods more or less proprietary. 1 © 2019 CFA Institute Research Foundation. All rights reserved. 17 Performance Attribution Geometric Attribution. Some would suggest that the problem with multiperiod attribution analysis stems from an incorrect definition of excess return itself. Bacon (2002) defined the arithmetic excess return (r − b ) as the profit in excess of the notional fund expressed as a percentage of the initial value of the portfolio. That work defined the geometric excess return, [(1 + r ) / (1 + b )] − 1, as the profit in excess of the notional fund expressed as a percentage of the final value of the notional fund. In other words, the geometric excess return measures the added value in terms of a percentage of the value of the notional fund assets at the end of the period, not the start, which is surely the main concern of any asset owner. Bacon (2002) suggested that geometric excess returns are preferable because they are compoundable (geometric excess returns compound over multiple periods without residuals), they are convertible among currencies, and they are proportionate. The preferred definition of excess return should determine the choice of attribution methodology. Bain (1996), Burnie, Knowles, and Teder (1998), and Bacon (2002) each presented a geometric attribution model. Although developed independently and presented in different ways, the models are, in fact, identical, which is an indication of the robustness of the approach. Geometric models built on the idea of successive notional portfolios can be found in Holbrook (1977), Hymans and Mulligan (1980), and Allen (1991). As shown in Figure 6, each successive portfolio Figure 6. Successive Notional Portfolios Step III Portfolio Return i=n r = Σ wi × ri i=1 Step II Semi-Notional i=n bS = Σ wi × bi i=1 Step I Benchmark Return i=n b = Σ Wi × bi i=1 Source: Bacon (2008). 18 © 2019 CFA Institute Research Foundation. All rights reserved. Performance Attribution represents an isolated decision in a top-down investment decision process. Step I represents the strategic, policy, or benchmark return. The difference between Step II and Step I represents the value added by active asset allocation, and the difference between Step III and Step II represents the value added by security selection. More steps can be introduced for more complex, multistep investment decision processes. In the geometric attribution model, the difference between each step is calculated geometrically: (1 + bS ) (1 + r ) (1 + r ) −1= × − 1. b (1 + b ) (1 + ) (1 + bs ) Allocation (19) Selection Because geometric excess returns compound through time and because geometric allocation effects and geometric selection effects compound together within each period, geometric allocation and geometric selection must also compound through time. Bain (1996), Burnie et al. (1998), and Bacon (2002) provided formulas for individual attribution effects as follows: nn Allocation. The contribution to geometric excess return from asset allocation in the ith sector ( AiG ) is 1 + bi AiG = (wi − Wi ) × − 1 . 1+ b (20) nn Selection. The contribution to geometric excess return from stock selection in sector i (SiG ) is SiG = wi × (ri − bi ) (1 + bS ) (21) or 1 + ri (1 + bi ) SiG = wi × − 1 × . 1 + bi (1 + bS ) (22) In geometric attribution, interaction is typically included in the selection effect, although a geometric interaction effect can be derived if desired (see Weber 2018). © 2019 CFA Institute Research Foundation. All rights reserved. 19 Performance Attribution Burnie et al. (1998) described perhaps the most well-known geometric methodology. Interestingly, they made the case for geometric attribution in a single-period context, not necessarily a multiperiod one. They also drew a distinction between top-down and bottom-up investment decision processes. A bottom-up investment decision process, they noted, assumes that security selection takes precedence over asset allocation or is performed first. Weber (2018) would consider the Bain (1996), Burnie et al. (1998), and Bacon (2002) models to be semi-geometric models. The reason is that although total selection and allocation effects compound to the geometric excess return, the effects within selection and allocation are still arithmetically calculated. Menchero (2001/2002) offered a fully geometric model in which individual selection and allocation effects compound through time. Multicurrency Attribution In this section, we consider three works specifically directed to performance attribution in currency management. Allen (1991). In an article entitled “Performance Attribution for Global Equity Portfolios,” Allen took the Holbrook (1977) concept of successive intermediate portfolios and applied it in a multicurrency environment. Allen stated that the trick to quantifying the impact of each type of active management decision involved calculating the return on a theoretical portfolio where one type of decision had been neutralised. Comparison of the return on the manager’s portfolio (where no decisions had been neutralised) with the return on the portfolio where a class of management decisions had been neutralised allowed the impact of those neutralised decisions to be measured. When carried out correctly, this process is quite neat. No unexplained residuals remain. Allen’s article provides a framework for quantifying the marginal impact that both policy decisions and active management decisions have on the performance of a global equity portfolio. At the heart of this framework is the assumption that the total return on an actively managed global equity portfolio is explained by seven types of decisions: 1. the choice, at the policy level, of the unhedged dollar-denominated index against which the active equity manager is to be measured 2. security selection decisions by the active manager within each country 3. overweighting or underweighting of a country relative to the index based on the manager’s expectation of the country’s equity returns 4. overweighting or underweighting of a country relative to the index based on the manager’s expectation of the country’s currency returns 20 © 2019 CFA Institute Research Foundation. All rights reserved. Performance Attribution 5. the timing of purchases and sales of securities (which implicitly affect the currency appreciation of the portfolio) 6. the choice, at the policy level, of the benchmark percentage of the portfolio that is to be hedged passively against currency fluctuation 7. active decisions in the currency forward markets that cause the portfolio’s return to deviate from that of the passively hedged benchmark Allen’s article is noteworthy in that despite being based in the United States where arithmetic attribution prevails, he favored a geometric approach because the attribution factors are computed on a multiplicative basis, so they can be “chain-linked” across any time period to generate a cumulative factor. This method allows for evaluation of the long-term contribution of a selected investment strategy to total return. Ankrim and Hensel (1992, 1994). In 1992, Ankrim and Hensel published a Russell Research Commentary setting out a framework for multicurrency performance attribution. This article, building on work by Brinson and Fachler (1985), separated out the impact of currency decisions—in particular, it recognised the significance of differential interest rates in currency decision making. Ankrim and Hensel proposed a method of performance attribution that retains the simplicity and intuitive appeal of the Brinson and Fachler approach but breaks the returns from currency into two components: one that recognizes the opportunity cost of returns achievable in forward-currency markets and a second that measures the return attributable to the currency being less than fully hedged, both in the portfolio and in the benchmark with which the portfolio is compared. (p. 2) In the 1992 paper, Ankrim and Hensel identified three reasons that including currency exposure in performance attribution causes problems: First, and most obvious, it alters the returns ultimately received by the client. In Brinson and Fachler [1985], all returns are denominated in the home currency, but this convention makes it impossible to separate the contribution to returns made by the manager’s country and security selection decisions from the impact of currency translation gains and losses. Second, in practice, many portfolios have some portion (but not necessarily all) of their currency exposure hedged away. This requires an approach to performance attribution that allows the proportion of currency exposure hedged to be variable. Third, even if a portfolio is completely hedged, the client may choose to adopt a benchmark that is less than fully hedged. (p. 2) © 2019 CFA Institute Research Foundation. All rights reserved. 21 Performance Attribution The authors defined two components of currency return: currency surprise and the forward premium. Currency surprise is uncertain, but the forward premium element is not because it depends on interest rate differentials at the time of purchase: Any approach to multicurrency attribution that lumps the forward premium and currency surprise together will credit positive performance to managers who have large exposures in countries with positive forward premiums. Such an approach would penalize managers who hold large positions in countries with negative forward premiums. (p. 12) Karnosky and Singer (1994). Arguably the single most important paper published on attribution in the 1990s is Karnosky and Singer. This paper not only demonstrates that managing multicurrency portfolios is sub-optimal if currency is not managed independently but also provides a framework for calculating attribution effects, while critically, taking into account interest rate differentials. Karnosky and Singer resolved the issue of market and currency compounding by using continuously compounded returns in their model, admittedly this is only referred to in the small print, and solved the forward premium concern by thinking in terms of a “return premium” above local interest rates. Karnosky and Singer explained that the term “return premium” is used, rather than the more familiar “risk premium,” to make a subtle distinction in the underlying cash return. “Risk premium” refers to the return of a risky asset in excess of the return of a riskless asset, but in the authors’ analysis, the premiums depend on forward contracts with long maturities, where risk is probably not the dominant factor; hence, the term “return premium” is used. Karnosky and Singer’s framework demonstrates that separate currency and market strategies can be implemented within a global portfolio in which a specific currency weight is different from the weight of the associated country market. In effect, the authors applied the standard Brinson methodology twice—once to global portfolios by using local-return premiums (rather than simply local returns) to measure allocation and selection effects and, separately, to cash returns in the base currency of the asset owner in order to calculate independent currency effects, as shown in Figure 7. 22 © 2019 CFA Institute Research Foundation. All rights reserved. Performance Attribution Figure 7. Karnosky and Singer (1994) Multicurrency Attribution Excess Return Local-Return Premium Attribution Currency Attribution Quadrant IV Quadrant II Quadrant IV Quadrant II Quadrant III Quadrant III Quadrant I Quadrant I Source: Bacon (2008). Types of Attribution Three types of attribution have been identified, and they are characterized by the information used to calculate attribution effects, as follows: 1. Holdings-based attribution. Holdings-based or position-based attribution is calculated by analysing the underlying beginning-period holdings of the portfolio only. Typically, holdings-based attribution is calculated from monthly, weekly, or daily data, with the shorter time periods leading to greater accuracy. 2. Transaction-based attribution. Transaction-based attribution is calculated by using both the holdings of the portfolio and the transactions (purchases and sales) that occurred during the evaluation period. 3. Returns-based attribution. Returns-based (or factor) attribution uses the historical returns of the total portfolio and/or individual securities to decompose the total return into multiple factors. The multifactor model is a regression equation that describes the way a particular security or portfolio reacts to industry sector, fundamental, macroeconomic, and other market factors. © 2019 CFA Institute Research Foundation. All rights reserved. 23 Performance Attribution Asset-Grouping Attribution. The type of attribution favoured by the asset manager or asset owner will vary according to the investment decision process, investment objective, quality and availability of data, cost and time considerations, complexity, and reporting objective. Holdings-based and transaction-based attributions are mutually exclusive, and the choice is based on (for holdings based) cost, quality of data, ease of use, and rapid implementation against (for transaction based) the advantages of reconciliation to the client-reported return, completeness, and accuracy. A number of authors— Giguere (2003), Bonafede and McCarthy (2003), Spaulding (2003a), Menchero and Hu (2003), and Bacon (2008)—argued for one approach or another. Spaulding, without an eye to existing software (and thus genuinely independently), concluded that the question “which is better?” does not have a simple answer. Too many variables are at work; in the end, the choice comes down to user preference. In later research, Spaulding (2018) showed that the residual implicit in holdings-based attribution can be extensive and is not necessarily correlated with turnover. Both holdings-based and transaction-based attribution are also described as “asset-grouping” or “variance type” attribution, reflecting their requirements for asset allocation data and variance from benchmark data. Attribution Based on Returns. Returns-based attribution, in contrast to the asset-grouping approach, might complement either holdings- or transaction-based attribution in that it might reveal unexpected biases and make communication easier for asset managers using a factor-focused investment decision process. Returns-based attribution is a direct descendant of Fama (1972) decomposition, which is a single-factor model, via Sharpe (1988) and Fama and French (1993)—both three-factor models that include a general market factor together with a size and value factor—and Carhart (1997), a four-factor model that introduced a momentum factor. Amenc, Sfeir, and Martellini (2003) described four types of factor models: 1. Implicit factor model. In this model, some factor analysis (e.g., a principal components analysis) is performed to statistically extract the factors from the return’s time series. These factors may not be easily interpretable. 2. Explicit macro factor model. In this approach, macroeconomic variables are used as factors. 3. Explicit micro factor model. In this approach, microeconomic attributes are used as factors. 4. Explicit index factor model. In this approach, stock market indexes (including style subindexes of the market) are used as factors. 24 © 2019 CFA Institute Research Foundation. All rights reserved. Performance Attribution Within this framework, Amenc and Le Sourd (2003) described a wide range of multifactor models in detail. William F. Sharpe, who is associated with style attribution starting with his 1988 paper (see Sharpe 1988, 1992), used a 12-factor model to determine the passive return of a portfolio with the same style as the active asset manager. The difference between the actual portfolio return and the passive return with the same style would be a result of the manager’s selection ability. This type of style analysis provides a natural method for constructing appropriate style benchmarks. Sharpe (1988) stated that the usefulness of the asset-class model depends on the asset classes chosen for implementation. It is desirable that asset classes •• be mutually exclusive, •• be exhaustive, and •• have returns that differ. Each asset class should be proxied by a market capitalization–weighted index of the securities in it, and no security should be included in more than one asset class. Also, the asset classes should have low correlations with each other (or different standard deviations if correlations are high, as is the case in many fixed-income styles or asset subclasses). Surz (1999/2000) suggested a holdings-based or transaction-based form of style attribution based on the exclusive style characteristics of the holdings in the portfolio and benchmark. Clarke, de Silva, and Thorley (2005) proposed a regression-based attribution system linked to the parameters from the “Fundamental Law of Active Management” (Grinold and Kahn 1995, pp. 117–35). In a slight oversimplification, the fundamental law says that alpha is a function of manager skill as well as breadth (the number of independent bets made by the manager). The investigation by Clarke et al. (2005) indicated that the “law can be used to estimate and interpret the regressionbased results without substantial error while showing the investor how much of the active return is related to forecasting accuracy and how much is noise related to constraints in constructing the portfolio” (p. 81). Returns-based or regression-based attribution has the following characteristics: •• It does not necessarily require the composition of the portfolio. •• It complements traditional holdings- or transaction-based attribution. •• It is relatively easy and cost-effective to implement. © 2019 CFA Institute Research Foundation. All rights reserved. 25 Performance Attribution •• It is potentially most consistent with the investment decision process of the asset manager. •• It is easy to interpret. However, this method does have limitations: •• It is less accurate than other methods, particularly for concentrated strategies. •• It relies on the appropriate definition of factors. Risk-Adjusted Attribution Campisi (2000) listed the characteristics of a good performance attribution system as follows: •• It is consistent with the investment process and the manager’s decisionmaking process. •• It uses a benchmark that reflects the manager’s strategic (long-term) asset allocation. •• It measures the effect of the manager’s tactical (short-term) allocation shifts. •• It adjusts attribution of return for systematic risk(s). Campisi (2000) suggested that many attribution systems fail at least one of these criteria and some fail all of them. Often, this failure occurs because returns are not adjusted for systematic risk and style (high vs. low beta, large vs. small size, value vs. growth orientation, etc.). Simply applying the allocation/selection model often results in attribution results that are not necessarily meaningful because they do not reflect the investment process or the risks that drove return over the evaluation period. Risk-adjusted attribution is the real Cinderella of attribution analysis: In practice, risk-adjusted attribution is rarely performed. Ankrim (1992) proposed a form of risk-adjusted attribution using beta, as did Bacon (2008) and Obeid (2005). Kophamel (2003), thinking in terms of the volume of “performance cubes” rather than the familiar “performance areas” of the Brinson model, proposed attribution analysis in three dimensions. Spaulding (2016) suggested adjusting for total risk rather than systematic risk—in effect, attributing M2 returns (described in Modigliani 1997).2 Rather satisfactorily, Fisher and D’Alessandro (2019) Modigliani risk-adjusted performance (also known as M 2, M2, the Modigliani–Modigliani measure, or RAP) is a measure of the risk-adjusted returns of some investment portfolio. It measures the returns of the portfolio adjusted for the risk of the portfolio relative to that of some benchmark (e.g., the market). 2 26 © 2019 CFA Institute Research Foundation. All rights reserved. Performance Attribution took attribution full circle and tied risk-adjusted attribution back to the original Fama decomposition. Van Breukelen (2000) suggested a form of risk-adjusted attribution for fixed-income portfolios that takes into account the weighted duration “bets” of portfolio managers. Va