BMS 511 Biostats & Statistical Analysis Lecture Notes PDF
Document Details
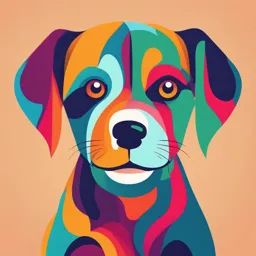
Uploaded by .keeks.
Marian University
Guang Xu
Tags
Summary
This document is lecture notes for a biostatistics course covering an introduction to statistical practices and data visualization. It outlines course information including schedule for exams, office hours, and a project, as well as required texts. Data types and graphical representations of data are also described.
Full Transcript
BMS 511 Biostats & Statistical Analysis Chapter 1 Intro & Displaying Data with Graphs Guang Xu, PhD, MPH Assistant Professor of Biostatistics and Public Health College of Osteopathic Medicine Marian University Biostatistics...
BMS 511 Biostats & Statistical Analysis Chapter 1 Intro & Displaying Data with Graphs Guang Xu, PhD, MPH Assistant Professor of Biostatistics and Public Health College of Osteopathic Medicine Marian University Biostatistics Biostatistics Course Info Course Description This course offers a comprehensive introduction to statistical practices relevant to biomedical and clinical research including the development of experimental questions and approaches to data collection. The course also provides an overview of statistical analysis including basic statistical concepts to selection of appropriate statistical methodology using examples from health care and research, emphasizing the relationship between statistics and medical research. Course Info Required Text: - Baldi, Brigitte, and David S. Moore. The practice of statistics in the life sciences, 4th edition. Macmillan Higher Education, USA. - Gordis, L. Epidemiology: With Student Consult Online Access, 5e. WB Saunders Co., Philadelphia, 5th edition, 2013. ISBN: 978-1455737338. http://libguides.marian.edu/c.php?g=550335&p=6231283 Recommended (Optional Texts): - Motulsky, Harvey. Intuitive biostatistics: a nonmathematical guide to statistical thinking, 4th edition. Oxford University Press, USA. - Robert H. Riffenburgh. Statistics in Medicine, 3rd edition. Elsevier, Netherlands. http://www.elsevierdirect.com/v2/companion.jsp?ISBN=9780123848642 Course Info ITEM % OF GRADE Exam I 16% Exam II 16% Exam III 16% Exam IV 16% In-class Quizzes 16% Homework (10 10% total) Exams are open-book and individual effort. Group Project 10% Quizzes (10) are close-book and graded Homework (10) is counted but not graded Group Project A chance to “apply” the statistics we have learned A chance to try to think critically oWhat’s the story? oWhat are the methods? oWhat are the results? oWhat are the conclusions? oWhat’s the future? oWhat do you think? Office Hour Name: Guang Xu, PhD, MPH Email: [email protected] Phone: 317-955-6496 Office Hours: Tuesday 10:00 – 11:30 (Time may change due to other duties). WebEx Meeting Room: https://mu.webex.com/meet/guangxu Learning Objectives Determine & Apply Picturing Distributions with Graphs Individuals and variables Two types of data: categorical and quantitative Ways to chart categorical data: bar graphs and pie charts Ways to chart quantitative data: histograms and dotplots Interpreting histograms Graphing time series: time plots Copyright © 2018 W. H. Freeman and Company Individuals and variables Individuals are the objects described in a set of data. Individuals may be people, animals, plants, or things. – Freshmen, newborns, golden retrievers, fields of corn, cells A variable is any property that characterizes an individual. A variable can take different values for different individuals. – Age, gender, blood pressure, blood type, leaf length, flower color Copyright © 2018 W. H. Freeman and Company Variable types (1 of 2) A variable can be either quantitative Some quantity assessed or measured for each individual. We can then report the average of all individuals. – Age (in years), blood pressure (in mm Hg), leaf length (in cm) Copyright © 2018 W. H. Freeman and Company Variable types (2 of 2) categorical Some characteristic describing each individual. We can then report the count or proportion of individuals with that characteristic. – Gender (male, female), blood type (A, B, AB, O), flower color (white, yellow, red) Copyright © 2018 W. H. Freeman and Company Classifying variables (1 of 2) Ask: What are the n individuals examined (in the sample or population)? What is being recorded about those n individuals? Is that a number ( quantitative) or a statement ( categorical)? Copyright © 2018 W. H. Freeman and Company Classifying variables (2 of 2) Individuals studied Diagnosis Age at death Patient A Heart disease 56 Patient B Stroke 70 Patient C Stroke 75 Patient D Lung cancer 60 Patient E Heart disease 80 Patient F Accident 73 Patient G Diabetes 69 Diagnosis: Each individual is given a description. Age at death: Each individual is given a meaningful number. Copyright © 2018 W. H. Freeman and Company Classifying variables examples (1 of 5) Researchers grafted human cancerous cells onto 20 healthy adult mice. Then 10 of the mice were injected with tumor-specific antibodies (anti- CD47) while the other 10 mice were not (IgG). Here are some published results. 10 Number of mice with metastases 8 6 4 2 0 IgG anti-CD47 Number of mice exhibiting metastases in each group Copyright © 2018 W. H. Freeman and Company Classifying variables examples (2 of 5) 4 Total number of metastases 3 2 1 0 IgG anti-CD47 Number of metastases detected in each mouse Who/what are the individuals? What are the variables, and are they quantitative or categorical? Copyright © 2018 W. H. Freeman and Company Classifying variables examples (3 of 5) Researchers grafted human cancerous cells onto 20 healthy adult mice. Then 10 of the mice were injected with tumor-specific antibodies (anti-CD47) while the other 10 mice were not (IgG). Here is what a table of the raw data would look like. Copyright © 2018 W. H. Freeman and Company Classifying variables examples (4 of 5) Mouse Treatment Presence of Number of metastases metastases 1 IgG yes 1 2 IgG yes 1 3 IgG yes 2 4 IgG yes 2 5 IgG yes 2 6 IgG yes 3 7 IgG yes 3 8 IgG yes 3 9 IgG yes 3 10 IgG yes 4 11 anti-CD47 no 0 12 anti-CD47 no 0 13 anti-CD47 no 0 Copyright © 2018 W. H. Freeman and Company Classifying variables examples (5 of 5) Mouse Treatment Presence of metastases Number of metastases 14 anti-CD47 no 0 15 anti-CD47 no 0 16 anti-CD47 no 0 17 anti-CD47 no 0 18 anti-CD47 no 0 19 anti-CD47 no 0 20 anti-CD47 yes 1 Copyright © 2018 W. H. Freeman and Company Graphing categorical data (1 of 5) Most common ways to graph categorical data: – Bar graphs Each characteristic, or level, is represented by a bar. The height of a bar represents either the count of individuals with that characteristic, the frequency, or the percent of individuals with that characteristic, the relative frequency. Copyright © 2018 W. H. Freeman and Company Graphing categorical data (2 of 5) – Pie charts A pie chart can only represent how one categorical variable breaks down into its components. Each characteristic is represented by a slice, and the size of a slice represents what percent of the whole is made up by that characteristic. Copyright © 2018 W. H. Freeman and Company Graphing categorical data (3 of 5) Do you like…? Subject Carrots Peas Spinach 1 yes yes yes 2 yes No no 3 yes yes no 4 no no no 5 yes no no 6 no yes yes Carrots Peas Spinach Percent who like 67% 50% 33% Percent who don't 33% 50% 67% (Note the numbers do not add to 100%. The values are summaries of three separate variables.) Copyright © 2018 W. H. Freeman and Company Graphing categorical data (4 of 5) Which one do you prefer? Subject Preference 1 Peas 2 Carrots 3 Carrots 4 Spinach 5 Carrots 6 Peas Percent who prefer Carrots 50% Peas 33% Spinach 17% (Note the numbers add to 100%. The values are summaries of one categorical variable.) Copyright © 2018 W. H. Freeman and Company Graphing categorical data (5 of 5) Copyright © 2018 W. H. Freeman and Company Interpreting bar graphs Percent of current marijuana users in each of four age groups: USA, 2009 Who/what are the individuals? What are the variables, and are they quantitative or categorical? What type of graph is this? Could these data be represented in a pie chart? Copyright © 2018 W. H. Freeman and Company Graphing quantitative data (1 of 2) Histograms – A histogram is a summary graph for a single variable. It is useful to understand the pattern of variability, especially for large data sets. Dotplots – A dotplot is a graph of the raw data. It is useful to describe the pattern of variability, especially for small data sets. Copyright © 2018 W. H. Freeman and Company Graphing quantitative data (2 of 2) Time plots – A time plot is a graph with a sequence for the horizontal variable, like time. The line connecting the points helps emphasize any change over time. Other graphs to display numerical summaries (see Chapter 2) Copyright © 2018 W. H. Freeman and Company Making a histogram (1 of 5) 1. The range of values that the quantitative variable takes is divided into equal-size intervals, or classes. This makes up the horizontal axis. 2. The vertical axis represents either – the frequency (counts) or – the relative frequency (percents of total). 3. For each class on the horizontal axis, draw a column. The height of the column represents the count (or percent) of data points that fall in that class interval. Copyright © 2018 W. H. Freeman and Company Making a histogram (2 of 5) Guinea pig survival time (in days) after inoculation with a pathogen (n = 72) Let’s build a histogram with classes of size 50, starting at zero (zero is included in the first class). Copyright © 2018 W. H. Freeman and Company Making a histogram (3 of 5) Copyright © 2018 W. H. Freeman and Company Making a histogram (4 of 5) Copyright © 2018 W. H. Freeman and Company Making a histogram (5 of 5) 43 45 53 56 56 57 58 66 67 73 74 79 80 80 81 81 81 82 83 83 84 88 89 91 91 92 92 97 99 99 100 100 101 102 102 102 103 104 107 108 109 113 114 118 121 123 126 128 137 138 139 144 145 147 156 162 174 178 179 184 191 198 211 214 243 249 329 380 403 511 522 598 Copyright © 2018 W. H. Freeman and Company Choosing histogram classes (1 of 2) It is an iterative process—try and try again. Not too many classes with either 0 or 1 counts (pancake graph) Not overly summarized that you lose all the information (skyscraper graph) Not so detailed that it is no longer a summary (pancake graph) Try starting with 5 to10 classes, then refine your class choice. (There isn’t a unique or “perfect” solution.) Copyright © 2018 W. H. Freeman and Company Choosing histogram classes (2 of 2) Copyright © 2018 W. H. Freeman and Company Interpreting histograms We look for the overall pattern and for striking deviations from that pattern. We describe the histogram’s Shape—Unimodal, Bimodal, Symmetric, Skewed, Irregular Center—Approximate midpoint Spread—Range of values taken Possible outliers Copyright © 2018 W. H. Freeman and Company Common distribution shapes (1 of 3) Symmetric distribution The left half of the shape is a mirror image of the right half. Copyright © 2018 W. H. Freeman and Company Common distribution shapes (2 of 3) Left-skewed distribution The left side (the side with the extreme values) extends much farther out than the right side. Copyright © 2018 W. H. Freeman and Company Common distribution shapes (3 of 3) Right-skewed distribution The right side (the side with extreme values) extends much farther out than the left side. Copyright © 2018 W. H. Freeman and Company Histogram shapes examples (1 of 4) Describe the shape of these histograms. 14 12 10 Frequency 8 6 4 2 0 22 27 32 37 42 Percent of births in each state delivered by C-section Copyright © 2018 W. H. Freeman and Company Histogram shapes examples (2 of 4) 7 6 5 Frequency 4 3 2 1 0 0.70 0.75 0.80 0.85 0.90 0.95 1.00 1.05 1.10 Realized growth rate of 21 shark populations Copyright © 2018 W. H. Freeman and Company Histogram shapes examples (3 of 4) 16000 14000 12000 10000 Frequency 8000 6000 4000 2000 0 0 6 12 18 24 30 Time of post-hospital discharge complication (in days) Copyright © 2018 W. H. Freeman and Company Histogram shapes examples (4 of 4) Describe the shape of this histogram. Patient age (in years) for 241,931 cases of Lyme disease reported in the U.S. (19922006, CDC) Remember: Not all distributions have a simple shape! Copyright © 2018 W. H. Freeman and Company Outliers (1 of 2) An important kind of deviation is an outlier. Outliers are observations that lie outside the overall pattern of a distribution. Always look for outliers and try to explain them. Anonymous class survey: weight (lbs) and height (in) were used to compute BMI. Copyright © 2018 W. H. Freeman and Company Outliers (2 of 2) Caution: the largest observation is not necessarily an outlier; for it to be an outlier, it must be different from the rest of the pattern. In this histogram, there are 4 intervals just before the outlier with no observations at all! Copyright © 2018 W. H. Freeman and Company Making a dotplot (1 of 2) 1) Create a single axis representing the quantitative variable’s range. 2) Represent each data point as a dot positioned according to its numerical value. 3) When two or more data points have the same value, stack them up. Copyright © 2018 W. H. Freeman and Company Making a dotplot (2 of 2) Raw data 28 12 23 14 40 18 22 33 26 27 29 11 35 30 34 22 23 35 Sorted data Copyright © 2018 W. H. Freeman and Company Graphing time series Data collected over time are displayed in a time plot, with time on the horizontal axis and the variable of interest on the vertical axis. We look for a possible trend (a clear overall pattern) and possible cyclical variations (variations with some Monthly atmospheric CO2 levels regularity over time) recorded at the Mauna Loa Hawaii observatory (March 1958– February 2014) Copyright © 2018 W. H. Freeman and Company Interpreting time series (1 of 2) Describe these two graphs. Copyright © 2018 W. H. Freeman and Company Interpreting time series (2 of 2) Copyright © 2018 W. H. Freeman and Company Homework Homework can be found at Canvas Module Homework