Hypothesis Testing PDF
Document Details
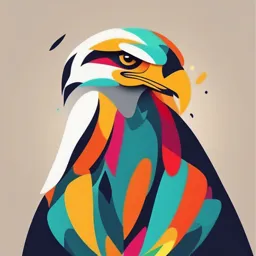
Uploaded by GlimmeringMars
Iloilo National High School
Tags
Summary
This document provides an overview of hypothesis testing, including definitions of terms, procedures, and examples. It covers various types of hypotheses, common phrases, and potential errors in decision-making.
Full Transcript
Hypothesis testing is a statistical method that is used in making statistical decisions using experimental data. Hypothesis testing is basically an assumption that we make about the population parameter. Procedure in Hypothesis Testing 1. State the null hypothesis and alternative hypothesis. 2....
Hypothesis testing is a statistical method that is used in making statistical decisions using experimental data. Hypothesis testing is basically an assumption that we make about the population parameter. Procedure in Hypothesis Testing 1. State the null hypothesis and alternative hypothesis. 2. Choose the level of significance. 3. Select appropriate test statistic. 4. Determine the critical values that divide the rejection and nonrejection regions (if the decision is to be based on P value it is not necessary to state the critical region). 5. Compute for the value of the test statistic from the sample data. 6. Make a statistical decision. 7. State the conclusion. Two Types of Statistical Hypotheses Null Hypothesis (H0 – “H sub Zero/ H null”) The null hypothesis states that a population parameter is equal to a value. The null hypothesis is often an initial claim that researchers specify using previous research or knowledge. It is a statement of no effect, relationship, or difference between two or more groups or factors. In research studies, a researcher is usually interested in disproving the null hypothesis. Examples: There is no difference between the average ages of male and female customers. The intervention and control groups have the same survival rate (or, the intervention does not improve survival rate). There is no association between the atmospheric temperature and the total sales of fruit shake. Alternative Hypothesis (H1 or Ha) An alternative hypothesis states that the population parameter is different from the value of the population parameter in the null hypothesis. The alternative hypothesis is what you might believe to be true or hope to prove true. It is the statement that there is an effect or difference. This is usually the hypothesis the researcher is interested in proving. Examples: The average age of male customers differs with the average age of female customers. The time to resuscitation from cardiac arrest is lower for the intervention group than for the control. There is an association between the atmospheric temperature and the total sales of fruit shake. The alternative hypothesis can be one- sided (only provides one direction) or two-sided. We often use two-sided tests even when our true hypothesis is one-sided because it requires more evidence against the null hypothesis to accept the alternative hypothesis. In order to state the hypothesis correctly, the researcher must translate correctly the claim into mathematical symbols. There are three possible sets of statistical hypotheses. 1. H0 : parameter = specific value (two-tailed test) Ha: parameter ≠ specific value 2. H0 : parameter = specific value (left-tailed test) Ha: parameter < specific value 3. H0 : parameter = specific value (right-tailed test) Ha: parameter > specific value Hypothesis Testing Common Phrases > < is greater than is less than is above is below is higher than is lower than is longer than is shorter than is bigger than is smaller than is increased is decreased or reduced from Hypothesis Testing Common Phrases ≥ is greater than or equal to is at least is not less than ≤ is less than or equal to is at most is not more than Hypothesis Testing Common Phrases = is equal to is exactly the same as/ is the same as has not changed from ≠ is not equal to is different from has changed from is not the same as Errors in decision making Possible situations in testing a statistical hypothesis Statistical H0 is TRUE H0 is FALSE Decision Do not Correct Type II error Reject H0 Decision (β) Correct Reject H0 Type I error (α) Decision A Type I error, also known as a false positive, occurs when a null hypothesis is rejected when it is actually true. In other words, it is the incorrect rejection of a true null hypothesis. For example, let's say a medical researcher is conducting a clinical trial to test a new drug's effectiveness in treating a certain disease. Ho: The drug has no effect, meaning there is no difference between the treatment group (given the drug) and the control group (given a placebo). Decision: The researcher concludes that there is a significant difference between the treatment group and the control group. Based on this conclusion, the researcher rejects the null hypothesis and concludes that the drug is effective in treating the disease. However, in reality, the drug actually has no effect, and the observed difference between the groups is due to random chance or other factors unrelated to the drug. This means that the null hypothesis was actually true (the drug has no effect), but it was incorrectly rejected. In this scenario, the Type I error would be the false conclusion that the drug is effective when it is not, leading to potentially incorrect treatment decisions and wasted resources. A Type II error, also known as a false negative, occurs when a null hypothesis is not rejected when it is actually false. In other words, it is the failure to reject a false null hypothesis. For example, a company manufacturing light bulbs claims that their bulbs have an average lifespan of 1000 hours. A consumer protection agency wants to test this claim and conducts a hypothesis test. Ho: The average lifespan of the bulbs produced by the company is indeed 1000 hours. Now, suppose that in reality, the average lifespan of the bulbs is not 1000 hours but is actually lower, let's say 900 hours. The consumer protection agency conducts a hypothesis test but fails to reject the null hypothesis based on their sample data. They conclude that there is not enough evidence to suggest that the average lifespan of the bulbs is different from 1000 hours. However, in reality, the bulbs do not meet the claimed lifespan of 1000 hours. This means that the null hypothesis was actually false (the average lifespan is not 1000 hours), but it was not rejected. In this scenario, the Type II error would be the failure to identify that the bulbs do not meet the claimed lifespan, leading to a false conclusion that the null hypothesis is true when it is actually false. This can result in consumers being misled about the product's quality. Level of Significance The probability of committing a Type I error is called the level of significance, denoted by the Greek letter alpha (α). The probability of committing a Type II error, denoted by β, is difficult to determine unless we have a specific alternative hypothesis. In hypothesis testing, the level of significance refers to the degree of significance in which we accept or reject the null hypothesis which is assumed as true. In hypothesis testing, 100% accuracy is not possible for accepting or rejecting a null hypothesis. So, we select a level of significance that is usually 0.01, 0.05and 0.10. That is, if the null hypothesis is rejected, the probability of a type I error will be 10%, 5% or 1%, and the probability of a correct decision will be 90%, 95%, or 99%, depending on which level of significance is used. For example, a 0.05 or 5% level of significance is chosen in designating a test of hypothesis, then there are about 5 chances in 100 that we would reject the hypothesis when it should be accepted, i.e., we are about 95% confident that we made the right decision. In a hypothesis-testing situation, the researcher decides what level of significance to use. it can be any level, depending on the seriousness of the type I error. Some properties of α and β The Type I and Type II errors are related. A decrease in the probability of one results in an increase in the probability of other. The size of the critical region, therefore the probability of committing Type I error can always be reduced by adjusting the critical value(s). An increase in the sample size will reduce α and β simultaneously. If the null hypothesis is false, β is maximum when the true value of a parameter approaches the hypothesized value. The greater the distance between the true value and the hypothesized value, the smaller the β will be. P value Wording Summary < 0.001 Extremely Significant *** 0.001 to 0.01 Very Significant ** 0.01 to 0.05 Significant * > 0.05 Not Significant ns P value Interpretation Highly statistically significant Less than 0.01 (very strong evidence against the null hypothesis) Statistically significant 0.01 to 0.05 (Adequate evidence against the null hypothesis) Greater than Not Significant (Insufficient evidence against the null hypothesis) 0.05 Definition of Terms Statistical hypothesis – an assertion or conjecture concerning the population or more populations. Null hypothesis – the hypothesis or assumption about the population parameter we wish to test. Alternative hypothesis – the conclusion we accept when the data fail to support the null hypothesis. Significance level - a value indicating the percentage of sample values that is outside a certain limits, assuming the null hypothesis is correct; i.e. the probability of rejecting the null hypothesis. Definition of Terms Power of the test – the probability of rejecting the null hypothesis when it is false, i.e. it is measure of how well the hypothesis test is working. Test statistic – a statistic used in deciding whether to reject or to accept the null hypothesis. Confidence level – the probability that the parameter tested is within the specified values in the hypothesis. Critical region or rejection region – part of the set of all possible values of a sample statistic for which the hypothesis to be tested is rejected. Definition of Terms Critical value – the last number observed in passing from the acceptance region into the rejection region. Dependent samples – samples drawn from two populations in such a way that the elements were not chosen independently of one another, in order to allow a more precise analysis or to control for some extraneous factors. One-tailed test – a hypothesis test in which there is only one rejection regions, i.e. we are concerned only with whether the observed values deviates from the hypothesis value in one direction. Definition of Terms Two-tailed test – a hypothesis test in which the null hypothesis is rejected if the sample is significantly higher or lower than the hypothesized value of the population parameter; a test involving two rejection regions. Type I error – rejection of the true hypothesis. Type II error – acceptance of the false hypothesis. Sample problems Example 1 A researcher wants to determine whether there is a significant difference in the mean scores of two groups of students who received different teaching methods. The researcher randomly selects 25 students from each group and records their test scores. Group A receives traditional teaching methods, while Group B receives a new experimental teaching method. The average test score for Group A is 85 with a standard deviation of 10, and the average test score for Group B is 90 with a standard deviation of 12. The researcher wants to test whether there is a significant difference in the mean test scores between the two groups at a significance level of 0.05. State the null hypothesis (Ho) and the alternative hypothesis (Ha), perform the hypothesis test, and interpret the results. Solution: Step 1: Ho: There is no significant difference in the mean test scores between Group A (μA) and Group B (μB). Ha: There is a significant difference in the mean test scores between Group A (μA) and Group B (μB). Step 2: Significance Level (α) = 0.05 Step 3: Since we are comparing the means of two independent samples and the sample sizes are relatively small (n < 30) and the population standard deviations are unknown, we can use a two-sample t-test for independent samples. Solution: Step 4: Determine the degrees of freedom (df): Since the sample sizes for both groups are the same (25), the degrees of freedom can be calculated as df = 25+25−2=48 Find the critical t-value at a significance level of 0.05 and degrees of freedom of 48 using a t-table or statistical software. For a two-tailed test with α = 0.05 and df = 48, the critical t-value is approximately ±2.012. Step 5. Computation Step 6. Decision: Compare the calculated t-score with the critical t-value: Since the calculated t-score (-1.60) falls within the critical region (-2.012, +2.012), we fail to reject the null hypothesis. Step 7. Interpret the result There is not enough evidence to conclude that there is a significant difference in the mean test scores between Group A and Group B. Therefore, the researcher cannot conclude that the new experimental teaching method has a statistically significant effect on test scores compared to traditional teaching methods. Example 2 A researcher wants to determine whether there is a significant linear relationship between the number of hours spent studying and the scores obtained on a standardized test. The researcher collects data from 20 students, recording the number of hours each student studied and their corresponding test scores. After calculating Pearson's correlation coefficient (r), the researcher obtains a correlation coefficient of 0.70. The researcher wants to test whether there is a significant correlation between the number of hours spent studying and the test scores at a significance level of 0.05. Solution Step 1. Ho: There is no significant correlation between the number of hours spent studying and the test scores (ρ=0). Ha: There is a significant correlation between the number of hours spent studying and the test scores (ρ ≠ 0). Step 2. Significance Level (α) = 0.05 Step 3. Since we are testing for a correlation between two variables, we can use Pearson's correlation coefficient (r) and perform a hypothesis test to determine if the correlation is significant. Step 4. Determine the critical values for the correlation coefficient at a significance level of 0.05. For a two-tailed test: df = 20-2 = 18 the critical values of r = ±0.444. Step 5. Compare the calculated correlation coefficient with the critical values. The calculated correlation coefficient (r) is 0.70. Step 6. Decision Rule Since the calculated correlation coefficient (r = 0.70) falls within the critical region, we reject the null hypothesis. Step 7. Conclusion. There is enough evidence to conclude that there is a significant correlation between the number of hours spent studying and the test scores. In other words, students who spend more hours studying tend to achieve higher test scores, and this relationship is statistically significant. Some Statistical Tools Purpose of Test Parametric Nonparametric (Random (Non-random sampling) sampling) Significant difference T –test (Independent Mann – Whitney U test between 2 groups samples) Paired samples Paired t – test Wilcoxon Signed -Rank Test Degree of association Pearson’s r (Pearson’s Spearman’s rho (spearman between the two variables product-moment rank correlation) correlation coefficient) Difference between chi-square test Chi-square goodness-of-fit categorical variables (Pearson’s chi-square test) test Significant difference One – way Analysis of Kruskal Wallis Test between 3 or more groups Variance (ANOVA)