BMS 511 Biostats & Statistical Analysis Chapter 10 PDF
Document Details
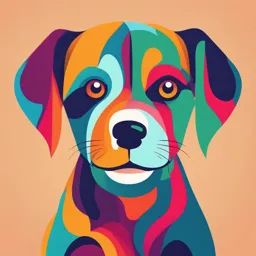
Uploaded by .keeks.
Marian University
2018
Guang Xu
Tags
Summary
This document is a chapter from a biostatistics textbook. It covers the concepts of independence and conditional probabilities, including examples and explanations. The document is from 2018.
Full Transcript
BMS 511 Biostats & Statistical Analysis Chapter 10 Independence and Conditional Probabilities Guang Xu, PhD, MPH Assistant Professor of Biostatistics and Public Health College of Osteopathic Medicine...
BMS 511 Biostats & Statistical Analysis Chapter 10 Independence and Conditional Probabilities Guang Xu, PhD, MPH Assistant Professor of Biostatistics and Public Health College of Osteopathic Medicine Marian University Copyright © 2018 W. H. Freeman and Company Previous Learning Objectives Introducing probability Randomness and probability Probability models Probability rules Discrete vs. continuous models Random variables Means and variances for discrete models Risk and odds Copyright © 2018 W. H. Freeman and Company Learning Objectives Define general rules of probability Independent events Conditional probability General addition rule Multiplication rule Tree diagrams Bayes’s theorem Diagnosis tests Copyright © 2018 W. H. Freeman and Company Independent events Two events are independent if knowing that one event is true or has happened does not change the probability of the other event. “male” and “get heads when flipping a coin” → independent “male” and “taller than 6 ft” → not independent “male” and “high cholesterol” → it’s not obvious (I’m guessing independent) “male” and “pregnant” → not independent Copyright © 2018 W. H. Freeman and Company Sampling without replacement (1 of 2) Pick one frog at random from your target population and don’t put it back. Then pick another frog, etc. Artificial pond with 10 male and 10 female frogs. P(1st frog is male) = 10/20 = 0.5. If 1st frog is male, P(2nd frog is male) = 9/19 = 0.47 (≠ 0.5). → Here, successive picks are not independent. Copyright © 2018 W. H. Freeman and Company Sampling without replacement (2 of 2) Survey of a whole county with thousands of frogs (half males, half females). P(1st frog is male) = 0.5. If 1st frog is male, P(2nd frog is male) ≈ 0.5. → Here, successive picks are “nearly” independent. Copyright © 2018 W. H. Freeman and Company Conditional probability Conditional probabilities reflect how the probability of an event can be different if we know that some other event has occurred or is true. The conditional probability 𝑃 𝐴 and 𝐵 of event B, given event A is: 𝑃 𝐵𝐴 = 𝑃 𝐴 (provided that P(A) ≠ 0) When two events A and B are independent, P(B | A) = P(B). No information is gained from the knowledge of event A. Copyright © 2018 W. H. Freeman and Company Independence example Probabilities of hearing impairment and blue eyes among Dalmatian dogs. HI = Dalmatian is hearing impaired B = Dalmatian is blue eyed P(HI and B) = 0.05 P(HI) = 0.28 P(B) = 0.11 P(HI | B) = P(HI and B) / P(B) = 0.05/0.11≈ 0.45 P(HI |B) ≠ P(HI ), therefore HI and B are not independent. Copyright © 2018 W. H. Freeman and Company Another independence example (1 of 2) Large-scale surveys indicate that 11% of the population smokes. Medical researchers know that the probability that a smoker will get lung cancer is 0.34. The probability that a person will get lung cancer if the person doesn’t smoke is 0.03. Copyright © 2018 W. H. Freeman and Company Another independence example (2 of 2) P(Lung cancer | Smoker) is A. 0.03 B. 0.0394 C. 0.34 D. 0.583 E. 0.97 Are “Smoker” and “Lung cancer” independent? A. Yes B. No Copyright © 2018 W. H. Freeman and Company General addition rule (1 of 2) Addition rule for disjoint events: When two events A and B are disjoint: P(A or B) = P(A) + P(B) Copyright © 2018 W. H. Freeman and Company General addition rule (2 of 2) General addition rule for any two events A and B: P(A or B) = P(A) + P(B) – P(A and B) Copyright © 2018 W. H. Freeman and Company Multiplication rule General multiplication rule: The probability that any two events, A and B, both occur is: P(A and B) = P(A)P(B|A) Multiplication rule for independent events: If A and B are independent, then: P(A and B) = P(A)P(B) Copyright © 2018 W. H. Freeman and Company Multiplication rule examples (1 of 2) Artificial pond with 10 male and 10 female frogs Successive captures are not independent. Probability of randomly capturing 2 male frogs in a row: P(male and male) = P(1st is male) P(2nd is male | 1st is male) = (10/20)(9/19) ≈ 0.237 Copyright © 2018 W. H. Freeman and Company Multiplication rule examples (2 of 2) Blood donation center Unrelated visitors are independent. Probability that the next two unrelated visitors are both type O: P(O and O) = P(O) * P(O) = (0.45)(0.45) ≈ 0.2025 Copyright © 2018 W. H. Freeman and Company Tree diagrams (1 of 2) Tree diagrams are used to represent probabilities graphically and facilitate computations. Probabilities of skin cancer among men and women by body locations. Copyright © 2018 W. H. Freeman and Company Tree diagrams (2 of 2) In a random individual with skin cancer: P(head) = 0.15 P(trunk) = 0.41 P(limbs) = 0.44 % in each group who are women: P(woman | head) = 0.56 P(woman | trunk) = 0.37 P(woman | limbs) = 0.80 Copyright © 2018 W. H. Freeman and Company Tree diagram example—diagnostic tests If a person gets a positive test result, what is the probability that he/she actually has the disease? This is the positive predictive value: PPV = P(disease | positive test) Copyright © 2018 W. H. Freeman and Company Bayes Rule Bayes Rule (also known as Bayes’ formula or theorem) Named for English clergyman Thomas Bayes (1702-1761). Formula for getting from one conditional probability to another: 𝑃 𝐴 𝑃(𝐵|𝐴) P(A|B) = 𝑃(𝐵) Example: P(die between 3 and 4|die between 1 and 3) = P(between 3 and 4)P(between 1 and 3|between 3 and 4) P(between 1 and 3) 2/6×1/2 = 3/6 = 1/3 Bayes’s theorem Suppose that A1, A2,..., Ak are disjoint events. Suppose that the probabilities of A1, A2,..., Ak add to 1. Suppose B is any other event. Bayes’s theorem states: 𝑃 𝐵 𝐴𝑖 𝑃 𝐴𝑖 𝑃 𝐴𝑖 𝐵 = 𝑃 𝐵 𝐴1 𝑃 𝐴1 + 𝑃 𝐵 𝐴2 𝑃 𝐴 2 + ⋯ + 𝑃 𝐵 𝐴𝑘 𝑃 𝐴 𝑘 Copyright © 2018 W. H. Freeman and Company Diagnosis tests If a person gets a positive test result, what is the probability that he/she actually has the disease? This is the positive predictive value: PPV = P(disease | positive test) Copyright © 2018 W. H. Freeman and Company Comparison of Screening/Diagnostic Test Results with Actual Disease Status Test Population Results Disease No Disease Positive Have disease and have No disease but have positive positive test result = true test result = false positive positive (TP) (FP) Negative Have disease but have No disease and have negative test result = false negative test result = true negative (FN) negative (TN) Diagnostic tests example (1 of 3) HIV-AIDS: Suppose that about 1% of a large population has HIV- AIDS antibodies. The enzyme immuno-assay test has sensitivity 0.9985 and specificity 0.9940. If a person who takes this test gets a positive test result, what is the probability that he or she actually has HIV-AIDS, P(HIV-AIDS | positive test)? Copyright © 2018 W. H. Freeman and Company Diagnostic tests example (2 of 3) What’s the positive predictive value of the enzyme immuno-assay test for HIV-AIDS? PPV = P(disease | positive) Copyright © 2018 W. H. Freeman and Company Diagnostic tests example (3 of 3) P(true-positive) = P(disease and positive) = P(disease)P(positive | disease) = (0.01)(0.9985) = 0.009985 P(false-positive) = P(no disease and positive) = P(no disease)P(positive | no disease) = (0.99)(0.006) = 0.00594 PPV = P(disease | positive) = P(disease and positive) / P(positive) = P(true-positive) / P(all positives) = 0.009985 / (0.009985 + 0.00594) ≈ 62.7% Copyright © 2018 W. H. Freeman and Company Another diagnostic tests example (1 of 3) What is the rate of prostate cancer among mature men? P(cancer)= ? For every 100 men who have a PSA blood test: 10 will have an elevated PSA 90 will have a normal PSA Copyright © 2018 W. H. Freeman and Company Another diagnostic tests example (2 of 3) How “sensitive” is the PSA test? P(+ | cancer)= ? How “specific” is the PSA test? P(– | no cancer)= ? If a man gets an elevated PSA test (+), what is the probability that he has prostate cancer? PPV = P(cancer | +)= ? Of the 10 with elevated PSAs: 3 will have prostate cancer (true positive) 7 will not have prostate cancer (false positive) Copyright © 2018 W. H. Freeman and Company Another diagnostic tests example (3 of 3) Of the 90 with normal PSAs: 1 will have prostate cancer (false negative) 89 will not have prostate cancer (true negative) Copyright © 2018 W. H. Freeman and Company Learning Objectives Define general rules of probability Independent events Conditional probability General addition rule Multiplication rule Tree diagrams Bayes’s theorem Diagnosis tests Copyright © 2018 W. H. Freeman and Company