IMG_3914.jpeg
Document Details
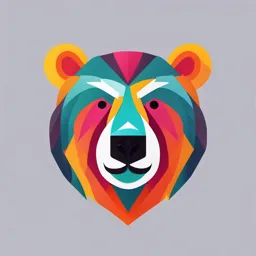
Uploaded by KindlyAltoFlute
Full Transcript
# Instrument Description This document describes various aspects of statistical methods, particularly Null Hypothesis Significance Testing (NHST). ## Parameters Parameters are estimated from data rather than measured. They are usually constants representing truths about relationships between var...
# Instrument Description This document describes various aspects of statistical methods, particularly Null Hypothesis Significance Testing (NHST). ## Parameters Parameters are estimated from data rather than measured. They are usually constants representing truths about relationships between variables. Examples include the mean and median; correlations and regression coefficients. ## Outcomes * Outcome = (b) + error; Represents outcomes predicted by one variable * Outcome = (bX₁ + b₂X₂) + error; Predicts outcomes from multiple variables (X₁, X₂). ## Hypothesis Testing ### Null Hypothesis (H₀) The null hypothesis states that your prediction is false (the predicted effect does not exist). It's denoted by H₀. ### Alternative Hypothesis (H₁) The alternative hypothesis proposes that your prediction is correct (the effect exists). It is denoted by H₁. For example, if you're presenting, the alternative hypothesis would state that anxiety differs from the control. ### One-tailed vs. Two-tailed Hypotheses * **Directional (one-tailed):** States the effect's direction (e.g., anxiety will increase). Results opposite to the predicted direction should be ignored in a one-tailed test, and a different significance level would apply if the opposite direction was measured. Failing to accept the null hypothesis when measuring the reverse outcome would erroneously support your alternative hypothesis. * **Non-directional (two-tailed):** Doesn't specify the direction of the effect (e.g., anxiety will change). ### Type I and Type II Errors * **Type I error:** Concluding there's an effect when there isn't (false positive). A-level is the probability of making this error (usually 0.05). * **Type II error:** Concluding there's no effect when there is (false negative). B-level is the probability of making this error (often 0.20 or 20%). ## Statistical Concepts * **p-value:** The probability of observing the data if the null hypothesis is true. Fisher advocated for a low p-value (e.g., 0.01 or less) to suggest strong evidence for a hypothesis, but the arbitrary value 0.05 is often used. * **Effect size:** Quantifies the magnitude of an observed effect. Common measures include Cohen's d, Glass' g, and Pearson's correlation coefficient (r). * **Power:** The probability of detecting an effect if it truly exists. Higher power is desired. * **Meta-analysis:** Combines results from multiple studies to produce a more accurate estimate of the true effect size. ## Tests * **t-tests:** Compare the means of two groups. * **Independent-samples t-test:** Used when different participants are in different conditions. * **Paired-samples t-test:** Used when the same participants are in both conditions. ## Fisher's Main Point Fisher focused on calculating and evaluating the probability of an event in context. He argued that a low p-value (e.g., 0.01) strongly supports a hypothesis. ## Problems with NHST * **Small/unimportant effects:** May appear statistically significant but not practically important. * **Sample size:** Small samples may miss important effects.