BRCA Thesis Capstone PDF
Document Details
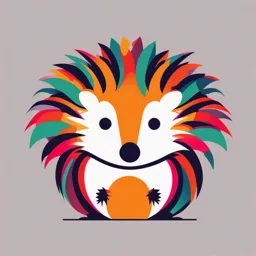
Uploaded by GrandAphorism
Abu Dhabi University
2024
Tags
Summary
This document is a thesis analyzing family history, genetic variants, and demographic factors influencing breast and ovarian cancer testing outcomes in the UAE. The study was conducted at Abu Dhabi University.
Full Transcript
Spring 2023-2024 BMS 44910C A Comprehensive Analysis of Family History, Inherited Genetic Variants, and Demographic Factors Influencing Breast and Ovarian Cancer Testing Outcomes Principle Investigator: Dr. Afsheen Raza and Dr. Hem...
Spring 2023-2024 BMS 44910C A Comprehensive Analysis of Family History, Inherited Genetic Variants, and Demographic Factors Influencing Breast and Ovarian Cancer Testing Outcomes Principle Investigator: Dr. Afsheen Raza and Dr. Hemad Yasaei Students: Malak Issa (1080383), Nabia Naushad (1080779), Nadeen Quzah (1078831), Noura Nedal (1079692), Saja Masalmeh (1078614) Institutes: Abu Dhabi University and National Reference Laboratory Course Instructor: Dr Abdulmajeed Almutary Submission Date: 06/06/2024 1 Table of Contents Abstract................................................................................................................................................. 6 1.0 Introduction.................................................................................................................................... 7 Genetic Mechanisms and Cancer Risk......................................................................... 7 Familial Breast Cancer (FBC) and BRCA Mutations.................................................... 8 Other Inheritable Mutations Increasing Ovarian Cancer Risk........................................ 9 1.1 Literature Review.......................................................................................................................... 9 1.2 Demographic Factors in Genetic Testing............................................................. 10 1.3 Prevalence and Impact of BRCA Mutations........................................................ 11 1.4 Genetic Variants Beyond BRCA......................................................................... 12 1.5 Role of Family History and age in Cancer Risk.................................................... 14 2.0 Prevalence of Hereditary Cancer Genes in the UAE Population........................................ 15 3.0 Technological Advancements in Genetic Screening.......................................................... 16 4.0 Clinical Implications and Management......................................................................... 18 7.0 Methodology................................................................................................................................. 19 7.1 Study Design and Population............................................................................. 19 7.2 Data Collection and Management...................................................................... 21 7.3 Statistical Analysis............................................................................................ 22 7.5 Genetic Variants Analysis................................................................................... 23 7.6 Correlation Analysis.......................................................................................... 23 7.8 Database Matching............................................................................................ 24 7.9 Gene Variants................................................................................................... 24 8.0 Software and Tools....................................................................................................... 25 Group 2: Patients of Arab Ethnicity........................................................................... 29 Group 3: Patients of non-Arab Ethnicity.................................................................. 34 Group 4: Patients with Positive Genetic Results for Breast/Ovarian Cancer................... 40 Group 5: Patients with Positive Family History of BC/OC............................................ 43 Group 6: Patients with "Uncertain Significance" Results............................................ 44 Group 7: Unspecified Family history, positive for Breast Cancer................................... 47 Group 8: Ovarian Cancer Patients............................................................................ 47 2 Group 9: Males....................................................................................................... 47 ClinVar Results...................................................................................................... 48 Clinvar Associated conditions.................................................................................. 52 10.0 Discussion.................................................................................................................................... 53 11.0 Conclusion................................................................................................................................... 63 12.0 Future Prospects........................................................................................................ 65 References........................................................................................................................................... 67 3 Tables Table 1: Pathogenic/Likely Pathogenic Variants Identified in BRCA1/2 Mutations from Previous Studies……………………………………………………………………………………..12 Table 2: Common Genes and their Associated Cancers................................................................. 14 Table 3: Demographic Distribution.................................................................................................. 26 Table 4: Pathogenic Variants Identified in Arab Population…………………………….……….31 Table 5: Type of Pathogenic Variants in Arab Population………………………………………..32 Table 6: VUS Identified in Arab Population with Confirmed Family History………………….33 Table 7: Type of VUS in Arab Population…………………………………………………………34 Table 8: Pathogenic Variants Identified in Non-Arab Population……………………………….37 Table 9: Type of Pathogenic Variants Identified in Non-Arab Population………………...……37 Table 10: VUS Identified in Non-Arab Population with Confirmed Family History…………..39 Table 11: Type of VUS in Non-Arab Population with Confirmed Family History...................... 39 Table 12: Distribution of Pathogenic Variants Identified in Arab vs Non-Arab Populations.... 42 Table 13: Distribution of Variants of Uncertain Significance Identified in Arab vs Non-Arab Populations with Confirmed Family History.................................................................................. 46 Table 14: Genetic Variants with Conflicting Interpretations......................................................... 51 Table 15:Associated conditions with Variants of Uncertain Significance found in study population........................................................................................................................................... 52 4 Figures Figure 1: Next Generation Sequencing Workflow…………………………………………….…..18 Figure 2: CONSORT diagram showing the flow of participants, exclusion, and data categories…………………………………………………………………………………………….20 Figure 3: Age vs Genetic Result……………………………………...……………………….……27 Figure 4:Genetic Result vs Ethnicity…………………………………………………..…………..27 Figure 5: Distribution of Genetic Test Results by Age Group…………………………………....27 Figure 6: Spearman’s Correlation for age vs Pathogenic Classification………………….……..28 Figure 7: Distribution genetic results across age groups in Arab Population…………………..29 Figure 8: Spearman’s Correlation for Age vs Pathogenic Classification in Arab Populations…………………………………………………………………………………………..30 Figure 9: Distribution Genetic Results Across Age Groups in non- Arab Population…….……34 Figure 10: Spearman’s Correlation to Compare Age and Pathogenicity in non-Arab Population………………………………………………………………………………………...…35 Figure 11: Distribution of Variant Types Across Different Genes………………...……………..40 Figure 12: Distribution of Genes Affected Between Both Ethnicities………………...…………40 Figure 13: Comparing Positive Result for Arabs & Non-Arabs VS. Age………..……….……..41 Figure 14: Comparison of Age with the Type of Variant…………………………………………43 Figure 15: Comparison of the Age of Arab & Non-Arab + Family History…………..………...43 Figure 16: Genetic Result vs Ethnicity……………..….…………………………………………..44 Figure 17: VUS Genes Distribution……………………………………………..…………………45 Figure 18: Type of Mutation Across Genes Affected……………………………………….……..45 Figure 19: Distribution of genetic variants according to ClinVar interpretation (N = 621), and the absolute number of variants with conflicting interpretation by gene (n = 148)……..……...49 Figure 20: Variants with Multiple Submissions of Conflicting Interpretation of Pathogenicity………………………………………………………………………………………...49 Figure 21: Distribution of All Genetic Variants with Multiple Submissions in ClinVar by Gene……………………………………………………………………………………………….....50 Figure 22: Distribution of Pathogenic Genetic Variants in Arab and Non-Arab Populations…………………………………………………………………………………….……56 Figure 23: Distribution of the Type of Pathogenic Genetic Variants in Arab and Non-Arab Populations……………………………………………………………………………………......…57 Figure 24: Distribution of the Gene Affected by a VUS in Arab and Non-Arab populations.....57 Figure 25: Distribution of the VUS Types in Arab and Non-Arab Populations…………..…….58 Figure 26: Proposed Plan of Action When VUS Are Detected……………………….………..…62 5 Abstract This study explores the genetic predispositions to breast and ovarian cancer within the diverse population of the United Arab Emirates (UAE), with a specific emphasis on distinguishing between Arab and non-Arab subsets. Key findings highlight the prevalence of pathogenic and potentially pathogenic mutations in hereditary cancer genes, with notable ethnic-specific variations identified— such as BRIP1, APC, and SDHA among Arabs, and PALB2, CHEK2, and NF1 among non-Arabs. Despite demographic factors showing no statistically significant associations with pathogenic classifications, trends suggest potential insights with larger sample sizes. The study underscores the complex interplay between genetic findings, family history, and cancer risk, emphasizing the need for enhanced genetic counseling. Comparison with existing research underscores variations in genetic mutation frequencies among different ethnic groups, advocating for tailored screening strategies in ethnically heterogeneous populations like the UAE. The study also addresses the challenges posed by variants of uncertain significance (VUS), particularly prevalent in Arabs, necessitating further functional studies for clinical validation. Insights from the ClinVar database support broader implications of these VUS beyond breast and ovarian cancer, enhancing early diagnosis efforts. Moreover, the study introduces "VUS Guidelines" based on cohort-specific variant data to improve genetic counseling and early intervention strategies. It advocates for personalized screening approaches in precision medicine, highlighting the clinical impact of variant interpretation discrepancies, especially in genes like ATM, PMS2, and PALB2. 6 1.0 Introduction Hereditary breast and ovarian cancer (HBOC) are part of a broader category of diseases known as hereditary cancer syndromes, which also include conditions like Bloom syndrome, Fanconi anemia, Nijmegen breakage syndrome, and Ataxia-telangiectasia. These syndromes are characterized by the inheritance of pathogenic mutations that significantly increase the risk of developing various cancers. HBOC, specifically, is associated with mutations in the BRCA1 and BRCA2 genes, which are crucial for DNA repair and maintaining genomic stability (Imyanitov et al., 2023). Individuals with mutations in BRCA1 and BRCA2 genes carry a defective protein function and face a higher likelihood of developing breast and ovarian cancers, often at a younger age than the general population (Imyanitov et al., 2023). Hereditary cancer syndromes are typically defined by the presence of inherited mutations that predispose individuals to multiple cancer types. These mutations often result in the biallelic inactivation of genes necessary for DNA repair, leading to significant immunological deficits and an increased cancer risk. Approximately 2% of healthy individuals may carry pathogenic mutations linked to a higher risk of developing certain malignancies ( Imyanitov, et al., 2023). Understanding the mutations in these syndromes is vital for improving cancer prevention, early detection through population screening, and personalized treatment strategies. Genetic Mechanisms and Cancer Risk Human cells can usually withstand a single mutation in a cancer-related gene due to various defensive mechanisms. However, cancer generally requires multiple mutations in the same cell. Inheriting mutations in cancer-related genes significantly increases the risk of developing cancer compared to the general population. For instance, carriers of a germline pathogenic variant (PV) can have an estimated 40% to 80% probability of developing cancer during their lifetime, which is significantly higher than the general population (Imyanitov et al., 2023). Cells accumulate numerous mutations throughout life, but most do not lead to cancer unless they specifically inactivate key tumor suppressors or activate oncogenes (Moore et al., 2021). The body employs various mechanisms to repair DNA and eliminate cells with potentially harmful mutations, thereby preventing cancer development (Moore et al., 2021). Most hereditary cancer genes are tumor suppressors that must be inactivated in both copies to cause cancer, as seen in genes like RB1, BRCA1, BRCA2, MLH1, and MSH2. Some genes, like PALB2 and CHEK2, can cause cancer if only one copy is mutated due to haploinsufficiency, while others require both copies to be mutated (Imyanitov et al., 2023). Genetic pathways are critical for understanding cancer risk, particularly in hereditary 7 malignancies like breast and ovarian cancer. An estimated 5-10% of breast cancer cases and a significant proportion of ovarian cancer cases are caused by inherited genetic abnormalities (Yoshida, 2021). The most well-known genes linked to increased cancer risk are BRCA1 and BRCA2, which are critical for the repair of DNA double-strand breaks via homologous recombination, necessary for genomic stability. When these genes are altered, the DNA repair process is disrupted, resulting in the accumulation of genetic damage and a considerably increased risk of developing cancer (Yoshida, 2021). Mutations in BRCA1 and BRCA2 are not the only genetic changes linked to an elevated risk of breast and ovarian cancer. Advances in next-generation sequencing (NGS) technology have allowed for the discovery of numerous other non-BRCA genes contributing to hereditary breast and ovarian cancer, such as TP53, PTEN, and PALB2. Each of these genes is involved in diverse cellular processes like DNA repair, cell cycle regulation, and apoptosis, and mutations can disrupt these processes, leading to cancer development (Yoshida, 2021). Familial Breast Cancer (FBC) and BRCA Mutations Familial breast cancer (FBC) refers to the 15% of breast cancer patients with multiple family occurrences of breast or ovarian cancer (Yoshida, 2021). Individuals with a hereditary predisposition to cancer fall under FBC. According to the National Cancer Institute, HBOC is an inherited condition that significantly increases the risk of ovarian and breast cancer, particularly before age 50. BRCA1 or BRCA2 gene mutations account for most HBOC cases (Yoshida, 2021). Additionally, those with HBOC have an elevated risk of prostate, pancreatic, and melanoma cancers (Yoshida, 2021). BRCA1 and BRCA2 are tumour suppressor genes involved in repairing DNA double-strand breaks by homologous recombination, thereby maintaining genomic stability. They also regulate centrosome dynamics, chromosomal segregation, and cytokinesis, contributing to cellular stability (Yoshida, 2021). Disruption of BRCA1/2 functions leads to genomic instability and a hormone-dependent carcinogenic environment, promoting breast cell malignancy. BRCA1 also plays crucial roles in embryonic development, centrosome replication, spindle pole production, and other cellular activities (Yoshida, 2021). ClinVar, a public archive of human genetic variants, lists approximately 2,900 pathogenic BRCA1 variants and over 3,400 BRCA2 variants (Yoshida, 2021). About 80% of these variants result in premature stop codons, truncating the protein and reducing its production through nonsense-mediated mRNA degradation (Yoshida, 2021). Pathogenic missense mutations, which account for 10% of all variants, frequently occur in critical domains such as the RING and BRCT domains of BRCA1 or the 8 OB-fold and helical domains of BRCA2. Additionally, copy number anomalies are observed in about 10% of cases, varying by population (Yoshida, 2021). The most common causes of high-risk hereditary breast and ovarian cancers (HBOCs) are BRCA1 and BRCA2 mutations, affecting all ethnic groups and races. In the general population (excluding Ashkenazi Jews), approximately one in every 400-500 people carries a harmful mutation in BRCA1 or BRCA2 (Yoshida, 2021). Other Inheritable Mutations Increasing Ovarian Cancer Risk Aside from BRCA1 and BRCA2, other inheritable mutations such as PALB2, RAD51C, and BRIP1 can raise the risk of ovarian cancer. Removing the fallopian tubes and ovaries can minimize this risk, but the advantages for non-BRCA mutations remain unknown. The fallopian tubes of women with these inherited abnormalities provide significant information about how ovarian cancer begins and progresses (Samuel, Diaz-Barbe, Pinto, Schlumbrecht, & George, 2022). Hereditary ovarian cancer syndromes (HOC) involve multiple tumour suppressor genes and account for 24% of epithelial ovarian cancer cases ( Imyanitov, et al., 2023). The most frequent mutations are in BRCA1 and BRCA2, which increase the risk of ovarian cancer by 40% and 18%, respectively. Other genes in the Fanconi anaemia pathway, such as PALB2, ATM, RAD51C/D, and BRIP1, also increase risk. Mutations in mismatch repair genes (MLH1, MSH2, and MSH6) are linked to an increased lifetime risk of ovarian cancer ( Imyanitov, et al., 2023). 1.1 Literature Review Genetic studies of families with a history of breast cancer revealed the presence of two genes, BReast CAncer Gene 1 (BRCA1) and BReast CAncer Gene 2 (BRCA2), which are connected to hereditary breast and ovarian cancer. Through the use of tandem repeat markers and DNA polymorphism markers, linkage analysis was able to identify BRCA1 on chromosome 17q21 and BRCA2 on chromosome 13q12 (Hatano et al., 2020). BRCA1 contains 22 exons, encoding a nuclear protein of 1,863 amino acids. It is expressed in tissues such as the ovaries and breast. BRCA2 has 27 exons. Although BRCA1 and BRCA2 have similar exon structures, they lack sequence homology (Lee et al., 2020). BRCA2 primarily interacts with homologous recombination (HR)-related proteins like RAD51, BRCA1 uses its multiple functional domains to connect with a variety of proteins to control DNA damage detection, cell cycle regulation, and chromatin remodelling. These genes' mutations result in HR deficits, which promote the development of cancer by causing error-prone DNA repair and genomic instability (Hatano et al., 2020). 9 Demographic Factors in Genetic Testing Demographics are extremely important in genetic testing for breast and ovarian cancer. Different demographic factors, such as age, socioeconomic level, education, and ethnicity, can have a substantial impact on the choice to undertake genetic testing and risk management based on test results. Moreover, age is an important factor, since younger people may undertake testing to make educated decisions regarding their reproductive possibilities or preventative operations. The incidence of testing positive of BOC mainly depends on these factors. BOC occurs mainly in middle age or older women around the age of 60, only a very small percentage that are diagnosed with BOC are younger than 45, this can be attributed to the combined effects of somatic and germline mutations. Breast and ovarian cancer risk increases with age, therefore younger women who test positive for BRCA mutations may choose more intensive monitoring or preventative procedures, such as prophylactic mastectomies or oophorectomies (Phillips, 2024). Socioeconomic status and education also impact genetic testing uptake. Individuals with higher education levels and socioeconomic status are more likely to undergo genetic testing due to better access to healthcare resources and more comprehensive health literacy, which aids in understanding the benefits and implications of genetic testing (Steffen et al., 2017). Additionally, cost is a significant barrier; those without financial limitations are more likely to participate in genetic testing due to the high costs associated with the tests and subsequent risk management (Steffen et al., 2017). Ethnicity also affects genetic testing owing to variations in genetic predisposition and cultural attitudes toward healthcare. For example, particular mutations seen in specific ethnic groups might influence the prevalence of testing. For instance, Ashkenazi Jewish women are more likely to carry BRCA mutations, increasing their risk considerably. African American women have a greater probability to be diagnosed with severe breast cancer subtypes at a younger age, which are frequently associated with higher mortality rates. (Williams et al., 2016). In the USA, non-Hispanic Whites have the highest incidence rates for both cancers compared to other ethnic groups. However, non-Hispanic Black women experience significantly higher breast cancer mortality rates than non-Hispanic White, Hispanic, or Asian women (Chapman-Davis et al., 2020). A study by Alhuqail et al. (2018) identified the BRCA germline mutation frequency and spectrum in Saudi breast and ovarian cancer patients. Out of the 171 patients recruited for the study, 108 of them were breast cancer patients and the remaining 65 were ovarian cancer patients. Pathogenic BRCA mutations are more prevalent in ovarian cancer patients than breast cancer patients, with 29% of ovarian cancer patients having a BRCA mutation. Both groups show a higher frequency of BRCA1 mutations compared to BRCA2. Most BRCA1 10 mutations (79%) are concentrated in five exonic regions: 10, 11, 17, 19 and 23. Moreover, 54% of BRCA mutation carriers have one of four specific mutations: c.1140dupG, c.4136_4137delCT, c.5095C>T, and c.5530delC. The study found a higher prevalence of BRCA mutations in Saudi Arabian patients, likely due to consanguinity. While 13 of the 17 BRCA mutations were rare, the four key mutations were recurrent, with c.5095C>T and c.1140dupG particularly common in ovarian cancer patients. The c.5530delC mutation is unique to Saudi Arabian patients, whereas the other mutations have been observed globally (Alhuqail et al., 2018). Prevalence and Impact of BRCA Mutations Over 1600 mutations in BRCA1, particularly in regions encoding the RING and BRCT domains and exons 11-13, have been linked to breast cancer, with common mutations like 185delAG in Ashkenazi Jews and 5382insC in Northern Europeans (Scandinavia or Northern Russia). Over 1800 mutations have been identified in BRCA2, mainly frameshift, deletion, and nonsense mutations, with the 6174delT mutation in exon 11 prevalent among Ashkenazi Jews (Gorodetska et al., 2019). In a study to identify mutations in BRCA1/BRCA2 in patients with high risk of familial breast and ovarian cancer in Jordan, 12 pathogenic variants (PVs) were identified in the genes (Abu-Helalah et al., 2020). Five of the variants: c.5186C > A and c.4065_4068delTCAA in BRCA1, and c.5042_5043delTG and c.5351dupA in BRCA2 were previously identified in populations comprising Caucasians, Westerners, and Latin Americans. Three variations, c.1224delA and c.5224C > T in BRCA1 and c.6634_6637delTGTT in BRCA2, were noted previously in Asian populations and were first reported in China and Japan. Several recurrent variations were discovered in Arab populations: in Palestinian patients, c.121C > T in BRCA1 and c.2254_2257delGACT in BRCA2, and in Moroccan and Saudi populations, c.5158C > T in BRCA1. The BRCA2 variations c.6224_6225delAA and c.8696A > G are unique since they have never been reported in databases like as HGMD (Human Gene Mutation Database) Professional, ClinVar, or BIC (Breast Cancer Information Core) (Abu-Helalah et al., 2020). Although BRCA1 and BRCA2 share a close functional relationship, they have different effects on the onset and spread of cancer (Loboda et al., 2023). Triple-negative breast cancer (TNBC), which lacks HER2, progesterone receptors, and estrogen receptors, is mainly linked to BRCA1 mutations. On the other hand, most luminal-like breast tumors that are positive for the estrogen receptor are associated with BRCA2 mutations. Furthermore, compared to BRCA1 mutations, BRCA2 mutations are more commonly linked to various epithelial malignancies, including prostate, pancreatic, and male breast cancers. These genes also differ in the risk of ovarian cancer; BRCA1 mutations have an earlier onset of 40–45% of ovarian cancer, while BRCA2 mutations have a lower risk of 10–20% (Loboda et al., 2023). Some of the mutations identified in previous studies has been shown in Table 1. 11 Table 1 Pathogenic/Likely Pathogenic variants Iidentified in BRCA1/2 Mmutations from previous Studies Pathogenic/Likely Pathogenic/Variants of Mutated Gene Variant Uncertain Significance (VUS) c.5186C > A Pathogenic BRCA1 c.4065_4068delTCAA Pathogenic c.5042_5043delTG Pathogenic c.5351dupA Pathogenic BRCA2 c.2254_2257delGACT Pathogenic c.6634_6637delTGTT Pathogenic c.1224delA Not specified c.5224C > T Not specified BRCA1 c.5158C > T Not specified c.121C > T Not specified c.6224_6225delAA VUS BRCA2 c.8696A > G VUS Genetic Variants Beyond BRCA Genetic testing for breast and ovarian cancer risk normally concentrates on the BRCA1 and BRCA2 genes, although numerous additional genes have been shown to have a substantial effect on cancer risk. Variants in genes including PMS2, ATM, POLE, PALB2, CHEK2, and MUTYH may predispose people to a variety of malignancies, including breast, ovarian, and others. Two patients with ovarian cancer who were diagnosed before the age of 40 were found to have pathogenic or potentially pathogenic mutations in the TP53 and ATM genes. In addition, a patient with a frameshift mutation in TP53 was found to have intriguing and uncommon mutations in ATM and PMS2. Patients with breast cancer were found to have additional harmful mutations, two of whom also carried uncommon variants in other genes. Different allelic frequencies were found in the cohort's Panels A and B for a number of SNPs in different genes, including ATM, BARD1, BRIP1, CDH1, NBN, PALB2, PTEN, RAD51C, RAD51D, STK11, TP53, and CHEK2. A multitude of novel genes linked to cancer are being discovered as a result of the application of whole exome sequencing and multi-gene panel testing in clinical settings (Nunziato et al., 2023). In a study by Melchor and BenÃtez (2013), 25% of the patients had mutations in the BRCA1/2 genes, 5% of the patients had four high-susceptibility genes (CDH1, PTEN, STK11, and TP53), 5% had medium- penetrance genes, and 14% had low-penetrance genes. In 51 % of the patients the causative gene was unidentified (Melchor & BenÃtez, 2013). Moreover, PVs in BRCA1 and BRCA2 were similar in a 12 Caucasian-centred cohort, whereas, in an Asian-centred cohort the PVs in BRCA2 where high in comparison to CHEK2/ATM which were low (Yoshida, 2020). PMS2 is a component of the mismatch repair (MMR) mechanism, which corrects DNA replication mistakes. Pathogenic mutations in PMS2 are linked to Lynch syndrome, which raises the risk of colorectal, ovarian, and endometrial malignancies. PMS2 mutations are uncommon, but when they do occur, they dramatically increase the risk of cancer. (Roberts et al., 2018). The ATM (Ataxia Telangiectasia Mutated) gene is critical for DNA repair and cell cycle regulation. ATM variants are associated with a higher risk of breast cancer, and to a lesser degree, ovarian and pancreatic cancer. The most severe ATM mutations produce shortened or non-functional proteins, increasing cancer risk owing to faulty DNA damage response mechanisms (Stucci et al., 2021). Mutations in the ATM gene can cause ataxia-telangiectasia, an uncommon neurological condition in children characterized by a high risk of acquiring malignancies such as leukemia and lymphoma (Rothblum-Oviatt et al., 2016). Mutations in the POLE, POLD1, and NTHL1 genes, which control DNA replication and repair, POLE have been linked to colorectal cancer and a kind of endometrial cancer known as polymerase proofreading-associated polyposis (PPAP). POLE mutations, while not usually related to breast cancer, do contribute to genomic instability. (Magrin et al., 2021). PALB2, a partner and localizer of BRCA2, is essential for DNA repair via the homologous recombination pathway. Mutations in this gene can increase the risk of breast cancer by almost as much as BRCA2 mutations do. PALB2 mutations also raise the risk of pancreatic and ovarian cancer. In addition, increasing data suggests a possible association between PALB2 mutations and a slight elevated risk of male breast cancer and children’s malignancies, demonstrating the gene's wide influence on cellular repair pathways. (Toss et al., 2023; Antoniou et al., 2014). Lastly, The CHEK2 gene encodes a protein kinase involved in DNA repair and cell cycle regulation. CHEK2 gene mutations, such as the c.1100delC and I157T variants, have been linked to a modestly elevated risk of breast cancer, as well as hazards for colorectal and prostate cancers. (Weischer et al., 2008). Some of the mutations are shown in Table 2. 13 Table 2 Common Genes and their Associated Cancers Gene Associated Cancers Notes BRCA1/2 Breast, ovarian Core genes for hereditary breast and ovarian cancer PMS2 Colorectal, ovarian, Part of the mismatch repair (MMR) mechanism; endometrial linked to Lynch syndrome ATM Breast, ovarian, pancreatic, Critical for DNA repair and cell cycle regulation; leukaemia, lymphoma associated with ataxia-telangiectasia POLE Colorectal, endometrial Linked to polymerase proofreading-associated polyposis (PPAP) PALB2 Breast, pancreatic, ovarian Works with BRCA2 for DNA repair; increases risks significantly CHEK2 Breast, colorectal, prostate Involved in DNA repair and cell cycle regulation; variants like c.1100delC and I157T identified TP53 Breast, ovarian, and others Frequently mutated in severe cancer cases, associated with Li-Fraumeni syndrome MUTYH Various, often colorectal Associated with MUTYH-associated polyposis CDH1 High susceptibility to gastric Linked to hereditary diffuse gastric cancer cancer PTEN Breast, thyroid, endometrial Part of PTEN hamartoma tumor syndrome STK11 Peutz-Jeghers syndrome, Associated with various gastrointestinal cancers increased cancer risk RAD51C Ovarian, breast Linked to moderate cancer risk RAD51D Ovarian Similar function and cancer associations as RAD51C BARD1 Breast, ovarian Functions in conjunction with BRCA1, linked to increased cancer risk BRIP1 Ovarian Associated with moderate risk of ovarian cancer CDH1 Breast, gastric Linked to hereditary diffuse gastric cancer NBN Breast, ovarian Part of the MRN complex important for DNA double- strand break repair PTEN Breast, prostate, and others Part of Cowden syndrome, a disorder characterized by multiple noncancerous, tumor-like growths STK11 General increased cancer risk Linked to various cancers due to cellular processes Role of Family History and age in Cancer Risk Family history is important in determining cancer risk, not only because of inherited genetic mutations, but also because of common environmental and lifestyle variables. Understanding the importance of family history is critical for early identification, prevention, and tailored treatment strategies. However, the association between family history and breast cancer presentation and outcomes is conflicting. Some studies show no association with tumor size, nodal status, hormone receptor status, or grade, while others report smaller, higher tumor stages, lymph node-negative, estrogen receptor-positive, ER- negative/progesterone receptor-negative, and higher-grade tumors. Most studies show no significant 14 association with better prognosis (Figueiredo et al., 2006). The effect of family history on cancer risk is mostly due to germline mutations, which are inherited and present in all cells of the body. These mutations may predispose individuals to hereditary cancer syndromes. Mutations in BRCA1 and BRCA2 are widely recognized to increase the risk of breast and ovarian cancer. Other genes, including those linked to Lynch syndrome (MLH1, MSH2, PMS2), raise the risk of colorectal and endometrial cancer. In contrast, somatic mutations arise in individual cells after conception and are not inherited. These mutations can induce carcinogenesis but are not heritable and are not passed into the next generations. While family history is less directly connected to somatic mutations, the existence of certain germline mutations might increase the chance of somatic mutations, affecting cancer risk and development. Families frequently share circumstances and habits that increase cancer risk. Diet, exposure to environmental contaminants, and physical activity levels can all contribute to the development of identical cancer forms in a family, regardless of genetics. These common characteristics may aggravate the hazards associated with germline mutations. (Johansson et al., 2021). Age ≤ 35 is recognized as a potential prognostic indicator, with more aggressive characteristics and increased use of adjuvant chemotherapy. A population-based study revealed that breast cancer diagnosed in women under 35 has more aggressive characteristics, increased adjuvant chemotherapy use, and reduced hormone therapy compared to older women. In addition, tumors with certain clinical features, such as high grade, ER-negativity, lymphovascular invasion (LVI), and high proliferation fractions were observed in such women (Figueiredo et al., 2006). 2.0 Prevalence of Hereditary Cancer Genes in the UAE Population Breast cancer accounts for almost one-third of all female cancer diagnoses in the UAE and continues to be the leading cause of cancer-related deaths among women. The illness often manifests at a younger age in the UAE population, with the median age at diagnosis being about 48 years. Notably, the majority of breast cancer incidences (21.5%) occur between the ages of 30 and 40 (Al-Shamsi et al., 2023). According to the annual report from the UAE National Cancer Registry, there were a total of 883 breast cancer cases reported among the UAE population. This included 209 cases in female UAE citizens and 1 case in a male UAE citizen. Among non-UAE citizens, there were 666 cases in females and 7 cases in males. Additionally, the report highlighted 100 ovarian cancer cases within the UAE population. Of these, 24 cases were among female UAE citizens, while 76 cases were found in non- UAE citizens (Shelpai, 2019). The UAE's medical infrastructure for breast cancer is sophisticated, according to the most recent international norms and treatment techniques, including access to cutting- edge medications. Given the prevalence of breast cancer in the UAE, there is an urgent need for 15 targeted research to address the disease's early beginning in the population, genetic predispositions, and considerable impediments to efficient screening. The information is especially important for developing health policy and refining screening programs to better suit the UAE's demographic and cultural setting. In 2017, the UAE National Cancer Registry recorded 834 new cases of breast cancer, accounting for 20.23% of all cancer occurrences in the nation. The incidence rates highlight the critical need for focused public health policies and increased awareness efforts to improve early diagnosis and treatment results (Al-Shamsi et al., 2023). The estimated cancer incidence and mortality rates collected by the UAE National Cancer Registry in 2019 provide specific information about cancers and cancer fatalities in the UAE and highlights key data on breast and ovarian cancer and other forms. For 2019 data, breast cancer emerged as the most common type of neoplastic disease, accounting for 20.2% of all cancer cases and contributing to the highest cancer-related deaths, which stands at 11.6% of the annual total of cancer fatalities. Another cancer that was also common and causing high fatalities among women in the UAE was ovarian cancer. Female population had a higher total cancer incidence rate at 56.2% as compared to 43.8% of the men, and its age-standardized incidence rate was 78.4 per 100,000 population. The five most common cancer types reported were breast, thyroid, colorectal, skin and leukemia. This data reveals the extent to which these cancers are fatal in women in the UAE and highlights the need for cancer prevention and intervention strategies in the UAE. 3.0 Technological Advancements in Genetic Screening The first-generation DNA sequencing methods, Sanger dideoxy synthesis and Maxam-Gilbert chemical cleavage, established the groundwork for modern sequencing (Slatko et al., 2018). The Maxam-Gilbert method, though less commonly used now due to its complexity and toxicity, involves chemical modification and cleavage of DNA. Sanger sequencing, developed in 1977, became the standard by using chain-terminating nucleotides to determine DNA sequences. Key advancements, such as fluorescent dyes, thermal-cycle sequencing, and capillary electrophoresis (CE), improved its efficiency and accuracy. While slower than next-generation sequencing, Sanger sequencing is still valuable for low-throughput projects and various specialized applications, including enzyme activity analysis and glycobiology (Slatko et al., 2018). However, first-generation sequencing methods were limited by low throughput, particularly when sequencing diploid DNA, which required labour- intensive processes (Hu et al., 2021). This complexity contributed to the first human genome project taking over a decade and costing $2.7 billion. Despite advancements reducing the cost to $10 million per genome, the technology had reached its limits in terms of time and cost, necessitating the 16 development of new sequencing technologies. Next-generation sequencing (NGS) technologies emerged between 2004 and 2006 and revolutionized biomedical research by producing vastly larger amounts of data. This was made possible by advancements in nanotechnology that allowed for the massively parallel sequencing of individual DNA molecules. By displacing the labour-intensive Sanger methods with high throughput and single-molecule sequencing, NGS enhances data capture and processing. Massively parallel sequencing of short reads is achieved by second-generation systems such as Illumina and Ion Torrent, which use DNA fragmentation, end-repair, adapter ligation, surface attachment, and in-situ amplification. Reassembling these small reads for lengthy DNA sequences, however, might be difficult, especially in regions with low complexity and structural changes (Hu et al., 2021). NGS technologies have revolutionized genetic screening, allowing for comprehensive investigation of pathogenic variants, and improving early detection and intervention options for high- risk individuals. The process of NGS sequencing initially starts with DNA fragmentation where the target DNA is fragmented into short segments, typically 100-300 base pairs in length (Qin, 2019). This can be accomplished using methods like mechanical disruption (e.g., sonication), enzymatic digestion, or other techniques. Specific DNA segments are then extracted either through hybridization capture using complementary probes or by amplification via polymerase chain reaction (PCR) in an amplicon assay. These fragments are prepared for the subsequent stage, which is library preparation. Second step is library preparation where the DNA segments undergo modification to include sample-specific identifiers for identification purposes, along with sequencing adaptors. This adjustment facilitates the attachment of sequencing primers to the DNA segments, thereby preparing them for extensive parallel sequencing. Third step in the process is Sequencing where the prepared DNA library is loaded onto a sequencing platform, such as a flow cell in an Illumina sequencer or a sequencing chip in an Ion Torrent sequencer. This enables the simultaneous sequencing of all DNA segments. The resulting data is then processed using bioinformatics software. The final stage involves bioinformatics analysis and data interpretation which encompassing tasks such as base calling, read alignment, variant detection, and annotation. The sequenced data is compared against a reference human genome to identify any genetic variants or mutations. Subsequently, the sequenced segments are assembled to reconstruct the complete sequence of the target DNA. The interpreted results are provided to the user, highlighting each identified variant and its potential implications in biology or clinical applications (Qin, 2019). Figure 1 17 Figure 1: Next Generation Sequencing Workflow (Aryal, 2023) 4.0 Clinical Implications and Management Individuals with a personal or family history of breast cancer may benefit from hereditary risk evaluations to determine their risk and that of their family members. Expertise in managing patients undergoing genetic testing is essential to ensure appropriate tests are ordered, results are accurately interpreted, and effective management and risk reduction strategies are recommended to at-risk patients or family members. This retrospective cohort study of breast and ovarian cancer testing results is aimed at clinical oncology specialties and Department of Health (DOH) screening. It establishes criteria for identifying cancer patients who would benefit from genetic counselling and germline genetic testing for hereditary breast and ovarian cancer syndrome. Furthermore, it outlines strategies for managing pathogenic mutations linked to breast, ovarian, and other cancers, including recommendations for risk reduction measures such as adjusting screening ages and providing relevant information for effective patient care. The detection of these genetic abnormalities has important consequences for cancer risk assessment and management. For example, patients diagnosed with cancer who harbor pathogenic or likely pathogenic (P/LP) variants in BRCA1 or BRCA2 may benefit from tailored cancer treatment options such as PARP inhibitors, which are especially effective in tumors with deficiencies in DNA repair pathways (Yoshida, 2021). Additionally, individuals with these mutations can undergo more regular 18 and stringent screening processes to discover cancer at an earlier, more treatable stage. Recognizing one's genetic susceptibility might also guide preventive procedures like prophylactic operations (e.g., mastectomy or salpingo-oophorectomy) to lower the risk of developing cancer (Yoshida, 2021). Furthermore, this genetic information is critical not only for the affected patients but also for their relatives, who may have the same inherited mutations. Family members can undergo genetic testing to evaluate their cancer risk and take appropriate preventive measures if necessary. Hereditary counseling becomes an important part of this process, informing individuals and families about their hereditary risks and the available strategies for monitoring and mitigating them (Yoshida, 2021). 5.0 Hypothesis We hypothesize that the prevalence of pathogenic and likely pathogenic variants will be significant in cohort from UAE with certain genes showing higher mutation rates. Additionally, we anticipate identifying recurrent Variants of uncertain significance (VUS) that could warrant further investigation, thereby contributing to the understanding of genetic predispositions to breast and ovarian cancer in the UAE. 6.0 Study Objectives The study aims to identify genetics variants that could be linked to risk of breast and ovarian cancers and data could be utilized for better patient management. The main objectives of the study are to: Estimate the incidence of pathogenic and potentially pathogenic mutations in inherited cancer genes in the UAE and to identify the most frequently altered cancer genes Identify recurring Variants of unknown significance (VUS) that could be suitable for future functional investigation. 7.0 Methodology 7.1 Study Design and Population This study uses a retrospective cohort approach to investigate the impact of family history, genetic variants, and demographic factors on breast and ovarian cancer test results. The data is derived from a vast medical database that contains extensive genetic, demographic, and clinical information on people who have undergone cancer-related genetic testing. The data collection period spans January 2010 to December 2021. ensuring a representative sample. All people with a confirmed diagnosis of breast or ovarian cancer who have undergone relevant genetic testing are included in the study population. 19 The inclusion criteria are strictly fulfilled, and only persons with complete demographic and genetic information are included, ensuring that the data is robust and credible. Patients with inadequate data or those diagnosed with other cancers are eliminated, ensuring that the focus remains on breast and ovarian cancer therapy. This rigorous selection procedure ensures that the research sample is homogenous in terms of circumstances, hence improving the study's internal validity. IRB for the study was obtained by the principal Investigator from Dubai Health Authority. All data was collected by representatives of the research team from Dubai. The students were not involved in data extraction and collection. Deidentified data was shared with the students for analysis purposes. Sample size was 171 patients who were tested for Common Hereditary Cancers. From the initial cohort, 48 patients were excluded because they were diagnosed with cancer types other than breast or ovarian cancer leaving 123 out of the original 171 participants available for data analysis. Flowchart shown in Figure 2 Figure 2: CONSORT diagram showing the flow of participants, exclusion, and data categories. 20 7.2 Data Collection and Management Data collection was extensive, with many levels of verification to ensure accuracy and completeness. The collected information is classified into three categories: demographic data, genetic data, and family history data. Demographic data includes each patient's age, gender, and ethnicity. Age is treated as a continuous variable and evaluated using summary statistics such as median and interquartile range (IQR) Gender is expressed as a categorical variable with two levels: male and female Ethnicity is split into Arab and non-Arab groups, where the Arab category consist of the 22 Arab countries mentioned by Al-Shamsi et al., 2023 which are Algeria, Bahrain, Comoros, Djibouti, Egypt, Iraq, Jordan, Kuwait, Lebanon, Libya, Mauritania, Morocco, Oman, Palestinian Territories, Qatar, Saudi Arabia, Somalia, Sudan, Syria, Tunisia, UAE, and Yemen. This makes it easier to examine ethnic differences in genetic testing and results Gender and ethnicity data are presented as proportions and examined using chi-square tests to see whether there are any significant differences in distribution among the research population. This comprehensive procedure ensures that all demographic parameters are recorded and accurately represented, establishing the framework for future analyses. sGenetic data consists of genetic testing results, which are classified into three categories: Genetic data consists of genetic testing results, which are classified into three categories: Harmful (genetic variants that are known to be associated with an increased risk of breast or ovarian cancer) Variants of uncertain significance (VUS) Negative The collection included unique genetic variants uncovered via testing, providing detailed information on each patient's genetic profile. Data verification by quality control (performed by the designated research team) ensured that genetic data is correct and valid, allowing for robust data analysis. Patient questionnaires and medical records are used to collect family history information, which determines if a patient has a positive, negative, or unspecified family history of cancer. This information is crucial in identifying genetic risk factors for breast and ovarian cancer. The extensive documentation of family history enables a more advanced analysis of hereditary patterns and their 21 impact on genetic testing results. The study reaches a high level of data quality and reliability by merging data from several sources and evaluating its accuracy through stringent methods. 7.3 Statistical Analysis The statistical study is intended to thoroughly investigate the links between demographic parameters, family history, genetic variations, and cancer test findings. Various statistical approaches are used to examine the data, resulting in accurate conclusions. The demographic analysis entails multiple processes to evaluate the demographic features of the research population and their relationship with cancer testing findings, including: 1. Age Analysis: Summary statistics, such as the median and interquartile range, are computed for patient age. A histogram is constructed to visualise the age distribution and find any significant patterns or clusters in the data. This aids in understanding age-related patterns in cancer incidence and genetic testing results. 2. Gender Proportion: Chi-square tests are used to examine gender distributions and identify significant differences between the two groups. This study aids in discovering any gender differences in cancer testing results. 3. Ethnicity Distribution: The distribution of patients by ethnicity (Arab versus non-Arab) is examined. Chi-square tests are used to discover substantial racial discrepancies in the dataset. This aids in understanding the impact of ethnicity on genetic testing findings. 4. High-Risk Age Groups: Age groups with higher cancer rates are identified, allowing for more targeted screening and intervention activities. This aids in identifying certain age groups that require more specialized medical care and resources. Family History The influence of family history on genetic testing findings is crucial for understanding hereditary cancer risk. The study begins with the creation of a contingency table that compares family history (categorized as positive, negative, or unspecified) to genetic test findings (categorized as positive, negative, or variations of VUS. This comparison provides the framework for assessing the relationship between family history and genetic test results. Following that, a chi-square test for independence is used to establish whether there is a statistically significant link between family history and genetic test outcomes. This stage is critical because it gives information on the role of hereditary variables in the likelihood of certain genetic discoveries. Additional studies utilizing chi-square or Fisher's exact tests 22 (depending on sample size) are performed to better define the precise types of mutations found in those with a family history of cancer. These tests aid in the identification of certain genetic variants that are more common in those with a familial susceptibility to cancer, hence improving our understanding of hereditary cancer syndromes. 7.5 Genetic Variants Analysis The Genetic variations Analysis seeks to methodically identify the most prevalent genetic variations in the research population and explain their clinical significance. This requires numerous thorough steps. Initially, all genetic variations found in the sample are methodically extracted and listed. Each variant is tallied to offer a thorough snapshot of the genetic landscape, allowing researchers to better understand the frequency of specific genetic variants among individuals. Following that, the frequency of each genetic variant is estimated as a percentage of the total number of patients, providing a clear image of the research population's most common genetic variants, which is critical for finding relevant genetic markers. Furthermore, each genetic variant is assigned to one of three categories: harmful, variants of unknown significance (VUS), or negative. The proportions of these categories are computed to demonstrate the distribution of genetic results among patients. This categorization is critical for determining the clinical significance of various genetic variations in relation to cancer risk. The findings are thoroughly evaluated, providing insights into the genetic basis of cancer risk. 7.6 Correlation Analysis Correlation analysis investigates the correlations between variables to gain a better understanding of how different elements interact. The major studies performed include looking at the link between age and positive genetic test findings using Pearson correlation or Spearman rank correlation, depending on the data distribution. This research aids in determining if age has a substantial impact on genetic test results, which may then be used to define age-specific screening techniques. Furthermore, a contingency table is created to compare specific genetic variants to clinical indications, followed by a chi-square test for independence to determine the relationship between specific genetic variants and clinical outcomes. This technique aids in the identification of genetic variations that are highly related with certain clinical indications, which is critical in personalized medicine. Another contingency table compares ethnicity to mutation type, with chi-squared or Fisher's exact tests used to determine the significance of the connection between the two. This approach is critical for better understanding racial differences in genetic mutations and developing more fair healthcare interventions. 23 7.7 Analysis of Variance (ANOVA) The Analysis of Variance (ANOVA) is used to investigate the influence of an independent factor on various dependent clinical outcomes at the same time. In this study, dependent factors include the existence of various cancer kinds and severity levels, whereas independent variables include age, gender, family history, clinical indication, and genetic variations. During the data preparation step, the dependent and independent variables are checked for normality and variance homogeneity, and transformations are made as needed to fulfil the ANOVA assumptions. The ANOVA is then run using statistical software to look for variations in clinical outcomes depending on the independent variables. Several criteria are used to determine the significance of the multivariate test, including Wilks' Lambda, Pillai's Trace, Hotelling's Trace, and Roy's Largest Root. If the ANOVA findings are significant, post-hoc analyses are used to determine which specific dependent variables are influenced by the independent factors. These findings are used to evaluate the combined impact of demographic and genetic variables on clinical outcomes, resulting in a more complete knowledge of how these elements interact to influence health. The combined influence of multiple factors on breast and ovarian cancer risk is then analyzed using logistic regression, with cancer as the dependent variable and age, gender, family history, ethnicity, clinical indication, and specific genetic mutations as independent variables. 7.8 Database Matching Genetic variations discovered in the study are compared to recognized genetic databases such as ClinVar, COSMIC, and the BRCA Exchange to determine their clinical relevance. This procedure entails collecting genetic variations from the dataset and structuring them to ensure interoperability with reference databases. The polymorphisms are then compared to various databases to gather specific information on their clinical importance, such as pathogenicity, related diseases, and population prevalence. The results of the database searches are painstakingly analyzed and integrated into the research findings, with a focus on identifying variations with recognized clinical importance. This stage is critical because it gives insights into the genetic basis of cancer risk, therefore validating the research findings and verifying that the detected variations are clinically relevant. 7.9 Gene Variants To offer a full perspective of the genetic landscape, detailed tables with particular gene variant frequencies are prepared. Each mutation is rigorously analyzed for its relationship to clinical outcomes, revealing significant genetic indicators that affect cancer risk. To offer a complete picture of the research population's genetic profile, genetic variations detected in the dataset are extracted and listed. 24 The frequency of each genetic variant is computed, and their distribution is examined to determine common and unusual variants. This investigation contributes to a better knowledge of the prevalence of certain genetic variants and how they may affect cancer risk. The detected genetic variations are then compared to known harmful mutations and variants of unknown importance to identify their clinical relevance. This stage is critical because it gives insights into the genetic basis of cancer risk, therefore validating the research findings and confirming that the detected variations are clinically meaningful. The findings are painstakingly analyzed to give insights into the genetic foundation of cancer risk, which may then be used to support focused screening and intervention techniques, resulting in more personalized and effective treatment. 8.0 Software and Tools The research makes use of a variety of software and technologies to help with data analysis and management. Statistical tools such as R, SPSS and GraphPad Prism are used for a variety of data analysis tasks, including descriptive statistics, correlation analysis, association studies, and logistic regression. ClinVar was utilized for in-depth analysis of gene variants identified. Secure data management systems are used to store and manage patient data while adhering to data protection requirements. This combination of techniques enables accurate, efficient data analysis and strong conclusions, which contribute to the study's dependability and validity. 9.0 Results Among the 123 patients included in the study, 96.7% were female, with a mix of Arab (55.3%) and non-Arab (44.7%) ethnic groups. The cohort comprised people with a family history of breast/ovarian cancer (41.5%) and those with positive genetic findings for breast and ovarian cancer (16.3%). A detailed breakdown of demographic characteristics is illustrated in Table 3. The cohort was subsequently divided into various subsets based on demographic characteristics. 25 Table 3 Demographic Distribution Demographic Characteristic (n=subset) % (N=123) Gender and Ethnicity Male 4 3.3 Female 119 96.7 Arab 68 55.3 Non-Arab 55 44.7 Patients who Tested Positive for Breast Cancer & Ovarian Cancer Pathogenic Genes n subset (%) Total % n=20 (%) 16.3 Male 2 (10) 1.6 Female 18 (90) 14.6 Arab 10 (50) 8.1 Non-Arab 10 (50) 8.1 Patients with + Family History for BC/OC n=51 (%) 41.5% Male 3 (5.9) 2.4 Female 48 (94.1) 39.0 Arab 28 (55) 22.8 Non-Arab 23 (45) 18.7 + Family History for BC/OC and tested positive for BC/OC Pathogenic Genes n=12 (%) 9.7% Male 2 (16.7) 1.6 Female 10 (83.3) 8.1 Arab 5 (41.7) 4.1 Non-Arab 7 (58.3) 5.7 Patients who Tested ‘Uncertain Significance’ for BC/OV Pathogenic Genes n= 51 (%) 41.5% Male 1 (2) 0.8 Female 50 (98) 40.7 Arab 31 (60.8) 25.2 Non-Arab 20 (39.2) 16.3 + Family History and tested ‘Uncertain Significance’ for BC/OV Pathogenic Genes n=22 (%) 17.9% Male 1 (4.5) 0.8 Female 21 (95.5) 17.1 Arab 14 (64) 11.4 Non-Arab 8 (36) 6.5 Unspecified family history and tested positive for BC/OV Pathogenic Genes n=8 (%) 6.5% Male 0 (0) 0 Female 8 (100) 6.5 Arab 5 (63) 4.1 Non-Arab 3 (37) 2.4 Patients with Ovarian Cancer Clinical Indications n=6 (%) Arab 4 (66.7) 3.3 Non-Arab 2 (33.3) 1.6 Arab + Positive Genetic Result 2 (33.3) 1.6 Non-Arab + Positive Genetic Result 0 (0) 0 Uncertain Genetic Result 2 (33.3) 1.6 26 Group 1: All patients screened for BC/OC Age was the only continuous variable collected in the data; thus, it was tested for normality. The findings of the Shapiro-Wilk test showed a W-statistic of 0.9913 and a p-value of 0.6410, so the null hypothesis cannot be rejected, and the data follow a normal distribution. A one-way ANOVA was performed to investigate the difference of age across genetic results, but no significant age differences across genetic test results was found (p=0.1454) (Figure 3). As the data did not meet necessary requirements to conduct chi-squared to compare age groups and genetic results, Fisher’s Exact test was employed. The findings were not significant (p=0.0900), though it was very close to the cutoff (Figure 5). Fisher’s exact test was also used to compare age genetic results, though the findings were also not significant (p=0.1454) (Figure 4). Figure 3: Age vs Genetic Result Figure 4: Genetic Result vs Ethnicity Figure 5: Distribution of Genetic Test Results by Age Group 27 Spearman’s correlation was then executed to compare age and the pathogenicity of the genetic variants. Results showed a weak negative correlation with R value of -0.1989, but a non-significant p value of 0.096 (Figure 6). Chi-squared was performed to compare Ethnicity and genetic classification, but the findings were not significant (p=0.4600). Figure 6: Age vs pathogenic classification Logistic regression was leveraged to investigate possible risk factors amongst the UAE population, and various models were prepared. Various models were built to compare the presence or absence of a pathogenic breast cancer gene variant with age, gender, ethnicity, and family history as predictors with different combinations. None of the predictors were statistically significant in any of the models. However, the odds ratios indicated non-significant trends in some models. When performing logistic regression for all the models, family history showed an increase in disease odds (OR=2.296, CI: 0.8570 to 6.416). In the model that only investigated family history and ethnicity as predictors, Family history showed a non-significant increase in disease odds (OR=2.462, CI: 0.9351 to 6.802), while Arab ethnicity showed a non-significant decrease in disease odds (OR=0.7749, CI: 0.2896 to 2.071). Models were also created to understand whether the same predictors can be used to predict the presence of a BC/OC related genetic variant, whether it was pathogenic or a VUS, but again, none of the models were statistically significant. In the model comparing presence of variant with ethnicity and family history, family history showing a non-significant increase in variant presence (OR=1.899, CI: 0.9096 to 4.058) and being Arab was not a significant predictor (OR=0.7759, CI: 0.2940 to 2.046). 28 Group 2: Patients of Arab Ethnicity The findings of the Shapiro-Wilk test for age showed a W-statistic of 0.9913 and a p-value of 0.9221, so the null hypothesis cannot be rejected, and the data follow a normal distribution. A one- way ANOVA was performed to investigate the difference of age across genetic results, but no significant age differences across genetic test results was found (p=0.6230). Fisher’s Exact test was used to compare age groups and genetic results, but the findings were not significant (p=0.4302) (Figure 7). Fisher’s exact test was also used to compare age and genetic results, though the findings were also not significant (p=0.0545), though the p-value was close to the cutoff of 0.05. Spearman’s correlation was then executed to compare age and the pathogenicity of the genetic variants. Results showed a very weak negative correlation with sr value of -0.1224, and a p value of 0.446 (Figure 8). Figure 7: Distribution Genetic Results Across Age Groups in Arab Population 29 Figure 8: Spearman’s Correlation for Age vs Pathogenic Classification of Genetic Result in Arab Populations Logistic regression was leveraged to investigate possible risk factors amongst the Arab patients within the UAE population, Various models were built to compare the presence or absence of a pathogenic breast cancer gene variant with age, gender, and family history as predictors with different combinations. None of the predictors were statistically significant in any of the models. However, the odds ratios indicated non-significant trends in some models. When performing logistic regression for all the models, age showed a slight non-significant decrease in disease odds (OR=0.9697, CI: 0.8930 to 1.048), while Family history showed a non-significant increase in disease odds (OR=1.446, CI: 0.3577 to 5.812). The same was done to understand whether the same predictors can be used to predict the presence of a BC/OC related genetic variant, whether it was pathogenic or a VUS. The models were not good predictors, but in the model using age and family history as predictors, age showed a slight non-significant increase in variant presence (OR=1.009, CI: 0.9523 to 1.071), while family history showed a non-significant increase in variant presence (OR=1.752, CI: 0.6451 to 4.973). In the Arab subset, at least nine pathogenic variants were identified across different genes. Table 4 details the cDNA changes, the amino acid changes, the number of carriers and the type of variation that was identified in the tested genes. The most frequently identified gene with the pathogenic variants was BRCA1 as three carriers were identified with the same cDNA change (c.4065_4068del), while 1 carrier had a c.4289 deletion. More pathogenic variants were found in the genes ATM, BRIP1, 30 RAD51D and SDHA, and each different variant was detected in one carrier. Table 5 categorizes the different types of variants that were identified. frameshift being the most common type, representing 50% of all variants, followed by nonsense mutations, which represented 40% of all variants. Table 4: Pathogenic Variants Identified in Arab Population cDNA change Amino Acid Change Carrier number Type of variation ATM c.895G>T p.Glu299* 1 Nonsense c.90dup p.Lys31* 1 Nonsense BRCA1 c.4065_4068del p.Asn1355Lysfs*10 3 Frameshift c.4289del p.Pro1430Leufs*4 1 Frameshift BRCA2 c.7643_7644del p.His2548Leufs*5 1 Frameshift BRIP1 c.2947dup p.Ile983Asnfs*19 1 Frameshift Table 3 Pathoge nic Table 2: Variants RAD51D Identifie d in Arab Popula ti ons c.803G>A p.Trp268* 1 Nonsense SDHA c.223C>T p.Arg75* 1 Nonsense 31 Table 5: Type of Pathogenic Variants in Arab Population Type of Variation Frequency % Nonsense 4 40 Frame shift 6 50 Missense 0 0 UTR 0 0 Splicing 0 0 Total 9 100 A total of 21 variations of unknown significance (VUS) were found in total across multiple genes in the Arab subset with a verified family history of breast cancer, all of which were missense mutations. Table 6 lists these variants along with relevant information such as particular cDNA and amino acid changes, while Table 7 provides an overview of the distribution of the types of mutations. 32 Table 6: Variants of Uncertain Significance Identified in Arab Population with Confirmed Family History cDNA change Amino Acid Change Carrier number Type of variation BC Status of Patient APC c.2356C>G p.Arg786Gly 1 Missense US ATM c.1351C>T p.Arg451Cys 1 Missense US c.3175G>T p.Ala1059Ser 1 Missense US c.5185G>C p.Val1729Leu 1 Missense US c.8921C>T p.Pro2974Leu 1 Missense US BLM c.455A>G p.Asn152Ser 1 Missense Positive c.1490A>G p.Gln497Arg 1 Missense Positive BRCA2 c.3973A>G p.1325Ala 1 Missense US c.8774A>G p.Gln2925Arg 1 Missense US MSH3 c.1655C>T p.Thr552Ile 1 Missense US MSH6 c.1814C>G p.Thr605Ser 1 Missense US MUTYH c.841C>T p.Arg281Cys 1 Missense US c.904G>A p.Val301Met 1 Missense US NF1 c.8080G>T p.Ala2694Ser 1 Missense Positive NTHL1 c.527T>C p.Ile176Thr 1 Missense US PMS2 c.1004A>G p.Asn335Ser 1 Missense US c.2266G>A p.Asp756Asn 1 Missense US POLE c.1346C>T p.Thr449Met 1 Missense US c.3155C>T p.Thr1052Met 1 Missense US c.6040G>A p.Gly2014Arg 1 Missense US TSC2 Ta ble 4: Varia nts of U ncertain Sig nifica nce Identif ied in Arab Populatio n wit h Co nfirme d Family History c.4207G>T p.Asp1403Tyr 1 Missense Positive 33 Table 7: Type of VUS in Arab Population Type of Variation Frequency % Nonsense 0 0 Frameshift 0 0 Missense 21 100 UTR 0 0 Splicing 0 0 Total 21 100 Group 3: Patients of non-Arab Ethnicity The findings of the Shapiro-Wilk test for age showed a W-statistic of 0.9810 and a p-value of 0.5323, so the null hypothesis cannot be rejected, and the data follow a normal distribution. One- way ANOVA was performed to investigate the difference of age across genetic results, but no significant age differences across genetic test results was found (p=0.2093). Fisher’s Exact test was used to compare age groups and genetic results (Figure 9), but the findings were not significant (p=0.5486). Spearman’s correlation was then executed to compare age and the pathogenicity of the genetic variants. Results showed a weak negative correlation with a sr value of -0.3806, close to the cutoff for a moderate correlation, and a statistically significant p value of 0.038 (Figure 10). Figure 8: Figure 9: Distribution Genetic Results Across Age Groups in non- Arab Population 34 Figure 9: Spearman’s Correlation to Compare Age and Pathogenicity in non-Arab Population Figure 10: Spearman’s correlation to compare Age and pathogenicity in non-Arab Logistic regression was leveraged to investigate possible risk factors amongst the non-Arab patients within the UAE population, Various models were built to compare the presence or absence of a pathogenic breast cancer gene variant with age, gender, and family history as predictors with different combinations. None of the models showed good predictive ability. However, a few odd ratio trends were identified, as family history consistently showed an increase in pathogenic gene odds across all models (OR=4.045, CI: 0.9391 to 21.62 in the model with age and family history as a predictor and OR=4.229, CI: 1.024 to 21.79 when only family history was used as a predictor. The same was done to understand whether the same predictors can be used to predict the presence of a BC/OC related genetic variant, whether it was pathogenic or a VUS. The models also did not have strong predictive ability, but the odds ratio again showed a trend in family history, as the predictor showed a non- significant increase in variant presence (OR=2.067, CI: 0.6912 to 6.480) in addition to age, which showed a slight non-significant decrease in variant presence (OR=0.9806, CI: 0.9071 to 1.057). Twelve pathogenic mutations were found in the non-Arab fraction of the cohort spanning multiple genes linked to ovarian and breast cancer. Table 4 lists these variants along with the type of variation, number of carriers, particular cDNA modifications, and amino acid changes. The most commonly impacted gene was BRCA1, where two frameshift mutations were found in each carrier. There was also one splicing mutation and one frameshift mutation in the BRCA2 gene. The pathogenic variant types found in the non-Arab population are categorized in Table 8. With 50% of the variants, frameshift mutations were the most prevalent type. These were followed by splicing mutations (8.3%), 35 missense mutations (16.7%), and nonsense mutations (25%) (Table 9). These results shed light on the harmful variants that are common in non-Arab populations and point to the necessity for more research on the clinical implications of these mutations as well as focused genetic screening. 36 Table 8: Pathogenic Variants Identified in Non-Arab Population cDNA change Amino Acid Change Carrier number Type of variation BRCA1 c.1504_1508del p.Leu502Alafs*2 1 Frameshift c.5266dup p.Gln1756Pro*74 1 Frameshift BRCA2 c.8332-1G>T p.? 1 Splicing c.9113dup p.Pro3039Thrfs*5 1 Frameshift CHEK2 c.499G>A p.Gly167Arg 2 Missense c.1100del p.Thr367Metfs*15 1 Frameshift MUTYH c.452A>G p.Tyr151Cys 1 Missense PALB2 c.1633G>T p.Glu545* 1 Nonsense c.3113G>A p.Trp1038* 1 Nonsense c.355del p.Gln119Lysfs*58 1 Frameshift RAD51C c.181_182del p.Leu61Alafs*11 1 Frameshift Table 9: Type of Pathogenic Variants Identified in Non-Arab Population 37 There were 11 VUS found in total across several genes in the non-Arab subset with a verified family history of breast cancer. Table 10 lists these variants together with the patient's status for breast cancer, the type of variation, the number of carriers, and specific cDNA and amino acid changes. Eighty-two percent of the variations that were discovered were missense mutations. The types of VUS found in the non-Arab population with a verified family history are categorized in Table 11. 82% of the variants were missense mutations, which were followed by splicing mutations (9%), UTR variants (9%), and other mutations. In this subset, no frameshift or nonsense mutations were found. 38 Table 10: Variants of Uncertain Significance Identified in Non-Arab Population with Confirmed Family History cDNA change Amino Acid Change Carrier number Type of variation BC Status of Patient BARD1 c.54C>G p.Asn18Lys 1 Missense VUS MSH3 c.1655C>T p.Thr552Ile 1 Missense VUS MSH6 c.-3G>A p.? 1 UTR VUS MUTYH c.934-2A>G p.? 1 splicing VUS PALB2 c.13C>T p.Pro5Ser 1 Missense VUS c.3035C>T p.Thr1012Ile 1 Missense VUS PMS2 c.1477G>A p.Asp493Asn 1 Missense VUS c.1534G>A p.Gly512Ser 1 Missense VUS POLE c.712A>T p.Ile238Phe 1 Missense VUS RAD51D c.746A>G p.Asn249Ser 1 Missense VUS SMAD4 c.535A>G p.Ile179Val 1 Missense VUS VUS = Variants of Uncertain Significance Table 5: Type of VUS in Non-Arab Population with Confirmed Family History Type of Variation Frequency % Nonsense 0 0 Frameshift 0 0 Missense 9 82 UTR 1 9 Splicing 1 9 Total 11 100 39 Group 4: Patients with Positive Genetic Results for Breast/Ovarian Cancer The findings of the Shapiro-Wilk test for age showed a W-statistic of 0.955 and a p-value of 0.452, so the null hypothesis cannot be rejected, and the data follow a normal distribution. Fisher’s Exact test was performed to compare the gene affected by the type of mutation; this was a statistically significant association as the p-value was 0.0019 (Figure 11). Statistical Significance was not found when comparing the variant type between ethnicities, using Fisher’s Exact Test (p=0.6008), but the test produced significant results when used to compare the gene affected between ethnicities (p=0.0024) (Figure 12). Mann-Whitney U Test was executed to compare age for positive Arabs and non-Arabs, but the result was not statistically significant (p=0.1174) (Figure 13) Figure 11: Distribution of Variant Types Across Different Genes Figure 12: Distribution of Genes Affected Between Both Ethnicities 40 Figure 13: Comparing Positive Result for Arabs & Non-Arabs VS. Age The distribution of pathogenic variants differed amongst the ethnic populations, as illustrated by Table 12. In the Arab patients, the most frequent mutation is in the BRCA1 gene, accounting for 40% of the total mutations observed. Next was the ATM gene at 20%, and BRCA2, BRIP1, and SDHA each at 10%. Notably, there are no mutations observed in the APC, CHEK2, NF1, PALB2, and RAD51D, all of which appeared in the non-Arab population. In non-Arabs, CHEK2 and PALB2 mutations being the most common, each constituting 25% of the total mutations. Mutations in BRCA1 and BRCA2 each account for 16.7%, while mutations in RAD51D and NF1 only represented 8.3% each. ATM, APC, BRIP1, and SDHA genetic variations were not present in the non-Arab population. 41 Table 12: Distribution of Pathogenic Variants Identified in Arab vs Non-Arab Populations 42 Group 5: Patients with Positive Family History of BC/OC The findings of the Shapiro-Wilk test for age showed a W- statistic of 0.990 and a p-value of 0.951 so the null hypothesis cannot be rejected, and the data follow a normal distribution. One-way ANOVA was performed to investigate the difference of age across genetic results, but no significant age differences across genetic test results was found (p=0.3490). There were no significant associations between gender and pathogenic classification (p=0.1668) when performing Fisher’s Exact Test. Additionally, there were no significant associations between ethnicity and pathogenic classification (p=0.5805) when using chi squared (Figure 16). Man-Whitney was used to compare age and ethnicity (p=0.0708), as well as age between pathogenic classification (p=0.0566) (Figure 15), and finally, age with the type of genetic variant, which was statistically significant (p=0.0109) (Figure 14). Figure 14: Comparison of Age with the Type of Variant Figure 15: Comparison of the Age of Arab & Non-Arab + Family History 43 Figure 16: Genetic Result vs Ethnicity Logistic regression was leveraged to investigate possible risk factors only within patients who had confirmed family history for BC/OC. Various models were built to compare the presence or absence of a pathogenic breast cancer gene variant with age, ethnicity, and family history as predictors with different combinations. None of the models showed good predictive ability. However, a few odd ratio trends were identified, as in the model that considered all the factors as predictors, age showed a slight non-significant decrease in disease odds (OR=0.9627, CI: 0.8861 to 1.041) and being of Arab decent showed a non-significant decrease in disease odds (OR=0.5716, CI: 0.1419 to 2.186). Gender showed a non-significant large increase in disease odds (OR=12.60, CI: 0.8918 to 338.4). The same trends were found in models with different combinations of these factors. The same was done to understand whether the same predictors can be used to predict the presence of a BC/OC related genetic variant, whether it was pathogenic or a VUS. The models also did not have strong predictive ability, but the odds ratio again showed a trend, as age showed a slight non-significant increase in variant presence (OR=1.013, CI: 0.9461 to 1.086) and being of Arab ethnicity showed a non-significant increase in variant presence (OR=1.064, CI: 0.3138 to 3.581). Group 6: Patients with "Uncertain Significance" Results The findings of the Shapiro-Wilk test for age showed a W of 0.992 and a p-value of 0.9813 so the null hypothesis cannot be rejected, and the data follow a normal distribution. Fisher’s Exact test was conducted to compare ethnicity and the gene affected, but the results were insignificant (p=0.1228) (Figure 17). The same was test was employed to compare ethnicity and the type of variant, but the results were also insignificant. Fisher’s Exact Test was finally employed to compare the variant type with the gene affected and the results were not statistically significant (p=0.1228) (Figure 18). Man- 44 Whitney U Test was preformed to compare the ages of Arabs and Non-Arabs with VUS genetic results, but the p-value was slightly above the threshold of 0.05, at 0.0600. Figure 17: VUS Genes Distribution Figure 18: Type of Mutation Across Genes Affected 45 The frequency and percentage distribution of gene mutations in Arab and non-Arab populations are shown in Table 13. The ATM gene has the highest frequency of mutations among Arabs (19% of total mutations), followed by POLE (14.3%), BLM (9.5%), BRCA2, and MUTYH. APC, BRCA1, MSH3, MSH6, NTHL1, PALB2, PMS2, and TSC1 exhibit rates that range from 4.8% to 9.5%, whereas other genes display lower frequency. The most common mutations in the non-Arab population are in PALB2 and PMS2, accounting for 18.2% of all mutations. BARD1, MSH6, and POLE follow at 9.1% apiece. APC, BLM, BRCA1, BRCA2, MSH3, MUTYH, NTHL1, RAD51D, SMAD4, TSC1, and TSC2 are among the genes in non-Arabs that do not exhibit any mutations. Table 13: Distribution of Variants of Uncertain Significance Identified in Arab vs Non-Arab Populations with Confirmed Family History 46 Group 7: Unspecified Family history, positive for Breast Cancer In patients who tested positive for breast cancer without specified family history, 6 of the 8 patients were Arab, or 75%. The majority of patients (5 out of 8) were tested for breast cancer, one tested for ovarian, and one tested for both breast and ovary. BRCA1 and BRCA2 were the most affected genes in the cohort, with pathogenic mutations found in several patients. Other affected genes included ATM, BRIP1, RAD51C and SDHA, with a range of ‘variants of uncertain pathogenic significance’. Multiple patients exhibit frameshift mutations in BRCA1, BRCA2 or BRIP1, which are known to always truncate gene products and therefore have the potential to cause cancer. Nonsense mutations and a splicing mutation were also identified. No single genetic variant dominated across different patients or across specific ages. Group 8: Ovarian Cancer Patients Only 6 patients had any clinical indications related to ovarian cancer, 4 of which were Arabs and 2 were not Arabs, with 41 being the most common age. Three patients had only ovarian cancer, while 1 had Breast/pelvic/perianal cancer and 1 had Family history of breast cancer, ovarian cancer and 1 had breast and ovarian cancer. Family history was mostly unspecified (5 patients) and 1 patient had positive family history. 2 of the patients with unspecified family history had a positive family history while 2 patients had uncertain significance and one had negative results. The patient with positive family history had a negative result. No frequent finding in variants of genes affected, which included BRCA2, BRIP1, MUTYH, and PTEN. No frequent finding