Big Data Unit 1 PDF
Document Details
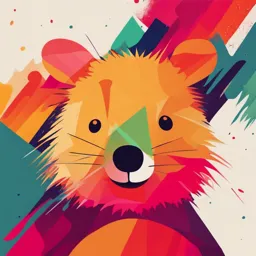
Uploaded by ExaltingSeries1860
Tags
Related
Summary
This document covers Unit 1's content related to Big Data, exploring its history, different types, importance for businesses, and the challenges involved.
Full Transcript
Unit 1 The History of Big Data Although the concept of big data itself is relatively new, the origins of large data sets go back to the 1960s and '70s when the world of data was just getting started with the first data centers and the development of the relational database. Around 200 5, peop...
Unit 1 The History of Big Data Although the concept of big data itself is relatively new, the origins of large data sets go back to the 1960s and '70s when the world of data was just getting started with the first data centers and the development of the relational database. Around 200 5, people began to realize just how much data users generated through Facebook, YouTube, and other online services. Hadoop (an open-source framework created specifically to store and analyze big data sets) was developed that same year. NoSQL also began to gain popularity during this time. The development of open-source frameworks, such as Hadoop (and more recently, Spark) was essential for the growth of big data because they make big data easier to work with and cheaper to store. In the years since then, the volume of big data has skyrocketed. Users are still generating huge amounts of data—but it’s not just humans who are doing it. With the advent of the Internet of Things (IoT), more objects and devices are connected to the internet, gathering data on customer usage patterns and product performance. The emergence of machine learning has produced still more data. While big data has come far, its usefulness is only just beginning. Cloud computing has expanded big data possibilities even further. The cloud offers truly elastic scalability, where developers can simply spin up ad hoc clusters to test a subset of data. “Big data” is high-volume, velocity, and variety information assets that demand cost-effective, innovative forms of information processing for enhanced insight and decision making.” This definition clearly answers the “What is Big Data?” question – Big Data refers to complex and large data sets that have to be processed and analyzed to uncover valuable information that can benefit businesses and organizations. However, there are certain basic tenets of Big Data that will make it even simpler to answer what is Big Data: ❑It refers to a massive amount of data that keeps on growing exponentially with time. ❑It is so voluminous that it cannot be processed or analyzed using conventional data processing techniques. ❑It includes data mining, data storage, data analysis, data sharing, and data visualization. ❑The term is an all-comprehensive one including data, data frameworks, along with the tools and techniques used to process and analyze the data. Part I of the definition “big data is high-volume, high-velocity, and high-variety information assets” talks about voluminous data (humongous data) that may have great variety (a good mix of structured, semi- structured, and unstructured data) and will require a good speed/pace for storage, preparation, processing, and analysis. Part II of the definition “cost effective, innovative forms of information processing” talks about embracing new techniques and technologies to capture (ingest), store, process, persist, integrate, and visualize the high- volume, high-velocity, and high-variety data. Part III of the definition “enhanced insight and decision making” talks about deriving deeper, richer, and meaningful insights and then using these insights to make faster and better decisions to gain business value and thus a competitive edge. Data → Information → Actionable intelligence → Better decisions → Enhanced business value (Data is an individual unit that contains raw materials which do not carry any specific meaning. Information is a group of data that collectively carries a logical meaning.) Why is Big Data Important? 1. Cost Savings: Some tools of Big Data like Hadoop and Cloud-Based Analytics can bring cost advantages to business when large amounts of data are to be stored and these tools also help in identifying more efficient ways of doing business. 2. Time Reductions: The high speed of tools like Hadoop and in-memory analytics can easily identify new sources of data which helps businesses analyzing data immediately and make quick decisions based on the learning. 3. Understand the market conditions: By analyzing big data you can get a better understanding of current market conditions. For example, by analyzing customers’ purchasing behaviors, a company can find out the products that are sold the most and produce products according to this trend. By this, it can get ahead of its competitors. 4. Control online reputation: Big data tools can do sentiment analysis. Therefore, you can get feedback about who is saying what about your company. If you want to monitor and improve the online presence of your business, then, big data tools can help in all this. 5. Using Big Data Analytics to Boost Customer Acquisition and Retention The customer is the most important asset any business depends on. There is no single business that can claim success without first having to establish a solid customer base. However, even with a customer base, a business cannot afford to disregard the high competition it faces. If a business is slow to learn what customers are looking for, then it is very easy to begin offering poor quality products. In the end, loss of clientele will result, and this creates an adverse overall effect on business success. The use of big data allows businesses to observe various customer related patterns and trends. Observing customer behavior is important to trigger loyalty. 6. Using Big Data Analytics to Solve Advertisers Problem and Offer Marketing Insights Big data analytics can help change all business operations. This includes the ability to match customer expectation, changing company’s product line and of course ensuring that the marketing campaigns are powerful. 7. Big Data Analytics As a Driver of Innovations and Product Development Another huge advantage of big data is the ability to help companies innovate and redevelop their products. Why bigdata? The quantity of data on planet earth is growing exponentially for many reasons. Various sources and our day to day activities generates lots of data. With the invent of the web, the whole world has gone online, every single thing we do leaves a digital trace. With the smart objects going online, the data growth rate has increased rapidly. The major sources of Big Data are social media sites, sensor networks, digital images/videos, cell phones, purchase transaction records, web logs, medical records, archives, military surveillance, eCommerce, complex scientific research and so on. All these information amounts to around some Quintillion bytes of data. By 2020, the data volumes will be around 40 Zettabytes which is equivalent to adding every single grain of sand on the planet multiplied by seventy-five. Big Data is a term used for a collection of data sets that are large and complex, which is difficult to store and process using available database management tools or traditional data processing applications. The challenge includes capturing, curating, storing, searching, sharing, transferring, analyzing and visualization of this data. Sources of Big data Social networking sites: Facebook, Google, LinkedIn all these sites generates huge amount of data on a day to day basis as they have billions of users worldwide. E-commerce site: Sites like Amazon, Flipkart, Alibaba generates huge amount of logs from which users buying trends can be traced. Weather Station: All the weather station and satellite gives very huge data which are stored and manipulated to forecast weather. Telecom company: Telecom giants like Airtel, Vodafone study the user trends and accordingly publish their plans and for this they store the data of its million users. Share Market: Stock exchange across the world generates huge amount of data through its daily transaction. Characteristics of data ⮚ Composition: The composition of data deals with the structure of data, that is, the sources of data, the granularity, the types, and the nature of data as to whether it is static or real-time streaming. ⮚ Condition: The condition of data deals with the state of data, that is, “Can one use this data as is for analysis?” or “Does it require cleansing for further enhancement and enrichment?” ⮚ Context: The context of data deals with “Where has this data been generated?” “Why was this data generated?” “How sensitive is this data?” “What are the events associated with this data?” and so on. Big Data Characteristics 1. VOLUME Volume refers to the ‘amount of data’, which is growing day by day at a very fast pace. The size of data generated by humans, machines and their interactions on social media itself is massive. Researchers have predicted that 40 Zettabytes (40,000 Exabytes) will be generated by 2020, which is an increase of 300 times from 2005. 2. VELOCITY Velocity is defined as the pace at which different sources generate the data every day. This flow of data is massive and continuous. There are 1.03 billion Daily Active Users (Facebook DAU) on Mobile as of now, which is an increase of 22% year-over-year. This shows how fast the number of users are growing on social media and how fast the data is getting generated daily. If you are able to handle the velocity, you will be able to generate insights and take decisions based on real-time data. 3. VARIETY As there are many sources which are contributing to Big Data, the type of data they are generating is different. It can be structured, semi-structured or unstructured. Hence, there is a variety of data which is getting generated every day. Earlier, we used to get the data from excel and databases, now the data are coming in the form of images, audios, videos, sensor data etc. as shown in below image. Hence, this variety of unstructured data creates problems in capturing, storage, mining and analyzing the data. 4. VERACITY Veracity refers to the data in doubt or uncertainty of data available due to data inconsistency and incompleteness. In the image below, you can see that few values are missing in the table. Also, a few values are hard to accept, for example – 15000 minimum value in the 3rd row, it is not possible. This inconsistency and incompleteness is Veracity. Data available can sometimes get messy and maybe difficult to trust. With many forms of big data, quality and accuracy are difficult to control like Twitter posts with hashtags, abbreviations, typos and colloquial speech. The volume is often the reason behind for the lack of quality and accuracy in the data. Due to uncertainty of data, 1 in 3 business leaders don’t trust the information they use to make decisions. It was found in a survey that 27% of respondents were unsure of how much of their data was inaccurate. Poor data quality costs the US economy around $3.1 trillion a year 5. VALUE After discussing Volume, Velocity, Variety and Veracity, there is another V that should be taken into account when looking at Big Data i.e. Value. It is all well and good to have access to big data but unless we can turn it into value it is useless. By turning it into value I mean, Is it adding to the benefits of the organizations who are analyzing big data? Is the organization working on Big Data achieving high ROI (Return On Investment)? Unless, it adds to their profits by working on Big Data, it is useless. Types of Big Data 1. Structured : The data that can be stored and processed in a fixed format is called as Structured Data. Data stored in a relational database management system (RDBMS) is one example of ‘structured’ data. It is easy to process structured data as it has a fixed schema. Structured Query Language (SQL) is often used to manage such kind of Data. 2. Semi-Structured : Semi-Structured Data is a type of data which does not have a formal structure of a data model, i.e. a table definition in a relational DBMS, but nevertheless it has some organizational properties like tags and other markers to separate semantic elements that makes it easier to analyze. XML files or JSON documents are examples of semi-structured data. 3. Unstructured : The data which have unknown form and cannot be stored in RDBMS and cannot be analyzed unless it is transformed into a structured format is called as unstructured data. Text Files and multimedia contents like images, audios, videos are example of unstructured data. The unstructured data is growing quicker than others, experts say that 80 percent of the data in an organization are unstructured Examples of Big Data Walmart handles more than 1 million customer transactions every hour. Facebook stores, accesses, and analyzes 30+ Petabytes of user generated data. 230+ millions of tweets are created every day. More than 5 billion people are calling, texting, tweeting and browsing on mobile phones worldwide. YouTube users upload 48 hours of new video every minute of the day. Amazon handles 15 million customer click stream user data per day to recommend products. 294 billion emails are sent every day. Services analyses this data to find the spams. Modern cars have close to 100 sensors which monitors fuel level, tire pressure etc. , each vehicle generates a lot of sensor data. Applications of Big Data Smarter Healthcare: Making use of the petabytes of patient’s data, the organization can extract meaningful information and then build applicationsthat can predict the patient’s deteriorating condition in advance. Telecom: Telecom sectors collects information, analyzes it and provide solutions to different problems. By using Big Data applications, telecom companies have been able to significantly reduce data packet loss, which occurs when networks are overloaded,and thus, providing a seamless connection to their customers. Retail: Retail has some of the tightest margins, and is one of the greatest beneficiaries of big data. The beauty of using big data in retail is to understand consumer behavior. Amazon’s recommendation engine provides suggestion based on the browsing history of the consumer. Traffic control: Traffic congestion is a major challenge for many cities globally. Effective use of data and sensors will be key to managing traffic better as cities become increasingly densely populated. Manufacturing: Analyzing big data in the manufacturing industry can reduce component defects, improve product quality, increase efficiency,and save time and money. Search Quality: Every time we are extracting information from google, we are simultaneously generating data for it. Google stores this data and uses it to improve its search quality. Challenges 1. Need For Synchronization Across Disparate Data Sources As data sets are becoming bigger and more diverse, there is a big challenge to incorporate them into an analytical platform. If this is overlooked, it will create gaps and lead to wrong messages and insights. 2. Acute Shortage Of Professionals Who Understand Big Data Analysis The analysis of data is important to make this voluminous amount of data being produced in every minute, useful. With the exponential rise of data, a huge demand for big data scientists and Big Data analysts has been created in the market. It is important for business organizations to hire a data scientist having skills that are varied as the job of a data scientist is multidisciplinary. Another major challenge faced by businesses is the shortage of professionals who understand Big Data analysis. There is a sharp shortage of data scientists in comparison to the massive amount of data being produced. 3. Getting Meaningful Insights Through The Use Of Big Data Analytics It is imperative for business organizations to gain important insights from Big Data analytics, and also it is important that only the relevant department has access to this information. A big challenge faced by the companies in the Big Data analytics is mending this wide gap in an effective manner. 4. Getting Voluminous Data Into The Big Data Platform It is hardly surprising that data is growing with every passing day. This simply indicates that business organizations need to handle a large amount of data on daily basis. The amount and variety of data available these days can overwhelm any data engineer and that is why it is considered vital to make data accessibility easy and convenient for brand owners and managers. 5. Uncertainty Of Data Management Landscape With the rise of Big Data, new technologies and companies are being developed every day. However, a big challenge faced by the companies in the Big Data analytics is to find out which technology will be best suited to them without the introduction of new problems and potential risks. 6. Data Storage And Quality Business organizations are growing at a rapid pace. With the tremendous growth of the companies and large business organizations, increases the amount of data produced. The storage of this massive amount of data is becoming a real challenge for everyone. Popular data storage options like data lakes/ warehouses are commonly used to gather and store large quantities of unstructured and structured data in its native format. The real problem arises when a data lakes/ warehouse try to combine unstructured and inconsistent data from diverse sources, it encounters errors. Missing data, inconsistent data, logic conflicts, and duplicates data all result in data quality challenges. 7. Security And Privacy Of Data Once business enterprises discover how to use Big Data, it brings them a wide range of possibilities and opportunities. However, it also involves the potential risks associated with big data when it comes to the privacy and the security of the data. The Big Data tools used for analysis and storage utilizes the data disparate sources. This eventually leads to a high risk of exposure of the data, making it vulnerable. Thus, the rise of voluminous amount of data increases privacy and security concerns. Traditional Business Intelligence (BI) versus Big Data Differences that one encounters dealing with traditional BI and big data. 1. In traditional BI environment, all the enterprise’s data is housed in a central server whereas in a big data environment data resides in a distributed file system. The distributed file system scales by scaling in or out horizontally as compared to typical database server that scales vertically. 2. In traditional BI, data is generally analyzed in an offline mode whereas in big data, it is analyzed in both real time as well as in offline mode. 3. Traditional BI is about structured data and it is here that data is taken to processing functions (move data to code) whereas big data is about variety: Structured, semi-structured, and unstructured data and here the processing functions are taken to the data (move code to data). A Typical Data Warehouse Environment A Data Warehouse (DW) is a relational database that is designed for query and analysis rather than transaction processing. It includes historical data derived from transaction data from single and multiple sources. A Data Warehouse provides integrated, enterprise-wide, historical data and focuses on providing support for decision-makers for data modeling and analysis. A Data Warehouse is a group of data specific to the entire organization, not only to a particular group of users. It is not used for daily operations and transaction processing but used for making decisions. A Data Warehouse can be viewed as a data system with the following attributes: It is a database designed for investigative tasks, using data from various applications. It supports a relatively small number of clients with relatively long interactions. It includes current and historical data to provide a historical perspective of information. Its usage is read-intensive. It contains a few large tables. "Data Warehouse is a subject-oriented, integrated, and time-variant store of information in support of management's decisions." Subject Oriented Data warehouses are designed to help you analyze data. For example, to learn more about your company's sales data, you can build a warehouse that concentrates on sales. Using this warehouse, you can answer questions like "Who was our best customer for this item last year?" This ability to define a data warehouse by subject matter, sales in this case, makes the data warehouse subject oriented. Integrated Integration is closely related to subject orientation. Data warehouses must put data from disparate sources into a consistent format. They must resolve such problems as naming conflicts and inconsistencies among units of measure. When they achieve this, they are said to be integrated. Nonvolatile Nonvolatile means that, once entered into the warehouse, data should not change. This is logical because the purpose of a warehouse is to enable you to analyze what has occurred. Time Variant In order to discover trends in business, analysts need large amounts of data. This is very much in contrast to online transaction processing (OLTP) systems, where performance requirements demand that historical data be moved to an archive. A data warehouse's focus on change over time is what is meant by the term time variant. Subject - oriented A data warehouse target on the modeling and analysis of data for decision-makers. Therefore, data warehouses typically provide a concise and straightforward view around a particular subject, such as customer, product, or sales, instead of the global organization's ongoing operations. This is done by excluding data that are not useful concerning the subject and including all data needed by the users to understand the subject. Integrated A data warehouse integrates various heterogeneous data sources like RDBMS, flat files, and online transaction records. It requires performing data cleaning and integration during data warehousing to ensure consistency in naming conventions, attributes types, etc., among different data sources. Time variant Historical information is kept in a data warehouse. For example, one can retrieve files from 3 months, 6 months, 12 months, or even previous data from a data warehouse. These variations with a transactions system, where often only the most current file is kept. Non-Volatile The data warehouse is a physically separate data storage, which is transformed from the source operational RDBMS. The operational updates of data do not occur in the data warehouse, i.e., update, insert, and delete operations are not performed. It usually requires only two procedures in data accessing: Initial loading of data and access to data. Therefore, the DW does not require transaction processing, recovery, and concurrency capabilities, which allows for substantial speedup of data retrieval. Non-Volatile defines that once entered into the warehouse, and data should not change. Goals of Data Warehousing To help reporting as well as analysis Maintain the organization's historical information Be the foundation for decision making. Need for Data Warehouse 1) Business User: Business users require a data warehouse to view summarized data from the past. Since these people are non-technical, the data may be presented to them in an elementary form. 2) Store historical data: Data Warehouse is required to store the time variable data from the past. This input is made to be used for various purposes. 3) Make strategic decisions: Some strategies may be depending upon the data in the data warehouse. So, data warehouse contributes to making strategic decisions. 4) For data consistency and quality: Bringing the data from different sources at a commonplace, the user can effectively undertake to bring the uniformity and consistency in data. 5) High response time: Data warehouse has to be ready for somewhat unexpected loads and types of queries, which demands a significant degree of flexibility and quick response time. Benefits of Data Warehouse 1.Understand business trends and make better forecasting decisions. 2.Data Warehouses are designed to perform well enormous amounts of data. 3.The structure of data warehouses is more accessible for end-users to navigate, understand, and query. 4.Queries that would be complex in many normalized databases could be easier to build and maintain in data warehouses. 5.Data warehousing is an efficient method to manage demand for lots of information from lots of users. 6.Data warehousing provide the capabilities to analyze a large amount of historical data.