Sampling Distribution & Hypothesis Testing Lecture (PDF)
Document Details
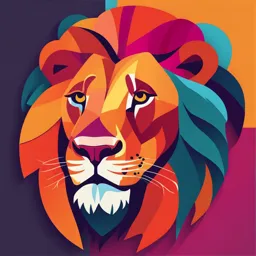
Uploaded by InvulnerableSense7127
Universiti Teknologi MARA
2022
Dr. Azwandi Ahmad
Tags
Summary
These lecture notes cover sampling distribution and hypothesis testing. The document details different types of sampling methods and their uses, as well as types of hypothesis. Includes definitions, examples, and practical applications in statistics.
Full Transcript
Lecture 3 ( 28 October 2022) Dr. Azwandi Ahmad 3pm 28/10/2022 DKF4 After completing this lecture, you should be able to: 1. Define sampling distribution 2. Explain each type of sampling distributions...
Lecture 3 ( 28 October 2022) Dr. Azwandi Ahmad 3pm 28/10/2022 DKF4 After completing this lecture, you should be able to: 1. Define sampling distribution 2. Explain each type of sampling distributions 3. Define each type of hypothesis 4. Explain the uses of hypothesis testing 5. Describe critical region and critical values 6. Type I and Type II error 7. Differentiate one-tailed and two-tailed tests Why we need statistics? Definition: ▪ A sampling distribution is a probability distribution of a statistic obtained through a large number of samples drawn from a specific population. Purpose of sampling: ▪ to collect data to answer a research question about a population With proper sampling methods: ▪ the sample results can provide “good” estimates of the population characteristics. A sample is a part of the population under study (n) It might not be possible to study an entire population We choose a subset of people/sample from a larger population This subset will represents the actual population in making an inference (n) If sample is too large If sample too small Good precision Inaccurate results Less error More source of bias Less bias. More power Power of the study comes down Wastage of time, money and Study fails to give meaningful resources information Resources could be as well be Waste of resources on an deviated to other project inaccurate study Not cost effective Ethical issue Statistically sig. but not Type II error practically sig. http://www.raosoft.com/samplesize.html Margin of error 5% or 0.05 Confidence level 95% Population size Bandar Puncak Alam 70,000 Sample size to be randomly selected is: 383 Type of sampling Probability sampling method/Random sampling Non-probability sampling method/Non-random sampling Probability sampling Non-probability sampling You can generalize to the You cannot generalize population define by beyond the sample sampling frame Allows use of statistics & Exploratory research, test hypotheses generate hypotheses Eliminate bias Adequacy of the sample Must have random can’t be known selection of units Cheaper, easier, quicker to carry out Sampling methods Probability Non-probability sampling sampling 1.Simple random 2.Systematic 5.Convinience 6.Judgement 3.Stratified 4.Cluster 7.Quota 8.Snowball Simple random sampling The purest form of probability sampling. Assures each element in the population has an equal chance of being included in the sample An initial starting point is selected by a random process, and then every nth number on the list is selected Subsamples are randomly drawn from samples within different strata that are more or less equal on some characteristic The primary sampling unit is not the individual element, but a large cluster of elements. Either the cluster is randomly selected or the elements within are randomly selected The sampling procedure used to obtain those units or people most conveniently available ▪ Speed and cost ▪ External validity? ▪ Internal validity ▪ Is it ever justified? Disadvantages ▪ Variability and bias cannot be measured or controlled ▪ Projecting data beyond sample not justified. The sampling procedure in which an experienced research selects the sample based on some appropriate characteristic of sample members to serve a purpose Disadvantages: ▪ Bias! ▪ Projecting data beyond sample not justified. The sampling procedure that ensure that a certain characteristic of a population sample will be represented to the exact extent that the investigator desires The sampling procedure in which the initial respondents are chosen by probability or non probability methods, and then additional respondents are obtained by information provided by the initial respondents Disadvantages ▪ Bias because sampling units not independent Hypothesis is a proposed explanation made on the basis of limited evidence as a starting point for further investigation TWO types of Hypothesis ❑Null Hypothesis (H0) ❑Alternative hypothesis (HA) or (H1) A null hypothesis is the general or default statement that nothing happened or changed (negative statement) or states that there is no relationship between the two variables or two groups (negative statement) Example 1: There is no relationship between the length of the job training program and the rate of job placement of trainees Example 2: Graduate assistant pay is not influenced by gender Example 3: Raloxifene has no effect to blood glucose level Example 4: Tomato plants do not exhibit a higher rate of growth when planted in compost rather than soil I a research, we aimed to disprove null hypothesis ❖Why need to disprove null hypothesis? ❖Or prove that null hypothesis is false ? It is easier to show that something is false once than to show that something is always true. It is easier to find disconfirming evidence against the null hypothesis than to find confirming evidence for the research hypothesis. The alternative hypothesis is contrary to the null hypothesis. The alternative hypothesis is what you might believe to be true or hope to prove true. Example 1: H0: There is no relationship between the length of the job training program and the rate of job placement of trainees HA: There is a relationship between the length of the job training program and the rate of job placement of trainees Example 2: H0 : Graduate assistant pay is not influenced by gender HA : Graduate assistant pay is influenced by gender Example 3: H0 : Raloxifene has no effect to body glucose level HA : Raloxifene has an effect body glucose level Example 4: H0:Tomato plants do not exhibit a higher rate of growth when planted in compost rather than soil. HA: Tomato plants do exhibit a higher rate of growth when planted in compost rather than soil. When we reject null hypothesis, we actually accept alternative hypothesis In research, we say “we reject null hypothesis” or “we do not reject null hypothesis” In research, we don’t say “we reject the alternative hypothesis” or “we do not reject the alternative hypothesis” How to decide whether we are rejecting null hypothesis or not rejecting null hypothesis in a research? We use “test of significance” Tests for statistical significance are used to address the question: what is the probability that what we think is a relationship between two variables is really just a chance? – we don’t like this in research (we want it to be less) We use “PROBABILITY” because we can never be completely 100% certain that a relationship exists between two variables. There are too many sources of error to be controlled, for example, sampling error, researcher bias, problems with reliability and validity, simple mistakes, etc. all is by chances And …….we are a human being (not perfect) So, tests for statistical significance is used to……… “determine whether we can reject the null hypothesis (H0) or not” By using probability theory and the normal curve, we can estimate the probability of being wrong (by chance), if we hypothesize that our finding is significant. If the probability of being wrong is small (5% or less), then we say that our observation of the relationship is a statistically significant finding Statistical significance means that there is a good chance (high probability, >95%) that we are right in finding that a relationship exists between two variables. Level of significant 5% or 0.05 Confidence level 95% Level of significant 1% or 0.01 Confidence level 99% Small? How small Good chance? How good “There must be a critical level that we are permitted to make an error by chances” How much error is acceptable? The level of statistical significance is often expressed as P-value (α). If the probability of difference occurred by chance is less than 5 %, ( 1/20), 0.05, then the null hypothesis is rejected (Alternative hypothesis is accepted) So, how much “chances of error” is acceptable? 0.05 or 5% P-value (α) is 0.05 However, lower chances is better. P- value (α) such as….. 0.01 or 1% …….depending on the type of research Remember source of error we discuss before: “sampling error, researcher bias, problems with reliability and validity, simple mistakes.etc” All of these should be less than 5% to reject the null hypothesis A researcher want to study the effect of biphosphonates drug to bone mass among elderly people in Puncak Alam. Bone mass 100 peoples were measured before and after taking biphosphonates. Calculated P-value in the study was 0.03. P-value (α) set is 0.05. Determine the null and alternative hypothesis. Can we reject the null hypothesis? H0: bisphosphonates has no effect to bone mass among elderly people in Puncak Alam HA: bisphosphonates has an effect to bone mass among elderly people in Puncak Alam Yes!, we can reject null hypothesis because calculated P-value is 0.03, less than α=0.05 ( pre-determined P-value (α) Reject Null hypothesis Do not reject Null hypothesis Critical value-separates the critical region from the non-critical region. Critical region- is the range of values of the test value that indicates that there is a significant difference and that the null hypothesis should be rejected. Non critical region Critical value Critical value Critical region Critical region Critical values for a test of hypothesis depend upon: 1) a test statistics, which is specific to the type of test 2) P-value (α), which defines the sensitivity of the test. Test statistics is compared to critical value. For positive side, if test statistics at the right of critical value we can reject the null hypothesis For negative side, if test statistics at the left of critical value we can reject the null hypothesis Non critical region Critical value Critical value Test statistics Critical value Calculated from samples by Obtained from distribution using a specific formulae table Based on t-test formulae, Based on t-table, z-table, F- F-test formulae, Chi-Square table, Chi-square table, etc. formulae Test statistics Critical value Test statistics > Critical Rejecting null value (Positive side) hypothesis Test statistics < Critical value (Negative side) Test statistics ≤ Critical Do not rejecting null value (Positive side) hypothesis Test statistics ≥ Critical value (Negative side) Type I Type II error error When the null hypothesis is true and you reject it, you commited a Type I error. When the null hypothesis is false and you fail to reject it, you commited a Type II error. Type I error is also known as “false positive” Type II error is also known as “false negative” The probability of making a Type I error is α (the P-value) which is the level of significance you set for your hypothesis test. An α of 0.05 indicates that you are willing to accept a 5% chance that you are wrong when you reject the null hypothesis. When the null hypothesis is false and you fail to reject it, you make a Type II error. The probability of making a type II error is β. Decision True null hypothesis False null hypothesis Fail to reject Correct decision Wrong decision (probability = 1-α) Commit Type II error because fail to reject the null when it is false (probability = β) Reject Wrong decision Correct decision Commit Type I error (probability = 1-β) because rejecting the null when it is true (probability = α) Statistical tests can be THREE (3) different types:- ❑ right-tailed test One tailed ❑ left-tailed test ❑ two-tailed test A statistical test in which the critical area of a distribution is one-sided so that it is either greater than or less than a certain value, but not both. If the sample that is being tested falls into the one-sided critical area, the null hypothesis will rejected So it has only one critical region Specific direction (left or right) ❑ A right tailed test is where your hypothesis statement contains a greater than (>) symbol. ❑ Resulting in a critical value on RIGHT tail. Thus, the decision rule for this as follows: Reject H0 if the test statistics > critical value OTHER WISE do not reject H0. Example:- Is the question referring to the test statistic having a significant increase from the expected? If so, this is a right tail question. 18 MAC 2016 18 MAC 2016 For example, you want to compare the half- life of a drug. If you want to know if the half-life of drug A is longer than expected. (let’s say 9 days), your hypothesis statements might be:- H0 = Half-life of drug A is 9 days ( μ = 9) HA = Half-life of drug A more than 9 days (μ > 9 days) 18 MAC 2016 If the alternative hypothesis has stated that the effect was expected to be negative, this is also a one-tailed hypothesis but left-tailed test. A left-tailed test is where your hypothesis statement contains a less than (