1. Group Comparisons copy.docx
Document Details
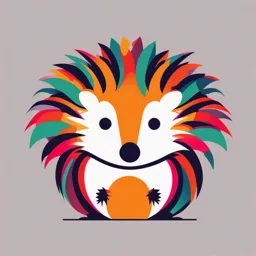
Uploaded by NoiselessPeace
Full Transcript
CRITICAL READING: CORNELL NOTES Group Comparisons Name: Date: 14 September 2023 Section: Lecture 1 Period: Questions/Main Ideas/Vocabulary Notes/Answers/Definitions/Examples/Sentences Comparing Two Groups Is there a difference in the academic performance of male and female high school...
CRITICAL READING: CORNELL NOTES Group Comparisons Name: Date: 14 September 2023 Section: Lecture 1 Period: Questions/Main Ideas/Vocabulary Notes/Answers/Definitions/Examples/Sentences Comparing Two Groups Is there a difference in the academic performance of male and female high school students? When participants are in one group or the other (not both). Independent samples t-test. Is there a difference in perfectionism ratings pre and post mindfulness intervention. When participants are in both groups. Related samples t-test. Comparing Three or More Groups Participants assigned to one of three test conditions: placebo, low alcohol and high alcohol. Comparing performance on a measure of cognitive functioning (Self-Ordered Pointing Task). Univariate Analysis of Variance (ANOVA). Parametric Tests t-tests and ANOVA are parametric tests. They rely on some important assumptions about the nature of the data we are applying the test to. If these assumptions aren’t met, then the results of the analyses may not be accurate. Assumptions of ANOVA & t-tests The dependent variable is normally distributed. The variances of the groups are approximately equal (homogeneity of variance). The group sizes are approximately equal. Normal Distribution Symmetrical distribution of values. Most values are close to the mean of the distribution. Some psychological variables are normally distributed (or close to normally distributed). Many are not. Skewed Distributions When data are skewed, the mean isn’t an accurate indicator of the central tendency of the distribution. This is important for t-tests and ANOVA as the mean is an essential part of the analysis. Testing for Normality Assumption Visualising the data using a histogram. Looking at the skew statistic. Using the Shapiro-Wilk test of normality. Homogeneity of Variance Variance describes the spread of a distribution. We can use the Levene’s test to determine if the homogeneity of variance assumption is met. Levene’s test tests the null hypothesis that the variances are equal. If the test is significant, then we haven’t met the assumption of homogeneity of variance. Near-Equal Group Sizes Just need to use your best judgement, there is no test. The larger the overall groups, the less of an issue this is. If we had three groups with sizes of 80, 95 and 90, I wouldn’t be too bothered. But if we had the same absolute differences with group sizes of 20, 35 and 30, I would certainly be worried. Unequal group sizes are particularly problematic if we are also violating the assumption of homogeneity of variance. Parametric Tests Are Robust If one of the assumptions of ANOVA and t-tests are violated, we don't need to be too worried. If two or more of these assumptions are violated, we need to use alternative forms of analysis. Wilcoxon Test The nonparametric equivalent of the independent samples t-test is known as the Wilcoxon test. Unlike the t-test, which relies upon means and standard deviations to test for a difference between groups, the Wilcoxon test is based upon the ranks of the two groups. We report the Wilcoxon test using the W statistic. We can report the effect size of this difference by converting the W to an r value. Example: A Wilcoxon test indicated a significant difference between the two conditions, and the size of the effect was strong (W = 161.6, p < .001, r = .51). Kruskal-Wallis Test The nonparametric word equivalent to ANOVA is the Kruskal- Wallis test. The Kruskal-Wallis test is a generalisation of the Wilcoxon test, for cases where there are three or more groups that need to be compared. We report a Kruskal-Wallis test using the chi-squared statistic: Example: A Kruskal-Wallis test indicated that there was a significant difference in performance between the three conditions (χ2 [2] = 28.61, p < .001). Why Don’t We Use These Tests All the Time? While these nonparametric alternatives are robust to violations of the assumptions of normality and homogeneity of variance, they are less powerful than parametric tests. This means that for a nonparametric test to find a significant difference, the effect size needs to be stronger than what would be needed for a parametric test. Cecause of this, researchers tend to prefer the parametric tests. But if it's not appropriate to apply a parametric test, then we should certainly use the nonparametric equivalent.