Chapter 3: Laboratory Statistics, Method Development, and Quality Control PDF
Document Details
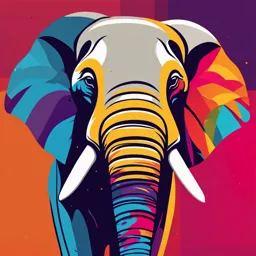
Uploaded by WellBalancedRadiance8883
Chattahoochee Technical College
Tags
Summary
This chapter discusses laboratory statistics, method development, and quality control in clinical laboratories. It covers topics such as descriptive and inferential statistics, quality control protocols (Westgard rules), laboratory errors, and data presentation. The chapter provides examples and explains how to select and interpret statistics for various types of data in a laboratory setting. Formulas are not discussed, but the chapter emphasizes appropriate statistical tool selection for accurate data interpretation in various types of data.
Full Transcript
48 CHAPTER 3 LAboRAToRy STATiSTiCS, METHod dEvELoPMEnT, And QuALiTy ConTRoL Key Terms Accuracy Descriptive statistics...
48 CHAPTER 3 LAboRAToRy STATiSTiCS, METHod dEvELoPMEnT, And QuALiTy ConTRoL Key Terms Accuracy Descriptive statistics Median Precision Arithmetic average Discordant results Mode Quality control bias Inferential statistics Nonnormal Range Calibration Limit of detection Nonparametric Reference interval Coefficient of variation (Cv) Linearity Parametric Sample Degrees of freedom (df, DF) Mean Population Skewness A CASE IN POINT Elaine assayed two serum quality control samples for glu-cose. 2. If no, why? The quality control result for the normal control was 3. What are some steps the Elaine should take to +1.5 standard deviations from the mean and the abnor-mal resolve this issue? quality control result was +3.3 standard deviations from the mean. Issues and Questions to Consider 1. Should Elaine proceed immediately with measuring patient samples? Whats Ahead 1. Identification and explanation of laboratory statistics commonly 5. Discussion of a protocol used to establish quality-control ranges. used in clinical laboratories. 6. Explanation andinterpretation of Westgardrules for quality 2. Discussion of appropriate utilization of selected statistics. control. 3. Interpretation of selected statistics for a given set of data. 7. Discussion of types of laboratory errors. 4. Definition and explanation of quality control and quality assurance. LABORATORY STATISTICS of appropriate statistics and accurate interpretation of the statisti-cal calculations. Statistics (e.g., arithmetic mean, standard deviation, and variance) should be viewed as tools that are available for the laboratory staff to use. These tools will assist the user in making important Basic Laboratory Statistics decisions about data collected during a study. Knowing which Data Presentation tool or statistic to use is important. Selecting the wrong stat Data can be presented in a variety of formats. Most data gen-erated will most likely result in a wrong assumption being made or an in the chemistry or hematology laboratory are numeric inappropriate action taken. A discussion of the tasks required in nature (e.g., 100 mg/dL or 4.0 * 106red blood cells/mm3). to produce reliable laboratory data is presented in this chapter. But for some laboratories, including blood bank and micro-biology, Procedures for doing these tasks are described. Guidelines for the data are mostly nonnumeric. Does this mean that selecting the proper statistics or tools to use to help make deci-sions these laboratories methods and instruments cannot be evalu-ated are included. Appropriate interpretation of data will also is using statistics? No. It would be impractical to attempt to presented. include examples of statistics that could be applied to all sorts Computation of most statistics is performed using any of of nonnumeric data. Instead, a brief discussion of examples of several computer software packages or calculators. Therefore, how data can be presented and an introduction to several types derivation and memorization of formulas are not necessary. Most of data will be presented. The reader can review any of the textbooks on clinical chemistry include formulas; thus, only alim-ited statistic references listed at the end of the chapter for specific few are included here. Whatis most important is the selection applications CHAPTER 3 LAboRAToRy STATiSTiCS, METHod dEvELoPMEnT, And QuALiTy ConTRoL 49 Three examples of how data can be presented include rank, Two additional important terms are population and sample. continuous, and discrete. Rankeddata are arranged from highest to A population refers to the universe of values or attributes, such as lowest according to magnitude and then assigned numbers that cor-respondall of the fasting serum cholesterol levels of all apparently healthy to each observation placed in the sequence. For example, malesin the United States. suppose a researcher is looking at the leading causes of death in Sample refer to a portion or subset of a population, such as the United States and the results of a literature search reveal the the serum cholesterol levels of all malesin the laboratory. Often following: the distinction between population and sample is not made very clear (e.g., a sampling could be argued to be a population). 1st = heart disease Parametersand statistics are terms associated with populations 2nd = cancer and samples, respectively. A parameter describes a quantitative 3rd = cerebrovasculardisease feature of a population. Statistics describe a quantitative feature of a sample. Based on the results, the found data are ranked such that heart Symbols are used throughout statistics. Table 3-1 ? compares disease is shown to be the leading cause of death in the United symbols used for parameters versus statistics. Parameters tend to States, cancer is second, and cerebrovascular disease third. use Greek symbols, whereas statistics use Roman or italicized Continuous data represent a continuous variable that can characters. assume any value within the range of scores that define the limits of the variable. Examples of continuous data include temperature, Measures of Central Tendency time, and the cholesterol level in a subject. Remember that there The arithmetic average or mean is commonly used to describe are no gaps in continuous data. data. For example, what is the average glucose value for all samples Discrete data are a subset of continuous data. A characteris-tic in a study? Summing all data and dividing the sum by the number of discrete data is that discrete data assume a defined set of of data items, represented by n or N, determines the arithmetic integers. Both order and magnitude are important. For example, mean. The median in a sample set is the middle value, or the age recorded in integral years is reported as 62 or 63 years old but 50th percentile value when the data are rank ordered by magni-tude. not 62.491 years old. Close observation of the data will show that half the data points are above and half are below the median. Mode has two Types of Data definitions in mathematics and statistics. A mode is defined as Nominaldata(categorical) area type of datain whichthe values the data point that occurs most frequently in a set of data. For fall into unordered categories or classes. Nominal data are num-bers example, the modein this set of data 51, 7, 7, 6, 4, 7, 5, 26 is 7. applied to nonnumeric variables. For example, a group of sub-jects A mode for a given distribution of data is defined as the local bloodtypes could be codedas group O = 1, group A = 2, maximum in a distribution chart of that set of data. Data for group B = 3, and group AB = 4. Noindividual or variablecan a sampling can be distributed within one mode(unimodal), two be assigned to morethan one group and order or sequence is not modes (bimodal),or several modes (polymodal) or have no dis-tinct important. mode at all. In Figure 3-1A , the distribution of data is Ordinal data (rank order) are numbers that are discrete and unimodal (i.e., there is only one local maximum). If the data for ordered (ranked). The order or ranking is important. For example, the set are distributed normally (i.e., Gaussian), then the mode is injuries may be classified according to their level of severity as the same asthe mean and median. If the data for the set is skewed follows: and unimodal (Figure 3-1B ), then the mode will be different 4 = the mostsevere or evenfatal injury than the population mean and median. Finally, a set of data may be bimodal; that is, it has two maximums, signifying that there 3 = a severeinjury are two distinct populations. This may reveal the presence of a 2 = moderatelysevere normal and abnormal subset within alarge population of subsets 1 = a minorinjury (Figure 3-1C ). Measures of central tendency are illustrated in The intervals are not usually known and are often unequal. Descriptive statistics include the mean, range, variability, and distribution of a data set. They represent the commonly used statistical computations in the clinical laboratory. ? TABLE 3-1 Terms and symbols associated with Inferential statistics are concerned with the relationship population and sample statistics. among different sets or samples of data. For example, is the mean Parameter Statistic of one set of data significantly different from another? If we find Arithmetic mean or average x a difference between the means of the two samples, what are the m s Standard deviation s probabilities associated with this difference? Examples of inferen-tial s2 variance s2 statistics include the following: Z Distribution of means t F-test Z-test m { 1.96s 95% confidence interval x { t1-a t-test chi square 50 CHAPTER 3 LAboRAToRy STATiSTiCS, METHod dEvELoPMEnT, And QuALiTy ConTRoL Mean ? TABLE 3-2 Data for 10 replicate measurements Mode of calcium in serum and results of selected descriptive Median statistics. Replicate Number Results 1 9.9 2 9.8 3 9.7 4 9.2 5 9.5 6 9.6 Unimodal 7 9.9 Symmetrical A Normal or Gaussian 8 9.3 9 9.6 Mean 10 9.6 Median Sum 96.1 Mode Arithmetic mean 9.61 Median 9.6 Mode 9.6 Range 9.29.9 Standard deviation 0.2331 variance 0.0543 Cv 2.4% Unimodal B Skewed values around a mean. It is derived from the curve of the normal distribution and bears a meaningful relation to the area under the Mode normal distribution curve so that, for example, the area under the Mode center ofthecurve represented bythemean{1.96s is ~95% of the whole area. A plot of a normal curve distribution is shown in Figure 3-2. Coefficient of Variation (CV) Coefficient of variation (CV) is C Bimodal another way of expressing standard deviation and is also referred FIGURE 3-1 Modes of distribution. A. Unimodal to asrelative standard deviation. It is defined as 100 times the standard symmetric (normal or Gaussian), B. unimodal deviation divided by the mean. CV is expressed as a percentage. skewed, and C. bimodal. It relates the standard deviation to the level at which the measure-ments are made. For example, from the data in Table 3-2, the mean Table 3-2 ?. Calculations of central tendency allow the deriva-tion and s of the measurements are 9.61 and 0.2331, respectively. The of graphical plots that can clearly show modal distributions CVfor the data setis 2.4%(0.2331/9.61 * 100). and skewness. Skewness is described as not symmetrical (asym-metrical). Data that are unimodal are symmetrical and the mean, s median, and mode coincide. When data are skewed, neither the Cv = * 100 (Eq. 3-1) x mean nor the mode estimates central tendency with a high degree of accuracy, although the median retains its distinction. Many CLS find CV a useful statistic. Because the numbers Measures of Dispersion are in percentage format, it is often easier to relate to CV than to Range Range is a measure of spread or variation in a set of standard deviation. Remember that the lower the %CV, the better data. The range of data is the difference between the largest and the performance of the assay. For example, from the data shown the smallest numbers of the data set or the smallest to the larg-est in Table 3-3 ?, it would appear that the precision (reproducibility) number. For example, the data in Table 3-2 ? show a range based on standard deviation of Method 4 is better than for Meth-ods of 9.29.9. 1, 2, and 3. But if the standard deviation is related to the mean concentration levels, Method 4 hasthe highest %CV and therefore Standard Deviation (s) Standard deviation is a commonly used is the poorest-performing method. estimator of dispersion because it is predictably related to a com-mon type of data distribution, the Gaussian or normal distribution. Variance(s2) Whenthe valuesof a set of observationslie close Standard deviation is a measure of the dispersion of a group of to their mean, the dispersion is less than when they are scattere CHAPTER 3 LAboRAToRy STATiSTiCS, METHod dEvELoPMEnT, And QuALiTy ConTRoL 51 x Outliers An outlier in a sample set is a measurement that belongs to a population other than the one to which most of the mea-surements belong. Outliers can distort the computed values of statistics and cause incorrect inferences to be made about the population parameters of interest. There are tests that can be used to determine whether a value is an outlier or not. The gap test1 and Prescott Test for Outliers2 are two examples used for clinical laboratory statistics. ChECkpoInt! 3-1 -3s -2s -1s x +1s +2s +3s A CLS was asked to determine the descriptive statistics Range for the data presented in the following table. The data 68.2% represent replicate measurements of sodium in serum samples. Twenty measurements were completed on one x + 1.00s sample. Determine the statistics listed for the data shown 90.0% in the table: x + 1.65s Arithmetic mean 95.0% Standard deviation x + 1.96s variance 99.0% Percent coefficient of variation x + 2.58s Median Mode 99.7% Range x + 3.00s FIGURE 3-2 A normal bell-shaped curve showing {1, 2,3sandtherelativepercentages associated Data Number Result Data Number Result with each area under the curve. 1 140 11 139 2 141 12 138 3 139 13 140 over a wide range. If the laboratory needs to know the measure of dispersion of data relative to the scatter of the values about the 4 140 14 140 mean, a statistic is available to provide this information. That statis-tic 5 140 15 141 is called variance. Variance is calculated by squaring the standard 6 138 16 139 deviation. It can also be derived by subtracting the mean from each 7 142 17 139 of the values, squaring the resulting differences, and then adding 8 141 18 141 up the squared differences. This sum of the squared deviations of the values from their mean is divided by the number of samples 9 141 19 140 minusone (N - 1) to obtain the sample variance. The variance 10 140 20 140 of the set of data shown in Table 3-2 ? is 0.0543. Calculated variances are also used in hypothesis testing such as the F-test. The F-test calculation shown later in this chapter uses a ratio of the larger variance to the smaller variance for two Population Distributions sets of data. Normal Distribution The normal distribution of an analytes in a selected population is a continuous distribution, symmetric aroundthe mean,predictablyrelatedto the standard deviation, or ? TABLE 3-3 Precision data for the measurement of sigma(s), and variance(s2). Gaussexplainedconceptsassociated glucose in pooled serum samples measured by four with normal distribution when he studied errors of replicate mea-suremen different methods. Graphicalplots of the patterns of clusteringabout the Pooled Sample Pooled Sample Pooled Sample mean with the symmetric distribution of outlying values produced Mean (mg/dL) SD (mg/dL) %CV a bell-shaped curve. This normal distribution of data characterized Method 1 240 12.2 5.1 manybiologicalattributes,such as height, weight,and biochemical Method 2 180 8.1 4.5 measurements. Method 3 120 6.5 5.4 Whenstudying measurements of an analyte in large popula-tions, Method 4 70 5.0 7.1 the parameterslisted in Table3-1 ? should be used. Thus, if an analyte concentration such as glucose is determined in 52 CHAPTER 3 LAboRAToRy STATiSTiCS, METHod dEvELoPMEnT, And QuALiTy ConTRoL parametric. If the data do not conform to the assumptions made for parametric statistics, then nonparametric methods of statistical inference should be used instead. Two examples are the Wilcoxon signed-rank test and the MannWhitney test. Nonparametric tests are well suited for data that are ordinal or nominal, which include data that are ranked. Frequenc Inferential Statistics 1 234567 Inferential statistics allow the CLS to address specific questions -3s -2s -1s 0 +1s +2s +3s regarding groups or sets of data. Examples of questions include m the following: FIGURE 3-3 A normal distribution curve with a meanand {1, {2, and {3 sigmas(s). Is the observed difference between two sample or population means due to chance alone? population of adults, the plot of frequency versus arithmetic mean Is the standard deviation (s) of one set of data significantly dif-ferent (m) or sigma (s) as presented in Figure 3-3 will be created. The from another? formula for Z answers the question of how many sigmas away Two commonly used tests that may provide answers to such from the population meanis the value in question. Thus, questionsarethe t-test and F-test, or F-ratio. Thesetests are dis-cussed in the next section, on hypothesis testing. Z = (x - m)/s (Eq. 3-2) Hypothesis Testing Hypothesis testing is useful if the CLS needsto drawsomeconclusion about a population parameter(e.g., a CLS needsto makean inference about the mean of a continuous Most CLSs are involved with smaller samplings that require a random variable, using the information contained in a sample of different approach than for population studies. Therefore, statistics observations). terms and symbols must be used (see Table 3-1). Additional ques-tions To perform such a test, we begin by claiming that the mean must be asked when evaluating distribution data using small of the population is equal to some postulated value m0. This state-ment samplings. For example, is this small sample likely to be from a about the value of a population parameteris calledthe null normally distributed population? Is the sample distribution sym-metrical hypothesis,or H0. The null hypothesis states that there is no signifi-cant or is it non-Gaussian? difference between specified populations, any observed dif-ferences being dueto sampling or experimental error. If the CLS ChECkpoInt! 3-2 needs to test whether the meanserum troponin I concentration of the subpopulation of patients with hypertension is equal to the A normal population study for uric acid in serum samples meanof the general population of 40-to 60-year-old males,for reveals that the mean of the group of samples is 6.0 mg/dL instance, the null hypothesis would be and the standard deviation is 0.5 mg/dL. What is the probability of a serum uric acid value of 6.9 or greater occurring in this series? H0:m1= m2 (Eq. 3-3) The alternative hypothesis, represented by HA, is a second Nonnormal Distribution Most laboratory data involving the statement that contradicts H0. This is shown as evaluation of methods, quality control, and proficiency surveys follow a normal distribution and lend themselves to calculations using fundamental descriptive statistics. This is not always the case HA: m1 ? m2 (Eq. 3-4) with analyte concentrations found in blood for a given analyte. In many cases, the distribution of data is nonnormal (asymmetrical Together, the null and the alternative hypothesis cover all pos-sible or skewed). The distribution may also be bimodal or log normal. values of the population mean(m); thus, one of the two state-ments If the distribution of the data is non-Gaussian, then alternative sta-tistical must be true. methods must be used to derive the parameters or statistics After formulating the hypothesis, we next draw a random shown in Table 3-1. Several of these methods are introduced in sample of size n from the population of interest. The sample specific sections of this chapter. size should be as large as possible. The mean of the samples is Parametric and Nonparametric Data Data that presume a nor-mal computed. Gaussian distribution are called parametric, and those that Another decision that needs to be made is which statistical do not are nonparametric or distribution free. Once the deci-sion test and formula to use. For example, should an F-test or t-test is made that a given distribution of data is Gaussian, then all (Students t-test, paired or unpaired) be used? The answer to these statistical tests and methods (e.g., means, s, t-test) are said to be questions goes back to the null hypothesis. Another way of stating CHAPTER 3 LAboRAToRy STATiSTiCS, METHod dEvELoPMEnT, And QuALiTy ConTRoL 53 this is to answer the question, What significance is being tested? mandatory. Thet-test can provide valuableinformation about It is beyond the scope of this text to present the numerous scenar-ios differences between the means of both sets of data. Two addi-tional a CLS mayface. Example 3-1 discusses a problem that occurs statistics necessary to calculate the t-value for paired data commonly in laboratories. are biasandthe standard deviation of differences(Sd).The datain Once the appropriate statistical test is selected, all the variables Table 3-4 ?indicate that the mean difference (bias) between paired of the formula must be addressed. For example, the formula may datais statistically different (see also Example 3-1). Thet-test has require a standard deviation, bias,square root of n, or standard provided a probability regarding whether or not there is a statisti-cal deviation of the difference to be determined. difference between the two methods under the conditions of Another important aspect of hypothesis testing is significance the evaluation. andsignificance level.Significanceasusedin statisticalinference refers Degrees of Freedom Degrees of freedom (df, DF) is to the probability statement (p), which implies that chance and described as the number of data points that affect the statistical random distortion of samplings have played only a small part in analysis. For example, suppose 30 measurements of glucose are the observed differences. Common significance levels for clinical obtained. The series has 30 degrees of freedom because no single applicationsare p 6 0.01 or p 6 0.05, which means that thereis measurement affected the other 29. However, if we calculate the only a 1% or 5% chance, respectively, that the observed difference meanofthe 30values, theseriesnow has(n - 1) or29 df because is due to chance alone. the 30th value in the series cannot be changed without altering Statistics tables may often use the symbol alpha (a) to rep-resent the mean. For small samplings of data, the df is calculated using significancelevels. Theareaunderthe curve or 1 - a n - 1.3Forlargersamplingsand populationstudies,the number is considered part of the sample population, and alpha is the of degrees of freedom may be calculated using other formulas such area under the curve that may represent the second overlapping asn - 2. population. One-Tailed and Two-Tailed Tests Another criterion that Bias requires a decision while formulating a hypothesis is whether to Bias is described and used in several different ways in laboratory evaluate the data using one-tailed or two-tailed tests. If the ques-tion statistics. Bias is the difference between two means, or the mean concerns the probability of observing a difference in only one difference. The value of the biasis usedin the t-test andin pro-tocols direction (i.e., does x1 exceed x2?), then select a one-tailed t-test. for method comparisons. Bias is also defined as the pres-ence The one-tailed t-test describes a distribution extending from minus of nonrandom events (e.g., estimating the serum cholesterol infinity to positives-or s@values that include 95% ofthe areaunder levels in apparently normal healthy individuals when it was not the curve, for ta = 0.05. If the question concerns only magnitude realized that they were on cholesterol-lowering medications). but not the direction (i.e., is x1 different from x2 ?), then select a Finally, bias may be described as lack of accuracy. For example, a two-tailed t-test and the area under the curve is the central 95%, laboratory is attempting to determine the accuracy of a method excluding 2.5% from each tail. by doing an analytical recovery experiment and does not realize Most clinical laboratory applications use the two-tailed test. that there is a reducing substance in the samples that constantly A two-tailed test decreases the chance of falsely rejecting the null affects the assay. hypothesis. Conversely, the two-tailed convention maycause failure to accept the difference when one exists. ? TABLE 3-4 A two-tailed paired-sample t-test Paired t-Test and Unpaired t-Test Two frequently used varia-tions for serum sodium measurements of 10 different of the t-test are the following: patient samples by two different methods The unpaired t-test (Students t-test or t-independent), in which (Biosensor vs. ISE). the means and standard deviations of two independent samples Sample Number Biosensor ISE are compared and each standard deviation is presumed to be from the same population. 1 141 138 2 140 136 The paired t-test or t-dependent, in which the average difference 3 144 147 between a series of paired observations is analyzed. 4 144 139 The unpaired t-test or t-independent is used to study the dif-ference 5 142 143 between two groups in which each subject is tested only 6 146 141 once. The question is whether or not there is a difference in the 7 149 143 data of one or more variable(s) between two groups that were 8 150 145 independent of one another. By independent we mean that the two 9 142 136 groups were not related in any way. CLSs rarely need to evaluate 10 148 14 unpaired or independent data; rather, most clinical laboratory stud-ies bias = 3.2 involve paired or dependent data. t = 3.402 Suppose the laboratory is implementing a second method p = 0.008 for measuring sodium. A comparison of the two methods is 54 CHAPTER 3 LAboRAToRy STATiSTiCS, METHod dEvELoPMEnT, And QuALiTy ConTRoL Standard Deviation of the Duplicates EXAMpLE 3-1 A standard deviation of the duplicates (also, standard deviation A laboratory purchased a new biosensor device to mea-sure of differences of paired data)is usefulto estimatethe variability sodium in blood; the new device will replace the cur-rently of measurements of the same sample in duplicate. It allows esti-mation used ion selective electrode. A split sample study of the minimumchange expectedfrom duplicateanalyti-cal was carried out, and the data are shown in Table 3-4. A error alone and permits estimation of whether a real change two-tailed paired-sample t-test was selected as a means in the patients status is likely to have occurred. The calculation to evaluate the two devices. The following information involves taking the squareroot of the sum of the differences of is included: duplicate data, divided by two, times the number of data pairs. H0: m1 = m2 Standard Error of the Mean of Differences HA: m1 ? m2 The standard error of the meanof differences quantifies the a = 0.05 uncertainty in the estimate of the mean of a population. This n = 10 uncertainty in the estimate is related to the random error that is present withall sampling techniques. Just asit is impossible to df = 9 repeat exactly a value for a single sample, it would beimpossible bias = 3.2 to obtain the exact meanfor the difference of paired data if the t = 3.402 samples wererepeated. It must be remembered that there is a difference between t0.05 = 2.262 the standard deviation and the standard error. The standard Conclusion: deviation quantifies the variability in the entire population of data, whereasthe standard error quantifies the variability in the Reject H0 estimate of the mean of the population. Therefore, the value 0.005 6 p 6 0.01 for the standard error will always beless than the valuefor the p = 0.008 standard deviation. Accept the alternative hypothesis, which is m1 ? m2. Confidence Intervals A confidence interval (CI) can be defined as a range around an experimentally determined statistic that hasa known probability of including the true parameter. Applicationsof confidenceinterval Standard Error of the Mean include the following: The measurement of serum sodium in either of the two methods Estimating the range of values that include a specified propor-tion shown in Table 3-4 is characterized by a certain degree of impre-cision. of all members of a population, such as the normal or The error of the mean measurement of the set of values reference interval of values for a laboratory test. is smaller than that of a single measurement, so the more times a measurement is made, the more certain you can be of its true Hypothesis testing. value. If several means are calculated from different groups of The confidence interval (e.g., 95%) associated with a given measurements of this population, the individual means are dis-tributed set of data will or will not actually include the true size of the about the actual population mean. The random variation sample population, but in the long run 95% of all possible CI in this group of means is distributed by the standard error of the willinclude the true difference of meanvalues associated with the mean. sample population. Assuch, CI not only describesthe size of the Standard Deviation of Differences The standard deviation of sample population but quantifies the certainty with which you can the differences (SDd) is a measure of the variability in the calcula-tion estimate the size of the sample population. of the difference between each member of a set of paired The size of the interval depends on the level of confi-dence data. In a comparison of methods, the SDd indicates the degree of you want that the sample studied will actually include the variability of the difference between the values of the new method true sample population. Therefore, it can be assumed with 95% and the values assumes a normal of the accepted distribution method. for these The standard differences deviation that is equally ofx-1.96(s/2N) tox+1.96(s/2N). This expressio is confidence that the true population meanlies within the range distributed on both sides of the bias. A large SDd may be due to termed the 95% CI. the following: Although a 95% CIis used mostoften in clinical practice, the laboratory is not restricted to this choice. Thelaboratory may The distribution of the difference about the bias is wide, prefer to have a greater degree of certainty regarding the value of indicating alarge amount of random error in the measurement the population mean; in this case,it could chooseto usea 99% CI on one or both methods. instead of a 95% interval. Because 99% of the observations in a The distribution may not be normally distributed. normaldistributionlie between-2.58and2.58,a 99%CIfor mi CHAPTER 3 LAboRAToRy STATiSTiCS, METHod dEvELoPMEnT, And QuALiTy ConTRoL 55 -2.58(s/2N) and 2.58(s/2N). Therefore, approximately 99 ChECkpoInt! 3-3 out of 100 confidence intervals obtained from 100 independent A clinical laboratory is required to correlate its new bio-sensor random samples of size n drawn from this population would cover method for whole blood potassium concentrations the true mean m. Asis expected, the 99% CI is wider than the 95% with results obtained from its existing method, which CI; the smaller the range of values considered, the less confident uses an ion selective electrode analyzer. The following one can be that the interval covers m. data were obtained on paired samples (note: the num-ber In some cases, sigma for a given population is known and of sample pairs has been reduced below the recom-mended one may be interested in determining a mean for a smaller sample number for comparison of methods to simplify group and assessing whether its sample meanfalls within the popu-lation the problem): confidence interval. Example 3-2 illustrates this application 1. Determine the following statistics from the data below: of confidence interval for a cholesterol study in women. bias Standard deviation of the difference EXAMpLE 3-2 Standard error of the mean of differences Estimating a 95% CI for M when S is known p-value The laboratory staff is interested in the distribution of 2. Assuming ta = 0.05 two-tailed distribution, should serum cholesterol levels for all females in the united the null hypothesis, H0: m1 = m2, be accepted or States who are hypertensive and smoke. The distribu-tion rejected? is approximately normal with an unknown mean, m, and the standard deviation, s, is 50 mg/dL. The labo-ratory Sample K+ meq/L Biosensor K+ meq/L ISE staff is interested in estimating the mean serum Number Technique Technique cholesterol for this population. before the staff members that the go interval x- 1.96(50/2N) tox+1.96(50/2N) out and select a random sampling, the probability 1 4.5 4.6 includes the true population mean, m, is 0.95. The staff 2 4.0 4.0 draws 14 samples from the population of hypertensive 3 3.9 4.0 smokers, and these women have a mean cholesterol level 4 3.8 3.9 of 220 mg/dL. based on this sampling, 220 mg/dL -1.96(50/214) =199 5 5.0 5.1 220 mg/dL +1.96(50/214) =246 6 7 5.5 3.0 5.7 3.2 8 3.4 3.6 9 3.1 3.3 10 4.2 4.2 Therefore, the group is 95% confident that the limits of 199246 mg/dL coverthe true mean,m. 11 4.4 4.7 12 5.2 5.3 Errors of Inference 13 5.1 5.0 A null hypothesis can be accepted or rejected. Either there is no 14 3.7 3.9 difference betweengroups or there is really aninequality (such as 15 4.4 4.5 the difference between two groups). There are two types of errors, identified as Type I (alpha) and Type II (beta), associated with an 16 3.2 3.3 inappropriate conclusion of hypothesistesting. 17 2.8 3.0 A type I error is defined as erroneously rejecting the null 18 6.0 6.3 hypothesis or proclaiming a difference to exist when one does not. 19 5.8 6.2 Atype I error is also known asthe levelof significance. It is often created whenthe level of significance (p-value) or the amount of 20 4.6 4.6 risk that oneis willing to take in any test of the null hypothesis is inappropriate. Atype II error is defined as erroneously accepting the null hypothesis, with the result that a real difference is not detected. F-Test In other words,there mayactually be a difference betweenthe The F-test or F-ratio is the ratio of the variance (s2) calculated populations represented by the sample group, but you mistakenly for both sets of data. A table of critical F-ratio values is used to conclude that there is not. determine if the null hypothesis should be accepted or rejected 56 CHAPTER 3 LAboRAToRy STATiSTiCS, METHod dEvELoPMEnT, And QuALiTy ConTRoL Or, simply stated, is the difference between the variances such that regression of y on x. Thefollowing arethe assumptionsunderlying chance alone could well account for it? the simple linear regression model.4 The statistical procedure associated with the F-test is similar Values of the independent variable x are said to be fixed. to that for the t-test. The differencesinclude the following: (1) the nature of the null hypothesis differs between the F-test and the The variable xis measured without error. p-test, (2) eachset of data mustaccount for numbers of degrees of For eachvalue of x, thereis a subpopulation of y-values. freedom, and (3) a unique probability table must be used. The variancesof the subpopulation of y are all equal. The means of the subpopulation of y all lie on the same Linear Regression and Correlation straight line. Regression The y-values are statistically independent. Regression analysis is useful in assessing specific aspects of the relationship between variables, and the ultimate objective is to pre-dict A least squares or best-fit straight line is included in or estimate the value of one variable based on a given value Figure 3-4. The graph shows that there is an association between of the second variable. Several examples of regression analysis are the two variables. In such cases,it is often desirable to use a math-ematical discussed in this chapter. description of such an x-yrelationship. Thesimplestsuch expression is the general equation for a straight line: Correlation Correlation statistics measure the strength of the relationship between variables. When measures of correlation are computed Y = a + bX (Eq. 3-5) from a set of data, we are interested in the degree of correlation between the variables. This linear model or simple linear regression formula Linear Regression by Least Squares contains two parameters: the intercept a and slope b. The data presented in Table 3-5 ? show that a nearly linear relationship Regression analysis is commonly used in the comparison of two between creatinine nonenzymatic Jaffe reaction (X) and creatinine methods ortwo instruments and to evaluate the linearity of an instru-ment or method. Regression calculations provide a simple and gen-eral descriptive statement relating one set of observations to another. ? TABLE 3-5 Least squares regression analysis of Linear regression by the method of least squares positions a creatinine Jaffe reaction measurements by two dif-ferent straight line among the points on the graph in such a way that the methods. sum of the squares of the vertical distances from each point to Creatinine (mg/dL) Creatinine (mg/dL) the fitted line is the smallest value possible. In Figure 3-4 , two Nonenzymatic Jaffe Enzymatic Jaffe sets of data, representing variables x and y, are plotted on a graph. Sample Number Reaction (x-axis) Reaction (y-axis) The data sets represented by closed circles form a scatterplot. The 1 0.9 0.9 x-variable is referred to asthe independentvariable becausethe inves-tigator 2 1.1 1.0 frequently controls it. The y-variable is called the dependent 3 1.0 0.8 variable,and the experimenter measures it to determinethe effect 4 1.3 1.2 of the independent variable; thus, the terminology refers to the