Build on Tiny Bets: AI Investigation Recommendations - PDF
Document Details
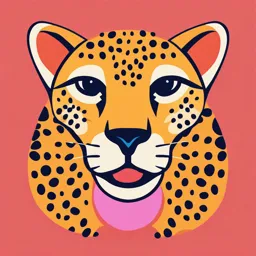
Uploaded by StaunchSurrealism
2025
Tags
Summary
This document, a presentation from Productside, provides recommendations for investigating and implementing AI strategies. It emphasizes the importance of starting small with "tiny bets" to achieve bigger wins, and includes advice such as staying calm and focusing on problem-solving. The content is designed for a professional audience, offering insights into product development and business strategy in the age of AI.
Full Transcript
The Toasted Bread Challenge for Homework © 2025 PRODUCTSIDE 2 AI Investigation Recommendations Agenda Conducting Your Own AI Investigation Stay Calm...
The Toasted Bread Challenge for Homework © 2025 PRODUCTSIDE 2 AI Investigation Recommendations Agenda Conducting Your Own AI Investigation Stay Calm Feel their Stake Out Stand on Unpack Build on Socialize and Focus Pains, an AI the Assumptio Tiny Bets an on the Learn their Playing Shoulders ns & for Big Outcomes Problem Gains Field of Giants Unknowns Wins Blueprint Space © 2025 Productside 3 Bets? Why Bets Bets have a payout - there's an "By treating decisions as expectation of meaningful progress explicit bets, product managers by the end of the cycle. can better avoid emotional Bets are commitments - teams get traps and find key uninterrupted time to work on the opportunities to learn and seek selected project. the truth." - Annie Duke, Professional Bets have a cap on downside - the Speaker & Decision Strategist maximum loss is the time allocated (e.g., 6 weeks). © 2025 Productside 4 What % of new products & feature ideas fail? © 2025 Productside 5 Solution Failure Rates +20% have a big impact “The most expensive way to test ~60% have little or no lift your idea is to build production quality software.” Jeff Patton, Author of ‘the User Story Map’ -20% hurt the business Sources: ’User Story Mapping: Discover the Whole Story, Build the Right Product’ ~ Jeff Patton ‘Trustworthy A/B Tests: Pitfalls in Online Controlled Experiments’ ~ Ronny Kohavi ‘Modernization - Clearing a Pathway to Success’ ~ Standish Group © 2025 Productside 6 Small AI Bets Big AI Bets Low upfront investment High upfront investment Limited scope Broad scope Quick to prototype Longer development cycle Low impact if it fails High impact if it fails Easy to scale Complex to scale © 2025 PRODUCTSIDE, 7 LLC AI ‘Haul’ of 1. Anki – Overbuilt without validating the market demand for consumer robots. Lost: Nearly $200 million in funding. Shame: 2. Jibo – Repeated delays and overpromises on the product’s functionality eroded These examples showcase failures market confidence. Hype without a Cause due to overhyping product Lost: Nearly $73 million over 6 years. capabilities, leading to unmet customer expectations and a failed 3. IBM Watson for Oncology – market fit. Overpromised advanced AI decision-but couldn’t overcome data, regulatory, & customer adoption hurdles. Lost: Over $4 billion over approximately 6 years. © 2025 Productside 8 Start with a small Process Docs Extract data from documents bet Begin by asking … Process Extract info from Images images Do we …” have the right data? What Problem HIGH LEVEL use CONSIDERATIONS Are We Process Derive Insights from a Prebuilt or Custom model? Solving Text unstructured text use a Private or Public model? & for Who? leverage existing APIs? Act on Make forecasts & Stay Flexible in a Dynamic Tabular predictions from Data structured data Environment Let your Dev & Data Teams lead! Create text, Build images, etc. Gen AI based on large amounts of data © 2025 PRODUCTSIDE, LLC 9 1. Rethink Robotics – Assumed robots AI ‘Haul’ of could meet high precision demands, but performance fell short. Lost: $149.5 million over 10 years. Shame: 2. Walmart's Bossa Nova Robotics – Overestimated robots' ability to match These companies faced issues with human workers in efficiency. The Tech that Couldn’t their AI technology not meeting Lost: ~$65M & 5 years invested, with expected performance, leading to 500 robots deployed before shutting failure due to technical limitations. down. 3. Lowe’s LoweBot – Underdelivered on AI’s customer service potential, with low adoption. Lost: ~30M & 2 years invested before discontinuing the project. © 2025 Productside 10 Fight to Keep the Scope of POCs TINY The Deployment Type Matters The Ask Scope the Set the Execute Evaluate Scale pilot: foundation the pilot: and responsibl : expand: y: 1.Pick the 1.Problem 1.Run a 1.Quantify 1.Prioritize Outcome focus Simulatio ROI Data 2.Pick the 2.Get n 2.Socialize 2.Land & Data Relevant 2.Run a Outcomes Expand Data Limited Trial © 2025 PRODUCTSIDE, 11 LLC 1. Utrip – Overbuilt a travel AI solution AI ‘Haul’ of without a sustainable revenue model. Lost: At least $4 million over 7 years. 2. Aria Insights (formerly CyPhy Works) Shame: – Pivoted too late, struggled to scale and compete in the drone industry. These examples show failure due to Lost: $39 million in funding over 11 Big Bets, No Payout poor business models and years. overbuilding without a clear path to profitability or scalability. 3. Bloomberg's LLMs – Overinvested in a specialized AI model that failed to scale or keep pace with broader innovations (specifically GPT—4o). Lost: 1.3 million GPU hours of compute power spent on a 50 billion parameter model © 2025 Productside 12 What about AI Generated Media Content … … like Storyboards? © 2025 PRODUCTSIDE 13 Framing your Bet as a Hypothesis Understand the advantages of validating with metrics UNIT 01 | L E SS O N 0 3 © 2025 PRODUCTSIDE, 14 LLC How might we shape and measure our solution ? © 2025 Productside 15 Validating BUILD — MEASURE — LEARN — Value REPEAT Hypothesis The purpose of product LEARN BUILD discovery is to quickly separate the good ideas from the bad. The output of product discovery is a validated Data Product product backlog. Marty Cagan, Author of ‘Inspired’ MEASURE © 2025 Productside 16 Get the right Ask engineers & DevOps about scope, effort, and people in the technical impact. Use simple metaphors to translate technical room complexities to stakeholders. Clarify expectations on timelines, costs, and resources. Base discussions on informed, validated assumptions. Collaborate with finance to define budgetary amounts. Link past efforts to predict future success. © 2025 PRODUCTSIDE, 17 LLC The Good, The Bad, The Risks & Validated Assumptions Buffer: Fielded a landing page to validate demand before building Goal: the tool. Minimize risk by validating Webvan: Based on untested assumptions early. growth assumptions, they Choose discovery strategies overbuilt infrastructure. that allow learning without heavy commitment. Amazon: Used A/B testing to minimize the impact of failed features. © 2025 PRODUCTSIDE, 18 LLC Discovery Strategy: The Solution Hypothesis 1. If/Then Hypothesis: Proposes a solution and predicts its impact on the target persona. 2. Validation Experiments: Details TAD experiments to validate the hypothesis. 3. Validation Measures: Set success metrics and a deadline to assess the hypothesis. © 2025 Productside 19 AI & Tiny Acts of Discovery (TADs) AI TADs for Viability AI TADs for Desirability (should we do this?) (do people want this?) Interviews: Conduct short discussions with potential AI User Journeys: Generate user scenarios with AI. users. Guerrilla Interviews: Conduct quick, informal user Synthetic Data: Explore simulated market behaviors. talks. Data Mining: Examine data for feature support and Social Listening: Use AI for social media sentiment traits. analysis. Market Sizing: Use CoPilot to estimate market size. Data Analysis: Analyze data for user behavior patterns. Monte Carlo Test: Demonstrate likely outcomes to potential customers running a simple simulation. Observation Sessions: Perform short observations of user settings. © 2025 Productside 20 Success Metrics Success Metric Characteristics Sample Success Metrics 1. Quick to Assess: Speedy evaluation for swift 1. Demo Sign-ups: 30 interests in 24 hours. decisions. 2. Quick Feedback: 80% feedback in two days. 2. Minimal Resources: Requires few resources to test. 3. Early Use: 50% engage with prototype in one day. 3. Clear Direction: Guides next steps: proceed, pivot, or stop. 4. Willing Users: 60% positive on adoption in three days. 4. User-Focused: Centers on user feedback and engagement. 5. Usability Insights: 70% insights from first 48-hour use. 5. Early Proof: Provides initial proof of concept viability. © 2025 Productside 21 ACTIVITY Solution Craft a solution hypothesis linking user Hypothesis GOAL insights to a proposed solution in an "If... then..." format. Craft a solution hypothesis statement based on Plan a concise experiment to test this validated assumptions and targeted outcomes hypothesis, like a prototype or user to align development with measurable interview. business and user value. Identify clear metrics to evaluate the test, TOOLS setting specific targets to validate your Mural hypothesis. TIME 20 minutes © 2025 Productside 22 Validating Through Lo-fi Experimentation Get Valuable Feedback Fast & Cheap © 2025 Productside 23 ”… one of the most time consuming and expensive ways to learn is to build potentially shippable software. ” Jeff Patton Source: Dual Track Development is not Duel Track © 2025 Productside 24 Consider the Cost of Delivery … Cost Factor Machine Learning (ML) Large Language Models (LLMs) Data Acquisition $10,500 - $70,000 for 100K samples $100,000+ for larger datasets Data Annotation Up to $70,000 for 100K samples Included in data acquisition costs Infrastructure Costs $100 - $30K/month (complex projects) $200K+ per month for large-scale deployments Compute Resources Varies significantly by model complexity $300,000 for 64 GPUs for 30 days Model Development Varies significantly depending on scope Millions of dollars for large models Model Training $10K+ for extensive model training $500K - $4.6M for training GPT-3 Fine-tuning Varies by project, typically lower than LLMs $10K - $100K depending on the model Personnel Costs Varies significantly based on team size $500K for a team of 5 over 6 months Total Estimated Cost Wide range, depends on project scale and complexity $1.2M - $4.6M+ for large models like GPT-3 © 2025 Productside 25 Consider the Cost of Discovery … How do we Try to make validate the Right your discoveries Thing to Build here! without having to Build it? © 2025 Productside 26 Lo-fi Experimentati on Techniques Concierge test Wireframes “Wizard of Oz” Explainer Videos ON!TRACK Landing Pages Crowdfunding ASSET Paper Models Storyboards MANAGEMENT An asset-tracking solution designed for construction Images courtesy of: https://hstk.com/prototype-examples-for-your-application/ professionals https://www.ponoko.com/blog/how-to-make/how-to-make-a-product-prototype/ https://www.hilti.co.za/content/hilti/META/ZA/en/business/business/equipment/on-track.html LEARN MORE https://www.ipcm.it/en/article/why-and-how-are-3d-printed-parts-coated.aspx https://www.sciencebuddies.org/science-fair-projects/engineering-design-process/storyboards www.hilti.com © 2025 Productside 27 Storyboarding Think of your solution like a movie. Aim for: Authenticity Simplicity Emotion Your user is the hero of the story! https://www.uxbooth.com/articles/how-to-use-storyboards-for-product-development/ © 2025 Productside 28 ACTIVITY Storyboarding Get creative! Use images, drawings, and GOAL text to tell a story. Illustrate your solution with a storyboard TOOLS Whiteboarding tool Be ready to return and share your findings with the group. TIME 20 minutes © 2025 Productside 29