Gender Gap in Competitive Chess Across Countries (2021) PDF
You are viewing a limited preview. Upgrade to access the full document.
Upgrade NowDocument Details
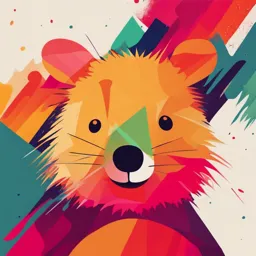
Uploaded by PlayfulVision
Saint Mary's University
2021
Maryam Dilmaghani
Tags
Summary
This journal article by Maryam Dilmaghani examines the gender gap in competitive chess across countries. It explores the relationship between a country's history of a command economy and the representation of females at competitive chess' top levels. The analysis utilizes data from the World Chess Federation and other development indicators.
Full Transcript
Journal of Comparative Economics 49 (2021) 425–441 Contents lists available at ScienceDirect Journal of Comparative Economics journal homepage: w...
Journal of Comparative Economics 49 (2021) 425–441 Contents lists available at ScienceDirect Journal of Comparative Economics journal homepage: www.elsevier.com/locate/jce The gender gap in competitive chess across countries: Commanding queens in command economies Maryam Dilmaghani * Department of Economics, Sobey School of Business, Saint Mary’s University, Halifax, Nova Scotia, Canada A R T I C L E I N F O A B S T R A C T JEL Classification: Currently, the World Chess Federation lists 1,643 male Grandmasters against only 37 females. J16 While the greater attainment of men in competitive chess is well known, the lesser known fact is Z20 that the gender gap in competitive chess varies strongly across countries. For instance, for every P20 100 male players with the attainment level of Candidate Master, there are about 48, 47, 38, 4, 3, Keywords: and 2 female players in Vietnam, Georgia, China, US (Japan), France (Sweden), and Denmark Gender (Finland), respectively. Noting these large gender discrepancies, this paper constructs a cross- Gender Role Attitudes country panel to explore the determinants of these gaps. Controlling for main economic devel Competitiveness Socialism opment indicators and several measures of gender equality, a legacy of command economy is Political Regimes found to be the most significant predictor of a smaller gender gap in competitive chess across countries. Various explanations and their implications are discussed. 1. Introduction Competitive chess is a male domain (Chabris & Glickman 2006; Bilalić et al. 2009; Blanch 2016). The July 2019 report of the World Chess Federation (FIDE) lists 1,643 male Grandmasters against only 37 females (FIDE 2019). This dramatic gender gap is one of the largest observed among the male-dominated domains, which include the STEM fields, financial trading, and senior management. The cross-disciplinary literature dedicated to the root-causes of the lack of female representation at the top levels of certain domains has been active for many years (Bertrand & Hallock 2001; Hyde & Mertz 2009; Lippmann & Senik 2018), where the two explanations of biological and socialization differences between men and women are put forth. Notwithstanding the interaction between the two explanations, the biology-based explanation implies that little or no difference in gender gaps must be observed across countries. On the other hand, given the prevailing sociocultural differences around the world, the socialization-based explanation is favoured if the gender gaps are found to vary across countries. Interestingly, a close look at the FIDE data reveals a pattern of large cross-country variations. For instance, the 2012 to 2018 FIDE data indicate that for every 100 males with the attainment level of Candidate Master, there are about 48, 47, 38, 4, 3, and 2 females in Vietnam, Georgia, China, US (Japan), France (Sweden), and Denmark (Finland), respectively. In this attainment level, the global average is 6 females for every 100 males. Many would expect a smaller gender gap in the Western developed economies than in the rest Compliance with Ethical Standards: The author declares no conflict of interest. This study was not funded. This article does not contain any studies with human participants or animals performed by any of the authors. Informed consent: Not applicable. The manuscript has not been published elsewhere and is not under submission elsewhere. The manuscript has a single author. * Corresponding author: 923 Robie Street, Halifax, NS, B3H 3C3, Canada E-mail address: maryam.dilmaghani@smu.ca. https://doi.org/10.1016/j.jce.2020.09.006 Received 27 June 2019; Received in revised form 16 September 2020; Accepted 17 September 2020 Available online 22 September 2020 0147-5967/© 2020 Association for Comparative Economic Studies. Published by Elsevier Inc. All rights reserved. M. Dilmaghani Journal of Comparative Economics 49 (2021) 425–441 of the world. However, the above figures imply a much greater relative female presence at the top levels of competitive chess in countries with a legacy of central planning than in the Western world. A review of the extant literature indicates that this pattern is not accidental. In the literature dedicated to the effects of socialist institutions and policies on the labour market, there is some consensus that women under communism had a significant gender equality advantage in comparison with women in the geopolitical West (Dijkstra & Hanmer 2000; Pollert 2003; Esping-Andersen 2013). More recent studies provide compelling evidence for the persistence of these differences post-disintegration of the Soviet bloc, within and beyond the labour market (Amini & Commander 2012; Lipp mann et al. 2020). For instance, Lippmann and Senik (2018) show that among German pupils, the gender gap in mathematics is smaller in regions formerly part of East Germany. Consistently, Campa and Serafinelli (2019) show that women from the former East Germany place a greater importance on career success than women from the former West Germany. Informed by this literature, the present paper explores the origins of the significant differences across countries regarding female attainment in competitive chess as compared with men. This investigation is instrumental in shedding light on several actively researched economic questions. First, the present study contributes to a better understanding of gender difference in competitiveness. Gender difference in competitiveness is attributed to both innate and contextual factors (Gneezy et al. 2003; Niederle & Vesterlund 2007; Gneezy et al. 2009; Frick 2011; Zhang 2019). If this gender difference is affected by contextual factors, then the gender gap in chess must also vary across countries. The comparative examination of the gender gap in chess provides further evidence as to whether differences between men and women in competi tiveness are affected by societal factors. The second contribution of this paper is to the research agendas focused on documenting the cross-country differences in female representation at the top levels of male-dominated fields such as mathematics (Guiso et al. 2008; Hyde & Mertz 2009; Kane & Mertz 2012). The third contribution of this paper is to the literature that examines the effects of so ciopolitical institutions on gender-role attitudes and beliefs, and the persistence of these effects (Alesina & Fuchs-Schündeln 2007; Amini & Commander 2012; Lippmann & Senik 2018; Campa & Serafinelli 2019). Finally, much of the related literature has relied on laboratory or field experiments, whereas this paper enriches the extant evidence by using real-world data. The World Chess Federation, whose acronym FIDE comes from its French name Fédération Internationale des Échecs, reports the official Elo ratings of chess players by their country of flag, multiple times a year. The Elo rating is a reliable measure of a player’s chess skill, and is determined by the outcomes of FIDE-sanctioned matches. The 2012 to 2018 FIDE data of chess players’ Elo ratings, which contain more than 1.5 million individual observations, are complemented by other sources, such as the World Development Indicators, to construct a cross-country panel. The paper first showcases stylized facts on the level of gender equality in competitive chess around the world, which are both surprising and instructive. Subsequently, regression analysis is used in order to identify national-level variables associated with female participation and attainment in competitive chess. Controlling for variables such as GDP per cap ita, economic growth rate, and female labour market attainment, having a history of command economy is found to be the most significant predictor of a smaller gender gap in competitive chess, especially at high levels of attainment. The sheer extent of the gaps, unaccounted for by country-level economic performance indicators and observed measures of gender equality, provides support for the socialization-based explanations of female scarcity at the top ranks of male-dominated fields (Guiso et al. 2008; Kane & Mertz 2012; Lippmann & Senik 2018). Moreover, the results produce further evidence in line with the growing scholarship on the persistence of the effects of political regimes on gender-role attitudes (Amini & Commander 2012; Campa & Serafinelli 2019; Lippmann et al. 2020). The remainder of this paper is organized as follows. The next section reviews the related literature. Section III is concerned with the data and descriptive statistics. The methodology is outlined in Section IV. The results are presented in Section V. A discussion and the concluding remarks follow. 2. Literature Review Given the nature of chess as a deterministic strategic game and the thorough documentation and digitization of chess matches, chess data are potentially fruitful for the investigation of demographic differences in latent individual traits, such as risk taking, time preference, and competitiveness. Accordingly, chess data are increasingly used in economic studies (Backus et al. 2016). For instance, using chess data, Gerdes and Gränsmark (2010) found that female chess players are more risk-averse than their male counterparts, whereas Gränsmark (2012) reported that men are more impatient while women are more time inconsistent. Combining chess data with ratings of chess players’ attractiveness, Dreber et al. (2013) found that male chess players choose significantly riskier strategies when they play against attractive females. More recently, Linnemer and Visser (2016) examined the determinants of self-selection into different ranks of chess competitions, while Dilmaghani (2019) reported that female players underperform male players of the same skills when the chess game is played under time constraint. As the above review shows, economists have not yet been concerned with documenting and explaining the strikingly low number of female players at the top skill levels of competitive chess. In psychology, the small number of women among top chess players (Bilalić et al. 2009; Blanch 2016) has been viewed within the general context of female slight representation at the top levels of highly competitive and demanding activities, such as the STEM fields (Xie & Shauman 2003; Ceci & Williams 20, 2007), engineering (Hunt 2016), and senior management (Heilman & Okimoto 2007). The recent development of the rather serious field of “electronic sports” has produced another domain in which female representation is very low in professional and top ranks (Ruvalcaba et al. 2018). Electronic sports (e-sports) refers to the competitive play of video games. While the prevalence of casual videogame playing does not vary by a very large measure across genders (Duggan 2015), there are no women among the top 300 professional players with positive earnings from the e-sport competitions (E-sports Earnings 2018). The female scarcity in e-sports seems more dramatic than that observed in chess, where currently, Yifan Hou is the one and only woman among the top 100 active players (FIDE 2019). The literature has offered several explanations for female scarcity in certain domains, ranging from innate differences in intellectual 426 M. Dilmaghani Journal of Comparative Economics 49 (2021) 425–441 and psychological attributes (Jianakoplos & Bernasek 1998; Benbow et al. 2000; Spelke 2005; Cárdenas et al. 2012) to behavioral differences acquired through socialization (Massa et al. 2005; Andreescu et al. 2008), as well as the existence of overt and covert sociocultural and institutional barriers (Ćorić 2018). Across the studies, little difference between the average scores of men and women on intelligence and aptitude tests has ever been found (Gneezy & Rustichini 2004; Hyde & Mertz 2009). But, the top achievers in competitive and demanding domains are consistently found to be males (Kane and Mertz 2012). For instance, the average SAT per formance of females is only slightly below that of males, due to the test’s math portion (Gneezy & Rustichini 2004). But, in the top ranks of science and mathematics, a disproportionately large gender gap in representation is observed (Bilalić et al., 2009). Likewise, among the highest-paid executives of a large set of US firms, only 2.5% were women (Bertrand & Hallock 2001). Contrasted with the gender wage gap, this representation gap is much more drastic (Goldin, 2006). As the above examples illustrate, to reasonably examine the gender gap in male-dominated domains, distinctions must be made among three interrelated issues of mean attainment differences, the differential participation rates, and the low female representation at the top levels. Statistically, differences in participation rates can partially explain the differences in the extreme values (Chabris & Glickman, 2006; Bilalić et al. 2009). Using a sample of German chess players, Bilalić et al. (2009) showed that 96% of the observed extreme value differences are expected given the much greater number of men who play chess. However, the estimations reported in Bilalić et al. (2009) have been challenged by Knapp (2010) and Blanch (2016), who suggest that the narrower female “pipeline” is unlikely to fully account for the large attainment gaps at the top levels. The participation gaps are most relevantly examined in the scholarship on the gender differences in the tendency to compete (Gneezy et al., 2003). Gneezy et al. (2003) were first to document that competition depresses female performance while it has an enhancing effect on males. Following Gneezy et al. (2003), a growing number of studies report that women with the same preferences as men and who are equally capable, are less likely to compete for the opportunities which maximize their productivity (Niederle & Vesterlund 2007; Gneezy et al. 2009; Booth & Nolen 2012; Cárdenas et al. 2012; Dreber et al. 2014; Eckel & Füllbrunn 2015; Zhang 2019). Across these studies, between 20% to 50% competitiveness gap is observed between men and women. There are economic efficiency losses associated with this pattern (Zhang 2019). Regarding the scarcity of women in the top managerial positions, the glass ceiling metaphor is used to refer to the invisible, discriminatory barriers against female access to the top (Oakley 2000; Ćorić 2018). Perhaps as a result of a more fluid way in which managerial performance can be evaluated, this literature is heavily focused on the effects of discrimination and negative gender stereotypes (Swim et al. 1989). This literature indicates that, in the so-called male domains, even the unambiguous proof of women’s competence can create problems such as social rejection (Heilman & Okimoto 2007), because such successful women are perceived in violation of the normative “oughts” of their gender (Heilman & Okimoto 2007; Ćorić18). These detrimental consequences, although specifically documented for top managerial positions, appear applicable to any context, and may be responsible for the pipeline problem in other male-dominated domains. In the same vein, the psychology literature on the “stereotype threat” (Steele 1997) ties the existence of negative stereotypes to female underperformance in mathematics and chess (Niederle & Vesterlund 2010; Lippmann & Senik 2018; Stafford 2018). Accordingly, various measures of contextual and institutional gender inequality have been shown to correlate with the size of the gender gap in mathematics and science, as measured by the PISA scores (Fortin et al. 2015; Guiso et al. 2008; Nollenberger et al. 2016), International Mathematics Olympiad data (Hyde & Mertz, 2009; Kane & Mertz 2012), and American state-level educational scores (Pope & Sydnor 2010; Else-Quest et al. 2010).1 The Elo ratings, as a measure of a player’s skill, do not lend itself to a subjective and biased interpretation. But, the extant bias against successful women in the male domains can possibly suppress the participation rates, and negatively affect the performance of the existing players (Guiso et al. 2008; Reuben et al. 2014). In Western liberal democracies, which score substantially higher than the global average on various measures of gender equality (Dilli et al. 2019), negative gender stereotypes are believed to be less prevalent (Ćorić 2018). Therefore, it is expected to see a greater female presence at the top ranks of male domains in these countries. But, Ćorić (2018) finds that many developing countries stand better with respect to the share of women in senior management than most Western liberal democracies. The same type of comparative evidence is reported for countries with a legacy of state-socialism (Alesina & Fuchs-Schündeln 2007; Amini & Commander 2012; Bauernschuster & Rainer 2012; Campa & Serafinelli 2019; Lippmann et al. 2020). Notably, Lippmann and Senik (2018), examining the gender gap in mathematics performance in Germany, show that girls’ mathematics disadvantage compared with boys is largely reduced in regions previously part of the former East Germany. They further demonstrate that the shrinkage of the gap is due to differing attitudes among girls, namely their higher confidence and greater competitiveness. Against this backdrop, the present paper uses a global sample to investigate how the gender gap in competitive chess varies across countries. 3. Data and Descriptive Statistics The chess-performance data used in this paper are retrieved from the FIDE website (FIDE 2019). Multiple times a year, the FIDE website publishes the list of players who have achieved an Elo rating of 1000 or above in FIDE-sanctioned tournaments. These tournaments, coordinated by national chess federations, are in-person matches where opponents are randomly assigned. FIDE-sanctioned competitions can be both mixed-gender or single-gender. But, the inclusion in the FIDE mixed-gender rating lists requires the establishment of the rating in mixed-gender tournaments, as Elo ratings are only valid in the pool they are established. The Elo rating is a measure of chess skill, determined by tournament performance of a chess player, first proposed by Arpad Elo (Elo 1978), a Hungarian-American physicist. The Elo rating increases or decreases, depending on the outcomes of matches between rated players. 1 Note that while some claim chess training contributes to mathematics performance, there is no conclusive evidence that the observed correlation is not spurious (Bilalić et al. 2007; Sala & Gobet 2016). 427 M. Dilmaghani Journal of Comparative Economics 49 (2021) 425–441 Every match, even if it ends in a draw, generally causes a change in the Elo ratings, in a zero-sum manner. The allocation of the Elo rating points after a match is based on the rating discrepancies between the two opponents. If a player with a lower Elo rating beats a player of a higher Elo rating, this match results in large losses for the more skilled player and equally large gains for the less-skilled player. If a match ends in a draw, provided that there are rating discrepancies between the two opponents, rating changes ensue. The FIDE lists include name, gender, country of flag, and the year of birth of the players. This paper uses 2012 to 2018 data, as the FIDE lists were not available in consolidated electronic formats for years before 2012, and the controls were not available post-2018. Since lists published in a given year are nearly identical for most players, the FIDE reports for July in every given year are used in this study, with a cumulative number of 1,551,866 observations. This breaks down to 152,995 players in 2012 (the smallest dataset) and 310,903 players in 2018 (the largest dataset). For about 0.2% of the players (between 200 and 600 observations, depending on the year), the gender was missing or was recorded differently over the years. These observations were dropped. The FIDE lists also include the official titles of the players, if applicable. These titles are Grandmaster (GM, minimum Elo rating of 2300), International Master (IM, minimum Elo rating of 2200), FIDE Master (FM, minimum Elo rating of 2100) and Candidate Master (CM, minimum Elo rating of 2000). The US Chess Federation offers the alternative title of “Chess Expert” to those with an Elo rating of 2000 and above. The FIDE lists include up to 192 independent chess federations (country of flag) across the years. These federations had at least one FIDE-rated player. The flags are based on the classification of the International Olympic Committee (IOC). Hence, a few of these flags do not pertain to a sovereign country, such as Scotland and Wales. The participants under these flags are assigned to their respective sovereign countries.2 Table 1 provides more information on the variables and the countries in the sample. The FIDE tournament participants are not a random sample of chess players in a given population. While it is unlikely that players with high levels of skill systematically did not participate in FIDE-sanctioned tournaments, it remains a possibility regarding those with average and low skills. Specifically, the number of FIDE-sanctioned tournaments per year varies by country. Therefore, in some countries, it is relatively less costly to participate in such tournaments, potentially enticing the participation of a greater number of low-skilled players. If there are gender differences in this regard, biases can be introduced in the estimation of the gender gaps in the central tendency measures, such as means and medians. An Elo rating of 1000 (the minimum rating in the FIDE lists) indicates a low- skill level, and the tournament participants with this skill-level are likely a non-representative sample of the population with chess knowledge, raising the concern for a selection bias. To remedy this issue, players with an Elo rating below 1400 are excluded. An Elo rating of 1400, arguably, indicates the attainment of a semi-serious level of skill. For instance, the Internet Chess Club (ICC), one of the oldest online chess interfaces, starts any new member with the default rating of 1400 (ICC 2018). Rather similarly, Lichess.com, one of the most popular free chess websites, starts new members with a default rating of 1500 (Lichess 2018).3 These default ratings pertain to the medians observed among the website users. Table 2 reports the player counts by skill level, for a selection of countries, grouped by geopolitical regions. As shown in Table 2, in some countries, above 95% of all participants pass the threshold of +1400, while in others, mainly European countries where the number of FIDE-sanctioned tournaments is relatively high, the share is about 80%. With these cross-country differences, the exclusion of the listed players with below 1400 Elo ratings seems reasonable. Note that this exclusion is not consequential for the estimation of the cross-country gaps in female representation at the top levels of performance, the main focus of this paper. Regarding the measures of central tendency, alternative estimates are produced including all players, with no effect on the conclusions. While the existence of a gender gap in chess is widely reported, its measurement has not yet been the subject of scholarly attention. Since chess is an individual game, even in popular sources, there is no accurate information regarding the relative strength of countries in chess by gender. Popular media sources tend to rank countries by computing the average Elo ratings of the top 10 players or the number of Grandmasters (Chess.com 2019). Based on the average ratings of the highest rated Grandmasters, the Russian Federation, China, and Azerbaijan currently occupy the top three spots.4 But, using such an approach to measure the gender gaps in chess across countries would be misleading, as the average values generally mask the representation gaps along the chess-skill scale. In this paper, various measures of the gender gap in chess performance are created, in order to capture both the differences in the central tendency and the gaps in representation. First, the general participation gaps are assessed through the computation of the female-to-male ratio of the listed players. As previously indicated, to do away with the selection bias, those rated below 1400 Elo points are dropped. This ratio should be taken as an indicator of the gender gap in general interest in chess. The individual-level data indicate 9 female participants per 100 males. Second, the ratio of female-to-male mean Elo ratings is computed, with those rated below 1400 Elo points excluded. Based on the individual-level data, the ratio is 0.951 worldwide. Third, the female-to-male ratio of the count of players rated at 1500, 1600, 1700, 1800, 1900, and 2000 (the minimum Elo rating required for FIDE Candidate Master title/US Chess Expert title) are computed. Female representation gradually falls, as the skill threshold is tightened. For example, for every 100 males rated at 1700 Elo points and above, there are 7.3 females worldwide. At 2000 Elo points and above, the worldwide ratio falls to 6.4 females per 100 males. Rather drastic 2 The other such federations are the US Virgin Islands, British Virgin Islands, Puerto Rico, Macao, and Hong Kong. These regions, which had separately reported World Development Indicators, appeared to be too culturally different to be pooled with their sovereign countries. But, no separate Global Gender Gap Index, an explanatory variable in the regressions, is reported for them. Given the missing data, the players under these federations are dropped from the sample. 3 In 2019, Lichess.com switched to the Glicko rating system, proposed by Mark Glickman (Glickman 1995). The Glicko system is a modification of the Elo rating system, not used by FIDE. 4 According to Chess.com, the top 9 countries of 2019 are as follows: 1. Russian Federation; 2. China; 3. Azerbaijan; 4. USA; 5. India; 6. Ukraine; 7. France; 8. Poland; 9. England (Chess.com 2019). 428 M. Dilmaghani Journal of Comparative Economics 49 (2021) 425–441 Table 1 Definitions and Data Sources Formerly Communist Albania; Bosnia and Herzegovina; Bulgaria; Croatia; Czech Republic; Hungary; Macedonia; Montenegro; Poland; Romania; Serbia; Slovak Republic; Slovenia. Post-Soviet Armenia; Azerbaijan; Belarus; Estonia; Georgia; Kazakhstan; Kyrgyz Republic; Latvia; Lithuania; Moldova; Russian Federation; Tajikistan; Turkmenistan (dropped in the regressions as a result of missing GGGI); Ukraine; Uzbekistan. Other EU Austria; Belgium; Cyprus; Denmark; Finland; France; Germany; Greece; Ireland; Italy; Luxembourg; Malta; Netherlands; Portugal; Spain; Sweden; United Kingdom. Other OECD Australia; Canada; Chile; Iceland; Israel; Japan; Republic of Korea; Mexico; New Zealand; Norway; Switzerland; Turkey; United States. Other Countries Angola; Afghanistan; Algeria; Angola; Argentina; Bangladesh; Barbados; Bolivia; Botswana; Brazil; Burundi; Brunei Darussalam; Cambodia; Cameroon; (China); Colombia; Costa Rica; Cote d’Ivoire; (Cuba); Dominican Republic; Ecuador; Egypt; El Salvador; Ethiopia; Fiji; Gabon; Ghana; Guam; Guatemala; Guyana; Haiti; Honduras; India; Indonesia; Iran; Iraq; Jamaica; Jordan; Kenya; Kuwait; (Laos); Libya; Lebanon; Lesotho; Liberia; Madagascar; Malawi; Malaysia; Maldives; Mali; Mauritania; Mauritius; (Mongolia); Morocco; Mozambique; Namibia; Nepal; Nicaragua; Nigeria; Oman; Pakistan; Panama; Papua New Guinea; Paraguay; Peru; Philippines; Qatar; Rwanda; Saudi Arabia; Senegal; South Africa; Sri Lanka; Suriname; Timor-Leste; Thailand; Trinidad and Tobago; Tunisia; Uganda; United Arab Emirates; Uruguay; (Vietnam); Yemen; Zambia; Zimbabwe. Countries with no Player Rated at In these countries, for one or several year(s), no player was rated at 2000 Elo points and above. Hence, the representation þ2000 ratio(s) could not be defined. The list is Bahamas; Brunei Darussalam; Republic of Congo; Cape Verde; Eritrea; Djibouti; Guyana; Laos; Lesotho; Maldives; Nauru; Palau; Sierra Leone; Solomon Islands; Sao Tome and Principe; Swaziland; Tanzania; Timor-Leste. Countries with no Female Rated In these countries, for one or several year(s), no female was rated at 1400 Elo points or above. Hence, the mean female at þ1400 attainment is assumed unobserved. The list is Afghanistan; Bhutan; Burundi; Cambodia; Côte d’Ivoire; Cameroon; Haiti; Saudi Arabia; Kuwait; Mali; Mauritius; Papua New Guinea; Senegal; San Marino; Somalia; Togo. GGGI The Global Gender Gap Index, devised and published by the World Economic Forum. Elo rating A rating which indicates the relative strength of players, computed based on their tournament outcomes. Chess data source The Standard Chess rating reports, published by FIDE. Economic data source The World Development Indicators, published by the World Bank. Note: More information on FIDE data is available at: http://www.fide.com/ cross-country differences emerge regarding these ratios. For instance, for every 100 males in the +1700 Elo level, there are about 61, 49, 42, 39, and 31 females in Vietnam, Mongolia, Georgia, China, and Turkmenistan. In contrast, for every 100 males in the +1700 Elo level, there are about 1, 2, 3, 4, and 5 females in the 5 Nordic countries of Denmark, Finland, Sweden, Norway and Iceland, respectively. The latter countries regularly occupy the top spots in various gender equality indices, such as the Global Gender Gap Index, used in this paper (WEF 2019). If the skill threshold is tightened to +2000 Elo points, Vietnam (48 females/100 males), Georgia (47 females/100 males), China (37 females/100 males) rank the highest in the world. The corresponding figures for UK, US, and France are 5, 4 and 3 females per 100 males, respectively. Table 2 contains more of such descriptive statistics. Figures 1 to 3 provide visuals for the descriptive statistics. Computing 2012-2018 averages for each country, Figure 1 plots the mean female Elo ratings against the mean male Elo ratings. This figure contains two sets of data-points: solid grey circles for the raw data and hollow circles for when the players rated below 1400 Elo points are excluded. As shown in Figure 1, male and female ratings are positively correlated across countries. The correlation is stronger when players with low ratings are excluded. Averaged over 2012- 2018, Figure 2 shows the cross-country count ratios of those who have attained at least 1700 Elo points against those rated at 1400 and above. Worldwide, 65.1% of the listed players are rated above 1700 Elo points. Hence, this threshold is not an exclusive attainment level, but indicates a “respectable” chess skillset. As shown in Figure 2, there is a strong positive correlation between the gender ratio at the general participation level (+1400 Elo points) and the attainment of a “respectable” standing (+1700 Elo points) across countries. Figure 3 shows the gender ratios at +2000 Elo points, for countries situated at the top quartile of the sample. The +2000 threshold corresponds to a high skill level, as it is the eligibility point for the coveted FIDE title of Candidate Master (and the Chess Expert title in the US). Worldwide, only 30.6% of the listed players are rated above 2000 Elo points. As shown in Figure 3, Australia is the only “Western” developed country present in the top quartile. Appendix Figures show worldwide heatmaps for the count ratio of players rated above 1400 Elo points, the female-to-male mean Elo rating ratio, and the count ratio of players rated at 2000 Elo points and above. As indicated by the above descriptive statistics, countries with a legacy of central planning have substantially more gender-equal outcomes in competitive chess than the rest of the world. In Table 2 and later regressions, countries with a history of central planning are grouped in two clusters of Formerly Communist Countries of Europe (e.g. Bulgaria, Poland, and Romania), and Post-Soviet countries (e.g. Russian Federation, Azerbaijan, and Ukraine), alongside China, Vietnam, Cuba, and Mongolia, which are considered separately. There was no North Korean chess player in the FIDE lists. The People’s Democratic Republic of Mongolia was established in 1924. In the 1990s, the country transitioned to a market economy and “People’s Democratic” was dropped from the name of the country (Pastore 2010). Therefore, Mongolia had a lengthy exposure to state-socialism. In addition, the Lao People’s Democratic 429 M. Dilmaghani Table 2 Descriptive Statistics, Selected Countries Player Count, All Player Count, +1400 Player Count, +1700 Player Count, +2000 Mean Rating, +1400 Female Male Ratio Female Male Ratio Female Male Ratio Female Male Ratio Female Male Ratio China 221 524 0.420 209 507 0.412 173 451 0.387 124 332 0.374 2036 2114 0.963 Cuba 264 1,374 0.191 261 1,373 0.190 241 1,345 0.180 103 931 0.110 1952 2073 0.942 Laos 2 8 0.250 0 2 0 0 0 NA 0 0 NA 1259 1334 0.944 Mongolia 101 177 0.575 82 164 0.521 58 122 0.493 26 80 0.328 1879 1974 0.952 Vietnam 174 265 0.653 163 251 0.647 121 200 0.606 55 113 0.484 1873 1956 0.958 Post-Soviet 5,236 27,450 0.191 4,295 24,871 0.173 3,070 20,513 0.150 1,495 12,143 0.123 1867 1967 0.949 Azerbaijan 194 734 0.274 146 580 0.260 96 387 0.249 50 215 0.234 1852 1911 0.970 Georgia 242 565 0.437 195 491 0.404 139 329 0.422 85 180 0.473 1918 1906 1.001 Latvia 89 374 0.241 80 348 0.232 61 285 0.213 35 159 0.217 1907 1971 0.968 Moldova 78 350 0.223 69 310 0.225 45 217 0.207 20 107 0.183 1818 1892 0.961 Russia 3,424 19,005 0.177 2,800 17,313 0.162 1,995 14,512 0.138 929 8,589 0.108 1864 1985 0.939 Former Comm. 3,275 31,039 0.106 2,591 28,684 0.090 1,665 22,405 0.074 780 10,835 0.072 1833 1946 0.942 Bulgaria 179 1,085 0.164 154 1,014 0.152 107 825 0.130 54 498 0.109 1852 1976 0.937 Czech Rep. 309 5,480 0.056 266 5,273 0.051 170 4,278 0.040 77 1,714 0.045 1821 1902 0.957 Hungary 431 4802 0.089 356 4,494 0.079 239 3,480 0.069 125 1,663 0.075 1841 1903 0.968 Poland 1,087 6,570 0.165 758 5,519 0.138 456 3,793 0.120 180 1,819 0.099 1786 1861 0.959 430 Romania 386 2,534 0.151 287 2,229 0.130 181 1,526 0.119 88 653 0.135 1816 1858 0.978 Other EU 4,944 77,734 0.064 3,726 71,862 0.052 2,090 54,196 0.039 710 23,440 0.030 1788 1896 0.943 Austria 135 2,607 0.051 123 2,577 0.047 76 2,288 0.033 27 1,136 0.023 1798 1962 0.916 Finland 27 775 0.034 24 764 0.031 14 703 0.020 5 398 0.014 1789 2005 0.892 France 1,283 14,518 0.087 800 12,356 0.065 334 7,791 0.043 76 2,685 0.028 1693 1805 0.938 Ireland 27 370 0.077 24 364 0.068 16 305 0.054 8 122 0.068 1815 1942 0.935 Spain 831 16,367 0.050 588 14,840 0.040 294 10,115 0.029 72 3,496 0.021 1731 1829 0.946 Other OECD 1,513 17,217 0.088 1,180 15,632 0.075 698 12,167 0.057 279 6,345 0.044 1794 1916 0.936 Canada 81 910 0.089 75 900 0.084 52 807 0.065 27 508 0.053 1859 2025 0.918 Japan 14 130 0.104 13 129 0.098 8 117 0.071 2 54 0.037 1760 1958 0.899 New Zealand 30 268 0.110 25 258 0.098 19 223 0.083 6 109 0.051 1808 1959 0.924 Journal of Comparative Economics 49 (2021) 425–441 Norway 80 1556 0.054 69 1,375 0.052 42 953 0.044 15 409 0.036 1807 1883 0.961 USA 252 3845 0.064 231 3,801 0.060 180 3,495 0.052 98 2,260 0.044 1930 2046 0.943 Rest of World 5,818 46,539 0.125 3,243 33,808 0.096 1,485 21,136 0.070 414 7,997 0.053 1692 1869 0.905 Argentina 130 2,847 0.046 118 2,735 0.044 74 2,194 0.034 37 1,127 0.033 1831 1942 0.943 Colombia 270 1,460 0.186 244 1,423 0.174 166 1,205 0.140 43 481 0.089 1807 1923 0.940 India 1,851 16,445 0.113 732 8,936 0.082 307 3,855 0.080 107 1,257 0.085 1702 1711 0.995 Jordan 26 279 0.09 15 248 0.064 9 166 0.053 1 59 0.010 1748 1841 0.950 Uganda 10 57 0.179 8 56 0.146 1 51 0.029 0 32 0 1616 2073 0.780 Cross-Country 153 1,454 0.105 114 1,288 0.089 71 971 0.073 29 1,150 0.025 1761 1897 0.928 Note: The table shows averages over 2012 to 2018. The data source is FIDE. For more information, see http://www.fide.com/ M. Dilmaghani Journal of Comparative Economics 49 (2021) 425–441 Figure 1. Mean Elo Ratings by Gender Note: The labels are suppressed to keep the plot legible. The data source is FIDE. For more information, see: https://ratings.fide.com/ Figure 2. Female to Male Count Ratios Note: The labels are suppressed to keep the plot legible, except for countries far away from the general clusters. The country abbreviations are based on the International Olympic Committee Codes. The data source is FIDE. For more information, see: https://ratings.fide.com/ 431 M. Dilmaghani Journal of Comparative Economics 49 (2021) 425–441 Figure 3. Female to Male Count Ratio, +2000 Elo Points Note: The data source is FIDE. For more information, see: https://ratings.fide.com/ Republic (Laos) is a currently communist country.5 But, for Laos, FIDE-rated players were only present since 2016, and with mean ratings below 1400, regardless of gender. The exclusion criteria of +1400 Elo points means that Lao is not included in the main analyses.6 Two other geopolitical clusters are created, one comprised of EU countries not included in the Formerly Communist and Post-Soviet clusters (e.g. France, Italy, and Spain), and the second including OECD countries not included in the three previous clusters (e.g. US, Japan, and Australia). This clustering is rooted in geopolitics rather than a statistical analysis. But, as shown in Table 2, the variations across the countries in each cluster are limited. 4. Methodology To conduct a multivariate regression analysis, the FIDE lists are complemented by the World Bank data on socioeconomic- development indicators (World Bank 2019). As it is the case with any cross-country analysis with a global sample, there are data availability constraints to overcome. For instance, ideally, the measures of gender gaps in educational attainment should have been used in the regressions. Unfortunately, the World Bank data contained a large number of missing observations regarding educational attainment by gender. To remedy this data limitation, the World Economic Forum’s Global Gender Gap Index (GGGI) is retrieved to be used as a control (WEF 2019). The GGGI summarizes multiple dimensions of gender gaps in socioeconomic attainment in a single number, varying between 0 and 1. Namely, the GGGI captures differences between men and women regarding economic participation, educational attainment, political empowerment, and health (Kane & Mertz 2012). This index is considered more comprehensive than a few other comparable indices (Dilli et al. 2019), and has been used in several economic studies of the gender gap in mathematics (Guiso et al. 2008; Kane & Mertz 2012). Combining all these three data sources, a cross-country panel of gender gaps in competitive chess is constructed. While the data structure is a cross-country panel, the variables of interest, which are the country-cluster dummies, are time- invariant. Therefore, the standard panel fixed effects estimators are not implementable. Both panel random effects and pooled cross-section with country/country-cluster dummies can be implemented. But, these approaches are less desirable as they assume that the country-specific effect is a random variable that is uncorrelated with the explanatory variables in the past and current time periods. Because time-invariant regressors are important in many applications, over the years, several methods that allow the inclusion of time- 5 Historically, “People’s Democratic Republic” regimes also existed in Angola (1975-1992), Mozambique (1975-1990), Congo (1969-1992), and Ethiopia (1987-1991). However, the lengths of these regimes have been rather short, and it is not clear whether the regime changes in these countries led to effective policy implementations. Hence, these countries, some of which excluded from the sample through other restriction criteria, are not examined separately. 6 In the Appendix, the estimations cover all the players regardless of ratings. The Lao People’s Democratic Republic appears in these regressions. 432 M. Dilmaghani Journal of Comparative Economics 49 (2021) 425–441 invariant regressors in panel data have been proposed (Mundlak 1978; Chamberlain 1982; Arellano & Bond 1991; Kripfganz & Schwarz 2019). In this paper, in order to include time-invariant variables in the regressions, two different panel-data methods are employed, alongside pooled cross-section regressions. First, the 2-stage, fixed-effects method of Kripfganz and Schwarz (2019) is implemented. This methodology, with static panels like the one estimated here, is identical to a Hausman-Taylor estimator (Hausman & Taylor 1981). According to this method, to obtain consistent coefficients for the time-invariant estimators, first, a fixed-effects model is fitted to the data, excluding the time-invariant variables. In the second stage, the residuals of the first stage estimation are regressed on the time-invariant variables. The strength of this method hinges on the derivation of analytical standard errors for the estimated coefficients, allowing for valid inferences (Kripfganz & Schwarz 2019). The first stage equation can be written as follows: (1) ′ Gender Ratioit = δ0 + xit δ + λ t + μi + εit where the dependent variable, Gender Ratioit, pertains to various measures of gender difference in competitive chess for country i at time t. The time-varying controls are arranged in vector xit. The term λt captures the time dimension, showcasing the evolution of the gender gaps in the 2010s. The time-invariant unit-specific unobservables, assumed independently distributed across i, are denoted by term μi; and the time-varying disturbances are captured by the term, εit. The second stage equation is: ∑ K (2) ′ δ 0 − xit ̂ Gender Ratioit − ̂ δ− ̂ λt = β0 + βK IK + eit i=1 In Equation (2), the term IK stands for country/country-cluster dummies, and eit is the error term. The main text results rely on the simultaneous estimations of Equations (1) and (2). Second, and as a robustness test, a hybrid Mundlak-Chamberlain method is fitted to the data (Mundlak 1978; Chamberlain 1982; Schunck 2013). The Mundlak-Chamberlain method is also known as “correlated random effects,” where the explanatory variables are assumed strictly exogenous conditional on unobserved effects (Mundlak 1978; Chamberlain 1982; Wooldridge 2019). The corre sponding equation can be written as follows: ∑ K (3) ′ ′ Gender Ratioit = β0 + βk IK + δ1 (xit − xi ) + δ2 xi + λt + μi + εit k=1 In Equation (3), the coefficients for the de-meaned variables resemble to the fixed effects “within” estimators, while the coefficients of the panel-unit means over time can be interpreted as “between” estimators. After constructing the auxiliary variables, Equation (3) is estimated by random effects (Schunck 2013; Wooldridge 2019), and the results are shown in the Appendix Table 2, alongside other sensitivity tests. The dependent variable in these equations, Gender Ratioit, is varied to captured three different concepts. The first ratio is ( ) Female Count, +1400 Male Count, +1400 , which allows to assess the cross-country differences in “general interest” in competitive chess. The assumption is it that the FIDE lists for players rated at 1400 Elo points and above reflect the number of men and women who have enough interest in ( ) competitive chess to attain an adequate level of skills and participate in competitions. The second ratio is Female Mean Elo Male Mean Elo. This it dependent variable captures the average level of performance of females relative to males, conditional on playing. Finally, the count ratios of the number of females to the number of males are computed for the Elo rating thresholds of 1500 to 2000, with intervals of ( ) Female Count, Elo≥ R 100 Elo points. The generic form of this set of dependent variables is Male Count, Elo≥ R , where R captures the previously noted it rating thresholds. These estimations are particularly important since, as the descriptive statistics showed, the largest cross-country differences lie in the female representation at the top levels (Bilalić et al. 2009; Blanch 2016).7 The controls, identical in all the estimations and denoted by xit, are selected such that the largest number of countries could be kept in the analyses. Therefore, the availability of the World Bank data has affected the choice of the controls. With this consideration, the controls are the natural logarithm of the GDP per capita in constant dollars, real GDP growth rate, female-to-male labour-force participation rates, and life expectancy at birth by gender. Male and female life expectancies at birth are used as controls since they constitute an important dimension of the UN Human Development Index (McGillivray & White 1993). Unfortunately, the other variables related to the Human Development Index (HDI), such as educational variables, had a large number of missing values, and were not usable. Instead, the Global Gender Gap Index (GGGI) of the World Economic Forum is used as an additional control, to proxy the omitted human capital variables. It can be argued that some of the control variables, such as labour force participation rates, rather than being confounders, are mechanisms through which the institutional contexts affect the gender gaps in competitive chess (Angrist 7 As indicated in the above, the main measures of the gender gap in chess attainment used in this paper are based on the “head count ratios.” Note that comparing the mean ratings of top X (X%) chess players of each gender by country (where X is an arbitrary number, such as 10 or 100) generally masks the gender discrepancies in representation. Additionally, note that the “head count ratios” based on Elo rating thresholds are particularly pertinent in the context of chess, because FIDE uses numeric values of the Elo ratings as indicative of the attainment of a discrete skillset. Accordingly, all the FIDE titles have a minimum Elo rating requirement. For instance, to become a Grandmaster (International Master), the player must be rated at least 2300 (2200) Elo points. 433 M. Dilmaghani Journal of Comparative Economics 49 (2021) 425–441 & Pischke 2008). To allow for an accurate interpretation of the results, the controls are sequentially added to the equations. In all the equations, robust standard errors are clustered by country. 5. Results The estimation methodology underlying the results reported in the main text is the 2-stage fixed effects Hausman & Taylor 1981; Kripfganz & Schwarz 2019), as expressed in Equations (1) and ((2). The first set of regressions, reported in Table 3, uses the ratio of the number of female players to the number of male players as dependent variable. To mitigate the selection issue, the count is restricted to those rated at 1400 Elo points and above. The Elo rating of 1400 is a rather inclusive level, and the results reported in Table 3 can be interpreted as the gender gap in “general interest in competitive chess.” In the subsequent regressions, examining female represen tation at the top levels of performance, the count ratios are computed for those attaining +1500 Elo up to +2000 Elo, with 100-points intervals. Evidently, if no men in a country had attained these thresholds, the ratios could not be defined. There was no country in which a woman was rated above 2000 Elo points (or any of the lower thresholds), but no male player had an equivalent attainment. To keep the samples identical across this set of regressions, the data are generally restricted to the countries with at least one male player with a rating of 2000 Elo points and above. This restriction brings the number of countries to 134.8 Column (1) of Table 3 only includes dummies for China, Cuba, Mongolia and Vietnam, in addition to the 4 country-cluster dummies of (i) Post-Soviet countries, which takes the value of 1 for countries such as Georgia, Ukraine, and the Russian Federation; (ii) Formerly Communist countries, which takes the value of 1 for countries such as Bulgaria and Poland; (iii) Other EU, which takes the value of 1 for the EU countries not included in the previous categories; and (iv) Other OECD, which takes the value of 1 for the OECD countries not included in any of the previous clusters. The reference category includes all other countries as listed in Table 1, and is referred to as the Rest of the World (ROW). Column (2) adds a time trend. Column (3) adds the natural logarithm of the GDP per capita in constant dollars. Column (4) further adds the annual real GDP growth rates. Here, the intention is to see how the differences in economic development affect the extent of the gaps, since more developed countries have generally higher levels of gender equality (Ćorić 2018). Column (5) additionally includes the female-to-male labour-force participation rates. Column (6) further augments the equation with male and female life expectancies at birth. Finally, Column (7) adds the Global Gender Gap Index (GGGI). The variables included in Columns (5) to (7) account for the observable levels of gender equality in human capital and labour market attainment, across countries. As Column (1) of Table 3 shows, the gender ratio is 53.0 (40.4) percentage points higher in Vietnam (Mongolia) than the rest of the world. For China, Cuba, and Post-Soviet countries, the coefficients indicate the presence of 29.5, 10.7, and 7.3 more females per 100 males in chess competitions on behalf of these countries than the rest of the world. In contrast, there are 6.5 fewer female participants in Other EU. The coefficients for Formerly Communist, and Other OECD clusters are not statistically significant at the zero-order. The inclusion of the time trend, done in Column (2), has a negligible effect on the coefficients of interest. The time trend itself turns up with a negative coefficient across all the specifications, indicating that female-to-male chess participation ratio has not been increasing between 2012 and 2018. The coefficients for natural logarithm of GDP per capita in constant dollars, real GDP growth rate, and labour- force participation ratio turn up statistically insignificant, with negligible effects on the coefficients of interest. The inclusion of the life expectancies at birth, done in Column (6), exercises the largest influence on the coefficients of interest. The correlation is positive (negative) for female (male) life expectancy with the outcome. Hence, one can deem them with the expected signs. These variables likely proxy-omitted human capital variables. Finally, the inclusion of the GGGI, itself with a positive but insignificant coefficient, has a small effect on the coefficients of interest. As shown in Column (7), where all the controls are accounted for, the gender ratio is 50.2 (35.3) percentage points higher in Vietnam (Mongolia) than the rest of the world. The coefficients for China, Cuba, and Post-Soviet countries are also statistically sig nificant and positive indicating the presence of 31.6, 9.4, and 6.6 more females per 100 males in chess competitions on behalf of these countries than the rest of the world. In contrast, there are 6.2 and 3.0 fewer female participants in Other EU and Formerly Communist clusters per 100 males than the Row. The coefficient for Other OECD is not statistically significantly different from zero. Table 4 follows the same structure as Table 3, only it replaces the dependent variable with the ratio of the mean Elo ratings by gender. Here too, players with an Elo rating below 1400 are dropped from the individual-level dataset. In Table 4, the sample is smaller than in Table 3, with 121 countries. The reason is that a few countries did not have a single female rated player. While “0 female player” is a valid value for the count ratios, for the mean rating ratios, the absence of female players means that female performance is not observed in the data. More precisely, since the mean rating ratio is used as an indicator of relative chess skill in the population, assuming no chess skill (i.e., a rating of 0 Elo point) for females of these countries may be inaccurate. Hence, these countries are excluded from this set of regressions. Table 1 lists the excluded countries.9 In Column (1), only the variables of interest are included. All the coefficients are statistically significant, positive, and generally close in size. As shown in the Table 1, China has the largest coefficient, closely followed by Vietnam, Mongolia, and Post-Soviet cluster. With the gradual addition of the controls, all the coefficients fall, except that of Mongolia. According to Column (7), the female-to-male 8 The countries with no player rated at 2000 and above, in one or several year(s), are: Bahamas; Brunei Darussalam; Republic of Congo; Cape Verde; Eritrea; Djibouti; Guyana; Laos; Lesotho; Maldives; Nauru; Palau; Sierra Leone; Solomon Islands; Sao Tome and Principe; Swaziland; Tanzania; Timor-Leste. 9 These countries, for one or several year(s), did not have any rated female player: Afghanistan; Bhutan; Burundi; Cambodia; Côte d’Ivoire; Cameroon; Haiti; Saudi Arabia; Kuwait; Mali; Mauritius; Papua New Guinea; Senegal; San Marino; Somalia; Togo. 434 M. Dilmaghani Journal of Comparative Economics 49 (2021) 425–441 Table 3 Female to Male Count Ratio, Elo +1400 (1) (2) (3) (4) (5) (6) (7) China 0.295*** 0.294*** 0.293*** 0.291*** 0.282*** 0.311*** 0.316*** (0.013) (0.013) (0.012) (0.013) (0.018) (0.017) (0.018) Cuba 0.073*** 0.072*** 0.071*** 0.072*** 0.074*** 0.100*** 0.094*** (0.013) (0.013) (0.012) (0.012) (0.012) (0.017) (0.017) Mongolia 0.404*** 0.403*** 0.403*** 0.401*** 0.394*** 0.353*** 0.353*** (0.013) (0.013) (0.012) (0.013) (0.019) (0.025) (0.024) Vietnam 0.530*** 0.529*** 0.531*** 0.529*** 0.519*** 0.495*** 0.502*** (0.013) (0.013) (0.017) (0.018) (0.027) (0.030) (0.030) Post-Soviet 0.107*** 0.107*** 0.106*** 0.106*** 0.100*** 0.064** 0.066** (0.022) (0.022) (0.021) (0.021) (0.025) (0.030) (0.029) Formerly Communist -0.022 -0.023 -0.025 -0.025 -0.030* -0.031* -0.030* (0.016) (0.016) (0.016) (0.016) (0.017) (0.017) (0.017) Other EU -0.065*** -0.066*** -0.071*** -0.071*** -0.081*** -0.061** -0.062** (0.014) (0.014) (0.025) (0.025) (0.023) (0.024) (0.024) Other OECD -0.018 -0.019 -0.023 -0.023 -0.032 -0.010 -0.011 (0.023) (0.023) (0.031) (0.031) (0.031) (0.031) (0.031) Time Trend — -0.004*** -0.004*** -0.004*** -0.004*** -0.002** -0.003** (0.001) (0.001) (0.001) (0.001) (0.001) (0.001) GDP per Capita (ln) — — 0.002 0.002 0.003 0.010 0.010 (0.010) (0.010) (0.009) (0.010) (0.010) GDP Growth Rate — — — 0.001 0.001 0.001 0.001 (0.001) (0.001) (0.001) (0.001) Female/Male Labour- — — — — 0.052 0.017 -0.003 force Participation (0.058) (0.049) (0.049) Life Expectancy — — — — — 0.010*** 0.009** (Female) (0.004) (0.004) Life Expectancy — — — — — -0.012*** -0.012*** (Male) (0.004) (0.004) GGGI — — — — — — 0.108 (0.168) Observations 811 811 811 811 811 811 811 Number of Countries 134 134 134 134 134 134 134 Note: The sample is restricted to the countries in which at least one player is rated at +2000 Elo points, so that the gender ratios could be defined. Robust standard errors, shown in parentheses, are clustered by country; * significant at 10% level; ** significant at 5% level; *** significant at 1% level. rating ratio is 5.8 percentage points higher for both China and Mongolia than the ROW, followed by Vietnam and Post-Soviet countries at 5.7 and 4.1 percentage-points advantages, respectively. These coefficients are not statistically significantly different from each other. The coefficient for the Formerly Communist cluster is also statistically significant and positive, but smaller, at a 2-percentage points advantage. However, the coefficients for Cuba, Other EU, and Other OECD are not statistically significant in Column (7). Regarding the controls, unlike the “general participation ratio” regressions of Table 3, there is a small positive time trend. Therefore, it appears that while the relative number of female chess players has not been rising, the mean relative attainment of women has slightly increased between 2012 and 2018. No other control is statistically significant in the full specification of Column (7). Table 5, exposing 7 regressions, gradually increases the thresholds of chess skills, from 1400 to 2000. The full set of controls are employed at once in all the regressions. The regression of Column (1) is identical to the regression reported in Column (7) of Table 3. It is reproduced here to ease the comparisons, as the skill thresholds ascend. To the benefit of a clearer discussion, the coefficients are reviewed by country/country cluster, rather than by column. Regarding China, the participation-level coefficient of 31.6 more females per 100 males (Elo +1400, Column 1) increases to just above 34 more female chess players rated at +2000 Elo in Column (7) than in the ROW. Hence, there is no evidence that female chess players in China are induced to participate in the competitions without suitable skills. In Cuba, at the general participation level (Elo +1400), there are 9.4 more females per 100 males than the ROW. The coefficient for Cuba increases up to 11.5 more females per 100 males at the level +1800 Elo points. But, in the subsequent columns, the ratio falls. In Column (7), showing the female-to-male ratio at +2000 level, there are 7.2 more women per 100 men in Cuba than in the ROW. At the general participation level (Elo +1400), Mongolia starts with 35.3 more women per 100 men than the Row. For Mongolia, the gender ratio increases in the same pattern as Cuba, up to the rating of +1800 Elo points. For those rated at 1800 Elo points and above, there are 42.3 more women in Mongolia than in the ROW. But, the female-to-male ratio falls as the attainment level becomes more exclusive. In the +2000 Elo points level, there are just above 28 more women in Mongolia than in the ROW per 100 men. Vietnam seems to be the most gender equal country in competitive chess. With 50.2 more women for every 100 men at the +1400 level, the gap follows a non-linear pattern, similar to Cuba and Mongolia. At the +1600 level, there are just below 57 more females per 100 males in Vietnam than in the rest of the world. In the most exclusive level examined here (+2000 Elo points), Vietnam has 45 more women per 100 men than the ROW. 435 M. Dilmaghani Journal of Comparative Economics 49 (2021) 425–441 Table 4 Female to Male Mean Elo Rating Ratio (1) (2) (3) (4) (5) (6) (7) China 0.064*** 0.065*** 0.056*** 0.059*** 0.065*** 0.055*** 0.058*** (0.007) (0.007) (0.007) (0.008) (0.009) (0.012) (0.014) Cuba 0.043*** 0.044*** 0.036*** 0.035*** 0.034*** 0.019* 0.015 (0.007) (0.007) (0.007) (0.006) (0.007) (0.010) (0.009) Mongolia 0.054*** 0.055*** 0.056*** 0.057*** 0.064*** 0.057*** 0.058*** (0.007) (0.007) (0.007) (0.008) (0.009) (0.009) (0.009) Vietnam 0.059*** 0.060*** 0.076*** 0.077*** 0.087*** 0.052*** 0.057*** (0.007) (0.007) (0.010) (0.011) (0.013) (0.018) (0.020) Post-Soviet 0.053*** 0.053*** 0.048*** 0.048*** 0.053*** 0.039*** 0.041*** (0.011) (0.011) (0.010) (0.010) (0.011) (0.012) (0.012) Formerly Communist 0.044*** 0.045*** 0.027*** 0.027*** 0.031*** 0.019** 0.020** (0.010) (0.010) (0.009) (0.009) (0.009) (0.010) (0.010) Other EU 0.045*** 0.046*** 0.003 0.003 0.010 -0.001 -0.002 (0.010) (0.010) (0.015) (0.015) (0.015) (0.015) (0.015) Other OECD 0.039*** 0.039*** -0.000 -0.000 0.004 -0.004 -0.004 (0.010) (0.010) (0.015) (0.015) (0.015) (0.015) (0.015) Time Trend — 0.004*** 0.004*** 0.004*** 0.003*** 0.003*** 0.003*** (0.001) (0.001) (0.001) (0.001) (0.001) (0.001) GDP per Capita (ln) — — 0.018*** 0.018*** 0.018*** 0.009 0.009 (0.006) (0.006) (0.006) (0.007) (0.007) GDP Growth Rate — — — -0.001 -0.001 -0.000 -0.001 (0.001) (0.001) (0.001) (0.001) Female/Male Labour- — — — — -0.041 -0.020 -0.033 force Participation (0.030) (0.032) (0.041) Life Expectancy — — — — — 0.004* 0.003 (Female) (0.002) (0.002) Life Expectancy — — — — — -0.001 -0.001 (Male) (0.002) (0.002) GGGI — — — — — — 0.072 (0.088) Observations 744 744 744 744 744 744 744 Number of Countries 121 121 121 121 121 121 121 Note: The sample is restricted to the countries in which at least one female player is rated at +1400 Elo points, so that the gender ratios are observed in the data. Robust standard errors, shown in parentheses, are clustered by country; * significant at 10% level; ** significant at 5% level; *** significant at 1% level. In the Post-Soviet countries, the entry-level gender ratio (Elo +1400) indicates 6.6 more women per 100 men than the ROW. For the Post-Soviet countries, the ratio monotonically increases as the skill threshold is tightened. For those rated at 2000 Elo points and above, there are 13.5 more women in these countries than in the ROW. For the Formerly Communist countries of Europe, the coef ficient is negative at the general participation level (Elo +1400), indicating 3 fewer women per 100 men than the ROW. But, similarly to the Post-Soviet countries, the coefficient increases as the skill thresholds are tightened. At +1700 level, the coefficient becomes statistically insignificant. At +2000 level, there are 2.7 more women per 100 men in the Formerly Communist countries than in the ROW. All in all, these results indicate that not only there are relatively more women participating in chess competitions in these countries, but also, they are not generally adversely selected. In contrast, at +1400 level, there are about 6 fewer women per 100 men in Other EU than the ROW. For Other EU cluster, at +2000 level, the negative coefficient shrinks to just above 2 fewer women per 100 men than the ROW. Finally, for Other OECD, the coefficient at the +1400 level, is not statistically significant, and remains as such for all the levels. Regarding the controls, the coefficient for the time trend is statistically significant and negative (except for the highest threshold, where it is insignificant). Therefore, it seems that no notable improvement in female representation in chess occurred between 2012 and 2018. Few other variables have statistically significant coefficients. Notably, male and female life expectancies are statistically significant for skill levels below +1900 with expected signs. The coefficients for the GGGI is positive for all skill thresholds, but only statistically significant at +1800 and +1900 levels. Hence, higher values of the GGGI, all else held constant, weakly predicts a greater female presence at the top levels of attainment in chess. Similar findings have been previously reported regarding female attainment in mathematics (Guiso et al. 2008; Kane & Mertz 2012). Various robustness tests have been conducted. First, the dependent variable is extended to the ratio of those rated +2100, the ratio of FIDE-titled players (FIDE Master, International Master, and Grandmaster), as well as the ratio of the medians. Second, the esti mations were made using the entire sample, including also those rated below 1400 Elo points. These regressions are shown in Appendix Table 1. As the table shows, when the count ratio is based on an Elo rating of +2100, the previously noted pattern of cross-country gaps remains. At +2100, Vietnam, China, and Mongolia rank the highest. The regression assessing the extent of the gender gaps in the number of titled players, shown in Column (2) of Appendix Table 1, indicates that Mongolia ranks the highest, followed by China. As shown in Column (3) of this table, the results on the median ratio is rather similar to those found for the mean ratio. Finally, using the 436 M. Dilmaghani Journal of Comparative Economics 49 (2021) 425–441 Table 5 Female to Male Count Ratios Elo Ratings → +1400 +1500 +1600 +1700 +1800 +1900 +2000 (1) (2) (3) (4) (5) (6) (7) China 0.316*** 0.318*** 0.323*** 0.329*** 0.341*** 0.333*** 0.342*** (0.018) (0.016) (0.018) (0.011) (0.009) (0.008) (0.009) Cuba 0.094*** 0.098*** 0.091*** 0.109*** 0.115*** 0.093*** 0.072*** (0.017) (0.015) (0.017) (0.012) (0.010) (0.009) (0.010) Mongolia 0.353*** 0.370*** 0.395*** 0.403*** 0.423*** 0.390*** 0.282*** (0.024) (0.017) (0.019) (0.012) (0.010) (0.009) (0.009) Vietnam 0.502*** 0.525*** 0.568*** 0.530*** 0.528*** 0.454*** 0.450*** (0.030) (0.025) (0.030) (0.019) (0.015) (0.013) (0.012) Post-Soviet 0.066** 0.084*** 0.087*** 0.115*** 0.131*** 0.132*** 0.135*** (0.029) (0.026) (0.030) (0.025) (0.026) (0.028) (0.029) Formerly Communist -0.030* -0.024 -0.030* 0.001 0.019* 0.021** 0.027** (0.017) (0.016) (0.018) (0.012) (0.010) (0.011) (0.011) Other EU -0.062** -0.062*** -0.065*** -0.042*** -0.024** -0.032*** -0.022* (0.024) (0.022) (0.024) (0.014) (0.011) (0.011) (0.012) Other OECD -0.011 -0.015 -0.011 -0.016 -0.003 -0.010 -0.004 (0.031) (0.027) (0.033) (0.014) (0.010) (0.010) (0.011) Time Trend -0.003** -0.004*** -0.002** -0.003*** -0.003*** -0.002*** -0.000 (0.001) (0.001) (0.001) (0.001) (0.001) (0.000) (0.000) GDP per Capita (ln) 0.001 0.001 0.001 0.001 0.001 0.001 0.001 (0.001) (0.001) (0.001) (0.001) (0.001) (0.001) (0.001) GDP Growth Rate 0.010 0.010 0.009 0.010* 0.006 0.007 0.009** (0.010) (0.009) (0.009) (0.006) (0.005) (0.004) (0.005) Female/Male -0.003 -0.016 -0.017 -0.051** -0.034 -0.016 -0.011 Labour-force Partic. (0.049) (0.037) (0.042) (0.024) (0.021) (0.021) (0.021) Life Expectancy 0.009** 0.008** 0.010** 0.006** 0.005** 0.003 0.002 (Female) (0.004) (0.003) (0.004) (0.003) (0.002) (0.002) (0.002) Life Expectancy -0.012*** -0.010*** -0.011*** -0.006** -0.005** -0.003 -0.003 (Male) (0.004) (0.004) (0.004) (0.003) (0.002) (0.002) (0.002) GGGI 0.108 0.048 0.008 0.116 0.125* 0.125* 0.107 (0.168) (0.124) (0.153) (0.071) (0.069) (0.066) (0.075) Observations 811 811 811 811 811 811 811 Number of Countries 134 134 134 134 134 134 134 Note: The sample is restricted to the countries in which at least one player is rated at +2000 Elo points, so that the gender ratios could be defined. Robust standard errors, shown in parentheses, are clustered by country; * significant at 10% level; ** significant at 5% level; *** significant at 1% level. entire sample and not dropping those rated below 1400 Elo points results in no change in country/cluster rankings. A result of note in this set of regressions is that, when the entire sample is used, the Lao People’s Democratic Republic has the largest positive coefficient in mean and median ratios. Hence, it appears that in Laos both men and women perform poorly, but the attainment gap in Laos, measured through the mean and median ratios, is smaller than other countries. Additionally, all the equations were re-estimated using the within-between method of Mundlak-Chamberlain (Mundlak 1978; Chamberlain 1982; Schunck 2013; Wooldridge 2019), shown in Equation (3). These results are reported in Panel A of Appendix Table 2. As another set of sensitivity tests, the pooled cross-section estimations are shown in Panel B of Appendix Table 2. The results are almost identical. Finally, the main equations were augmented by a gender-specific, third-degree polynomial in age, and the results are shown in Panel C of Appendix Table 2. The age variables pertain to the means at the general participation level (+1400 Elo points). The choice of using a third-degree polynomial in age is informed by the literature on the effects of aging on chess performance (Roring & Charness 2007; Bertoni et al. 2015). No major difference in country rankings emerges from these estimations. Moreover, all the equations were estimated for one year at a time, using OLS. Appendix Table 3 shows the by-year results for the three outcomes of general participation (count ratio, Elo +1400), mean rating ratio, and representation at the top (count ratio, Elo +2000). While no conclusion is affected, there are some differences in the sizes of the coefficients as the sample of countries in the ROW varied to some extent in FIDE’s yearly lists. Finally, it was insured that the country-cluster coefficients are not driven by a single country. This was done by dropping one country from a cluster at a time, and re-estimating the equations. These regressions, not reported in the Appendix, are available upon request. Some limitations of this study must be noted. First, unfortunately, due to data limitations, players participating under the German flag could not be subdivided based on their regions of upbringing. It would have been more accurate to assign those who grew up in the regions belonging to the former East Germany to the Formerly Communist cluster. But, it was not possible. However, dropping Germany from the sample does not affect any conclusion. Second and relatedly, the players are assigned to the countries under whose flag they played at the time of data collection. But, possibly, there are world-class players who have obtained the citizenship of another country, owing to their exceptional talent in chess. The same applies to those who emigrated from their country of upbringing through other means, hence have been generally exposed to another culture earlier in life. While the migration flows are usually from 437 M. Dilmaghani Journal of Comparative Economics 49 (2021) 425–441 developing countries towards developed countries, without information about the gender composition of the immigrant players, it is not possible to assess the direction of this potential bias. 6. Discussion and Conclusion The present study is the first worldwide quantitative investigation of the extent of the gender gap in competitive chess. Using several measures of gender gap, three interrelated questions have been investigated. First, the differences across countries in the gender ratio among chess competition participants have been examined. Second, the cross-country differences in mean attainment ratio have been estimated. Finally, and most importantly, the paper examined the female representation gaps at the top levels of performance across countries. Globally, women were found to show a significantly lower interest in competitive chess than men. However, controlling for the main indicators of economic development and observable measures of gender equality, countries with a legacy of command economy were found to have much higher relative female participation rates than the rest of the world. In comparison, the mean attainment figures, almost everywhere in favour of men, varied to a lesser extent across countries. But here too, more gender equal outcomes are found for countries with a legacy of central planning. Finally, in the female representation at the top levels of attainment, substantial differences across countries remained unaccounted for by the indicators of economic development and observed levels of gender equality. Again, countries with a history of state-socialism were found to have much higher gender ratios. Western liberal democracies performed largely below these countries and at times, even below the rest of the world. The findings contribute to the scholarship regarding gender differences in competitiveness, female representation at the top levels of male- dominated fields, and the influence of sociopolitical institutions on gender-role attitudes and gender gaps in outcomes. Below, these contributions are further linked to the past scholarship. Some strands of the literature suggest that gender differences in competitiveness are biologically driven. Evolutionary biology argues that the marginal benefit from competing for reproductive purposes is higher for males than for females in most species, including humans. This discrepancy, combined with selection pressures, has led to the greater presence of competitive traits in males (Cassar et al. 2016). But, the more recent scholarship tends to somewhat reject this assertion (Henrich et al. 2012; Cassar et al. 2016). For instance, Cassar et al. (2016) provide evidence that child-benefitting rewards can increase competitive tendencies in mothers more than in fathers. The evidence for the alternative explanation, that the gender differences in competitiveness are induced by social ization, has been growing in the past couple of decades. Gneezy et al. (2009) showed that in a matrilineal society in India, where men have considerable responsibilities in childcare, the gender gap in competitiveness is reversed. Consistently, Zhang (2019), comparing the gender gaps in competitiveness among the Chinese ethnic majority group of Han and the ethnic minority groups of Yi and Musou, finds no statistically significant gender gap in competitive inclination among the Han Chinese subjects. Given that the Chinese ethnic minorities were exempt from the application of communist policies since the inception of the regime, Zhang (2019) suggests that communist policies promoting gender equality have played a role in reversing women’s attitudes towards competition. Notwithstanding the gender gap in competitiveness, women are much scarcer in certain fields than in others. Mathematics and mathematics-intensive fields are prime examples. The educational attainment of women, as measured by years of schooling, has now surpassed that of men in many countries (Goldin 2006, 2014; Kane & Mertz 2012; Fortin et al. 2015), but women still shy away from mathematics-intensive fields (Lindberg et al. 2010; Lippmann & Senik 2018). Here too, gender differences are attributed to the two main sets of factors: biology and socialization (Guiso et al. 2008). In this strand of literature, one extensively examined biology-based hypothesis is the “male greater variability” (Shields 1982). This hypothesis implies relatively smaller gender differences in central tendency measures, with greater gender differences in extreme values (Hyde & Mertz 2009; Kane & Mertz 2012). But, the “male greater variability” hypothesis does not accommodate the staggering cross-country differences found here. Kane and Mertz (2012), examining mathematics performance, also find that the male-to-female performance variance ratio significantly differs across coun tries, which is inconsistent with the greater male variability hypothesis. The socialization-based explanation has many facets, from culture and religion, to labour-market conditions. For instance, if women foresee a lower labour market return to education, due to fertility breaks, burden of household chores, or discrimination, they may self-select into less demanding fields (Hunt 2016; Lippmann & Senik 2018). Such rationally motivated channels add to the culturally imbedded barriers, such as gender-role attitudes. A growing number of studies show that individual beliefs about gender roles are endogenous to political regimes, and that the effects are persistent (Lippmann et al. 2020). Notably, Bauernschuster and Rainer (2012) show that East Germans remain significantly more likely to hold egalitarian sex-role attitudes than West Germans, while Lippmann and Senik (2018) find a smaller gender gap in mathematics among pupils in regions previously part of East-Germany. The present paper provides strong evidence for the influence of contextual factors on female attainment in chess. The fact that Post-Soviet countries and Mongolia largely stood out alongside China, Vietnam, and Cuba, support the possibility of persistent effects for socio-political institutions. Particularly, it may be the case that by making gender-role attitudes less traditional, the socialist in stitutions have steered women towards investing in activities that have been traditionally male dominated. Across disciplines, it has been documented that women in communist countries had a significant gender equality advantage over women in Western capitalist countries (Dijkstra & Hanmer 2000; Pollert 2003). In the formerly communist countries, the male breadwinner system of Western Europe has been strongly challenged or overturned (Brained 2000), as various public policies tried to minimize the conflicts between occupational and familial obligations, to ensure a high female labour-force participation (Campa & Serafinelli 2019). By the 1980s, half of the labour force in Eastern Europe consisted of women, against 32% in Western Europe (Molyneux 1990). With compressed wage scales, Eastern European women fared at least as well as their counterparts in Western Europe in terms of gender wage gap (Brainerd 2000). While the communist model explicitly promoted female labour-force partici pation as the unique way to emancipation (Molyneux 1990), Western countries centered their gender equality efforts on the 438 M. Dilmaghani Journal of Comparative Economics 49 (2021) 425–441 establishment of a welfare state (Esping-Andersen 2013) and policies such as affirmative action (Bracha et al. 2019). For instance, Ireland and Britain remained strong male breadwinner states, as the social policies failed to override the assumption of women as dependent housewives (Lewis 1992; Pascall & Manning 2000). Even in countries such as Sweden, which managed to weaken the male breadwinner regime, a gender job segregation developed, with women highly present in the service sector (Lewis 1992; Anker 1998). In contrast, in the command economies, the imperative of industrialization steered women into somewhat gender-atypical occupations (Zhang 19). At the same time, the positive strides in female educational attainment feminized several graduate professions, such as medicine, law, and accounting (Zajda 1980). Consequently, “Eastern” women entered qualified professions in larger numbers than in the West (Pollert 2003). China, Vietnam, Cuba, and the former People’s Republic of Mongolia were subjected to similar policies (Liu 2004). As reported in Zhang (2019), upon the inception of the People’s Republic in China, propaganda campaigns emphasized that collective labour was the only way for women’s emancipation, showcasing females working in traditionally male jobs such as truck driving. Consistently, Pham and Reilly (2007) found no evidence of a wage-related glass ceiling for Vietnamese women. With compressed wage scales, the gender wage gap in Cuba is reported to align with those prevailing in the developed countries (Antecol 2000). Regarding educational attainment, greater overall achievement and a smaller gender gap is reported for Cuba than some of the comparable developing countries of Latin America (Gasperini, 2000; McEwan & Marshall 20, 2004). Likewise, according to Pastore (2010), Mongolian women have a higher educational attainment, greater educational aspirations, and better employment prospects than Mongolian men. While the above review on the effects of gender-equal policies of centrally planned countries is compatible with the results reported here, there may also be something specific about competitive chess. A less general explanation for the results can be offered based on the history of promotion of excellence in sports in the command economies (Zajda 1980). The former Soviet Union and China’s emphasis on excellence in sports is well documented (Riordan 1980; Keys 2003; Moul & Nye 2009). It entailed centralized efforts to detect and nurture talents at a young age, to the benefit of the nation (Krüger 1995; Gasperini 2000). Such centralized efforts of communist regimes may have changed the generally negative public perception of females’ intensive investment in sports, inclusive of chess (Messner et al. 1993). If this conjecture is true, then, the effect seems to be persistent. All in all, in light of the previously discussed scholarship (Bauernschuster & Rainer 2012; Lippmann & Senik 2018; Campa & Serafinelli 2019; Zhang 2019; Lippmann et al. 2020), the possibility of a lasting effect for socialist institutions on both female competitiveness and the normalization of female investment towards excellence in the male domains seems plausible. Future comparative scholarship, examining other facets of gender inequality across sociopolitical regimes, may further enrich this strand of literature. Acknowledgements The author is grateful to two anonymous referees, Professor Sebastian Kripfganz, and the participants of conferences held by the Canadian Economic Association and the Society for Advancement of Behavioral Economics for helpful comments and suggestions.