3001-5.pptx
Document Details
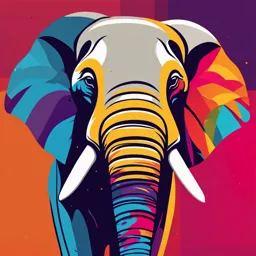
Uploaded by NimbleGyrolite6460
Full Transcript
PROBABILITY: BRIEF HISTORY PROBABILITY: QUANTIFIYING CHANCE From Wikipedia: “The mathematical methods of probability arose in the investigations first of Gerolamo Caardano in the 1560s (not published until 100 years later), and then in the correspondence [between] Pierre de Fermat and Blaise Pasca...
PROBABILITY: BRIEF HISTORY PROBABILITY: QUANTIFIYING CHANCE From Wikipedia: “The mathematical methods of probability arose in the investigations first of Gerolamo Caardano in the 1560s (not published until 100 years later), and then in the correspondence [between] Pierre de Fermat and Blaise Pascal (1654) on such questions as the fair division of the stake in an interrupted game of chance.” PROBABILITY: QUANTIFIYING CHANCE There is correspondence between PROBABILITY and FREQUENCY. Probability = Frequency/Total Frequency That is, a probability distribution can be derived from a frequency distribution. THE NORMAL DISTRIBUTION THE NORMAL DISTRIBUTION The normal distribution is a THEORETICAL PROBABILITY distribution. THE NORMAL (GAUSSIAN) DISTRIBUTION From Wikipedia: “In probability theory, the normal (or Gaussian) distribution is a continuous probability distribution that has a bell-shaped probability density function, known as the Gaussian function or informally as the bell curve.” “Gauss’s fame as a mathematician made him a worldclass celebrity. In 1807, as the French army was approaching Gottingen, Napoleon ordered his troops to spare the city because ‘the greatest mathematician of all times is living there.’” (Bernstein, 1998, p. 136). Bernstein P.L. (1998) Against the gods: The remarkable story of risk. Wiley & Sons Inc: New York. THE NORMAL (GAUSSIAN) DISTRIBUTION The area under the normal distribution represents 100% (or p = 1.00) The area under the normal distribution is divided by the MEAN and STANDARD DEVIATION THE NORMAL (GAUSSIAN) DISTRIBUTION Frequency Mean (Average) 50% 50% THE NORMAL (GAUSSIAN) DISTRIBUTION Frequency Mean (Average) 34% 34% Standard deviation Standard deviation 2½% 2½% 95% “Significance” limit “Significance” limit “THE WISDOM OF CROWDS” From Wikipedia: “At a 1906 country fair in Plymouth, 800 people participated in a contest to estimate the weight of a slaughtered and dressed ox. Statistician Francis Galton observed that the MEDIAN guess, 1207 pounds, was accurate within 1% of the true weight of 1198 pounds.” INDEPENDENT scores (measures) often (not always) TREND towards a NORMAL DISTRIBUTION. “THE WISDOM OF CROWDS” The MEAN (average) of the normal distribution often (not always) tends to approximate the true mean (or value). The MORE INDEPENDENT SCORES (measures) the MORE ACCURATE the APPROXIMATION to the TRUE MEAN mean (or value). AD HOC IN CLASS EXPERIMENT NUMBER OF PAGES (SHEETS IN A TEXTBOOK) N = 33 Independent estimates 900 The data were CODED (by hand) from paper to spreadsheet on a “first come first served” basis (i.e., no predetermined selection). See later comment re DATA CODING. 800 700 600 500 400 300 200 100 0 1 2 3 4 5 6 7 8 9 10 11 12 13 14 15 16 17 18 19 20 21 22 23 24 25 26 27 28 29 30 31 32 33 900 Outlier The data were then sorted from lowest estimate through highest, only for purposes of illustration. Note the two OUTLIERS (from visual observation) indicated by arrows. (Statistical analysis indicates only ONE outlier, as determined from the number of standard deviations from the mean.) 800 700 600 500 MEDIAN: 308 * MEAN: 307 400 * Excludes ONE outlier 300 200 Outlier 100 0 1 2 3 4 5 6 7 8 9 10 11 12 13 14 15 16 17 18 19 20 21 22 23 24 25 26 27 28 29 30 31 32 33 900 Outlier The data were then sorted from lowest estimate through highest, only for purposes of illustration. Note the two OUTLIERS (from visual observation) indicated by arrows. (Statistical analysis indicates only ONE outlier , as determined from the number of standard deviations from the mean.) 800 700 600 500 MEDIAN: 308 * MEAN: 307 400 * Excludes ONE outlier 300 200 100 0 1 2 3 4 5 6 7 8 9 10 11 12 13 14 15 16 17 18 19 20 21 22 23 24 25 26 27 28 29 30 31 32 33 6 MEDIAN: 306 * MEAN: 307 True Value: 302 BINS: 25 * 5 Excludes ONE outlier 4 3 2 1 0 100 125 150 175 200 225 250 275 300 325 350 375 400 425 450 475 500 More 7 MEDIAN: 306 * MEAN: 307 True Value: 302 BINS: 50 6 * Excludes ONE outlier 5 4 3 2 1 0 100 150 200 250 300 350 400 450 500 More 12 True Value: 302 BINS: 100 MEDIAN: 306 * MEAN: 307 * 10 Excludes ONE outlier 8 6 4 2 0 100 200 300 400 500 More SAMPLING SAMPLES AND POPULATIONS Two key words: REPRESENT (REPRESENTATION) and GENRALIZE (GENERALIZATION) Typically, we CANNOT access the entire population. Fortunately, this limitation does not matter IF the SAMPLE is REPRESENTATIVE of the POPULATION. SAMPLES AND POPULATIONS We sample to REPRESENT the population. We gather and analyze data from the sample and GENERALIZE the findings to the population. SAMPLES AND POPULATIONS Hereafter, we consider ONE sample drawn from ONE population (N = 1). The following consideration extends to MANY samples drawn from MANY populations, each sample representing the population from which it is drawn (N > 1). SAMPLES AND POPULATIONS Sampling: Representation – for later purposes of generalization. POPULATION SAMPLE 8 POPULATION (subjects of interest) Generalize findings from sample to population Select sample from population SAMPLE (subjects in study) 8 TARGET population ACCESSIBLE population Population SAMPLE Accessible Population Sample Sampling BIAS will lead to INACCURACIES when generalizing the findings from the sample to the population of interest. Sampling BIAS may arise through CHANCE (see later) or through SELECTION BIAS. 8 SAMPLING TECHNIQUES In science, sampling occurs on the basis of PROBABILITY (although note later caveat). RANDOM (probability) SAMPLING provides the BEST REPRESENTATION of a POPULATION. The reason is that random sampling is UNBIASED ,although a biased sample may still result from chance. 8 SAMPLING TECHNIQUES The FIDELITY (ACCURACY) of a RANDOM SAMPLE to the POPULATION increases with the NUMBER of samples (observations). That is, INCREASING the SAMPLE SIZE increases its fidelity (accuracy) to represent the population from which it was drawn. Example: Below is a a population of fifty white marbles and fifty black marbles. Increasing the sample size, say from N = 10 to N = 20, 50 etc., will better represent the 50:50 ratio in the population. Same example applies for coin tosses. TYPES OF PROBABILITY SAMPLES RANDOM SAMPLING Population Accessible Population Sample For the sample to be random, there is an EQUAL and INDEPENDENT chance that each subject will be selected from the (accessible) population. Random sampling is NON-DISCRIMINATORY. Random sampling can take place WITH or WITHOUT replacement (usually WITHOUT). Increasing the sample size, say to N = 100 means that the random sample drawn WITHOUT replacement now IS the population. In contrast, a random sample WITH replacement means that each draw is always from 100 marbles. Random sampling does NOT guarantee that the sample will be representative (cf. the marbles and/or coin toss examples). REPEAT random sampling yields a sampling DISTRIBUTION: The NORMAL (or bell) CURVE. POPULATION S10 S5 S2 S7 S9 S6 S 4 S1 S8 S3 9 Frequency Mean (Average) S8 S2 SS 6 1 S9 S4 S3 SS 7 10 S5 9 Frequency Mean (Average) Standard Deviation Standard Deviation Population S8 S2 SS 6 1 S9 S4 S3 SS 7 10 S5 CENTRAL TENDENCY: Mean SPREAD: Standard deviation (i.e., variation). Variance is the square of variation. 9 REPEAT random samplings yield CLOSER APPROXIMATIONS to the MEAN. (This finding is known as the CENTRAL LIMIT THEOREM, or the law of large numbers). In practice, however, we usually take only ONE sample, from an infinite number, and draw CONFIDENCE INTERVALS (or LIMITS) from our sample. These limits are sometimes referred to as the MARGIN OF ERROR. POPULATION S10 S5 S 7 S2 S8 S9 S6 S4 S1 S3 Frequency Mean (Average) Standard Deviation S1 NOTE: Sample 1 (S1) has a mean and standard deviation (observed) that approximates that of the population (unobserved), as quantified using probability theory. Frequency Mean (Average) Standard Deviation S1 S1: 95% Confidence Limits NOTE: Sample 1 (S1) has a mean and standard deviation (observed) that approximates that of the population (unobserved), as quantified using probability theory. CONFIDENCE INTERVALS (LIMITS) indicate the level of (likely) PRECISION in the ESTIMATE (APPROXIMATION) of the SAMPLE DISTRIBUTION to the POPULATION DISTRIBUTION. SAMPLING TECHNIQUES Random Stratified random Proportionate stratified random Systematic Combined strategy RANDOM SAMPLING As before. STRATIFIED RANDOM SAMPLING Identify STRATA in the POPULATION and then draw SAMPLES from each STRATA (or subpopulation). Increases the level of REPRESENTATION in the SAMPLE, particularly for small percentages of the population. STRATIFIED RANDOM SAMPLING Family Income $250,000+ $100,000 - $249,999 $75,000 - $99,999 $50,000 - $74,999 $25,000 - $49,999 Less than $25,000 % Population 2% 8% 15% 35% 25% 15% PROPORTIONATE STRATIFIED RANDOM SAMPLING Same as stratified sampling except that the strata are sampled on a PROPRTIONATE basis as per the known make-up of the population. Family Income $250,000+ $100,000 - $249,999 $75,000 - $99,999 $50,000 - $74,999 $25,000 - $49,999 Less than $25,000 % Population 2% 8% 15% 35% 25% 15% PROPORTIONATE STRATIFIED RANDOM SAMPLING Therefore, in the example below, 2% of the sample drawn from $250,000+, 8% from $100,000 - $249,999, etc. (Preferred.) Family Income $250,000+ $100,000 - $249,999 $75,000 - $99,999 $50,000 - $74,999 $25,000 - $49,999 Less than $25,000 % Population 2% 8% 15% 35% 25% 15% CLUSTER SAMPLING Sample CLUSTERS (e.g., schools) then sample WITHIN CLUSTERS (e.g., classes). Samples of samples are LESS ACCURATE. SYSTEMATIC SAMPLING Use a sampling INTERVAL – E.g., select every mth sample from N subjects. This method CAN cause NON-REPRESENTATION if the sampling frame is somehow PATTERNED (i.e., ordered). COMBINED-STRATEGY SAMPLING Sometimes use a mix of sampling strategies, if appropriate, to try to get a good representation of the population. Proportionate stratified random sampling is an example of a combined-strategy sampling approach. SAMPLE SIZES: SOME CONSIDERATIONS The SMALLER the POPULATION, the LARGER the SAMPLING RATIO (Sample:Population) that is required for an accurate sample. For SMALL SAMPLES, SMALL INCREASES in SAMPLE SIZE yield LARGE GAINS in ACCURACY. (Think of the marble and/or coint toss example.) Three factors to consider when sampling: (A) The degree of ACCURACY required (B) VARIABILITY (or diversity) of the POPULATION (C) NUMBER of VARIABLES to be examined Increasing sample size is a good idea, notwithstanding practical limitations, keeping in mind the “law of diminishing returns. Sampling occurs so that INFERENCES can be drawn from the SAMPLE(S) to the POPULATION(S). Inferences of DIFFERENCES between POPULATIONS, as represented by samples, are drawn using INFERENTIAL STATISTICS E.g., ttest, ANOVA, etc. CAVEAT: NON-PROBABILITY SAMPLING CONVENIENCE SAMPLING: Subjects that are available/accessible/willing to participate in the study. In practice, we use convenience sampling NOT straight forward random sampling. Some requirements (ethics). Some practical restrictions (access). Some consequent limitations re generalizability. DESCRIPTIVE STATISTICS The normal distribution is just that, a DISTRIBUTION of data. There are distributions of data that may NOT be “normal”. For example, a LINEAR distribution. Y X One may argue that below is a linear distribution. Y X Regarding CORRELATION it is NOT considered as such. DESCRIPTIVE STATISTICS Statistics, including inferential statistics, are a means of MANAGING and ANALYZING data for purposes of INTERPRETATION (and UNDERSTANDING). DATA PROCESSING Sometimes it is necessary to CODE (TRANSLATE) the data prior to statistical analysis. CODED data should be checked for ERRORS: Errors of TRANSLATION, INTERNAL INCONSISTENCIES, OUTLIERS. DATA PROCESSING Data may (will) sometimes be “GROUPED” (COLLAPSED) before proceeding to statistical analysis, if desired (required). For example, take the MEAN or MEDIAN from, say, three measures (e.g., skinfold measures). FREQUENCY DISTRIBUTIONS As indicated, DESCRIPTIVE statistics are used to DESCRIBE the numerical data in the (frequency) DISTRIBUTION. There are Univariate, Bivariate or Multivariate statistics, referring to one, two or many (i.e., more than two) dependent variables. FREQUENCY DISTRIBUTIONS The dependent variables fall into one of the four levels of measurement: Nominal, Ordinal, Interval and Ratio. Some common types of frequency distributions include Histogram, Bar Chart, Pie Chart and Scattergram. KEY DESCRIPTORS FOR FREQUENCY DISTRIBUTIONS CENTRAL TENDENCY: Mean, Median and Mode. The four types (levels) of measures that can be represented by each of the measures of central tendency (3M’s) vary. KEY DESCRIPTORS FOR FREQUENCY DISTRIBUTIONS MODE: The most frequent value. Applies to Nominal, Ordinal, Interval and Ratio scales. MEDIAN: The middle value (i.e., the 50th percentile). Applies to Ordinal, Interval and Ratio scales. MEAN: The average value. Applies to Interval and Ratio scales. KEY DESCRIPTORS FOR FREQUENCY DISTRIBUTIONS The mode, median and mean for each measure DIFFERENT PROPERTIES of CENTRAL TENDENCY. These measures may or may not give the same/similar results depending on the data. KEY DESCRIPTORS FOR FREQUENCY DISTRIBUTIONS In general, the mean is used for most statistics on central tendency. For SKEWED distributions, the MEDIAN tends to be used, however, since it is LESS SENSITIVE to extreme values (i.e., outliers). Central panel: Normal distribution Left panel: Negative skewed distribution Right panel: Positive skewed distribution Frequency SD -ve +ve Mode Mean Median Mode, Median AND Mean Frequency 4 DATA: 5 5 1 4 8 3 2 3 2 5 1 6 MODE: 5 MEAN: 4 MEDIAN: 4.5 1 0 1 2 DATA: 3 1 4 1 5 2 3 6 4 7 5 8 5 5 9 6 10 8 The spread, dispersion or variability is another important characteristic of a distribution. The range, percentile and standard deviation each measure the spread of a distribution. These measures will give DIFFERENT results from the SAME data. RANGE: The DIFFERENCE between the LOWEST and HIGHEST value. PERCENTILE: The DIFFERENCE between TWO percentiles, typically the 25th and 75th percentiles, sometimes the 10th and 90th percentiles. STANDARD DEVIATION: The AVERAGE DISTANCE between EACH SCORE and the MEAN. SD = ((X - X)2)/N Mean Or SD = ((X - X)2)/(N – 1) STANDARD DEVIATION: EXAMPLE Data 1 2 3 4 5 Mean 3 3 3 3 3 Difference -2 -1 0 1 2 Difference Squared 4 1 0 1 4 Sum 10 Sum 10 N 5 N-1 4 SD2 2 SD2 2.5 SD 2 SD 2.5 Frequency 4 DATA: 5 5 1 4 8 3 2 5 1 6 RANGE: 3 7 3.5 PERCENTILE: * 2 SD: 2.26 1 0 1 * * 2 DATA: 3 1 4 1 Quarter (25th) Percentile 2 5 3 6 4 7 5 8 5 5 9 6 10 8 Z-SCORES Z-scores STANDARDIZE normal distributions allowing for COMPARISONS. z = (Score – Mean)/SD In effect, a score in a normal distribution is expressed in a z-score, indicating the distance of the score from the mean in standard deviation units. For example: Frequency Mean = 100 Score = 112 Standard deviation = 20 Score = 65 Not to scale. In effect, a score in a normal distribution is expressed in a z-score, indicating the distance of the score from the mean in standard deviation units. For example: Frequency Z = (65–100)/20 Mean = 100 Z = (112 – 100)/20 = 0.6 Standard deviation = 20 Z = -1.75 Not to scale. EXAMPLES Two sample distributions, one for women and one for men. Two independent variables. Height: Jill has a z-score of 0.80, Jack has a zscore 0f -0.9. Who is TALLER within their respective normal distributions. JILL Weight: Zoe has a z-score of 0.20, Zac has a z-score of 0.25. Who is LIGHTER within their respective normal distributions. ZOE COVARIATION TWO variables MAY or MAY NOT be RELATED. If two variables are related then they will COVARY, if they are not related then they will NOT COVARY. In other words, the variables will be INDEPENDENT of each other. The SCATTERGRAM plots the two variables on a single graph (cf. correlation). The convention is to use the Y-AXIS to plot the measure of interest, i.e., the DEPENDENT variable. COVARIATION: SOME EXAMPLES y x No relation y x Correlation y x Exponential relation There are THREE aspects of interest when expressing a relationship (or pattern) between two variables: FORM: Linear, Non-linear DIRECTION: Positive, Negative PRECISION: The degree of adherence to the relationship. STATISTICAL MEASURES OF ASSOCIATION The STRENGTH (cf. precision) and DIRECTION of a relationship is expressed in a measure of association, typically a single number; For example, r = 0.68, r = -0.83; The CORRELATION COEFFICIENT reflects how much one variable (z1-score) VARIES with another variable (z2-score), i.e., it is a measure of COVARIATION; C0RRELATION: AGE AND PRICE OF WINE HYPOTHETICAL DATA Difference Wine Age A B C D E 2 3 5 6 4 Difference2 $ Pric Age Price Age e 10 -2 -5 4 5 -1 -10 1 20 +1 +5 1 25 +2 +10 4 15 0 0 0 Total 20 Mean 4 1.6 SD 75 15 7.9 Price z Age Price 25 -1.41 -0.71 100 -0.71 -1.41 25 0.71 0.71 100 1.41 1.41 0 0.00 0.00 z Product 1.00 1.00 0.50 2.00 0.00 4.50 0.90 30 Price 25 Excel Statistical Analysis 20 Age 1 0.9 Age Price Price 1 15 r = 0.9 r2 = 0.81 10 5 0 1.5 Age 2 2.5 3 3.5 4 4.5 5 5.5 6 6.5 MULTIPLE REGRESSION Used to examine the effects of multiple (more than one) INDEPENDENT variables on a DEPENDENT VARIABLE. The amount of COVARIATION (or SHARED VARIANCE) is represented as R2. (The amount of COVARIATION is represented as r2 for BIVARIATE relations i.e., CORRELATION). A r2 B If rAB = 0.6 then r2 = 0.36 (or 36%) [6/10 * 6/10 = 36/100] Not to scale. A r2 B If rAB = 0.6 then r2 = 0.36 (or 36%) Example-1: A. Sex (Independent Variable) B. Strength (Dependent Variable) Example-2: A. Height (Dependent Variable) B. Weight (Dependent Variable) Not to scale. B C R2 R2 = 0.19 or 19% A Example: A. Sex (Independent Variable) B. Age (Independent Variable) C. Age (Independent Variable) Dependent Variable: Strength Not to scale.