BMS 511 Biostats and Statistical Analysis Chapter 15 PDF
Document Details
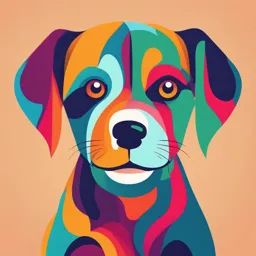
Uploaded by .keeks.
Marian University
2018
Guang Xu
Tags
Summary
This document is a chapter from the BMS 511 textbook, focusing on biostatistics and statistical analysis, specifically dealing with the practical application of inference. The chapter covers key concepts such as confidence intervals, sample size, significance tests, and errors, providing insights into statistical methods. The content is updated to 2018.
Full Transcript
BMS 511 Biostats & Statistical Analysis Chapter 15 Inference in practice Guang Xu, PhD, MPH Assistant Professor of Biostatistics and Public Health College of Osteopathic Medicine Marian University...
BMS 511 Biostats & Statistical Analysis Chapter 15 Inference in practice Guang Xu, PhD, MPH Assistant Professor of Biostatistics and Public Health College of Osteopathic Medicine Marian University Copyright © 2018 W. H. Freeman and Company Previous Learning Objectives Demonstrate the following features of inference Statistical estimation Margin of error and confidence levels Confidence interval for a Normal population mean (σ known) Hypothesis testing The P-value Test for a Normal population mean (σ known) Tests from confidence intervals Copyright © 2018 W. H. Freeman and Company Learning Objectives Apply Inference in practice Conditions for inference in practice How confidence intervals behave How hypothesis tests behave Planning studies Power Copyright © 2018 W. H. Freeman and Company Conditions for inference: random sampling (1 of 2) The data must be a probability sample or come from a randomized experiment. Statistical inference cannot remedy basic design flaws, such as voluntary response samples or uncontrolled experiments. If your data is not gathered appropriately, your conclusions may be challenged. The margin of error does not cover all errors: The margin of error in a confidence interval covers only random sampling error. Copyright © 2018 W. H. Freeman and Company Conditions for inference: random sampling (2 of 2) Undercoverage, nonresponse, or other forms of bias are often more serious than random sampling error (e.g., our elections polls). The margin of error does not take these into account at all. For instance, most opinion polls have very high nonresponse (about 90%). This is not taken into account in the margin of error. Copyright © 2018 W. H. Freeman and Company Conditions for inference: Normality The sampling distribution must be approximately Normal. This is not true in all instances We rarely know the shape of the population distribution, so we rely on our sample data. Always check for outliers and deal with them appropriately. Copyright © 2018 W. H. Freeman and Company Confidence intervals in practice Confidence intervals are used to estimate a population parameter, with a built-in estimate of the precision of that estimate. The basic form of the confidence intervals we have studied is Estimate ± Margin of Error Confidence intervals rely on simple random sampling, and the central limit theorem Copyright © 2018 W. H. Freeman and Company Confidence interval behavior The margin of error for the z confidence interval is Decrease s Decrease confidence (and thus the value of z*) Increase our sample size Copyright © 2018 W. H. Freeman and Company The effect of sample size (1 of 3) For the same confidence level, narrower confidence intervals can be achieved by using larger sample sizes. Copyright © 2018 W. H. Freeman and Company The effect of sample size (2 of 3) Copyright © 2018 W. H. Freeman and Company The effect of sample size (3 of 3) Copyright © 2018 W. H. Freeman and Company Significance tests in practice (1 of 2) Statistical significance only says whether the effect observed is likely to be due to chance alone because of random sampling. Statistical significance doesn’t tell about the magnitude of the effect. Statistical significance may not be practically important. With a large sample size, even a small effect could be significant. Copyright © 2018 W. H. Freeman and Company Significance tests in practice (2 of 2) – A drug to lower temperature is found to consistently lower a patient’s temperature by 0.4° Celsius (P-value < 0.01). But clinical benefits of temperature reduction require a 1°C decrease or greater. Copyright © 2018 W. H. Freeman and Company Sample size affects statistical significance Because large random samples have small chance variation, very small population effects can be highly significant if the sample is large. Because small random samples have a lot of chance variation, even large population effects can fail to be significant if the sample is small. Copyright © 2018 W. H. Freeman and Company Beware of multiple analyses (1 of 2) The probability of incorrectly rejecting H0 (Type I error) is the significance level . If we set = 5% and make multiple analyses, we can expect to make a Type I error about 5% of the time. – If you run only 1 analysis, this is not a problem. – If you try the same analysis with 100 random samples, you can expect about 5 of them to be significant even if H0 is true. Copyright © 2018 W. H. Freeman and Company Beware of multiple analyses (2 of 2) You run a test of hypotheses for extra sensory perception on an individual chosen at random. You then run the same test on 19 other individuals also chosen at random. What's wrong with that? For a significance level = 5%, you can expect that one individual will have a significant result just by chance even if extrasensory perception doesn’t exist. Copyright © 2018 W. H. Freeman and Company Multiple analyses examples (1 of 3) Cell phones and brain cancer Might the radiation from cell phones be harmful to users? Many studies have found little or no connection between using cell phones and various illnesses. Here is part of a news account of one study: A hospital study that compared brain cancer patients and similar patients without brain cancer found no statistically significant association between cell phone use and brain cancer (glioma). But when 20 types of glioma were considered separately, an association was found between phone use and one rare form of glioma. Puzzlingly, however, this risk appeared to decrease rather than increase with greater cell phone use. Interpretation? Copyright © 2018 W. H. Freeman and Company Multiple analyses examples (2 of 3) Mammary artery ligation Angina is the severe pain caused by inadequate blood supply to the heart. Perhaps we can relieve angina by tying off (“ligation”) the mammary arteries to force the body to develop other routes to supply blood to the heart. Patients reported a statistically significant reduction in angina pain. Problem? – This experiment was uncontrolled, so the reduction in pain might be nothing more than the placebo effect. Copyright © 2018 W. H. Freeman and Company Multiple analyses examples (3 of 3) – A randomized comparative experiment later found that ligation was no more effective than a placebo. Surgeons abandoned the procedure. – Statistical significance says that something other than chance is at work, but it doesn’t say what that something is. Copyright © 2018 W. H. Freeman and Company Planning studies: sample size You may need a certain margin of error (e.g., drug trial, manufacturing specs). In many cases, the population variability (s) is fixed, but we can choose the number of measurements (n). Using simple algebra, you can find what sample size is needed to obtain a desired margin of error. Copyright © 2018 W. H. Freeman and Company Calculating sample size (1 of 2) Density of bacteria in solution A measuring equipment gives results that vary Normally with standard deviation σ = 1 million bacteria/mL fluid. How many measurements should you make to obtain a margin of error of at most 0.5 million bacteria/mL with a confidence level of 90%? For a 90% confidence interval, z*= 1.645. Copyright © 2018 W. H. Freeman and Company Calculating sample size (2 of 2) Using only __ measurements will not be enough to ensure that m is no more than 0.5 million/mL. Therefore, we need at least ? measurements. Z* 0.674 0.841 1.036 1.282 1.645 1.960 2.054 2.326 2.576 2.807 3.091 3.291 50% 60% 70% 80% 90% 95% 96% 98% 99% 99.5% 99.8% 99.9% Confidence level C Copyright © 2018 W. H. Freeman and Company The power of a test The power of a test of hypothesis is its ability to detect a specified effect size (reject H0 when a given Ha is true) at significance level α. The specified effect size is chosen to represent a biologically/practically meaningful magnitude. What affects power? The size of the specified effect The significance level α The sample size n The population variance σ2 Copyright © 2018 W. H. Freeman and Company Power calculation Do poor mothers have smaller babies? The national average birth weight is 120 oz: N(natl =120, = 24 oz). We want to be able to detect an average birth weight of 114 oz (5% lower than the national average). What power would we get from an SRS of 100 babies born of poor mothers if we chose a significance level of 0.05? 80% Copyright © 2018 W. H. Freeman and Company Type I and Type II errors (1 of 2) Statistical conclusions are not certain. A Type I error occurs when we reject the null hypothesis but the null hypothesis is actually true (incorrectly reject a true H0). A Type II error occurs when we fail to reject the null hypothesis but the null hypothesis is actually false (incorrectly keep a false H0). Copyright © 2018 W. H. Freeman and Company Type I and Type II errors (2 of 2) The probability of making a Type I error (incorrectly rejecting a true H0) is the significance level . The probability of making a Type II error (incorrectly keeping a false H0) is labeled , a computed value that depends on a number of factors. The power of a test is defined as the value 1 − . A Type II error is not definitive, because “failing to reject the null hypothesis” does not imply that the null hypothesis is true. Copyright © 2018 W. H. Freeman and Company Type I error example A regulatory agency checks air quality for evidence of unsafe levels (> 5.0 ppt) of nitrogen oxide (NOx). The agency gathers NOx concentrations in an urban area on a random sample of 60 different days and calculates a test of significance to assess whether the mean level of NOx is greater than 5.0 ppt. A Type I error here would be to believe that the population mean NOx level A. exceeds 5.0 ppt when it really does. B. exceeds 5.0 ppt when it really doesn’t. C. is 5.0 ppt or less when it really is. D. is 5.0 ppt or less when it really isn’t. Copyright © 2018 W. H. Freeman and Company Learning Objectives Apply Inference in practice Conditions for inference in practice How confidence intervals behave How hypothesis tests behave Planning studies Power Copyright © 2018 W. H. Freeman and Company