Summary - Theory and Methods of Comparative Politics PDF
Document Details
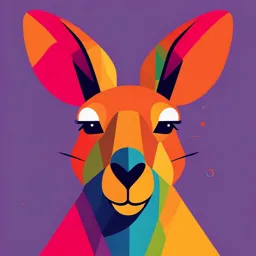
Uploaded by EntrancingChlorine
Universität Tübingen
Tags
Summary
This document provides a comprehensive summary of the theory and methods of comparative politics. It covers topics including comparative methods, data collection, case studies, and analysis techniques, such as qualitative comparative analysis and large n analysis. The document also explores the concept of the state, regime types, and transitions.
Full Transcript
Summary - Theory and methods of Comparative Politics Inhaltsverzeichnis {#inhaltsverzeichnis.Inhaltsverzeichnisberschrift} ================== [1. Why and How Do We Compare? 1](#why-and-how-do-we-compare) [1.1 Why Compare? 1](#why-compare) [Feasibility and Ethical Considerations 1](#feasibility-a...
Summary - Theory and methods of Comparative Politics Inhaltsverzeichnis {#inhaltsverzeichnis.Inhaltsverzeichnisberschrift} ================== [1. Why and How Do We Compare? 1](#why-and-how-do-we-compare) [1.1 Why Compare? 1](#why-compare) [Feasibility and Ethical Considerations 1](#feasibility-and-ethical-considerations) [Purposes of Comparison 1](#purposes-of-comparison) [The Comparative Method 1](#the-comparative-method) [1. 2. Compare What 1](#compare-what) [Comparability 1](#comparability) [Classes and Classification 2](#classes-and-classification) [What is Comparative Politics? 2](#what-is-comparative-politics) [Units of Analysis 2](#units-of-analysis) [Analytical Lenses: The Five/Six "I"s (Peters) 2](#_Toc189652008) [1. 3. Compare How 2](#compare-how) [Methodology vs. Method 2](#methodology-vs.-method) [Types of Methods 3](#types-of-methods) [Research Strategies (Modes of Inquiry) 3](#research-strategies-modes-of-inquiry) [1. 4 The importance of Concepts and Theory in Comparison 3](#the-importance-of-concepts-and-theory-in-comparison) [Concepts 3](#concepts) [Explanation & Variables 3](#explanation-variables) [Theories 4](#theories) [The Role of Theory (Peters) 4](#the-role-of-theory-peters) [Hypothesis Testing 4](#hypothesis-testing) [2. Causal Inference and Research 4](#causal-inference-and-research) [2.1 Research Traditions: Empirical-Analytic vs. Interpretivist 4](#research-traditions-empirical-analytic-vs.-interpretivist) 2.1 Key Terms in the Theory of Knowledge 4 [Research Traditions & Methods 5](#research-traditions-methods) [2.3 Quantitative vs. Qualitative 5](#quantitative-vs.-qualitative) [Similarities 5](#similarities) [Key Differences 5](#key-differences) [When to Use Which Approach? 6](#when-to-use-which-approach) [2.4 Logics of Inference 6](#logics-of-inference-schlussfolgerung) [Arguments 6](#arguments) [Explanations 7](#explanations) [Inference 7](#inference-schlussfolgerung) [Causality 7](#causality) [3. Comparing and Miscomparing 10](#comparing-and-miscomparing) [3.1 Conceptualisation and Operationalisation 10](#conceptualisation-and-operationalisation) [3.2 Classifications and Typologies 10](#classifications-and-typologies) [What is Classification? 10](#_Toc189652035) [What is a Typology? 10](#_Toc189652036) [Ideal vs. Real Types 10](#ideal-vs.-real-types-as-explanation-of-a-typology-by-dahl) [Requirements for Typologies 11](#requirements-for-typologies) [Conceptual Hierarchies and Typology Strategies 11](#conceptual-hierarchies-and-typology-strategies) [3.3 Research Designs 12](#research-designs) [What is Research Design? 12](#what-is-research-design) [Components of Research Design 12](#components-of-research-design) [Models of Research Designs 12](#models-of-research-designs) [Choosing the Right Research Design 13](#choosing-the-right-research-design) [3.4 The Ethical Dimension of Research 13](#the-ethical-dimension-of-research) [Considerations for Ethical Research 14](#considerations-for-ethical-research) [Ethical Dilemmas and Decisions 15](#ethical-dilemmas-and-decisions) [Making Ethical Choices Easier 15](#making-ethical-choices-easier) [APSA Guidelines on Ethics 15](#apsa-guidelines-on-ethics) [4. Collecting Data 15](#collecting-data) [4.1. Basics of Data Collection 15](#basics-of-data-collection) [4.2 How to Collect Data 16](#how-to-collect-data) [Primary vs. Secondary Data 16](#primary-vs.-secondary-data) [Methods of Data Collection 16](#methods-of-data-collection) [The S-P-R Model 16](#the-s-p-r-model) [4.3 Primary Data - Experiments, surveys, interviews, observation 17](#primary-data---experiments-surveys-interviews-observation) [1. Experimental Design 17](#experimental-design) [2. Survey Experiments 17](#survey-experiments) [3. Qualitative Interviews 17](#qualitative-interviews) [4.4 Secondary Data 19](#secondary-data) [Ex Post Measures 19](#ex-post-measures) [Core Methods 19](#core-methods) [Opportunities and Advantages 20](#opportunities-and-advantages) [Problems and Shortcomings 20](#problems-and-shortcomings) [5. Single and Small n-Analysis 20](#single-and-small-n-analysis) [5.1. Cases and Case Studies 20](#cases-and-case-studies) [What is a Case? 20](#what-is-a-case) [What is a Case Study? 20](#what-is-a-case-study) [Purposes of a Case Study (Van Evera, 1997) 21](#purposes-of-a-case-study-van-evera-1997) [Strengths and Weaknesses of Case Studies 21](#strengths-and-weaknesses-of-case-studies) [Approaches to Explanatory Case Study Research (Blatter & Haverland, 2012) 21](#approaches-to-explanatory-case-study-research-blatter-haverland-2012) [Case Studies and Theories 22](#case-studies-and-theories) [Case Selection Criteria (Van Evera, 1997) 22](#case-selection-criteria-van-evera-1997) [Avoiding Selection Bias 22](#avoiding-selection-bias) [5.2 Process Tracing 23](#process-tracing) [Process Tracing Tests 23](#process-tracing-tests) [5.3 Comparative Case Studies 23](#comparative-case-studies) [Controlled Comparisons 23](#controlled-comparisons) [Defending Small-n, Controlled Comparisons (Slater & Ziblatt, 2013) 24](#defending-small-n-controlled-comparisons-slater-ziblatt-2013) [6. Medium n-Analysis / QCA 24](#medium-n-analysis-qca) [6.1 Qualitative Comparative Analysis (QCA) 24](#qualitative-comparative-analysis-qca) [**Necessary and Sufficient Conditions** 25](#necessary-and-sufficient-conditions) [\"Multidimensional\" Conditionality 25](#multidimensional-conditionality) [Truth Tables 25](#truth-tables) [Software for QCA: 25](#software-for-qca) [6.2 Qualitative Content Analysis (QCA) 26](#qualitative-content-analysis-qca) [Basic Forms of Coding and Interpretation 26](#basic-forms-of-coding-and-interpretation) [Units of Analysis 26](#units-of-analysis-1) [Structuration in QCA 26](#structuration-in-qca) [Quality Criteria in QCA 27](#quality-criteria-in-qca) [Software for Content Analysis 27](#software-for-content-analysis) [7. Large n-Analysis 27](#large-n-analysis) [7.1 Procedures to test or Discover Structure in Data 27](#procedures-to-test-or-discover-structure-in-data) [7.2 Multivariate Analysis: Different Research Questions 28](#multivariate-analysis-different-research-questions) [Regression Analysis 29](#regression-analysis) [7.3 Factor Analysis 31](#factor-analysis) [Factor Analysis Steps: 32](#factor-analysis-steps) [7.4 Cluster Analysis 32](#cluster-analysis) [Objective: 32](#objective) [8. The nation State / The Modern State 34](#the-nation-state-the-modern-state) [8.1. Defining the State 34](#defining-the-state) [Questions on the State 34](#questions-on-the-state) [Theories of the State 34](#theories-of-the-state) [State Strength 35](#state-strength) [10.2 Definition of Stateness 35](#definition-of-stateness) [10.3 State-Society Relations and Social Contracts 35](#state-society-relations-and-social-contracts) [Definition of a social Contract 35](#definition-of-a-social-contract) [**10.4 State Theory** Conceptualizing the State: Four analytical traditions (vom Hau) 36](#state-theory-conceptualizing-the-state-four-analytical-traditions-vom-hau) [10.5 How strong is a state 36](#how-strong-is-a-state) [Measuring Stateness 37](#measuring-stateness) [The Three Gaps in States: Capacity, Legitimacy, and Security Gaps 38](#the-three-gaps-in-states-capacity-legitimacy-and-security-gaps) [9. Regimes and regime types 38](#regimes-and-regime-types) [9.1 Regime Types 39](#regime-types) [Totalitarian Regime 39](#totalitarian-regime) [Authoritarian Regime 39](#authoritarian-regime) [Democracy / Polyarchy 39](#democracy-polyarchy) [Comparing Regimes 40](#comparing-regimes) [Discussing Regime Types 40](#discussing-regime-types) [9.2 Democratic Regimes 40](#democratic-regimes) [Procedural Minimals for Democracy (Dahl): 40](#procedural-minimals-for-democracy-dahl) [Types of Democracies: 41](#types-of-democracies) [Majoritarian vs. Consensus Democracies: 41](#majoritarian-vs.-consensus-democracies) [Presidential vs. Parliamentary Democracies: 42](#presidential-vs.-parliamentary-democracies) [Measuring Democracies: 42](#measuring-democracies) [9.3 Authoritarian Regimes 43](#authoritarian-regimes) [Definition of Authoritarianism: 43](#definition-of-authoritarianism) [Forms of Authoritarian Rule: 43](#forms-of-authoritarian-rule) [Autocratic Subtypes (Kailitz, 2013): 43](#autocratic-subtypes-kailitz-2013) [Why Do Autocrats Stay in Power?: 43](#why-do-autocrats-stay-in-power) [10. Transitions 44](#transitions) [10.1 Terminology Regime Transformation 44](#terminology-regime-transformation) [Dimensions of Regime Change 44](#dimensions-of-regime-change) [10.2 Democratization 45](#democratization) [10. 3 Autocratization 45](#autocratization) [Questions on Regime Transition: 46](#questions-on-regime-transition) [10.4 **Consolidation and Deconsolidation** 46](#consolidation-and-deconsolidation) [Democratic Consolidation 46](#democratic-consolidation) [Deconsolidation 46](#deconsolidation) [10.5 How Do Regimes Transform? 47](#how-do-regimes-transform) [Theories on Democratization 48](#theories-on-democratization) [Culture and Democracy 49](#culture-and-democracy) [Importance of Civil Society 49](#importance-of-civil-society) [Theories on Autocratization 49](#theories-on-autocratization) [11. Elections and Electoral Systems 50](#elections-and-electoral-systems) [11.1 Introduction to Elections 50](#introduction-to-elections) [Elections and Their Role in Democracy 50](#elections-and-their-role-in-democracy) [11.2 The Chain of Democratic Choice 50](#the-chain-of-democratic-choice) [11.3 Electoral Integrity 51](#electoral-integrity) [Functions of Elections in Different Contexts 51](#functions-of-elections-in-different-contexts) [Functions of Elections 52](#functions-of-elections) [In different political systems 52](#in-different-political-systems) [11.4 Electoral Systems 53](#electoral-systems) [Approaches to Electoral System Research 53](#approaches-to-electoral-system-research) [Principles of Representation 53](#principles-of-representation) [Types of Electoral Systems 54](#types-of-electoral-systems) [Impact of Electoral Systems 55](#impact-of-electoral-systems) [Majoritarian vs. Proportional Systems 55](#majoritarian-vs.-proportional-systems) [11.5 Theories of Electoral Systems 55](#theories-of-electoral-systems) [Duverger\'s Laws (1986): 55](#duvergers-laws-1986) [Sartori\'s Laws (1968, 1986): 55](#sartoris-laws-1968-1986) [Voting Behaviour 56](#voting-behaviour) [12. Parties and party systems 56](#parties-and-party-systems) [12.1 Functions of Parties 56](#functions-of-parties) [Core Functions of Political Parties in Democracies 56](#core-functions-of-political-parties-in-democracies) [Sartori's Party Functions (2005) 56](#sartoris-party-functions-2005) [The Role of Parties in Autocracies 57](#the-role-of-parties-in-autocracies) [12.2 Party Types, Families, and Cleavages 57](#party-types-families-and-cleavages) [Classification of Political Parties 57](#classification-of-political-parties) [Party Families: Core Conflicts 57](#party-families-core-conflicts) [Historical Evolution of Party Types 58](#historical-evolution-of-party-types) [Western Trends in Party Development: 58](#western-trends-in-party-development-ein-bild-das-text-quittung-schrift-screenshot-enth%C3%A4lt.-ki-generierte-inhalte-k%C3%B6nnen-fehlerhaft-sein.) [Political Cleavages 59](#_Toc189652172) [12.3 The Trend of Partisan Dealignment and Party Systems 59](#the-trend-of-partisan-dealignment-and-party-systems) [12.4 Party Systems: Classification and Competition 60](#party-systems-classification-and-competition) [Types of Party Systems 60](#types-of-party-systems) [Competition in Multi-party Systems (Sartori, 2005): 60](#competition-in-multi-party-systems-sartori-2005) [Socio-Structural Explanations of Party Systems 60](#socio-structural-explanations-of-party-systems) [Institutional Explanations of Party Systems 61](#institutional-explanations-of-party-systems) [13. Capitalism, Development, and Welfare 61](#capitalism-development-and-welfare) [14. Political Culture and Participation 61](#political-culture-and-participation) 1. Why and How Do We Compare? ============================= 1.1 Why Compare? ---------------- **Comparing as a Method of Gaining Knowledge** - **Innate to human thinking**: "To compare is to be human" (Landman & Carvalho, 2017). - **Everyday vs. scientific comparisons**: - Everyday comparisons = intuitive, informal - Scientific comparisons = structured, transparent, and systematic ### Feasibility and Ethical Considerations - **Experimental vs. Comparative Method**: - The experimental method is common in natural sciences but limited in social sciences. - Social world = too "messy" for controlled experiments. - Ethical/practical constraints prevent real-world experimental conditions. - **Comparative method** offers an alternative for testing hypotheses indirectly. ### Purposes of Comparison 1. **Control** -- Testing hypotheses/theories - Comparative checking → controlling/excluding variables (large number of cases). 2. **Understand** -- Applying concepts to a case - Interpretation → using theories to analyze a single case. 3. **Explain** -- Building/revising theories - Analytical induction → adding cases to strengthen theories (small number of cases). 4. **Classify** -- Structuring empirical reality - Categorization → finding comparable units of analysis (medium number of cases). ### The Comparative Method *\"An analytical effort to exploit similarities and differences between political units as a basis for developing theory, testing hypotheses, inferring causality, and producing reliable generalizations"* (Schmitter, 1991). **Key Functions:** - Identifying common **conditions, causes, and consequences** of political events and institutions (Strom et al., 2015). - Testing theories and refining explanations through systematic case comparison. 1. 2. Compare What ------------------ ### Comparability - To compare, there must be **both similarities and differences** between entities. - **Key question**: In what ways are they comparable (or not)? - Example: Apples vs. oranges are comparable in some ways but not in others. - **Comparative analysis** relies on identifying shared and non-shared attributes. ### Classes and Classification - **Classification** = organizing political entities, structures, or phenomena based on common characteristics. - **Classes tell us** what is comparable and what is not. - Meaningful comparisons require **more differences between groups than within groups**. ### What is Comparative Politics? - **Subdiscipline of Political Science** → focuses on political structures and processes at national/subnational levels. - **Comparison as a methodological approach** → studying states, societies, and collective actors. #### Defining Politics - *Politics = "interactions through which values are authoritatively allocated for a society"* (Easton). - *Politics = "process by which people negotiate and compete in making and executing collective decisions"* (Hague et al., 2019). ### Units of Analysis *How do we structure what we compare?* #### Levels of Analysis - **International** → international organizations, regional alliances. - **National (Macro-Politics)** → political regimes. - **Subnational** → federal states, governorates. - **Regional** → electoral districts, provinces. - **Communal** → cities, towns, local politics. #### Actors & Institutions - **State vs. Non-state** / **Governmental vs. Non-governmental**. - **Branches of Government** → Legislative, Executive, Judicial. - **Organizational capacities & resources** → e.g., TNCs vs. TNAs. - **Size of actors** → number of members, influence. - **Social classes & strata**. #### Events & Processes - **Structurally occurring vs. Actor-initiated processes**. - **Binding vs. Non-binding decision-making**. - **Types of political change** → reform, revolution, civil war, regime change. - **Decision-making vs. Decision-implementation**. 1. 3. Compare How ----------------- ### Methodology vs. Method - **Methodology** = the overarching system of methods and principles used in a discipline. - *Example:* Comparison is a core **methodology** in political and social sciences. - **Method** = a specific tool or technique for testing/developing theories. - Includes **collecting evidence, formulating/testing hypotheses, and drawing conclusions**. ### Types of Methods #### **Qualitative Methods** (focus on meaning, detail, and causal links) - **Examples:** - Process tracing - Grounded theory - Qualitative content analysis - **Strengths:** - Captures complex details and contexts - Explores meanings and causal relationships - Helps refine quantitative findings - **Limitations:** Requires cultural/contextual understanding #### **Quantitative Methods** (focus on data, measurement, and generalizability) - **Examples:** - Regression analysis - Cluster analysis - Network analysis - **Strengths:** - Can handle large datasets (cases & variables) - Detects patterns and correlations - Helps identify key cases for qualitative research - **Limitations:** Requires statistical knowledge ### Research Strategies (Modes of Inquiry) - **Case Studies** → In-depth analysis of a single case - **Small-n Comparisons** → Few cases, more qualitative - **Medium-n Comparisons** → Balanced approach - **Large-n Comparisons** → Many cases, more quantitative - *General trend:* - **Few cases → Qualitative methods** - **Many cases → Quantitative methods** - **Mixed-methods approach** → Combines both for stronger analysis 1. 4 The importance of Concepts and Theory in Comparison -------------------------------------------------------- ### Concepts - **Concept** = an idea, term, or category (e.g., democracy, power) with a clearly defined meaning. - **Concept specification** = defining terms and concepts precisely. - **Theory specification** = explaining how concepts relate to one another. - *Example:* What does \"democratic\" mean? How do we define it? ### Explanation & Variables - **Explanation** = describes how a cause leads to an effect (e.g., *A causes B because A leads to q, which leads to r, which causes B*). - **Variables** = features in which cases differ. - **Independent Variable (IV)** → Cause (Explanans) - **Dependent Variable (DV)** → Outcome (Explanandum) ### Theories - **Theory** = a simplified representation of reality that organizes facts into a coherent whole. *(Lim 2016: 66)* - *Essentially, a story about how the world works.* - **Choosing a theory** = choosing a perspective on reality. - **Avoiding \"Selective Blindness\"**: - Use multiple perspectives or different methods (triangulation). - **Types of Theories**: - **Grand Theories** → Explain broad, large-scale phenomena. - **Middle-Range Theories** → Focus on specific political events or structures. ### The Role of Theory (Peters) 1. **Source of Questions & Puzzles** → Helps define what we are investigating. 2. **Reduces Complexity** → Helps determine relevant factors in comparisons. 3. **Provides General Principles** → Gives assumptions and rules for research. 4. **Links Micro & Macro Levels** → Connects individual behavior to institutions and systems. 5. **Source of Hypotheses** → Generates testable predictions. 6. **Outcome of Research** → Empirical findings can refine or create new theories. The dialogue between Data and Theory  ### Hypothesis Testing - **Hypothesis** = an unproven expectation about causal relationships between variables. - **Why compare?** - Comparison helps test **empirical relationships between variables** *(Lijphart)*. - **Scientific Knowledge Progresses Through Hypothesis Testing** - Hypotheses can come from **theory** or **educated guesses**. 2. Causal Inference and Research ================================ 2.1 Research Traditions: Empirical-Analytic vs. Interpretivist -------------------------------------------------------------- ### 2.1 Key Terms in the Theory of Knowledge - **Ontology** = The study of being. *What exists in the world?* - What assumptions do we have about the social world? - What can be studied? What does reality consist of? - **Epistemology** = The study of knowledge. *How do we know what we know?* - How do we acquire knowledge about the world? - **Methodology** = The study of methods. *How do we systematically acquire knowledge?* - Research strategies are shaped by epistemology and ontology. **Empirical-Analytic (Positivist, Naturalist)** **Interpretivist (Constructivist, Post-Structuralist)** ----------------------------------------------------------------------------- ----------------------------------------------------------------------------- **Ontology**: The real world exists independently of the observer. **Ontology**: Reality is constructed and shaped by the observer. Patterns and laws govern the world. The social world is different from the natural world. **Epistemology**: Knowledge is objective, universal, and cumulative. **Epistemology**: Knowledge is subjective, context-dependent, and relative. Truth is based on neutral facts. Knowledge depends on cultural and historical context. **Methodology**: Observation, measurement, and classification of variables. **Methodology**: Interpretation, meaning-making, and deconstruction. Deductive approach: theory → hypothesis → data collection → analysis. Inductive approach: understanding social meanings and experiences. Answers **"Why" questions** (causal explanation). Answers **"How" questions** (interpretation of meaning). ### Research Traditions & Methods - **Empirical-Analytic Research (Positivist Approach)** - Looks for patterns, regularities, and causal laws. - Uses structured data collection, large datasets, and statistical analysis. - *Example*: Studying right-wing party support through surveys and regression analysis. - **Interpretivist Research (Constructivist Approach)** - Focuses on meaning, context, and subjective experiences. - Uses qualitative methods like interviews, discourse analysis, and ethnography. - *Example*: Exploring how individuals perceive and justify their support for right-wing parties. #### Connecting Research Traditions to Qualitative & Quantitative Methods - **Quantitative Methods (Numbers & Statistics) → Empirical-Analytic Approach** - Example: Survey data on voting behavior, regression models. - **Qualitative Methods (Words & Meanings) → Interpretivist Approach** - Example: In-depth interviews on political beliefs, discourse analysis. - **Mixed-Methods Approach** → Combines both for a more comprehensive understanding. 2.3 Quantitative vs. Qualitative -------------------------------- ### Similarities Both approaches share core scientific principles: Solving scientific puzzles, answering research questions Ensuring valid descriptions and intersubjectivity (replicability & transparency) Conducting causal analysis and inference ### Key Differences **Quantitative Research** **Qualitative Research** -------------------------------------------------------------------------------------- ------------------------------------------------------------------------------- **Focus:** Populations, broad scope **Focus:** Cases, narrow scope **Causality:** Correlative & probabilistic understanding (statistical logic) **Causality:** Conditional & deterministic understanding (mathematical logic) **What it studies:** Effects of causes (**X-centered**) **What it studies:** Causes of effects (**Y-centered**) **Sample size:** Large N (many cases) **Sample size:** Small N (few cases) **Analysis:** Thin (broad but less detail), statistical tests **Analysis:** Thick (detailed but less generalizable), few statistical tests **Measurement level:** Higher (interval/ratio scales preferred) **Measurement level:** Lower (nominal/ordinal scales) **Reality capture:** Structured models, objective separation of researcher & subject **Reality capture:** Unstructured, researcher interacts with research unit **Results presentation:** Numbers & equations **Results presentation:** Words, narratives, detailed accounts ### When to Use Which Approach? **Factor** **Quantitative** **Qualitative** ------------------------------------ ---------------------------------------------- ---------------------------------------------------------- **Prior Knowledge** Well-developed theories, sufficient data Exploring new topics, little prior knowledge **Nature of Research** Straightforward, measurable variables Complex, contextual phenomena **Perspective** Researcher's external observation of reality Understanding reality from the participant's perspective **Interaction with Research Unit** No interaction, detached measurement Researcher actively interacts with subjects **Goal of Research** Generalizability, broad comparisons Deep contextual understanding 2.4 Logics of Inference (Schlussfolgerung) ------------------------------------------ ### Arguments An **argument** is a set of logically connected statements consisting of: - **Premises** → Statements assumed to be true within the argument - **Conclusion** → Claim supported by the premises **Valid Argument:** If the premises are true, the conclusion must be true.\ **Invalid Argument:** Even if the premises are true, the conclusion can be rejected. **Categorical Syllogism:** - **Structure:** Major premise → Minor premise → Conclusion - **Common form:** **If P, then Q** (conditional statement) - **Example:** "If a country is wealthy (**P**), then it will be a democracy (**Q**)."  ### Explanations **Answering "Why" Questions** Moving from **description** to **explanation** by identifying causal relationships. To explain a phenomenon: - Identify **causes** and **consequences** - Establish a **causal mechanism** between them **Example:** - **P** (Peace) - **D** (Democracy) - **E** (Economic Prosperity) - **I** (Institutions for Conflict Resolution) - Causal path: **Economic prosperity → Strong institutions → Peace → Democracy** ### Inference (Schlussfolgerung) Inference is drawing conclusions based on observed data. **Types of Inference:** - **Descriptive Inference:** Generalizing from what we observe to what we don't observe. - Example: Using a sample (survey) to infer about an entire population. - **Causal Inference:** Learning about **cause-and-effect relationships** from observed data. **How to Establish Inference?** - **Falsificationism:** Theories must generate **testable hypotheses** and be subject to rigorous testing. - Example: **Smoking-gun test** → If a suspect holds a smoking gun, they are likely guilty. If they don't, they are not necessarily innocent. ### Causality - Causality refers to a connection between two phenomena, where one leads to or produces the other. It's different from **correlation**, where two phenomena happen together without a deep connection. **Basic Idea of Causality:** - **x → y:** x causes y - **David Hume's View:** Cause precedes effect, and there is a regularity or constant connection between causes and effects. - **Ontological Assumption:** The world has order and structure, and change can be understood through patterns. #### Common Problems in Causal Analysis 1. **Spuriousness:** The omission of key variables that may explain both the outcome and other factors already identified. 2. **Fallacies:** - **Ecological Fallacy:** Inferences made from aggregate-level data to individual-level behavior. - **Individual Fallacy:** Inferences made from individual-level data to aggregate-level phenomena. #### Types of Causal Observation 1. **Data Set Observations:** - Comparable observations for applying quantitative methods. - Example: Comparing democratic quality across multiple states. - Contribution to causal inference: Foundation for correlation-based inference. 2. **Causal Process Observations:** - Focus on specific events or conditions that link cause and effect. - Contribution to causal inference: Provides insights into mechanisms and contexts. #### Types of Causality 1. **Probabilistic Causality:** - **Statistical logic:** Cause-effect relationships involve probabilities, not certainties. - The probability of an outcome (Y) increases or decreases based on the cause (X). - Methods: Regression analysis, large-n quantitative statistics. - Can assess regularities, but **not mechanisms**. 2. **Deterministic Causality:** - There is a one-to-one relationship between cause and effect. - If X occurs, Y will always follow (certainty). - Methods: Case studies, small-n comparisons (QCA), and process-tracing. - Can assess both **regularities** and **mechanisms**. #### Analyzing Regularity (Causal Statics) - „causal statics" (Heritier 2008) - based on data-set observations (Brady et al. 2004: 253) 1. **Probabilistic Causality:** - Based on **data set observations**. - The probability of Y occuring increases (or decreeases) with the level or occurence of X - Example: The probability of democracy increasing with wealth. - Method: Regression analysis. 2. **Deterministic Causality:** - **Necessary condition:** Without X, Y cannot happen. - **Sufficient condition:** If X occurs, Y will always happen. - Example: Wealth as a **necessary** condition for democracy. Ein Bild, das Text, Screenshot, Kreis, Schrift enthält. Automatisch generierte Beschreibung #### Analyzing Mechanisms and Processes (Causal Mechanisms & Process Tracing) "causal mechanisms" (Heritier 2008) „process-tracing" - focus on **links** between explanandum (dV) and explanans (iV), esp. in form of observable actions **Process Tracing:** - - - **Causal mechanism: intervening variables**  **Causal mechanism: Process tracing** Ein Bild, das Text, Diagramm, Entwurf enthält. Automatisch generierte Beschreibung **Difference Between Process and Mechanism:** Some -- but not all -- scholars see a difference: - - 3. Comparing and Miscomparing ============================= 3.1 Conceptualisation and Operationalisation -------------------------------------------- +-----------------------------------+-----------------------------------+ | CONCEPTUALISATION | OPERATIONALISATION | | | | | = definition of meaning | = Operational definition | +===================================+===================================+ | to ask questions, formulate | basis for indicators and | | theories | measurement | +-----------------------------------+-----------------------------------+ | clearly defining and specifying | translating abstract concepts | | abstract concepts | into concrete and measurable | | | variables or indicators | +-----------------------------------+-----------------------------------+ | "What is the difference between | "How can we measure the concepts? | | the two definitions?" | | +-----------------------------------+-----------------------------------+ 3.2 Classifications and Typologies ---------------------------------- []{#_Toc189652035.anchor}*What is Classification?*\ Classification is the process of ordering entities (like concepts or objects) into distinct groups. These groups must be **mutually exclusive** (no overlap) and **collectively exhaustive** (cover all possibilities). - This helps to make clear what you\'re talking about and allows you to make specific statements about a group. - **Example:** Classifying different types of welfare states (e.g., conservative welfare state). []{#_Toc189652036.anchor}*What is a Typology?*\ A **typology** is a more specific form of classification, where objects are grouped based on **two or more characteristics**. Each \"type\" in the typology represents a combination of those characteristics. - **Example:** Aristotle's six types of rule or Dahl's distinction between **democracy** (ideal type) and **polyarchy** (real type).  #### Ideal vs. Real Types (as explanation of a typology by Dahl) - **Ideal Type:** A broad, abstract concept not meant to represent actual cases but to highlight key features. - **Example:** Democracy as an ideal type, which may not exist perfectly in reality. - **Real Type:** A type based on the combination of observable characteristics, used to classify real-world cases. - **Example:** Polyarchy, which reflects real-world democracies. Ein Bild, das Text, Screenshot, Schrift, Informationen enthält. Automatisch generierte Beschreibung ### Requirements for Typologies 1. **Mutual Exclusiveness:** Each case must fit into only **one** category. 2. **Collective Exhaustiveness:** All cases must fit into one of the categories, leaving **none** out. ### Conceptual Hierarchies and Typology Strategies **Types of Typologies:** 1. **Classical Typologies (Kind Hierarchies):** - A **nested hierarchy** of concepts, where subordinate concepts are kinds of a higher-level category. - **Example:** Different types of welfare states, where each type has its own unique characteristics. 2. **Radial Typologies:** - Concepts are organized around a central idea, with secondary categories radiating out from it. - **Example:** Different forms of democracy, where they vary in how close or distant they are from the ideal form of democracy. **What Typologies Provide Us With** 1. **Reduction of Complexity:** Simplifies complex ideas or cases into manageable groups. 2. **Clustering Information:** Helps to organize information in a structured way. 3. **Building Blocks for Theory Development:** Typologies can help generate and test theoretical ideas. **Common Problems in Conceptualizing for Comparative Research** 1. **Parochialism:** - Using **ad-hoc terms** or concepts that lack shared, theoretical definitions. - Can lead to missed opportunities for comparison. - **Example:** Calling a government in the U.S. a \"coalition government\" when it doesn't meet the typical definition. 2. **Misclassification:** - Incorrectly categorizing a case. - **Example:** Labeling Turkey as a democracy when it doesn\'t meet the criteria. 3. **Degreeism:** - Treating **differences in kind** as **differences in degree** (e.g., thinking North Korea is "just less democratic" rather than an outright autocracy). 4. **Conceptual Stretching:** - Using **sloppy definitions**, causing concepts to be so broad that they lose their analytical value. - **Example:** Calling any organization linked to the Queen Consort of Jordan a \"civil society\" group when it doesn\'t fit the criteria for civil society. 3.3 Research Designs -------------------- ### What is Research Design? Research design is the plan that turns **research questions** into actionable **projects**. It helps to choose the best **methods** to gather evidence and provides the structure for answering those questions. - It is a **toolbox** that guides the process of research, ensuring you develop a **clear operational plan** and collect **valid, accurate** data to answer your research question. **Functions of Research Design** 1. Develop an **operational plan** for conducting research. 2. Ensure the methods used lead to **valid**, **objective**, and **accurate** results. ### Components of Research Design 1. **Purpose(s):** What is the research aiming to do? 2. **Conceptual Framework:** The theory or ideas behind your research. 3. **Methods:** How will data be gathered (qualitative, quantitative)? 4. **Sampling Strategy:** How will you choose the cases to study? ### Models of Research Designs 1. **Linear Model of Research:** - A **structured** approach to research, starting with a question and following a **logical sequence** (observation, theory, methods, analysis).  2. **Inductive Research:** - Begins with **observation** and uses it to develop theories. - Example: Studying electoral results in Germany and developing a theory about political socialization based on patterns in interviews and personal experiences. - **Observation:** Electoral results in Germany. - **Units:** Individuals. - **Indicators:** Qualitative data on politics, democracy, socialization. - **Theory:** Phenomenology (political lifeworld). - **Methods:** Interviews, content analysis. - **Analysis:** Coding patterns and behaviors. - **Explanation:** Distinct political lifeworlds lead to different voting behaviors. 3. **Deductive Research:** - Starts with a **theory** and uses it to test specific hypotheses or implications. - Example: Applying **cleavage theory** to study voting behavior in Germany and testing whether traditional social categories (class, religion) still explain voting patterns. **Deductive Example:** - **Observation:** Electoral results in Germany. - **Theory:** Cleavage theory (class, urban/rural, religion). - **Method:** Survey research (large samples). - **Units:** Individuals. - **Indicators:** Voting behavior, income, residence, religion. - **Analysis:** No clear patterns in traditional cleavages. - **Explanation:** New cleavage? (Libertarian vs. Traditional). ### Choosing the Right Research Design 1. **Purpose & Epistemology:** - **Analytical:** Aims to explain or generalize findings. - **Normative:** Focused on judging or suggesting changes. - **Critical:** Seeks to understand, deconstruct, or describe reality. 2. **Sequencing Theory and Empirical Reality:** - Moving between **theory development** and **testing** in different orders, or applying theory in practical settings. 3. **What is the Relevant Universe?** - Consider the **space** (where) and **time** (when) of your study. This helps decide **case selection**, **sampling**, and **data gathering methods**. 3.4 The Ethical Dimension of Research ------------------------------------- **How Research Ethics Became Central** *[Nuremberg Code (1949):]*\ Focused on protecting human subjects in research. - **Key principles**: - Voluntary consent is essential. - No harm or risk of injury. - Research risk must be justified. - Qualified personnel and protective environment. #### Belmont Report (1979) - Introduced core ethical principles for research: - Treat individuals as autonomous agents. - Protect those with diminished autonomy (e.g., children, prisoners). - **Application**: Ensure **informed consent** -- provide information, ensure understanding, and voluntary participation. 1. Information 2. Comprehension 3. Voluntarines - Do no harm, maximize benefits, minimize risks. - **Application**: Assess and justify the **risks and benefits** of the research. - nature and scope of the risks and benefits - systematic assessment of risks and benefits - assessment of the justifiability of research - Ensure **fair distribution** of benefits and burdens of research. - **Application**: Fair selection of research subjects and enrollment processes. - social justice - individual justice ### Considerations for Ethical Research - **Things to avoid**: 1. Harm to participants 2. Lack of informed consent 3. Invasion of privacy 4. Deception #### Guidelines for ethically correct behavior: 1. **Obligation to truth**: Be transparent and honest. 2. **Transparency**: Be open about methods and findings. 3. **Reflection**: Continuously reflect on your research practices. 4. **Consult others**: Engage with colleagues or experts for advice and feedback. #### Broader Ethical Perspectives in Research - **Academic Integrity**: Adhere to standards of honesty and transparency. - **Research Data Management**: Follow proper procedures for handling data. - **Power Relations**: Be mindful of power imbalances within research institutions and with funders. - **Researcher's Role**: Consider how factors like **gender**, **race**, and **class** affect your research and interactions. ### Ethical Dilemmas and Decisions - **Informed Consent**: In certain cases, such as minimal risk or when research might increase risk, **altering or forgoing consent** may be justified. Always justify and explain deviations in publications. - **Deception**: In cases involving powerful actors or sensitive topics, **deception** might be permissible, but it must be carefully managed (e.g., informed after the study, debriefing, allowing data withdrawal). - **Ethical Challenges in Theoretical Research**: Ethical considerations are just as relevant in **theoretical** research as they are in **empirical** studies. ### Making Ethical Choices Easier - **Check guidelines**: Refer to codes of ethics by professional associations. - **Plan ahead**: Anticipate potential challenges in your research design. - **Ask others**: Consult colleagues or ethics review boards when uncertain. - **Balance theory and practice**: Sometimes, ethical principles may conflict with practical needs, and careful reflection is required. ### APSA Guidelines on Ethics 1. **Respect Autonomy**: Researchers must respect participant autonomy and consider their well-being. 2. **Responsibility**: Researchers are responsible for ethical decisions, not just review boards. 3. **Deviations from Standards**: If ethical guidelines are deviated from, researchers must justify this in their publications. 4. Collecting Data ================== 4.1. Basics of Data Collection ------------------------------ - **Definition**: Data is any evidence that serves as the basis for understanding and explanation. - **Forms of Data**: - **Numerical** (e.g., statistics, measurements) - **Textual** (e.g., documents, transcripts) - **Visual** (e.g., photos, videos) - **Olfactory** (e.g., smells, chemical traces) - **Auditory** (e.g., recordings, sounds) - **Artifactual** (e.g., historical objects) - **Data Selection**: - **Justify selection process** (sampling strategy). - **Different data types and methods** can be combined. - **Results of collection**: - **Qualitative** (e.g., interviews, field notes, documents). - **Quantitative** (e.g., surveys, statistical data). - **Collected data requires analysis**. 4.2 How to Collect Data ----------------------- **Obtrusive vs. Non-Obtrusive Methods** - **Obtrusive** (involves interaction with subjects): - **Interviews** - **Surveys** - **Experiments** - **Ethnography** - **Non-Obtrusive** (no direct interaction): - **Distant observation** - **Archives & documents** ### Primary vs. Secondary Data - **Primary Data** (collected by the researcher during the study): - **Interviews & Surveys** - **Observations** - **Experiments & Measurements** - **Secondary Data** (pre-existing data used for analysis): - **Official statistics** - **Documents (e.g., reports, archives)** - **Transcripts from previous interviews** ### Methods of Data Collection **Qualitative Data Collection** - **Human Subjects**: Interviews, focus groups - **Documents**: Analyzing written records - **Field Research**: (Participant) observation **Quantitative Data Collection** - **Human Subjects**: Surveys, large-scale questionnaires - **Big Data**: Mining digital information - **(Quasi-)Experiments**: Controlled studies ### The S-P-R Model Stimulus → Person → Response - is a framework used to explain how individuals react to different stimuli. It highlights the role of personal factors in shaping responses. 4.3 Primary Data - Experiments, surveys, interviews, observation ---------------------------------------------------------------- ### 1. Experimental Design - **Goal**: Isolate causal effects - **Controlled setting**: Laboratory-like environment - **Key elements**: - Control of stimulus and variables - Variation of stimulus → Measure effect of variation **Example: The Milgram Experiment** - **Question**: Why do normal people obey authority to the point of harming others? - **Method**: Participants instructed to administer shocks to a learner - **Findings**: Obedience increases under authority pressure ### 2. Survey Experiments - Surveys = Standardized questionnaires with limited (closed) response options - **Purpose**: Measure mass opinions, especially in politics - **Uses standardized questionnaires with closed response options** - **Can be conducted via**: - In-person - Phone - Mail - Online #### Types of Survey Implementation 1. **Cross-Sectional** -- One-time survey 2. **Panel** -- Repeated surveys with the same respondents 3. **Pooled Time-Series** -- Repeated surveys with different respondents from the same population #### Factors Affecting Survey Quality - Response rates & sample representativeness - Time & cost - Possible biases (e.g., social desirability, interviewer effects) #### Strengths & Weaknesses **Strengths** - Tracks opinion/behavior changes over time - Compares attitudes across groups **Weaknesses** - Limited to predefined questions - Ineffective for complex topics - Response errors (e.g., non-response, social desirability bias) ### 3. Qualitative Interviews - **Goal**: Understand actions, events, and knowledge from participants\' perspectives - **Characteristics**: - **Asymmetric** -- Different roles for interviewer and interviewee - **Interactive** -- Communication process is key - **Interdependent** -- Both rely on each other #### Key Methodological Considerations - **Openness** -- Flexible, adapts as research progresses - **Flexibility** -- Follows natural flow of conversation - **Life as a Process** -- Focuses on personal experiences - **Grounded Theory** -- Builds theories from collected data - **Explication** -- Findings emerge during the study #### Steps in Conducting Interviews 1. **Preparation** - Define topics and questions - Select participants and obtain access 2. **During the Interview** - Create a comfortable environment - Stay engaged but neutral 3. **After the Interview** - Take notes immediately - Transcribe recordings #### Types of Interview Questions 1. **Introductory** -- Open-ended, sets the stage 2. **Follow-up** -- Clarifies earlier responses 3. **Probing** -- Encourages deeper insights 4. **Specifying** -- Asks for details 5. **Direct** -- Explicit questions 6. **Indirect** -- More subtle, avoids direct confrontation 7. **Silence** -- Allows participant to think and respond 8. **Interpreting** -- Asks for confirmation of understanding #### Types of Interviews   **Comparing Data Collection Methods** **Method** **Strengths** **Weaknesses** ----------------- ---------------------------------- --------------------------------- **Experiments** Causal analysis, high control Artificial setting **Surveys** Large samples, measurable trends Limited depth, potential biases **Interviews** Rich insights, flexible Time-consuming, small samples 4.4 Secondary Data ------------------ ### Ex Post Measures Secondary data includes: - **Text** (documents, books, reports) - **Sound** (recordings, interviews) - **Film** (videos, movies, news reports) - **Other artifacts** (photos, historical records) **Key Considerations:** - Research is **unobtrusive** (researcher does not influence data creation). - Always check for: - **Representativity** (Is the data representative of the population?) - **Comparability** (Can it be compared across studies?) - **Measurement Errors** (Are there inconsistencies or biases?) - **Appropriateness** (Does it fit the research question?) ### Core Methods #### Document Study - **Descriptive** (basic summary of content) - **Categorical** (grouping data into themes) - **Exploratory** (identifying new patterns) - **Comparative** (comparing different sources) - **Biographical Research** (life histories, personal accounts) #### Content Analysis - **Quantitative Approaches:** - Description - Valence Analysis (evaluating positive/negative tones) - Contingency Analysis (relationships between variables) - Contextual Analysis (interpreting meanings within a context) - **Qualitative Approaches:** - Summative (identifying key themes) - Inductive Category Construction (developing themes from data) - Explicating (detailed interpretation, broad or narrow) - Structuring (organizing information systematically) - Objective Hermeneutics (deep interpretation of meaning) #### Text Analysis - **Conversation Analysis (CA)** (studying spoken interactions) - **Discourse Analysis** (examining language use) - **Hermeneutics** (interpreting meanings in texts) ### Opportunities and Advantages - **Cumulation & Pooling** (combining datasets for better insights) - **Analyzing More Variables** (increased scope) - **Pooled Time Series** (merging multiple studies over time) - **Country Comparisons** (cross-national analysis) - **Data Aggregation** (multi-level analysis) - **Process-Simulation** (controlling variables for testing models) - **Construct Validity** (comparing indicators across studies) ### Problems and Shortcomings - **Data Availability Issues** - Some data is not published. - Delays in data publication. - **Theoretical Framework Limitations** (researcher must assess fit with their study). - **Quality of Studies & Data Gathering** - Differences in methods. - Incomplete or poor documentation. - **Common Errors in Secondary Data** - **Measurement Errors** (inconsistent definitions, inaccurate recording). - **Decontextualization of Questions** (losing original meaning). - **Interviewer & Situational Effects** (biases in data collection). - **Instrument Effects** (changes over time affecting consistency). - **Intercultural & Cross-Linguistic Issues** (differences in wording, cultural biases). 5. Single and Small n-Analysis ============================== 5.1. Cases and Case Studies --------------------------- ### What is a Case? A **case** is a **spatially and temporally defined phenomenon** that is studied at a single point in time or over a period. It represents the subject of study when trying to explain an event or process. (Gerring, 2007) ### What is a Case Study? A **case study** investigates a specific case in-depth. This requires: - **Defining its boundaries** (spatial and temporal). - **Clarifying what it represents** (*What is this a case of?*). ### Purposes of a Case Study (Van Evera, 1997) - **Testing theories** - **Creating theories** - **Identifying antecedent conditions** - **Testing the importance of antecedent conditions** - **Explaining cases of intrinsic importance** ### Strengths and Weaknesses of Case Studies **Weaknesses:** 1. **Limited control over third variables** - Need to consider covariance between IV (independent variable) and DV (dependent variable). - Selecting extreme values on the study variable can help mitigate this. 2. **Limited generalizability** - Single-case studies might miss rare conditions. - Comparing with other cases improves external validity. **Strengths:** 1. **Testing unique predictions that provide strong evidence for or against a theory.** 2. **Providing insights into the causal process** (How is the dependent variable caused?). ### Approaches to Explanatory Case Study Research (Blatter & Haverland, 2012) #### Co-Variational Approach (COV) -- \"Effects of Causes\" - **Focus:** Independent Variables (IVs) - **Key Question:** *Do different values of X lead to different outcomes?* - **Case Selection:** Multiple cases - **Data Generation:** - Observation - Measuring variables and indicators - **Data Analysis:** - Covariation between X and Y (IV and DV) - Controlling for other variables - **Generalization:** Statistical - **Example:** *Does government reorganization reduce public spending?* #### Causal-Process Tracing (CPT) -- \"Causes of Effects\" - **Focus:** Understanding what makes Y possible - **Key Question:** *Which factors or causal configurations lead to a specific outcome?* - **Case Selection:** - Few cases, chosen based on outcome relevance - Accessibility of data - **Data Generation:** - Temporal and spatial sequence of events - \"Smoking gun\" evidence (key proof of causality) - **Data Analysis:** - Identifying causal chains and necessary/sufficient conditions - **Generalization:** Possibilistic (showing what is possible) - **Example:** *What conditions lead to social revolution?* #### Congruence Analysis (CON) -- \"Theory-Centered\" - **Focus:** Comparing theories to find the best explanation - **Key Question:** *Which theoretical approach provides better insight?* - **Case Selection:** - Based on relevance in scientific discourse - Cases with different likelihoods for each theory - **Data Generation:** - Testing theoretical expectations - Confirming or contradicting theories - **Data Analysis:** - Comparing case-study findings with theoretical predictions - **Generalization:** Theoretical (improving theories) - **Example:** *Is Liberal Intergovernmentalism the best explanation for European Integration?* ### Case Studies and Theories #### Generating Theory - **Hypothesis generating** - **Heuristic** (exploratory) - **Archetypical cases** - **Uses inductive inference** (building theories from observations) #### Testing Theory - **Theory confirming or disproving** - **Plausibility probing** (testing assumptions) - **Deviant case analysis (unexpected cases challenging theory)** - **Uses deductive inference** (testing theories with data) #### Generating & Testing Theory - **Disciplined configurative studies (applying theories to cases systematically)** - **Representative cases** - **Combines induction and deduction** ### Case Selection Criteria (Van Evera, 1997) **Reasons to Select a Case:** 1. **Data richness** (availability of high-quality data) 2. **Extreme values on a variable** 3. **Large within-case variance** (variation in key variables) 4. **Divergent predictions from competing theories** 5. **Similarity to the context in which the theory was developed** 6. **Prototypical background characteristics** 7. **Matched for controlled cross-case comparison** 8. **Outlier case** (exceptional, unexpected cases) 9. **Intrinsic importance** (politically/socially significant cases) 10. **Good for replicating previous studies** 11. **Allows for new types of tests** (e.g., process tracing instead of a congruence test) ### Avoiding Selection Bias - **Ensure variation in the dependent variable** - **Compare multiple cases for broader context** - **Use strong theoretical foundations** - *\"Selecting cases on the dependent variable is a taboo that bedevils several major qualitative studies.\"* (Geddes, 1990) 5.2 Process Tracing ------------------- - Process tracing examines diagnostic evidence to refine causal inference. It assesses causal chains by testing necessary and sufficient conditions. (Collier, 2010) ### Process Tracing Tests These tests assess different levels of uniqueness and certitude to evaluate causal mechanisms. Ein Bild, das Text, Screenshot, Schrift, Zahl enthält. Automatisch generierte Beschreibung #### Straw-in-the-Wind Test - **Low uniqueness, low certitude** → Indecisive both ways - Example: If a military officer led a coup, he might have made a phone call to relevant units. #### Hoop Test - **High certitude, no uniqueness** → Failing the test disproves a theory, but passing does not strongly confirm it. - Example: Was the accused in the state on the day of the murder? If not, they are innocent. If yes, it does not prove guilt. #### Smoking-Gun Test - **High uniqueness, no certitude** → Passing strongly supports a theory, but failing does not disprove it. - Example: A suspect holding a smoking gun after a shooting is strong evidence of guilt, but the absence of a smoking gun does not prove innocence. #### Doubly-Decisive Test - **High uniqueness, high certitude** → Passing confirms the theory, failing disproves it. - Example: A security camera recording bank robbers' faces proves guilt or innocence. 5.3 Comparative Case Studies ---------------------------- ### Controlled Comparisons #### Most Similar Cases Design (MSCD) - **Method of Difference**: Cases are similar in many aspects except for the dependent variable (outcome), allowing analysis of differing independent variables. - **Key Question:** What explains the difference in outcomes? #### Most Different Cases Design (MDCD) - **Method of Agreement**: Cases differ in most respects but share the same outcome, suggesting a common independent variable. - **Key Question:** What explains the similarity in outcomes?  **Example: Luebbert's (1991) Study on Regime Types** - **Research Question:** What explains the emergence of liberalism, social democracy, and fascism in interwar Europe? - **Key Finding:** Class alliances determined regime types: - **Liberalism:** Strong middle class vs. weak working class - **Social Democracy:** Alliance between working class and middle peasantry - **Fascism:** Alliance between middle class and middle peasantry - **Design Used:** MDCD -- different country conditions but similar regime outcomes. ### Defending Small-n, Controlled Comparisons (Slater & Ziblatt, 2013) - Controlled comparisons can provide **both internal and external validity**: - **Internal Validity:** Ensures correctness within studied cases. - **External Validity:** Ensures correctness when applied to other cases. - Standards for strong controlled comparisons: 1. Use **general variables or mechanisms**. 2. Seek **representative variation**. 3. Select cases that **maximize control over alternative explanations**. 6. Medium n-Analysis / QCA ========================== 6.1 Qualitative Comparative Analysis (QCA) ------------------------------------------ **Basic Principles** - **Set-theoretic approach**: Uses Boolean algebra (Truth tables, Venn diagrams) to analyze combinations of factors leading to outcomes. - **Key focus**: Identify necessary or sufficient conditions for an outcome, using combinations of factors, not individual variables. - **Equifinality**: Different conditions can lead to the same outcome. - **Asymmetric causality**: The presence and absence of a phenomenon require separate analyses. **Main Questions in QCA:** 1. Under what conditions does outcome *y* occur? 2. Which configurations of variables (e.g., *x, x\'*) lead to *y*? ### **Necessary and Sufficient Conditions** +-----------------------+-----------------------+-----------------------+ | **Sufficient | **Necessary | **Necessary & | | condition** | condition** | Sufficient | | | | Condition** | +-----------------------+-----------------------+-----------------------+ | - **a condition is | - **a condition is | - **a condition is | | sufficient, if it | necessary, if it | necessary and | | leads to the | always exists | sufficient, if | | outcome in every | when the cóutcome | there is identity | | single case in | is present; does | of the two sets x | | the comparative | not imply a | and y** | | study** | causal | | | | direction** | - **Formal-logical | | - **Formal-logic | | notation:** | | notation:** | - **Formal-logic | | | | notation:** | **X ←→ Y** | | **X → Y (if x, then | | | | y)** | **X ← Y** | **(If x then and only | | | | then y)** | | - **asymmetric | - **does not imply | | | causality** | a causal | | | | direction!** | | | | | | | | - **asymmetric | | | | causality** | | +-----------------------+-----------------------+-----------------------+ ### \"Multidimensional\" Conditionality - **INUS Cause**: An insufficient but necessary part of a condition, which is itself unnecessary but sufficient for the outcome. - **SUIN Cause**: A sufficient but unnecessary part of a factor that is insufficient but necessary for the outcome. ### Truth Tables - **Purpose**: Analyze similarities and differences between cases. - Identify contradictory rows (same conditions, different outcomes). - **Empirical spread**: Identify which combinations of conditions are observed or missing. Ein Bild, das Text, Screenshot, Zahl, Schrift enthält. Automatisch generierte Beschreibung ### Software for QCA: - **Fuzzy QCA Software**: Software for Stata and R packages (e.g., SetMethods, QCA for crisp and fuzzy sets). - **Resources**: - [COMPASS Software Overview](http://www.compasss.org/software.htm) - [Tosmana Software](http://www.tosmana.net) - [fsQCA Software](http://www.fsqca.com) 6.2 Qualitative Content Analysis (QCA) -------------------------------------- **Overview**: - QCA is a methodical, empirical analysis of texts within their communication context. - It focuses on identifying and describing linguistic characteristics of texts to draw conclusions about broader social contexts. ### Basic Forms of Coding and Interpretation 1. **Reduction**: Summarizing the material to preserve essential content while creating a comprehensive overview. - **Techniques**: Summarizing, inductive category formation. - Example: - Original: "Democracy is conflict-prone, but that's also the creative aspect..." - Paraphrased: "Democracy is conflict-prone." - Generalized: "Democracy is conflictive." 2. **Explication**: Providing additional information to clarify or interpret text components. - **Techniques**: Narrow or broad context analysis. - Example: - Text: "We should produce a citizen's report..." - Broad Explication: This is related to citizen participation in democracy. - Paraphrase: "Citizens develop solution proposals." 3. **Structuring**: Organizing text into categories based on predefined criteria. - **Techniques**: Assigning nominal or ordinal categories. - Example: - Texts like "Democracy is a fight" (highly conflictive) or "It's about negotiating" (consensual) are categorized accordingly.  ### Units of Analysis 1. **Coding Unit**: The smallest portion of text that can be categorized. 2. **Context Unit**: The largest portion of text that can be categorized. 3. **Recording Unit**: Determines the text sections that are analyzed using a category system. ### Structuration in QCA - **Goal**: Identify structures within the material by defining categories, anchor samples, and coding rules. - **Steps**: 1. Define the research question. 2. Establish characteristics and categories. 3. Create coding guidelines. 4. Apply and revise coding based on categories. ### Quality Criteria in QCA 1. **Validity**: - **Semantic Validity**: Accuracy of interpretation. - **Sampling Validity**: Representativeness of the sample. - **Correlative Validity**: Consistency with related concepts. - **Predictive Validity**: Accuracy of predictions. - **Construct Validity**: Ensuring concepts are measured appropriately. 2. **Reliability**: - **Stability**: Consistency over time. - **Replicability**: Ability to repeat the analysis with similar results. - **Accuracy**: Precision in categorizing text. Ein Bild, das Text, Visitenkarte, Screenshot, Schrift enthält. KI-generierte Inhalte können fehlerhaft sein. ### Software for Content Analysis - **Resources**: - [Text Analysis Info](http://www.textanalysis.info/) - [ATLAS.ti](https://atlasti.com/) - [MAXQDA](https://www.maxqda.com/) - [NVivo](https://www.qsrinternational.com/nvivo/nvivo-products) 7. Large n-Analysis =================== 7.1 Procedures to test or Discover Structure in Data ---------------------------------------------------- +-----------------------------------+-----------------------------------+ | **Testing** | **Discovering** | +-----------------------------------+-----------------------------------+ | **Regression analysis** | **Principal component and factor | | | analysis** | | → How strong is the impact of an | | | x on a y? | → Are there non-observable | | | structures that explain a | | → Formula for describing how | variance of variables? | | variables respond to changes in | | | others | | +-----------------------------------+-----------------------------------+ | **Causal analysis** | **Cluster analysis** | | | | | → based on regression models | → assigning objects into groups, | | | so that objects from the same | | → Which x are causal for the | cluster are more simililar to | | change of y? | each other (---\> classification) | | | | | | → how can we group subjects so | | | they differ minimally inside of | | | the group but maximally between | | | groups? | +-----------------------------------+-----------------------------------+ | **(Multiple) Analysis of Variance | **Multidimensional scaling** | | (MANOVA)** | | | | → On the basis of which | | → Do certain nominally scaled x | dimensions are objects considered | | have an influence on values of | similar? | | metric variables? | | | | | | → Do they in different samples | | | differ significantly? | | +-----------------------------------+-----------------------------------+ | **Discriminant analysis** | **Correspondence analysis** | | | | | → Which combination of | → Is there an inner structure in | | characteristic values connects / | data matrices? | | separates the observed groups? | | +-----------------------------------+-----------------------------------+ | **Contingency analysis** | | | | | | → How often do items in a text | | | appear together? | | | | | | → Is the frequency non-random? | | +-----------------------------------+-----------------------------------+ | **Conjoint analysis** | | | | | | → What are the overall | | | preferences of a person with | | | regard to an object or a | | | situation? | | +-----------------------------------+-----------------------------------+ **Testing**: Methods that check the impact or significance of a relationship. - **Regression Analysis**: Measures how much an independent variable (X) affects a dependent variable (Y). - **Causal Analysis**: Focuses on identifying causal relationships using regression models. - **ANOVA (Analysis of Variance)**: Examines if nominal variables affect metric variables across different samples. - **Discriminant Analysis**: Identifies combinations of variables that separate observed groups. **Discovering**: Methods that uncover hidden structures in data. - **Principal Component/Factor Analysis**: Finds underlying structures explaining the variance between variables. - **Cluster Analysis**: Groups similar objects or subjects based on certain characteristics. - **Multidimensional Scaling**: Measures similarity based on various dimensions. - **Correspondence Analysis**: Unveils structure within data matrices. - **Contingency Analysis**: Examines how often items co-occur in text and whether it\'s random. - **Conjoint Analysis**: Analyzes preferences of individuals regarding objects or situations. 7.2 Multivariate Analysis: Different Research Questions ------------------------------------------------------- - **Regression analysis** - What effect does an independent variable have on the occurrence of the observed henomenon x? - Probabilistic variable logic - It\'s about probabilities - structural testing - **PCA and Factor analysis** - Is there a cause for a shared (or, co-) variance of (un)dependent variables? - Probabilistic Variable Logic - It\'s about background variables. - Discovering structures, if necessary, structure testing - **Cluster analysis** - How similar are the different n regarding specific variables? - Probabilistic variable logic - It\'s about distances / similarities - Discovering structures ### Regression Analysis - **Purpose**: Investigates the effect of an independent variable (X) on a phenomenon (Y) using probability logic and structural testing. #### Types of Regression: ##### Linear Regression: - Examines a relationship between one independent variable (X) and a dependent variable (Y). - a: constant - b1: slope (indicates how Y changes as X changes) **Estimate of a bivariate linear regression** - e = error term. There is unexplained variation. The larger this part is, the worse is the proximation of the linear regression - y\>i is the predictor of the real value yi  ##### **Multivariate Regression**: - Involves multiple independent variables. - More regressors increase R² (model fit), but they also increase standard error and reduce the reliability of the estimate. - **Adjusted R²**: Reflects model fit after accounting for the number of regressors used. - **Rule of Thumb**: Use as few variables as possible while explaining the most. (Ockham's Razor) #### Requirements for Regression: 1. **Metric scale** for variables (e.g., continuous numbers). 2. **Linear relationship** between variables. 3. **No outliers**. 4. **No heteroscedasticity** (variance inconsistency), **autocorrelation** (correlation over time), or **multi-collinearity** (high correlation between predictors). #### Key Regression Metrics - **R² (Coefficient of Determination)** - **What it tells you**: How much of the total variation in the dependent variable (DV) can be explained by the model. - **Formula**: R² \* 100 gives the percentage of variance explained by the model. - **Adjusted R²** - **What it tells you**: What R² would be if you took a new sample with the same number of cases and ran the same regression. It accounts for the number of predictors in the model. - **F-Test and Significance** - **What it tells you**: Checks if the regression model is significant. - **Threshold**: If the p-value is **less than 0.05**, the relationship is likely **significant** (a linear relationship exists). - **Degrees of Freedom (df)** - **What it tells you**: The more degrees of freedom, the more reliable the regression. - **Formula**: df = Sample size (n) - Number of independent variables (IV) - 1. - **Rule of Thumb**: You need at least **10 degrees of freedom** for the residuals. - **Regression Coefficient (for each variable)** - **What it tells you**: The change in the dependent variable (DV) for every one-unit change in the independent variable (IV). - **Beta** - **What it tells you**: The strength of the relationship between an independent variable (IV) and the dependent variable (DV). - **Higher Beta** means the IV has a stronger influence on the DV. - **Significance Level** - **What it tells you**: The probability that the relationship between the IV and DV is due to chance. A low significance (usually below **0.05**) indicates a real effect. - **Tolerance** - **What it tells you**: The correlation between the i-th variable and other independent variables in the model. - **Formula**: Tolerance = 1 - R². - **Key Points**: - Tolerance **\< 0.1** suggests **collinearity** (predictors are too similar). - Tolerance **\< 0.01** means **high collinearity**. - **VIF (Variance Inflation Factor)** - **What it tells you**: The degree of collinearity between independent variables. - **Formula**: VIF = 1 / Tolerance. - **Key Points**: - A high VIF means **high collinearity**. - Threshold value: **VIF \> 10** suggests problematic collinearity. #### Example: How to Explain Economic Development? - **Modernization Theory**: - **Idea**: Countries with better human development (education, health, etc.) tend to have stronger economies. - **Indicator**: Human Development Index (HDI). - **Classical / Neo-Classical Theory**: - **Idea**: The lower the taxes or state involvement, the more businesses are motivated to produce, leading to better economic development. - **Indicator**: Fiscal Freedom (how much control the government has over the economy). - **Endogenous Growth Theory (Romer)**: - **Idea**: If a country spends more on research and development (R&D), it leads to more knowledge, which in turn boosts the economy. - **Indicator**: R&D Expenditures. - **Creative Class Theory (Florida)**: - **Idea**: More advanced communication and a highly connected society lead to greater economic development. - **Indicator**: Internet user ratio (how many people use the internet). - **Keynesian Theory**: - **Idea**: The more the government spends and invests in the economy, the better the economic development. - **Indicator**: Public spending. - **Neo-Institutionalism**: - **Idea**: Countries with a well-established legal system and secure property rights have better economic development. - **Indicator**: Rule of Law (the strength of the legal system). - **Democracy Theory**: - **Idea**: Democracies tend to have stronger economies because they protect civil rights and encourage production. - **Indicator**: Type of regime (democracy vs. dictatorship). #### Rules for a Good Regression Analysis 1. **Define the Problem and Hypothesis** - Clearly state what you are investigating and formulate specific hypotheses. 2. **Identify Variables** - Specify your **dependent** (what you're measuring) and **independent** variables (what you think influences the dependent variable). 3. **Ensure a Large Enough Sample** - Your sample should be big enough (at least 10 degrees of freedom). 4. **For Bivariate Regression** - Use a **scatterplot** to check the relationship between the two variables. 5. **For Multivariate Regression** - Check the **correlation matrix** and **scatter plot matrix** for issues like: - **Multicollinearity** (when independent variables are too related) - **Heteroscedasticity** (when variance of errors changes) 6. **Check Regression Coefficients** - Make sure the **coefficients** (values showing the relationship between variables) match the expected direction (positive or negative). 7. **Verify Theoretical Assumptions** - Ensure your data follows the assumptions required for the model (like linearity, no outliers, etc.). 8. **Check Model Quality** - Use tests like **R²**, **F-test**, **t-test**, and the **Durbin-Watson test** to check if the model is good. 9. **Compare with Reality** - Do the results make sense? Ensure the findings align with what is known or expected in reality. 7.3 Factor Analysis ------------------- - Starting point: a large number of variables and observations. It is not known whether and in what way they are related, but they are assumed to represent certain complex facts **Purpose** - **Data Reduction**: Reduce many variables into a few factors. - **Interpretability**: Make large datasets easier to understand. - **Discover Hidden Structures**: Identify unobserved factors that explain patterns in the data. ### Factor Analysis Steps: 1. **Descriptive Statistics** - Analyze mean, standard deviation for your data. 2. **Correlation Matrices** - Look for significant correlations between variables. - Check **sphericity** (how well variables correlate) and **sampling adequacy**. 3. **Factor Extraction** - Use **principal components** to form factors. - Check the **screen plot** and **eigenvalue** to assess the explanatory power of factors. 4. **Rotation** - Adjust the factors to improve interpretation by \"rotating\" the factors in a multidimensional space. 5. **Factor Scores** - These scores are new variables derived from the factors, and can be used in further analysis. **Key Terms:** - **Latent Variables**: Unseen factors inferred from the observed data (e.g., \"service quality\" behind multiple related reviews). - **Factor**: A variable derived from the correlation of multiple variables. **Example: Economic Development** Factor analysis could be used to identify underlying factors that explain **economic development**, such as: - Variables: GDP, education levels, R&D spending, rule of law, etc. - **Latent factors**: Government policies, economic infrastructure, innovation capacity. #### Interpretation: - **Challenge**: Interpreting factor analysis results can be difficult or ambiguous. - **Goal**: Use the factors to better understand and explain complex data. - **Theoretical Insight**: Sometimes factors align with real-world structures, helping with deeper analysis. 7.4 Cluster Analysis -------------------- ### Objective: - Group similar cases or variables based on specific characteristics. - Helps **reduce complexity** and **discover patterns** in data. **Core Question:** - How can we measure and define similarity between objects (cases or variables)? #### Requirements: - **Data** should be on a metric scale (numbers that can be measured). - **Structured data** is needed (well-organized data with clear variables). **How Cluster Analysis Works:** 1. **Distance Measures**: - Used when the **absolute distance** between objects matters.\ Common measures: - **Euclidean Distance**: Straight-line distance between two points. - **Manhattan Distance**: Distance measured along axes at right angles. 2. **Proximity Measures**: - Used when the **profile** (the full characteristics) of the cases matters. **Steps for Clustering:** 1. **Choose a Clustering Method**:\ Decide on the type of clustering (e.g., hierarchical, cluster center analysis). 2. **Select a Proximity Measure**:\ Choose how to calculate the distances between cases (Euclidean, Manhattan, etc.). 3. **Follow These Steps**: - Define the problem. - Identify the objects or cases to be classified. - Select variables for clustering. - Decide how to measure similarity or distance. - Choose the grouping method. - Determine the number of clusters. - Perform the clustering. - Analyze and interpret the results. **How Many Clusters?** 1. **Heuristic Methods** (rules of thumb): - Look for clusters that **appear homogeneous**. - **Inverse Cream Test**: Check if the clusters are distinguishable. - Ensure clusters are **meaningful** and interpretable. 2. **Formal Tests**: - Use **F-values** to evaluate how well the variables fit within clusters. F= V(j,C)/V(j) - V(j,C): Variance of variable j in a cluster C - V(j): variance of variable j across all characteristic object **Example Method: Hierarchical Clustering** 1. **Proximity Calculation**: - Start by calculating the distance between all objects (cases). 2. **Cluster Formation**: - Each object starts in its own cluster. - The two closest clusters are combined. 3. **Iterative Process**: - Continue merging the closest clusters until all objects are in one cluster. 4. **Dendrogram**: - Visualize the clustering process as a tree-like diagram (dendrogram). **Clustering Types:** - **Hierarchical Cluster Analysis**: Builds clusters progressively. - Calculation of proximity values - Each object forms a cluster - The two clusters with the highest proximity are summarized as follows - Recalculation of proximity values - The two clusters with the highest proximity are summarized as follows - Iterative process until all objects are combined in a cluster. - **Cluster Center Analysis** (QuickCluster): Groups based on cluster centers. - **Two-Step Cluster Analysis**: Efficient for large datasets. - **Dendrogram**: A tree diagram showing how clusters are formed 8. The nation State / The Modern State ====================================== 8.1. Defining the State ----------------------- The state refers to an organized political structure that maintains authority over a population within a specific territory. Scholars offer several definitions of the state: - **Max Weber (1918, 1958)**: A state is a community that claims a monopoly on the legitimate use of physical force within a territory. - **Charles Tilly (1985)**: The state is a centralized, differentiated organization that claims control over violence within a given population and territory. - **Fishman (1990)**: A permanent structure of domination, coordination, with coercive powers and the ability to extract resources from society. - **Modern Concept of the State**: A set of political apparatuses distinct from both the ruler and the ruled, with supreme jurisdiction, monopoly over coercive power, and legitimacy derived from citizen support. ### Questions on the State Some important questions to consider about the state: - Why are nation-states dominant in the modern world? - When do states become fragile or contested? - How does immigration framing affect welfare state support? - How do rentier states adapt to global changes like decarbonization? - Is state functioning necessary for the emergence of democratic rule? - How should we understand the relationship between state and society---are they distinct or intertwined? - Is there a rise of \"developmental states\" in certain regions? - How can state strength be measured? ### Theories of the State Several theories explain the emergence and role of the state: - **Social Contract Theory (Hobbes)**: In \"Leviathan,\" Hobbes argues that individuals consent to give up certain rights in exchange for protection by an absolute sovereign, the state. This theory justifies the existence of a strong state for societal order. - **Weber\'s Concept of Legitimacy**: Weber's theory centers around the legitimacy of state authority, where the state\'s power is accepted because citizens see it as legitimate and necessary for maintaining social order. ### State Strength State strength refers to the capacity of a state to effectively govern and maintain control within its territory. State strength can be measured by: - **Centralization**: The concentration of power in the state's hands. - **Coercive Capacity**: The state's ability to use force to maintain order. - **Legitimacy**: The level of support or loyalty from citizens toward the state. 8.2 Definition of Stateness --------------------------- - Stateness: "state monopoly on the use of violence, administrative effectiveness of the state, and agreement on who are the citizens of the state" (Andersen et al 2014, 1204) - Stateness: "state authority, capacity and legitimacy" \[Grävingholt et al 2015, 286\]. Stateness refers to the core features that define a state's authority and legitimacy. It includes: 1. **Monopoly of Violence**: The state\'s capacity to use military or police forces to maintain public order. 2. **Administrative Effectiveness**: The ability of the state's bureaucracy to implement policies related to public services and regulations across the territory. 3. **Citizenship Agreement**: Popular acceptance of the state\'s authority, determining who qualifies as a citizen. If citizens disagree, the state\'s legitimacy weakens. 8.3 State-Society Relations and Social Contracts ------------------------------------------------ The relationship between the state and society has been a key subject of debate: - **Classical Theorists** (Hobbes, Locke, Rousseau): They argue that a **social contract** defines the relationship between the state and its citizens. Citizens agree to obey the state in exchange for protection and services. - **State-in-Society Approach (Migdal, 1988)**: This approach questions whether the state and society can be seen as separate entities, proposing that they are deeply interconnected. ### Definition of a social Contract A **social contract** is the set of explicit or implicit agreements between societal groups and the government, defining mutual rights and obligations (Loewe et al., 2021). **8.4 State Theory** Conceptualizing the State: Four analytical traditions (vom Hau) ------------------------------------------------------------------------------------  8.5 How strong is a state ------------------------- Grävingholt 2015 Ein Bild, das Text, Screenshot, Schrift, Zahl enthält. Automatisch generierte Beschreibung ### Measuring Stateness Several indices measure different aspects of stateness: #### Bertelsmann Transformation Index (BTI) Four indicators of stateness: 1. **Monopoly on the use of force**. 2. **State identity**. 3. **No interference from religious dogmas**. 4. **Basic administration**. These indicators assess the core functions needed for a state to operate effectively, such as the ability to maintain order and provide public services. #### Fragile State Index (FSI) Measures state strength across four categories: 1. **Social Indicators**: - Demographic pressures - Refugees or internally displaced persons - External interventions 2. **Political Indicators**: - Legitimacy of the state - Deterioration of public services - Rule of law and human rights 3. **Economic Indicators**: - Uneven economic development - Poverty or economic decline - Human flight and brain drain 4. **Cohesion Indicators**: - Security apparatus - Factionalized elites - Group grievances **Critiques of \"Failed States\" and \"State Fragility\" Concepts** - **Oversimplification**: The concept of \"failed states\" reduces complex issues to a binary of success or failure. - **Stigmatization**: Labeling a state as \"failed\" can marginalize its population and hinder constructive engagement. - **One-Size-Fits-All Approach**: Interventions based on the \"failed state\" label may ignore the unique contexts of each state. - **Missed Nuances**: These terms fail to capture the varying degrees and root causes of state weakness. **Alternative (Call, 2008)**: Instead of focusing on failure, consider **three gaps** in states: capacity, legitimacy, and security. ### The Three Gaps in States: Capacity, Legitimacy, and Security Gaps State-building efforts often aim to address three key gaps: 1. **Capacity Gap**: The state\'s ability to effectively govern and provide services. 2. **Legitimacy Gap**: The degree to which citizens trust and accept the state\'s authority. 3. **Security Gap**: The state\'s ability to ensure safety and maintain public order.  9. Regimes and regime types =========================== - A **regime** refers to the system of governance that defines the structure of political power, including: - **Access to Political Power**: Who can hold public office and how they get there. - **Rules for Political Decisions**: How decisions are made and who has the authority to make them. **Fishman (1990)** defines a regime as the formal and informal organization of political power, determining access to power and the relations between those in power and the rest of society. A regime is more permanent than a government but less permanent than the state. **Schmitter/Karl (1991)** defines a regime as the system of governance with specific patterns that: - Determine the methods of access to public offices. - Define who is allowed to access power. - Set rules for decision-making. A **political system** refers to the organization of government decisions and the interactions that allocate values for society (Easton, 1965). - Black Box Model by Easton: 9.1 Regime Types ---------------- There are three main regime types: 1. **Democracy** 2. **Authoritarian Regime** 3. **Totalitarian Regime** 4. **Autocracy** (a political system where rulers are not subject to enforceable laws) ### Totalitarian Regime A totalitarian regime is marked by: - **Overarching Ideology**: A single, all-encompassing ideology. - **Single Party**: Led by one person. - **Terroristic Police**: Used to control the population. - **Monopoly on Communications**: Control over media. - **Monopoly on Weapons**: Control over military and police. - **Centrally Directed Economy**: State controls economic activities. - **Oppression of Opposition**: Limited or no opposition allowed. This regime type is a **complete form of authoritarianism**. ### Authoritarian Regime Authoritarian regimes are characterized by: - **Limited Political Pluralism**: Few political parties, if any. - **No Guiding Ideology**: No clear ideology, but distinctive mentalities may exist. - **Low Political Mobilization**: Limited public involvement in politics. - **Power Concentration**: Power held by one leader or a small group. - **No Political Pluralism**: Political parties and opposition are suppressed. - **Centralized Power**: Strong state to maintain the status quo. - **Weak Rule of Law**: Laws are often disregarded or unevenly enforced. ### Democracy / Polyarchy Key features of democracy (or polyarchy) include: - **Meaningful Competition**: Regular, competitive elections. - **Inclusive Participation**: Broad involvement of citizens in politics. - **Civil and Political Liberties**: Protection of freedom of speech, assembly, etc. Dahl (1971) emphasizes that democracy allows competition, participation, and guarantees civil liberties to ensure fair political competition. ### Comparing Regimes  Key dimensions to compare regimes: - **Political Pluralism**: How many political parties and actors are allowed to participate? - **Ideology**: Does the regime have a unifying ideology or is it pragmatic? - **Power Concentration**: Who holds power---one leader, a small group, or the people? - **Civil Liberties**: Are freedoms and rights protected? - **Economic System**: Does the state control the economy or allow market forces? ### Discussing Regime Types **Grey Zones Between Regimes:** - **Hybrid Regimes**: Political systems that combine elements of both democracy and authoritarianism. - **Diminished Subtypes**: Terms like **illiberal democracy** and **competitive authoritarianism** describe regimes that are neither fully democratic nor fully authoritarian. - **Measuring Democraticness**: Some scholars argue for assessing \"degrees of democracy\" rather than a binary classification of regimes. **Challenges in Measuring Democracy:** - **Degreeism**: The risk of reducing complex political systems into a scale or degree, which may blur fundamental differences. - **Democracy Bias**: The assumption that all regimes should be measured against a democratic standard. **Transformation of Autocracies:** - **New Forms of Authoritarianism**: With technology and digitalization, autocracies are evolving. Terms like **\"post-modern totalitarianism\"**, **\"digital dictatorship\"**, and **\"neoliberal autocracies\"** describe new forms of autocratic rule blending technology, capitalism, and traditional power structures. 9.2 Democratic Regimes ---------------------- - **Democracy** can be defined in procedural terms as the method for political decision-making in which people gain power through competitive electio