RESM2001 (2024-25) Lecture 2.1 - From Sample To Population PDF
Document Details
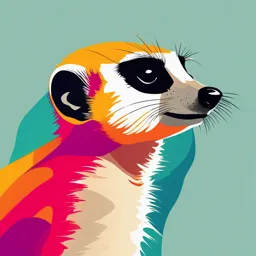
Uploaded by UnbeatableSphinx3591
University of Southampton
Tags
Summary
This lecture provides a concise introduction to Social Data Analytics. It outlines the basic concepts of descriptive and inferential statistics, along with exploring probability sampling, sampling distributions, and the application of the Central Limit Theorem (CLT).
Full Transcript
RESM2001: Social Data Analytics Lecture 2.1: From Sample to Population Lecture Outline Descriptive versus inferential statistics Sample and population Basic idea of statistical inference Probability sampling Sampling distributions Properties of sampling distributions –...
RESM2001: Social Data Analytics Lecture 2.1: From Sample to Population Lecture Outline Descriptive versus inferential statistics Sample and population Basic idea of statistical inference Probability sampling Sampling distributions Properties of sampling distributions – The Central Limit Theorem (CLT) Standard errors RESM2001 - Lecture 2.1 2 Descriptive versus Inferential Statistics Two main branches of statistics Descriptive Inferential statistics statistics Describe, organise, Use probabilistic summarise, display data techniques to analyse a sample to tell us something about a population RESM2001 - Lecture 2.1 3 Confidence Hypothesis Testing Inferential Intervals Sampling and CLT Normal Distribution Z-scores Correlation Shapes of Measures of Inequality Descriptive Distributions Spread Percentages and Graphical Methods Averages Percentiles Tables Data Types of Variable RESM2001 - Lecture 2.1 4 Sample and Population Population - all members of any class of units (e.g. all adults in the UK; all students in England). Census - complete enumeration of population. Sample - subset of population selected. Statistic - characteristic of the sample, e.g. mean weight of a sample of people. Parameter - characteristic of the population, e.g. proportion voting Conservative. RESM2001 - Lecture 2.1 5 An Example How many people in the UK lived in poverty in 2014? Population: all people living in the UK between 1 January and 31 December 2014. Sample: The UK Family Resources Survey: 23,000 households with 50,000 individuals in the UK sampled during 2014. Note: Need to be clear about definitions. RESM2001 - Lecture 2.1 6 All survey estimates are computed using a sample, e.g. Labour Force Survey, British Election Study. However, what we are really interested in is what the sample estimates tell us about the corresponding population parameters. Question: Why a sample? RESM2001 - Lecture 2.1 7 Basic Idea of Statistical Inference We draw a random sample (recall RESM1002) from a population. Measure characteristic in the sample, e.g. sample proportion who trust the police. Use the sample statistic to infer something about the corresponding population parameter, e.g. population proportion who trust the police. Might be interested in means, proportions, …. RESM2001 - Lecture 2.1 8 Will a sample give the same results as a population? No! There are 2 general sources of ‘error’: – Sampling error - use of a sample as opposed to the whole population. – Non-sampling error - mistakes during the sampling process, such as poor questions, bias by interviewers, measurement/response errors, non-response bias, incorrect data entry, etc. RESM2001 - Lecture 2.1 9 So how do we infer something about the population from our sample statistic? The key is the use of random sampling and various tools for inference. We need, however, a mechanism to take us from the sample to the population … RESM2001 - Lecture 2.1 10 Probability Sampling Recall (RESM1002) that a probability sample is one which is drawn randomly from the sampling frame, such that every unit of the population has a known and non-zero chance of being selected for inclusion into the sample. For this to hold, some random mechanism must be used to select the sample. – The person selecting the sample has no influence on who is selected. RESM2001 - Lecture 2.1 11 In this module, the methods we use assume that the sample has been selected using simple random sampling (SRS), which is a probability sampling method where each population unit has an equal chance of being selected into the sample. Recall (RESM1002) other popular probability sampling designs: – stratified simple random sampling – cluster sampling – systematic sampling – multi-stage sampling RESM2001 - Lecture 2.1 12 If your sample is not random and you want to make inferences about the population then you are in trouble! Recall (RESM1002) the opinion polls prior to the 2015 general election were predicting Labour and the Conservatives would have equal shares of the vote, but got it very wrong! RESM2001 - Lecture 2.1 13 Sampling Distributions How can we generalise to a population of 60 million from a sample of 30). Notice that the sample size matters for the variability in the sampling distribution. – The larger the sample, the smaller the variability in the sampling distribution (smaller SE). This fits with our intuition: a larger sample means more information about our population parameter. RESM2001 - Lecture 2.1 32 Recommended Reading/Homework Foster, L., Diamond, I. and Jefferies, J. (2015). Beginning Statistics: an Introduction for Social Scientists (2nd edition). London: Sage. Chapter 8. Blackboard material – Go through the recorded lecture giving a demonstration of how the CLT works. – Work through the two examples of the application of the CLT. RESM2001 - Lecture 2.1 33