Quantile Regression PDF
Document Details
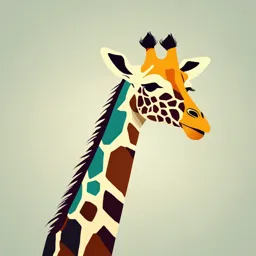
Uploaded by AppreciatedUranium
University of Bern
Blaise Melly
Tags
Related
- Repayment Flexibility & Risk Taking in Credit Contracts (2023) PDF
- NUTR 551 Starting Points in Data Analysis Lecture 3 PDF
- Introduction to Data Science Introductory Statistics/Probability Part II 2024 FM217 PDF
- Quantité de Matière, Concentration Molaire et Volume Molaire (PDF)
- La Quantité De Matière, La Concentration Molaire Et Le Volume Molaire PDF
- Exploring Spatial Patterns in Your Data PDF
Summary
This document is a presentation on quantile regression and related methods. It discusses causal analysis, motivations, economic examples, and applications, such as the JTPA. The document also includes graphs and examples to illustrate the concepts.
Full Transcript
Causal Analysis Quantile regression and related methods Blaise Melly Blaise Melly Quantile regression 1 / 88 Outline Until now, this course was only concerned with averages and average treatment effects. But the outcome can cha...
Causal Analysis Quantile regression and related methods Blaise Melly Blaise Melly Quantile regression 1 / 88 Outline Until now, this course was only concerned with averages and average treatment effects. But the outcome can change in a way not revealed by an examination of averages. The goal of the last part of the course is to present methods to estimate the causal effects of policies on the distribution of the outcome variable. Specific topics 1 Introduction: distribution, quantile, effect of randomized treatments 2 Conditional distributional treatment effects: quantile and distribution regression 3 Unconditional distributional treatment effects: counterfactual distributions 4 Instrumental variable methods (it time permits; i.e. probably not) Blaise Melly Quantile regression 2 / 88 Motivation What the regression curve does is give a grand summary for the averages of the distributions corresponding to the set of of X ’s. We could go further and compute several different regres- sion curves corresponding to the various percentage points of the distributions and thus get a more complete picture of the set. Or- dinarily this is not done, and so regression often gives a rather incomplete picture. Just as the mean gives an incomplete picture of a single distribution, so the regression curve gives a correspond- ingly incomplete picture for a set of distributions. Mosteller and Tukey (1977) Blaise Melly Quantile regression 3 / 88 Motivation Francis Galton in a famous passage defending the “charms of statistics” against its many detractors, disapproved his statistical colleagues [who] limited their inquiries to Averages, and do not seem to revel in more comprehensive views. Their souls seem as dull to the charm of variety as that of a native of one of our flat English counties, whose retrospect of Switzerland was that, if the mountains could be thrown into its lakes, two nuisances would be got rid of at once. Natural Inheritance, 1889 Blaise Melly Quantile regression 4 / 88 Economic examples Increasing wage inequality Effect of the minimum wage on wages Unemployment duration: special interest in long-term unemployment Birth weight: special interest in low birth weight Wages: special interest in people below the powerty line Educational inequality Always: we have more information when we know the effects on the distribution than only the effect on the mean. Blaise Melly Quantile regression 5 / 88 Job Training Partnership Act (JTPA) We start with the simplest case: a randomized treatment. It is a good way to introduce the basic concepts and tools that will appear again later in more complex frameworks. If something is not possible in the simplest case, it will also not be possible in more complicated cases. Example: the JTPA random assignment from an experimental evaluation of employment and training programmes classroom training, on-the-job training and job search assistance to the disadvantaged outcome: earnings in the 18 months following the assignment. Blaise Melly Quantile regression 6 / 88 I. Random assignment Treated and control potential outcomes: Y1 and Y0 Treatment status: D Observed outcome: Y = DY1 + (1 − D ) Y0 The average treatment effect is identified by random assignment: ATE = E [Y1 − Y0 ] = E [Y1 |D = 1] − E [Y0 |D = 0] = E [Y |D = 1] − E [Y |D = 0] Blaise Melly Quantile regression 7 / 88 Distribution treatment effect For a random variable Y , the cumulative distribution function evaluated at y is FY (y ) = Pr (Y ≤ y ) = E [1 (Y ≤ y )] By random assignment, FY1 (y ) and FY0 (y ) are identified FY1 (y ) = E [1 (Y ≤ y ) |D = 1] and FY 0 ( y ) = E [ 1 ( Y ≤ y ) | D = 0 ] Thus, the distribution treated effect is also identified: DTE (y ) = FY1 (y ) − FY0 (y ) Note that this is actually an average treatment effect but for 1 (Y ≤ y ) instead of Y. Blaise Melly Quantile regression 8 / 88 Univariate quantiles For a random variable Y , the quantile function evaluated at 0 < τ < 1 is Inverse, tells that quant. is the inv. of the distrib. QY (τ ) = FY−1 (τ ) = inf {y |FY (y ) ≥ τ } The quantile function is simply the inverse of the distribution function. The same relationships can be written in the reverse order: FY (y ) = QY−1 (y ) = sup {τ |QY (τ ) ≤ y } Z 1 = 1 (QY (τ ) ≤ y ) d τ 0 The quantiles are defined by a moment condition The quantile is equal to E [1 (Y ≤ QY (τ ))] = τ the expected value of the outcome being less than the quantile. Blaise Melly Quantile regression 9 / 88 Population median as optimizer The simplest case is the median QY (0.5) = arg minE [|Y − q |] q → Least Absolute Deviation (LAD). Intuition: First order condition ∂E [|Y − q |] ! = E [1 (Y > q ) − 1 (Y ≤ q )] = 0 ∂q At the minimum the probability that Y ≤ q is the same as the probability that Y > q. This is the definition of median. Blaise Melly Quantile regression 10 / 88 Population quantiles as optimizers General case: QY (τ ) = = arg minE [|Y − q | · {(1 − τ ) · 1 (Y ≤ q ) + τ · 1 (Y > q )}] q = arg minE [(Y − q ) · (τ − 1 (Y ≤ q ))] q ≡ arg minE [ρτ (Y − q )] q Note that the quantiles depend only on the signs of the residuals → robust to outliers. This is an advantage of using quantiles instead of averages. Another advantage: easy to bound. Blaise Melly Quantile regression 11 / 88 Quantile treatment effects: randomized case From the identification of FY1 (y ) and FY0 (y ) follows the identification of any function of these marginal distributions: Gini coefficient, Lorenz curves, quantile function,... In particular, the quantile treatment effects are identified QTE (τ ) = QY1 (τ ) − QY0 (τ ) Quantiles and quantile treatment effects have a natural and intuitive interpretation. The ATE is the average QTE ATE = E [QTE (τ )] τ Blaise Melly Quantile regression 12 / 88 QTE examples change in relationships for diff. quantiles Blaise Melly Quantile regression 13 / 88 Effects on the distribution vs. the distribution of the effects A percentile of a variable is NOT the percentile of another variable. Like 10% perc of income differs from 10% perc of education, not the same observation. Randomization identifies the effects of the treatment on the marginal distribution of the outcome. It does not identify the joint distribution of the potential outcomes. In particular, the distribution of the individual effects are not identified. Heckman, Smith and Clements (1996) discuss this problem and the bounds on the joint distribution. With rank invariance the QTE do have an interpretation as individual treatment effects. Blaise Melly Quantile regression 14 / 88 Estimation The natural estimator of the cumulative distribution function is the empirical distribution 1 n n i∑ F̂Y (y ) = 1 (yi ≤ y ) =1 The natural estimators of all functions of the CDF are the respective functions of the empirical distribution. For instance, Q̂Y (τ ) = inf y |F̂Y (y ) ≥ τ Blaise Melly Quantile regression 15 / 88 JTPA: CDFs Blaise Melly Quantile regression 16 / 88 JTPA: DTE Blaise Melly Quantile regression 17 / 88 JTPA: DTE as a function of the quantile Earnings based actual value Blaise Melly Quantile regression 18 / 88 JTPA: quantile functions Blaise Melly Quantile regression 19 / 88 JTPA: QTE Blaise Melly Quantile regression 20 / 88 Equivariance to monotone transformations For any monotone function h, quantile functions QY (τ ) are equivariant in the sense that Qh(Y ) (τ ) = h [QY (τ )]. For instance, the log of the median of Y is also the median of the log of Y. This property stands in contrast to conditional mean functions for which, generally, E [h (Y )] ̸= h (E [Y ]). This property will be preserved for conditional quantiles. It is especially useful for censored models. Blaise Melly Quantile regression 21 / 88 Asymptotic distribution The empirical distribution evaluated at one point is a sample mean of binary observations estimation 1 n of the F̂Y (y ) = ∑ 1 (yi ≤ y ). cumul. distrib. n i =1 function By the law of large number, we know that F̂Y (y ) → FY (y ) as By the central limit theorem, we know that √ n F̂Y (y ) − FY (y ) → N (0, FY (y ) (1 − FY (y ))) d Blaise Melly Quantile regression 22 / 88 Quantiles and other functionals We now consider the asymptotic properties of the estimated quantile function. The results for other functionals of FY (·) are similar because we always use the plug-in principle. An important difference between quantile and distribution functions: For quantiles, we obtain consistency and asymptotic normality only for continuously distributed outcomes. The previous results for the CDF are valid for all types of variables. The quantile function is consistent by the continuous mapping theorem. We need the functional delta method to derive the limiting distribution. Blaise Melly Quantile regression 23 / 88 Limiting distribution of the sample quantile The asymptotic distribution of the estimated quantile function can be derived from the asymptotic distribution of the estimated cumulative distribution function using the functional delta method. See chapters 20 and 21 in van der Vaart (1998) “Asymptotic Statistics”. Here: informal derivation of the result. Note that −1 Q̂Y (τ ) = F̂Y−1 (τ ) = FY + F̂Y − FY (τ ) Using the inverse function rule, a first-order Taylor expansion wrt F̂y evaluated at F̂y = FY can be written as F̂Y (FY−1 (τ )) − FY (FY−1 (τ )) F̂Y−1 (τ ) ≈ FY−1 (τ ) + fY (FY−1 (τ )) Blaise Melly Quantile regression 24 / 88 Limiting distribution of the sample quantile (cont.) √ Rearranging the terms, multiplying both sides by n, and inserting the asymptotic distribution for the cumulative distribution function, we obtain √ √ n Q̂Y (τ ) − QY (τ ) = n F̂Y−1 (τ ) − FY−1 (τ ) √ ! n F̂Y FY−1 (τ ) − FY FY−1 (τ ) τ (1 − τ ) ≈ → N 0, fY FY−1 (τ ) 2 d fY F −1 (τ ) Y If we consider a finite collection of quantiles: asymptotic joint normality of the vector of sample quantiles. Estimation of the standard errors: analytic estimator of the asymptotic variance: must estimate the density bootstrap is valid for the empirical distribution. Blaise Melly Quantile regression 25 / 88 QTE for JTPA with confidence bands Blaise Melly Quantile regression 26 / 88 Need for covariates 1 The treatment may not be binary. In the extreme case it may be continuous, such that these simple tools cannot be used. 2 The treatment may be binary and randomized but we may want to include covariates to increase the precision of the estimates. 3 In many cases the treatment has not been randomized. We may assume that the treatment is as good as randomized only after conditioning on some covariates. In all these cases, we need a method that allows estimating the conditional quantile/distribution function Blaise Melly Quantile regression 27 / 88 II. Conditional distribution and quantile function We can estimate either the conditional distribution function FY (y |X ) or the conditional quantile function QY (τ |X ). This distinction does not matter in the fully nonparametric case because one estimate is the inverse of the other one (e.g. fully saturated discrete regressors). In most cases nonparametric estimation is not practicable. We use parametric models to approximate the true function. Here it matters whether we modelize the QF or the CDF. Most of the literature uses quantile regression. We will briefly see an alternative: distribution regression. Blaise Melly Quantile regression 28 / 88 Example of conditional quantile curves Blaise Melly Quantile regression 29 / 88 Exogenous conditional linear quantile models We assume that the conditional quantile functions can be approximated using linear forms QY (τ |X ) = X ′ β (τ ) X can be a transformation of the original variables. Linearity is assumed for simplicity and computational convenience. β (τ ) is allowed to change with τ. This model nests the location shift model (OLS with independent error term): Y = X ′ β + V , V ⊥⊥ X QY (τ |x ) = x ′ β + QV (τ ). Parsimonious but restrictive, X only impact location of Y. β (τ ) is constant (except for the constant). Blaise Melly Quantile regression 30 / 88 Conditional quantiles as minimizers The unconditional mean solves h i µ = arg min E (Y − m )2 m OLS solves h 2 i β = arg min E Y − X ′b b The τ unconditional quantile solves QY (τ ) = arg min E [ρτ (Y − q )] q ρτ (U ) = (τ − 1 (U ≤ 0)) U The τ quantile regression solves β (τ ) = arg min E ρτ Y − X ′ b b Blaise Melly Quantile regression 31 / 88 Moment restrictions The population parameter solves the moment conditions E τ − 1 Y ≤ X ′ β (τ ) · X = 0 or τ − Pr Y ≤ X ′ β (τ ) |X E ·X = 0 These are the correct moment restrictions that arise from original conditional moment restrictions Blaise Melly Quantile regression 32 / 88 Estimation Replace the population mean by the sample mean 1 n ∑ ρτ Yi − Xi′ b β̂ (τ ) = arg min b n i =1 The check function is not differentiable, so common gradient procedure cannot be used. The problem can be written as a linear programming problem: quick algorithm for not too large data. Interior point methods & preprocessing for very large data. Relatively rudimentary implementation by Stata. Impressive set of commands in the quantreg package for R. Blaise Melly Quantile regression 33 / 88 Asymptotic distribution Under some regularity assumption (e.g. continuous Y with strictly positive density, no multicolinearity) √ n β̂ (τ ) − β (τ ) −→ N (0, V ) where = J (τ )−1 τ (1 − τ ) E XX ′ J (τ )−1 V J (τ ) = E fY X ′ β (τ ) |X XX ′ Bootstrap can be used. Blaise Melly Quantile regression 34 / 88 Link with the QTE If only a constant and a treatment indicator variable are included in the regression: the coefficient on the treatment variable is numerically equal to QTE defined in lecture 1. When additional control variables are included in the regression: QR identifies conditional QTE. The quantile is defined conditionally on the value of the covariates. For instance, in a wage regression, an individual with a college degree at the 2nd decile may have a higher wage than one with a minimal level of education who is at the 8th decile. Conditionally poor may be very different from unconditionally poor! This is a very common misunderstanding of QR. Blaise Melly Quantile regression 35 / 88 Randomized treatment It is quite common to include covariates in the regression even if the treatment is unconditionally exogenous (e.g. randomized). For the ATE there is a clean theory: OLS is always a consistent estimator of the ATE and may be more precise if the covariates have some explanatory power. This is not the case for QTE! Adding covariates changes the estimand. The quantiles get a different interpretation (from unconditional QTE to conditional QTE). The reason is that there is no law of iterated quantile: QY (τ ) ̸= E [QY (τ |X )] Blaise Melly Quantile regression 36 / 88 A model of infant birthweight Reference: Abrevaya (2001), Koenker and Hallock (2001) Data: June, 1997, Detailed Natality Data of the US. Live, singleton births, with mothers recorded as either black or white, between 18-45, and residing in the U.S. Sample size: 198,377. Response: Infant Birthweight (in grams) Covariates: Mother’s Education Mother’s Prenatal Care Mother’s Smoking Mother’s Age Mother’s Weight Gain Blaise Melly Quantile regression 37 / 88 Quantile Regression Birthweight Model I Blaise Melly Quantile regression 38 / 88 Quantile Regression Birthweight Model II Blaise Melly Quantile regression 39 / 88 Wage structure Changes in Wage Structure in the U.S. in 1980-2000. Here Y records log-wages for prime age white men, and X includes schooling and quadratic function in experience. Reference: Angrist, Chernozhukov and Fernandez-Val (2006) Blaise Melly Quantile regression 40 / 88 Wage structure Blaise Melly Quantile regression 41 / 88 An alternative: distribution regression Instead of estimating the conditional quantile function, it is possible to estimate the conditional distribution function. The conditional distribution is simply a conditional probability. Distribution regression model (Foresi and Peracchi 1995): FY (y |x ) = Λ(x ′ β(y )), where Λ is a link function (probit, logit, linear probability model, cauchit). X can have heterogeneous effects across the distribution:β (τ ) is allowed to change with y. Blaise Melly Quantile regression 42 / 88 Estimation and inference Maximum likelihood estimation of the parameter vector β(y ): 1 Create indicators 1{Y ≤ y }, 2 Probit/logit of 1{Y ≤ y } on X. Under correct specification, √ n β̂ (y ) − β (y ) → N (0, V ) d where −1 λ(X ′ β(y ))2 V =E XX ′ Λ(X ′ β(y ))[1 − Λ(X ′ β(y ))] and λ (·) is the derivative of Λ (·). Blaise Melly Quantile regression 43 / 88 III. Unconditional effects Conditional DTE are not always what we want to report. Policy makers are often interested in the unconditionally poor people, the unconditionally low-weight babies, the unconditionally long-term unemployed,... Very natural parameters: unconditional QTE and DTE QY1 (τ ) − QY0 (τ ) and FY1 (y ) − FY0 (y ) + one-dimensional function summary of the effects for the whole population + definition independent from √ the covariates + (can be estimated at the n rate without parametric assumptions) - does not allow analyzing heterogeneity with respect to the covariates (but allows for it) Blaise Melly Quantile regression 44 / 88 Definition vs. identification Definition of the estimand: we may not want to condition on the covariates. Identification: we want to condition on the covariates to relax the exogeneity restriction (selection on observables). Solution: estimate the conditional distribution and integrate it out to obtain the unconditional distribution. Example: the effect on the treated is the difference between FY (y |D = 1) and the counterfactual distribution Z FY (y | x, D = 0)dFX (x |D = 1) This lecture is based on Chernozhukov, Fernandez-Val and Melly (2013). Blaise Melly Quantile regression 45 / 88 Questions What would have been the wage distribution in 1979 if the workers had the same distribution of characteristics as in 1988? What would be the distribution of housing prices resulting from cleaning up a local hazardous-waste site? What would be the distribution of wages for female workers if female workers were paid as much as male workers with the same characteristics? In general, given an outcome Y and a covariate vector X. What is the effect on FY of a change in 1 FX (holding FY |X fixed)? 2 FY |X (holding FX fixed)? To answer these questions we need to estimate counterfactual distributions. Blaise Melly Quantile regression 46 / 88 Counterfactual distributions Let 0 denote 1979 and 1 denote 1988. Y is wages and X is a vector of worker characteristics (education, experience,...). FXk (x ) is worker composition in k ∈ {0, 1}; FYj (y | x ) is wage structure in j ∈ {0, 1}. Define Z FY ⟨ j | k ⟩ ( y ) : = FYj (y | x )dFXk (x ). FY ⟨0|0⟩ is the observed distribution of wages in 1979; FY ⟨0|1⟩ is the counterfactual distribution of wages in 1979 if workers have 1988 composition. Common support: FY ⟨0|1⟩ is well defined if the support of X1 is included in the support of X0. Blaise Melly Quantile regression 47 / 88 Policy effects We are interested in the effect of shifting the covariate distribution from 1979 to that of 1988. Distribution effects ∆DE (y ) = FY ⟨0|1⟩ (y ) − FY ⟨0|0⟩ (y ) The quantiles are often also of interest: QY ⟨j |k ⟩ (τ ) = inf {y : FY ⟨j |k ⟩ (y ) ≥ u }, 0 < τ < 1. Quantile effects ∆QE (τ ) = QY ⟨0|1⟩ (τ ) − QY ⟨0|0⟩ (τ ) In general, for a functional ϕ, the effects is ∆ ( w ) : = ϕ ( FY ⟨ 0 | 1 ⟩ ) ( w ) − ϕ ( FY ⟨ 0 | 0 ⟩ ) ( w ). Special cases: Lorenz curve, Gini coefficient, interquartile range, and more trivially the mean and the variance. Blaise Melly Quantile regression 48 / 88 Decompositions The counterfactual distributions that we analyze are the key ingredients of the decomposition methods often used in economics. Blinder/Oaxaca decomposition (parametric, linear decomposition of the mean difference): Ȳ1 − Ȳ0 = (X̄1 β 1 − X̄1 β 0 ) + (X̄1 β 0 − X̄0 β 0 ). This fits in our framework (even if our machinery is not needed in this simple case) as Y ⟨1|1⟩ − Y ⟨0|0⟩ = Y ⟨1|1⟩ − Y ⟨0|1⟩ + Y ⟨0|1⟩ − Y ⟨0|0⟩. Our results allow us to do similar decomposition of any functional of the distribution. E.g. a quantile decomposition QY ⟨1|1⟩ (τ ) − QY ⟨0|1⟩ (τ ) + QY ⟨0|1⟩ (τ ) − QY ⟨0|0⟩ (τ ). Blaise Melly Quantile regression 49 / 88 Causal treatment effects The counterfactual distributions we analyze are always statistically well-defined object. The decompositions are of interest even in non-causal framework. There is some disagreement about whether the policy variable must be in principle manipulable or whether a pure mental act is enough to define causal effects (Rubin and Holland versus Heckman and Pearl). In a treatment effect framework, the effects have a causal interpretation under a conditional independence assumption (selection on observables): (Y0 , Y1 ) ⊥⊥ D | X Then, for instance, FY ⟨0|1⟩ (y ) = FY0 (y |D = 1) and the DTE and QTE are identified for the treated. Blaise Melly Quantile regression 50 / 88 Estimation: plug-in principle R We estimate the unknown elements in FY0 (y | x )dFX1 (x ) by analog estimators. We estimate the distribution of X1 by the empirical distribution in period 1. The conditional distribution can be estimated by: 1 Location and location-scale shift models (e.g. OLS and independent errors), 2 Quantile regression, 3 Duration models (e.g. proportional hazard model), 4 Distribution regression. Blaise Melly Quantile regression 51 / 88 Conditional quantile models Location shift model (OLS with independent error term): Y= X ′ β + V , V ⊥⊥ X QY (u |x ) = x ′ β + QV (u ). Parsimonious but restrictive, X only impact location of Y. Quantile regression (Koenker and Bassett 1978): Y = X ′ β(U ), U | X ∼ U (0, 1) QY (u |x ) = x ′ β(u ). X can change shape of entire conditional distribution. Connect the conditional distribution with the conditional quantile Z 1 FY 0 ( y | x ) ≡ 1{QY0 (u |x ) ≤ y }du. 0 Blaise Melly Quantile regression 52 / 88 Conditional distribution models Distribution regression model (Foresi and Peracchi 1995): FY (y |x ) = Λ(x ′ β(y )), where Λ is a link function (probit, logit, cauchit). X can have heterogeneous effects across the distribution. Blaise Melly Quantile regression 53 / 88 Applications 1 Engel curve 2 Gender wage gap 3 Wage distributions 1979-1988 The first two applications are very short illustrations while the third is more substantial. Blaise Melly Quantile regression 54 / 88 Engel curve Relationship between food expenditure and annual household income. Engel (1857) data set, originally collected by Ducpetiaux (1855) and Le Play (1855), from 235 budget surveys of 19th century working-class Belgium households. Policy: neutral reallocation of income from above to below the mean that reduces the standard deviation of the observed income by 25% X1 = X̄0 + 0.75 (X0 − X̄0 ). Blaise Melly Quantile regression 55 / 88 Results using quantile regression Blaise Melly Quantile regression 56 / 88 Gender wage gap Albrecht, Björklund and Vroman (2003): “Is There a Glass Ceiling in Sweden?” They show that the gender log wage gap in Sweden increases throughout the wage distribution. Even after extensive controls for gender differences in age, education (both level and field), sector, industry, and occupation, they find that the glass ceiling effect persists. We do the same analysis using an extract from the MORG of the 2011 CPS. Y is the log wage, we control for potential experience, education, and region. Blaise Melly Quantile regression 57 / 88 Quantile “gender wage gap” Blaise Melly Quantile regression 58 / 88 Empirical application: wage distributions 1979-1988 DiNardo, Fortin and Lemieux (1996, DFL): institutional and labor market determinants of changes in the US wage distribution. Contributions: We provide consistent inference procedures and uniform confidence bands. We check the robustness of their findings by providing results based on alternative estimators. Data: ORG of the CPS in 1979 and 1988 Y : log hourly wage in 1979 dollars X = (U, C ): U is union status, C are worker characteristics including education, experience, and other controls Blaise Melly Quantile regression 59 / 88 Observed wage distributions in 1979 and 1988 Blaise Melly Quantile regression 60 / 88 Sequential wage decomposition Let FY ⟨(t,s )|(r ,v )⟩ be the distribution of log-wages Y with year t wage structure, year s minimum wage M, year r union status U, and year v worker composition C. Decomposition of observed changes in distribution: FY ⟨(1,1)|(1,1)⟩ − FY ⟨(0,0)|(0,0)⟩ = | {z } Observed Change [FY ⟨(1,1)|(1,1)⟩ − FY ⟨(1,0)|(1,1)⟩ ] + [FY ⟨(1,0)|(1,1)⟩ − FY ⟨(1,0)|(0,1)⟩ ] | {z } | {z } Minimum wage Union + [FY ⟨(1,0)|(0,1)⟩ − FY ⟨(1,0)|(0,0)⟩ ] + [FY ⟨(1,0)|(0,0)⟩ − FY ⟨(0,0)|(0,0)⟩ ] | {z } | {z } Composition Structure FY ⟨(1,0)|(1,1)⟩ , FY ⟨(1,0)|(0,1)⟩ and FY ⟨(1,0)|(0,0)⟩ are counterfactual distributions. Generalization of Oaxaca-Blinder decomposition. Blaise Melly Quantile regression 61 / 88 Wage decomposition: minimum wage The real value of the minimum wage decreased by 27 percent between 1979 and 1988. Following DFL, we assume that the minimum wage has no spillover effects, the conditional wage density at or below the minimum wage depends only on the value of the real minimum wage, and that the minimum wage has no employment effects. Under these assumptions, FY (1,1) (m0 |x ) Y(0,0) (y | x ) FY , if y < m0 ; F FY(1,0) (y | x ) = (0,0) (m0 |x ) Y(1,1) (y | x ) , if y ≥ m0 ; F R FY ⟨(1,0)|(1,1)⟩ (y ) = FY(1,0) (y |x )dFX1 (x ) can be estimated using sample analogs. To check robustness we also censor below minimum wage FY(1,0) (y | x ) = 0 if y < m0. Blaise Melly Quantile regression 62 / 88 Wage decomposition: de-unionization Unionization declined from 30% to 21% in the sample. To isolate the effect of the union status from other worker characteristics we need to estimate Z Z FY ⟨(1,0)|(0,1)⟩ (y ) = FY(1,0) (y | x ) dFU0 (u | c )dFC1 (c ). We estimate FU0 (1 | c ) = Pr(U0 = 1 | c ) by a logit model. FY ⟨(1,0)|(1,1)⟩ − FY ⟨(1,0)|(0,1)⟩ is the partial effect of union purged of other composition effects. Blaise Melly Quantile regression 63 / 88 Wage decomposition: other characteristics and prices Composition changes in the workforce can explain the evolution of the wage distribution. To isolate the effect of other worker characteristics from the effect of union status we can compare FY ⟨(1,0)|(0,1)⟩ with Z FY ⟨(1,0)|(0,0)⟩ (y ) = FY(1,0) (y | x ) dFX0 (x ). The last component (FY ⟨(1,0)|(0,0)⟩ (y ) − FY ⟨(0,0)|(0,0)⟩ (y )) is referred to as the price component. It is due to changes in the returns (coefficients) of worker characteristics including education and experience. Blaise Melly Quantile regression 64 / 88 Estimation of the conditional distribution Start with 4 models: linear location-shift model, linear quantile regression model, linear censored quantile regression model and logit distribution regression model. We discard the pure location model because the conditional distribution of wages is heteroskedastic. Nonlinearities induced by the minimum wage are problematic for the location model and the quantile regression model. The discreteness of the dependent variable due to rounding is problematic for the location, quantile regression and censored quantile regression models. ⇒ The distribution regression model is our favorite model for this application. Blaise Melly Quantile regression 65 / 88 Quantile policy effects Blaise Melly Quantile regression 66 / 88 Distribution policy effects Blaise Melly Quantile regression 67 / 88 Lorenz policy effects Blaise Melly Quantile regression 68 / 88 Robustness to link function Blaise Melly Quantile regression 69 / 88 Robustness to conditional model and minimum wage Blaise Melly Quantile regression 70 / 88 Summary of the empirical results Our results reinforce the importance of the decline in the real value of minimum wage. De-unionization plays a minor role. Effect of union is heterogenous (U-shaped) across the distribution. Changes in the composition of the workforce lead to increase in wage inequality at all quantiles (Lemieux, 06; Autor, Katz, and Kerney, 08). U-shaped changes in residual inequality extend “polarization of the labor market” phenomenon (Autor, Katz, and Kerney, 06) to the 80s. Results are robust to censoring of wages below the minimum wage and to the choice of the conditional model. Blaise Melly Quantile regression 71 / 88 Summary Chernozhukov, Fernandez-Val and Melly (2013) provide methods to perform inference on the effect on an outcome of interest of a change in either the distribution of policy-related variables or the relationship of the outcome with these variables. General approach based on functional differentiability allows us to consider general counterfactual changes, to analyze the effect on the entire outcome distribution, and to use resampling methods for simultaneous inference. Software available in Stata: commands counterfactual, cdeco, cdeco jmp and in R Blaise Melly Quantile regression 72 / 88 Propensity score reweighting Alternative approach to estimating these decompositions: inverse probability weighting (propensity score re-weighting). Pool all the populations and let Dk denote an indicator for the treated population with Pk = Pr (Dk = 1). Let Pk (X ) = Pr (Dk = 1|X ) be the propensity scores. By the law of iterated expectation FX (x |Dk = 1 ) Pk = Pk ( X ) F X ( x ) FX ( x | Dj = 1 ) Pj = Pj ( X ) F X ( x ) Pk ( X ) Pj =⇒ FX (x |Dk = 1) = FX ( x | Dj = 1 ) Pk Pj (X ) The counterfactual distribution has a re-weighted representation Pk ( X ) Pj Z FY ⟨ j | k ⟩ ( y ) = FY (y | x, Dj = 1) dFX (x |Dj = 1) Pk Pj ( X ) Blaise Melly Quantile regression 73 / 88 Propensity score reweighting (cont.) DiNardo, Fortin and Lemieux (1996) and Firpo (2006), among others, have sugggested estimators based on this representation. Estimation is simply: 1 parametric or nonparametric estimation of the propensity scores, 2 weighted CDF or quantile functions are consistent estimators. Implemented in Stata: command ivqte. Both the regression and reweighting approaches are perfectly valid. In fully saturated models they are numerically identical. When parametric estimators are used, these two estimators make different parametric assumptions. None is more general. Blaise Melly Quantile regression 74 / 88 Comparison of these approaches Advantage of the re-weighting approach: simpler to implement and quicker (only one regression). Advantage of the regression approach: the intermediate step—the estimation of the conditional model—is often of independent economic interest. One example: decomposition of the variance into between- and within-group inequality Var [Y ] = E [ β(U )]′ Var [X ]E [ β(U )] + trace {E [XX ′ ]Var [ β(U )]}. We find that the composition changes increased both components by about 10%. Example: increase in college graduates from 19 to 23%. Blaise Melly Quantile regression 75 / 88 IV. Instrumental variables The treatment is often endogenous =⇒ instrumental variable (IV) strategy. 2 approaches to endogeneity: restriction on the first-stage heterogeneity (monotonicity): identification for the “compliers” restriction on the second-stage heterogeneity (rank similarity): identification for the whole population We can still consider conditional or unconditional parameters. We start with conditional models and see later how we can integrate these conditional models. We do not consider nonparametric IV models with continuous treatments. Blaise Melly Quantile regression 76 / 88 Notation Instrument: Z Treatment: D (potential treatments Dz ) Continuous outcome: Y (potential outcomes Yd ) Control variables: X Blaise Melly Quantile regression 77 / 88 Abadie, Angrist and Imbens (2002) Abadie, Angrist et Imbens (2002) consider only the case with a binary instrument and a binary treatment. Following Imbens and Angrist (1994), they define four groups: 1 Always-takers: D = D = 1 0 1 2 Never-takers: D = D = 0 0 1 3 Compliers: D < D 0 1 4 Defiers: D > D 0 1 Blaise Melly Quantile regression 78 / 88 Assumptions 1 There are some compliers (relevance of the instrument) 2 There are no defiers (monotonicity) 3 Conditional exclusion restriction (Y0 , Y1 , D0 , D1 ) ⊥⊥ Z |X 4 Common support 0 < Pr (Z = 1|X ) ≡ p (X ) < 1 5 In addition, for practicability, they assume QY (τ |X , D, D1 > D0 ) = α (τ ) D + X ′ β (τ ) Blaise Melly Quantile regression 79 / 88 Identification without covariates Average treatment effect E (Y |Z = 1) − E (Yi |Z = 0) E [Y1 − Y0 |D0 < D1 ] = E (D |Z = 1) − E (D |Z = 0) Problem with QTE: non-additivity of the quantiles Separate identification of both marginal distributions FY1 (y |D0 < D1 ) = E (1 (Y ≤ y ) D |Z = 1) − E (1 (Y ≤ y ) D |Z = 0) E (D |Z = 1) − E (D |Z = 0) Blaise Melly Quantile regression 80 / 88 Including covariates While the conditional CDF and quantile functions are trivially identified by a conditional version of the previous expression, parametric estimation is not trivial. Abadie (2003) shows that there is also a weighting representation. Define D (1 − Z ) (1 − D ) Z κ (D, Z , X ) = 1 − − 1 − p (X ) p (X ) This function “finds” compliers. Note that κ = 1 for compliers, E [κ ] = 0 for always- and never-takers. A small problem: the κ can be negative. Blaise Melly Quantile regression 81 / 88 Weighted quantile regression Abadie (2003) shows that for any function h (Y , D, X ) E [κh (Y , D, X )] E [h (Y , D, X ) |D0 < D1 ] = Pr (D0 < D1 ) This result could be used to estimate a weighted quantile regression (α (τ ) , β (τ )) = arg minE κ · ρτ Y − aD − X ′ b a,b Problem: Because of the negative weights, the problem is no longer convex. There are many local minima. So they use the nonnegative weights κν = E [κ |Y , D, X ] = Pr (D0 < D1 |Y , D, X ) Blaise Melly Quantile regression 82 / 88 AAI: estimation 1 Nonparametric estimation of p (X ) ≡ Pr (Z = 1|X ). This is the IV propensity score. 2 Calculate κ. 3 Nonparametric estimation of E [κ |Y , D, X ]. The fitted values are the nonegative weights. 4 Standard weighted quantile regression of Y on D and X , using E [κ |Y , D, X ] as weights. Implemented in Stata: command ivqte. Blaise Melly Quantile regression 83 / 88 Application of AAI: JTPA While the assignment to the program was randomized, only about 60% of those offered training actually received JTPA. (Almost) one-sided perfect compliance: those not offered training could not participate. This means that monotonicity is (almost) certainly satisfied. Idea: use assignment as an instrument for effective program participation. Blaise Melly Quantile regression 84 / 88 Blaise Melly Quantile regression 85 / 88 Chernozhukov and Hansen (2005) They do not restrict the first stage equation. For instance, defiers can exist. Outcome equations Yd = φ (d, X , Ud ) where φ is strictly increasing in the scalar Ud. They assume either 1 Rank invariance: Conditional on X and Z , Ud = U, same rank in the potential outcome distribution across treatments. 2 Rank uniformity: Conditional on X , Z and V , {Ud } are identically distributed. This is weaker because it allows for noisy slippage of ranks across treatment status. It is a one-factor model. Blaise Melly Quantile regression 86 / 88 Moment condition The main statistical implication of CH model is E τ − 1 Y ≤ α ( τ ) D + X ′ β ( τ ) · (X , Z ) = 0 This suggest a natural GMM estimator. Problem: the objective function is not convex. Inverse quantile regression: find α (τ ) such that quantile regression of Y − α (τ ) · D on X and Z returns a coefficient of 0 on Z. Blaise Melly Quantile regression 87 / 88 Inverse quantile regression algorithm 1 For each α taken from a grid of possible values A, run QR of Y − Dα on X and Z : β̂ (α) , γ̂ (α) = arg minEn ρτ Y − Dα − X ′ b − Z ′ g (b,g ) 2 Pick α such that the Wald statistic for testing the exclusion of Z is as small as possible α̂ (τ ) = arg inf Wn (α) , α ∈A Wn (α) = n γ̂ (α)′ Ω̂− 1 γ γ̂ ( α ) This method works very well if endogenous regressors D are one-or two-dimensional. Instead of Z , E [D |X , Z ] can be used as instrument. Blaise Melly Quantile regression 88 / 88