Unit 2.pdf
Document Details
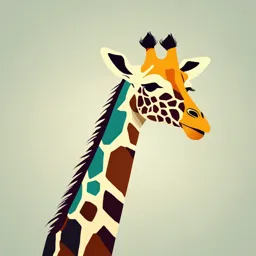
Uploaded by LuckyColumbus3355
Tags
Full Transcript
Unit 2.1 - Basics of Data Literacy Lesson Title: Basics of Data Literacy Approach: Session + Activity Summary: In this module, students are familiarized with the concept of Data Literacy. Further, they would be able to recognize the different categories of data and will be i...
Unit 2.1 - Basics of Data Literacy Lesson Title: Basics of Data Literacy Approach: Session + Activity Summary: In this module, students are familiarized with the concept of Data Literacy. Further, they would be able to recognize the different categories of data and will be introduced to the culture of data literacy. Learning Objectives Define data literacy and explain its importance with a real-world example Relate to the impact created by data literacy in everyday life Develop awareness about personal data, data privacy, and data security Learning Outcomes Define data literacy and recognize its importance Understand how data literacy enables informed decision-making and critical thinking Apply the Data Literacy Process Framework to analyze and interpret data effectively Differentiate between data privacy and security Identify potential risks associated with data breaches and unauthorized access. Learn measures to protect data privacy and enhance data security Pre-requisites: Basic knowledge of AI and data Key-concepts Understanding of data literacy Identify the difference between Quantitative (Numerical) and Qualitative (Categorical) Data Impact of data literacy with the help of case studies and scenarios Best practices for Cyber Security 2.1.1 Introduction to Data Literacy Data literacy means knowing how to understand, work with, and talks about data. It's about being able to collect, analyze, and show data in ways that make sense. Reference Video: https://www.youtube.com/watch?v=yhO_t-c3yJY Data Literacy Ability to read, comprehend, Raw Facts or Information and communicate in a language Data literacy is the ability to understand, interpret and communicate with data. Data Pyramid is made of different stages of working with data Let us understand different parts of Data pyramid Moving up from the bottom Data is available in a raw form. Data in this form is not very useful. Data is processed to give us information about the world. Information about the world leads to knowledge of how things are happening. Wisdom allows us to understand why things are happening in a particular way. Let’s understand Data Pyramid with a simple Traffic Light example: Rahul rated the 3 films he watched consecutively as bad, best and average respectively" Can you filter the data from this statement? Are they of the same type? _____________________________________________________________________________________ _____________________________________________________________________________________ _____________________________________________________________________________________ _________________________________________________________ 2.1.2 Impact of Data Literacy Activity: Impact of News Articles (Select any trending news) Session Preparation Logistics: For a class of 40 Students [Pair Activity] Materials Required: ITEM QUANTITY Online Data Sources Clues NA Computers 20 Purpose: The purpose of this activity is to engage participants in various scenarios that involve collecting data and analyzing its sources. Emphasizing the importance of validating data sources, the aim is to instill the concept of data literacy. By understanding how authentic data sources contribute to reliable and unbiased decision- making, participants will develop critical skills for navigating and interpreting data effectively. Brief: [Pair Activity] Participants will search the internet for data sources, extracting key information to support their decisions. How was the situation Key figures in the Author of the Source Weblink to the Source described by the Source source You have to rank the sources of the news articles from most accurate to least, state reasons for your choice. Rank Data Source Remarks So, we can conclude that every data tells a story, but we must be careful before believing the story Data literacy is essential because it enables individuals to make informed decisions, think critically, solve problems, and innovate. 2.1.3 How to become Data Literate? Every data tells a story, but we must be careful before believing the story. Data Literate is a person who can interact with data to understand the world around them. Let’s understand it with following example: Scenario: Buying a Video game online Data literacy helps people research about products while shopping over the internet How do you decide the following things when we are shopping online? Which is the cheapest product available? Which product is liked by the users the most? Does a particular product meet all the requirements? A data literate person can – Filter the category as per the requirement – If the budget is low, select the price filter as low to high Check the user ratings of the products Check for specific requirements in the product Data Literacy Process Framework The data literacy framework provides guidance on using data efficiently and with all levels of awareness. Data literacy framework is an iterative process. 2.1.4 What are Data Security and Privacy? How are they related to AI? Data Privacy and Data Security are often used interchangeably but they are different from each other. What is Data Privacy? Data privacy referred to as information privacy is concerned with the proper handling of sensitive data including personal data and other confidential data, such as certain financial data and intellectual property data, to meet regulatory requirements as well as protecting the confidentiality and immutability of the data. Here are examples of two things which may compromise our data privacy Downloaded an unverified mobile Accepted the Terms of Service application without reading Why is it important? A data breach at a government A breach at a corporation can A breach at a hospital can put The following besttoppractices agency can put secret can help you ensure data privacy: put proprietary data in the personal health information in the information in the hands of an hands of a competitor. hands of those who might misuse enemy state. it. Understanding what data, you have collected, how it is handled, and where it is stored. Necessary data required for a project should only be collected. User consent while data collection must be of utmost importance. What is Data Security? Data security is the practice of protecting digital information from unauthorized access, corruption, or theft throughout its entire lifecycle. Why is it important? Due to the rising amount of data in the cloud there is an increased risk of cyber threats. The most appropriate step for such an amount of traffic being generated is how we control and protect the transfer of sensitive or personal information at every known place. The most possible reasons why data security is more important now are: Cyber-attacks affect all the people The fast-technological changes will boom cyber attacks 2.1.5 Best Practices for Cyber Security Cyber security involves protecting computers, servers, mobile devices, electronic systems, networks, and data from harmful attacks. Reference Links: Video: https://www.youtube.com/watch?v=aO858HyFbKI CBSE Manual on Cyber Security: https://www.cbse.gov.in/cbsenew/documents/Cyber%20Safety.pdf Do’s Use strong, unique passwords with a mix of characters for each account. Activate Two-Factor Authentication (2FA) for added security. Download software from trusted sources and scan files before opening. Prioritize websites with "https://" for secure logins. Keep your browser, OS, and antivirus updated regularly. Adjust social media privacy settings for limited visibility to close contacts. Always lock your screen when away. Connect only with trusted individuals online. Use secure Wi-Fi networks. Report online bullying to a trusted adult immediately. Don’t ‘s Avoid sharing personal info like real name or phone number. Don't send pictures to strangers or post them on social media. Don't open emails or attachments from unknown sources. Ignore suspicious requests for personal info like bank account details. Keep passwords and security questions private. Don't copy copyrighted software without permission. Avoid cyberbullying or using offensive language online. Revision Time: 1. Cultivating Data Literacy means: a) Utilize vocabulary and analytical skills b) Acquire, develop, and improve data literacy skills c) Develop skills in statistical methodologies d) Develop skills in Math 2. Data Privacy and Data Security are often used interchangeably but they are different from each other a) True b) False 3. The_____________________ provides guidance on using data efficiently and with all levels of awareness. a) data security framework b) data literacy framework c) data privacy framework d) data acquisition framework 4. _____________ allows us to understand why things are happening in a particular way a) data b) information c) knowledge d) wisdom 5.__________ is the practice of protecting digital information from unauthorized access, corruption, or theft throughout its entire lifecycle. a) data security b) data literacy c) data privacy d) data acquisition 2.2 Acquiring Data, Processing, and Interpreting Data Lesson Title: Acquiring Data, Processing, and Interpreting Data Approach: Session + Activity Summary: You will get an understanding of data processing, data interpretation and keywords related to data. Learning Objectives Familiarizing youth with different data terminologies like data acquisition, processing, analysis, presentation, and interpretation Discussing different methods of data interpretation like qualitative and quantitative. Understanding the methods and different collection techniques Critically think about their advantages and disadvantages Identifying various data presentation methods with examples and interpreting them Gain awareness about the advantages and impact of Data interpretation on business growth Learning Outcomes Determine the best methods to acquire data. Classify different types of data and enlist different methodologies to acquire it. Define and describe data interpretation. Enlist and explain the different methods of data interpretation. Recognize the types of data interpretation. Realize the importance of data interpretation Pre-requisites: Acquaintance with data and its different types. Key-concepts Familiarizing with different data terminologies like data processing, analysis, presentation, and interpretation Quantitative and Qualitative Data Interpretation Types of Data Interpretation – Textual, Tabular and Graphical with examples. Activity Session Preparation Logistics: For a class of 40 Students [Pair Activity] Materials Required: ITEM QUANTITY Online Data Sources Clues NA Computers 20 Purpose: The purpose of this activity is to engage participants in acquiring data from online sources. The ability to locate and access relevant data sources is crucial for AI Projects. Brief: [Pair Activity] Participants will be locating an online dataset suitable for training an AI model. They will conduct a search for weather forecast related datasets on various online platforms and then paste images or screenshots of the datasets found. 2.2.1. Types of data Artificial Intelligence is crucial, with data serving as its foundation. We come across different types of information every day. Some common types of data include: Textual Data (Qualitative Data) Numeric Data (Quantitative Data) It is made up of words and phrases It is made up of numbers It is used for Natural Language Processing (NLP) It is used for Statistical Data Search queries on the internet are an example Any measurements, readings, or values of textual data would count as numeric data Example: “Which is a good park nearby?” Example: Cricket Score, Restaurant Bill Numeric Data is further classified as: Continuous data is numeric data that is continuous. E.g., height, weight, temperature, voltage Discrete data is numeric data that contains only whole numbers and cannot be fractional E.g. the number of students in the class – it can only be a whole number, not in decimals Types of Data used in three domains of AI: 2.2.2 Data Acquisition/Acquiring Data Data Acquisition, also known as acquiring data, refers to the procedure of gathering data. This involves searching for datasets suitable for training AI models. The process typically comprises three key steps: Acquiring Data – Sample Data Discovery Let’s say we want to collect data for making a CV model for a self-driving car We will require pictures of roads and the objects on roads We can search and download this data from the internet This process is called data discovery Acquiring Data – Sample Data Augmentation Data augmentation means increasing the amount of data by adding copies of existing data with small changes The image given here does not change, but we get data on the image by changing different parameters like color and brightness New data is added by slightly changing the existing data Acquiring Data – Sample Data Generation Data generation refers to generating or recording data using sensors Recording temperature readings of a building is an example of data generation Recorded data is stored in a computer in a suitable form Sources of Data Various Sources for Acquiring Data: Primary Data Sources — Some of the sources for primary data include surveys, interviews, experiments, etc. The data generated from the experiment is an example of primary data. Here is an excel sheet showing the data collected for students of a class. Secondary Data Sources—Secondary data collection obtains information from external sources, rather than generating it personally. Some sources for secondary data collection include: 2.2.3 Best Practices for Acquiring Data Checklist of factors that make data good or bad Data acquisition from websites Ethical concerns in data acquisition While gathering data and choosing datasets, certain ethical issues can be addressed before they occur 2.2.4 Features of Data and Data Preprocessing Usability of Data There are three primary factors determining the usability of data: 1. Structure- Defines how data is stored. 2. Cleanliness- Clean data is free from duplicates, missing values, outliers, and other anomalies that may affect its reliability and usefulness for analysis. In this particular example, duplicate values are removed after cleaning the data. 3. Accuracy- Accuracy indicates how well the data matches real-world values, ensuring reliability. Accurate data closely reflects actual values without errors, enhancing the quality and trustworthiness of the dataset. In this particular example, we are comparing data gathered from measuring the length of a small box in centimeters. Kaggle assigns a usability score to the data sets that are present on the website based on scores given by the users of that data. What kind of data is more usable, according to you? _____________________________________________________________________________________ _____________________________________________________________________________________ _____________________________________________________________________________________ _____________________________________________________________ If we have a lot of data which is not clean, is it good for AI? _____________________________________________________________________________________ _____________________________________________________________________________________ _____________________________________________________________________________________ _____________________________________________________________ Features of Data Data features are the characteristics or properties of the data. They describe each piece of information in a dataset. For example, in a table of student records, features could include things like the student's name, age, or grade. In a photo dataset, features might be the colors present in each image. These features help us understand and analyze the data. In AI models, we need two types of features: independent and dependent. Independent features are the input to the model—they're the information we provide to make predictions. Dependent features, on the other hand, are the outputs or results of the model—they're what we're trying to predict. 2.2.5 Data Processing and Data Interpretation Data processing and interpretation have become very important in today’s world Can you answer this? ▪ Niki has 7 candies, and Ruchi has 4 candies ▪ How many candies do Niki and Ruchi have in total? ▪ We can answer this question using data processing ▪ Who should get more candies so that both Niki and Ruchi have an equal number of candies? ▪ How many candies should they get? ▪ We can answer this question using data interpretation Data Processing ▪ Data processing helps computers understand raw data. ▪ Use of computers to perform different operations on data is included under data processing. Data Interpretation ▪ It is the process of making sense out of data that has been processed. ▪ The interpretation of data helps us answer critical questions using data. Understanding some keywords related to Data More than 60% of Students would be Acquire Data- Acquiring data is to collect data from various data interested in Sports! sources. Data Processing- After raw data is collected, data is processed to derive meaningful information from it. Data Analysis – Data analysis is to examine each component of the data in order to draw conclusions. Data Interpretation – It is to be able to explain what these findings/conclusions mean in a given context. Data Presentation- In this step, you select, organize, and group ideas and evidence in a logical way. Acquire Process Analyze Interpret Present Methods of Data Interpretation How to interpret Data? Based on the two types of data, there are two ways to interpret data- Quantitative Data Interpretation Qualitative Data Interpretation Qualitative Data Interpretation Qualitative data tells us about the emotions and feelings of people Qualitative data interpretation is focused on insights and motivations of people Reviews by customers – Pizza Qualitative data Jim and his toppings friends are regular customers here are so Veg Veg farmhouse tasty! farmhouse pizza is a popular choice pizza is the best here! Data Collection Methods – Qualitative Data Interpretation Record keeping: This method uses existing reliable documents and other similar sources of information as the data source. It is similar to going to a library. Observation: In this method, the participant – their behavior and emotions – are observed carefully Case Studies: In this method, data is collected from case studies. Focus groups: In this method, data is collected from a group discussion on relevant topic. Longitudinal Studies: This data collection method is performed on the same data source repeatedly over an extended period. One-to-One Interviews: In this method, data is collected using a one-to-one interview. Activity – Trend Analysis Purpose: ▪ This activity will engage youth with longitudinal studies – a study conducted over a considerable amount of time to identify trends and patterns ▪ The ability to identify trends and patterns in datasets allows us to make informed decisions about different tasks in our lives Activity Guidelines Let’s do a small activity based on Identifying trends. Visit the link: https://trends.google.com/trends/?geo=IN (Google Trends) Explore the website Check what is trending in the year 2022 – Global ▪ Make a list of trending sports (top 5) ▪ Make a list of trending movies (top 5) Check what is trending globally in the year 2022 List of trending athletes (top 5) List of trending movies (top 5) 5 Steps to Qualitative Data Analysis 1. Collect Data 2. Organize 3. Set a code to the Data Collected 4. Analyze your data 5. Reporting Quantitative Data Interpretation Counter – Number of Cumulative Grade Point website visit Average (CGPA) Cumulative Grade Point Average (CGPA) The average height Recording the of students will be height of students important to build in a class suitable tables and chairs for students ▪ Quantitative data interpretation is made on numerical data ▪ It helps us answer questions like “when,” “how many,” and “how often” ▪ For example – (how many) numbers of likes on the Instagram post Data Collection Methods -Quantitative Data Interpretation Interviews: Quantitative interviews play a key role in collecting information. Polls: A poll is a type of survey that asks simple questions to respondents. Polls are usually limited to one question. Observations: Quantitative data can be collected through observations in a particular time period Longitudinal Studies: A type of study conducted over a long time Survey: Surveys can be conducted for a large number of people to collect quantitative data. 4 Steps to Quantitative Data Analysis 1. Relate measurement scales with variables 2. Connect descriptive statistics with data 3. Decide a measurement scale 4. Represent data in an appropriate format Let’s summarize Qualitative and Quantitative data interpretation Qualitative & Quantitative Data Interpretation Qualitative Data Interpretation Quantitative Data Interpretation Categorical Numerical Provides insights into feelings and Provides insights into quantity emotions Answers how and why Answers when, how many or how often Methods – Interviews, Focus Groups Methods – Assessment, Tests, Polls, Surveys Example question – Why do students like Example question – How many students attending online classes? like attending online classes? Types of Data Interpretation There are three ways in which data can be presented: Data Interpretation Types Textual Tabular Graphical Textual DI ▪ The data is mentioned in the text form, usually in a paragraph. ▪ Used when the data is not large and can be easily comprehended by reading. ▪ Textual presentation is not suitable for large data. ▪ Example: In the Science Olympiad class of 45 Students, 3 students obtained the More than 60% of perfect score of 50. 10 students got a score of 45 and above, 15 students scored more students got a score of 40 and above, 8 students got a score of 30 and than 80% Marks in above, 6 students got a score of 20 and above and 3 got 19 and below. Olympiad! Tabular DI ▪ Data is represented systematically in the form of rows and columns. ▪ Title of the Table (Item of Expenditure) contains the description of the table content. ▪ Column Headings (Year; Salary; Fuel and Transport; Bonus; Interest on Loans; Taxes) contains the description of information contained in columns. Graphical DI Bar Graphs In a Bar Graph, data is represented using vertical and horizontal bars. Pie Charts ▪ Pie Charts have the shape of a pie and each slice of the pie represents the portion of the entire pie allocated to each category ▪ It is a circular chart divided into various sections (think of a cake cut into slices) ▪ Each section of the pie chart is proportional to the corresponding value Distribution of Math Score Perfect Score(=50) 7%7% 45 and Above(>=45) 13% 22% 18% 40 and Above(>=40) 33% Between 30-39 Between 20-29 19 and Below(