received_621282627466080.jpeg
Document Details
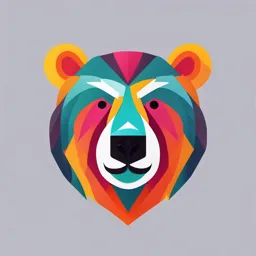
Uploaded by ThrilledChalcedony5168
Full Transcript
# The Poisson Process ## Definition A Poisson process is a model for a series of discrete events where the average time between events is known, but the exact timing of events is random. The process is memoryless, meaning that the time of the next event is independent of the time of the last event...
# The Poisson Process ## Definition A Poisson process is a model for a series of discrete events where the average time between events is known, but the exact timing of events is random. The process is memoryless, meaning that the time of the next event is independent of the time of the last event. Poisson processes are often used to model events such as: * Customers arriving in a store * Calls arriving at a call center * Particles emitted from a radioactive source * Traffic accidents ## Properties A Poisson process is characterized by the following properties: * Events occur one at a time * Events are independent of each other * The average rate of events is constant ## Formal Definition Let $N(t)$ be the number of events that have occurred up to time $t$. A Poisson process with rate $\lambda > 0$ is a stochastic process $\{N(t), t \geq 0\}$ satisfying: * $N(0) = 0$ * The process has independent increments: For any $0 \leq t\_1 < t\_2 <... < t\_n$, the random variables $N(t\_2) - N(t\_1), N(t\_3) - N(t\_2),..., N(t\_n) - N(t\_{n-1})$ are independent. * The number of events in any interval of length $t$ follows a Poisson distribution with mean $\lambda t$: $P(N(t + s) - N(s) = n) = \frac{e^{-\lambda t}(\lambda t)^n}{n!}$, for $n = 0, 1, 2,...$ ## Inter-arrival Times Let $T\_1, T\_2,...$ be the times between successive events in a Poisson process. These are called the inter-arrival times. The inter-arrival times are independent and identically distributed exponential random variables with parameter $\lambda$. $P(T\_i > t) = e^{-\lambda t}$ $f(t) = \lambda e^{-\lambda t}, t \geq 0$ $E[T\_i] = \frac{1}{\lambda}$ $Var(T\_i) = \frac{1}{\lambda^2}$ ## Example Suppose customers arrive at a store according to a Poisson process with a rate of 10 customers per hour. * What is the probability that no customers arrive in the first 30 minutes? $P(N(0.5) = 0) = \frac{e^{-10 \cdot 0.5}(10 \cdot 0.5)^0}{0!} = e^{-5} \approx 0.0067$ * What is the probability that more than 10 customers arrive in the first hour? $P(N(1) > 10) = 1 - P(N(1) \leq 10) = 1 - \sum\_{n=0}^{10} \frac{e^{-10}(10)^n}{n!} \approx 0.417$ ## Key Points * Poisson process models events occurring randomly in time. * The number of events in a given time interval follows a Poisson distribution. * The time between events follows an exponential distribution. * The rate $\lambda$ determines the average number of events per unit time.