Week 3 (ii) Lecture PDF
Document Details
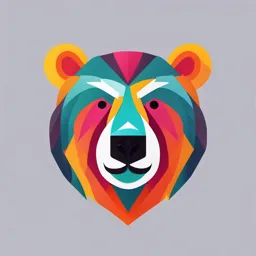
Uploaded by CleverNovaculite
Huddersfield Business School
Dr Artur Semeyutin
Tags
Related
- Module 2 Régression Linéaire Multiple PDF Printemps 2024
- Homework 3: Multiple Linear Regression
- Chapter 4: Multiple Linear Regression (PDF)
- Econometrics I Past Paper PDF
- Lecture 6 - Multiple Linear Regression PDF
- Applied Econometrics (5AEC) Specimen Test PDF - The London Institute of Banking & Finance
Summary
This lecture covers multiple linear regression, including functional forms and categorical variables. It discusses the generalisation of simple models, the constant term, and different ways to express multiple regression models. Key concepts like the OLS estimator, standard errors, and different ways of expressing the model are also explored.
Full Transcript
Lecture 2: MLR, Functional Forms and Categorical Variables Essential reading: Chapters 4 and 10 in Brooks. Dr Artur SemeyutinBIE0014: Econometrics Huddersfield Business School w/c 30/01/2023Dr Artur Semeyutin (BIE0014) MLR Business School1 / 33 Generalising the Simple Model to Multiple Linear Regre...
Lecture 2: MLR, Functional Forms and Categorical Variables Essential reading: Chapters 4 and 10 in Brooks. Dr Artur SemeyutinBIE0014: Econometrics Huddersfield Business School w/c 30/01/2023Dr Artur Semeyutin (BIE0014) MLR Business School1 / 33 Generalising the Simple Model to Multiple Linear Regression Before, we have used the model yt = α+ βx t + u t t = 1,2,..., TBut what if our dependent ( y) variable depends on more than one independent variable? For example the number of cars sold might plausibly depend on 1 the price of cars 2 the price of public transport 3 the price of petrol 4 the extent of the public’s concern about global warming Similarly, stock returns might depend on several factors. Having just one independent variable is no good in this case - we want to have more than one xvariable. It is very easy to generalise the simple model to one with k− 1 regressors (independent variables). Dr Artur Semeyutin (BIE0014) MLR Business School2 / 33 Multiple Regression and the Constant Term Now we write yt = β 1 + β 2x 2t + β 3x 3t + ... +β kx kt + u t, t=1,2,..., TWhere is x 1? It is the constant term. In fact the constant term is usually represented by a column of ones of length T: x 1 = 1 1 · · · 1 β 1 is the coefficient attached to the constant term (which we called α before). Dr Artur Semeyutin (BIE0014) MLR Business School3 / 33 Different Ways of Expressing the Multiple Linear Regression Model We could write out a separate equation for every value of t: y 1 = β 1 + β 2x 21 + β 3x 31 + · · · +β kx k1 + u 1 y 2 = β 1 + β 2x 22 + β 3x 32 + · · · +β kx k2 + u 2 . . . . . . . . . . . . y T = β 1 + β 2x 2T + β 3x 3T + · · · +β kx kT + u T We can write this in matrix form y= Xβ + u where: yis T ×1 X isT ×k β is k× 1 u is T ×1 Dr Artur Semeyutin (BIE0014) MLR Business School4 / 33 Inside the Matrices of the Multiple Linear Regression Model e.g. if kis 2, we have 2 regressors, one of which is a column of ones: y 1 y 2 . . . y T = 1 x 21 1 x 22 . . . . . . 1 x 2T β1 β 2 + u 1 u 2 . . . u T T ×1 T×2 2 ×1 T×1 Notice that the matrices written in this way are conformable. Dr Artur Semeyutin (BIE0014) MLR Business School5 / 33 How Do We Calculate the Parameters (the β) in this Generalised Case? Previously, we took the residual sum of squares, and minimised it w.r.t. αand β. In the matrix notation, we have ˆ u = ˆ u 1 ˆ u 2 . . . ˆ u T The RSS would be given by ˆ u ′ ˆ u = [ ˆ u 1 ˆ u 2 · · · ˆ u T ] ˆ u 1 ˆ u 2 . . . ˆ u T = ˆ u2 1 + ˆ u2 2 + · · · + ˆu2 T = X ˆ u 2 t Dr Artur Semeyutin (BIE0014) MLR Business School6 / 33 The OLS Estimator for the Multiple Regression Model In order to obtain the parameter estimates, β 1, β 2,..., β k, we would minimise the RSS with respect to all the βs. It can be shown that ˆ β = ˆ β 1 ˆ β 2 . . . ˆ β k = ( X′ X )− 1 X ′ y Dr Artur Semeyutin (BIE0014) MLR Business School7 / 33 Calculating the Standard Errors for the Multiple Regression Model Check the dimensions: ˆ β is k× 1 as required. But how do we calculate the standard errors of the coefficient estimates? Previously, to estimate the variance of the errors, σ2 , we used s 2 = P ˆ u 2 T −2. Now using the matrix notation, we use s2 = ˆ u ′ ˆ u T −k where k= number of regressors. It can be proved that the OLS estimator of the variance of ˆ β is given by the diagonal elements of s 2 (X ′ X )− 1 , so that the variance of ˆ β 1 is the first element, the variance of is the second element, and ... , and the variance of ˆ β k is the kth diagonal element. Dr Artur Semeyutin (BIE0014) MLR Business School8 / 33 Calculating Parameter and Standard Error Estimates for Multiple Regression Models: An Example Example: The following model with k=3 is estimated over 15 observations: y= β 1 + β 2x 2 + β 3x 3 + u and the following data have been calculated from the original X’s. ( X ′ X )− 1 = 2 .0 3 .5 −1.0 3 .5 1 .0 6 .5 − 1.0 6 .5 4 .3 , (X ′ y ) = − 3.0 2 .2 0 .6 , ˆ u ′ ˆ u = 10 .96 Calculate the coefficient estimates and their standard errors. Dr Artur Semeyutin (BIE0014) MLR Business School9 / 33 Calculating Parameter and Standard Error Estimates for Multiple Regression Models: An Example (Cont’d)To calculate the coefficients, just multiply the matrix by the vector to obtain ( X′ X )− 1 X ′ y . To calculate the standard errors, we need an estimate of σ2 . s 2 = RSS T −k= 10 .96 15 −3= 0 .91 The variance-covariance matrix of ˆ β is given by s 2 (X ′ X )− 1 = 0 .91( X′ X )− 1 = 1 .82 3 .19 −0.91 3 .19 0 .91 5 .92 − 0.91 5 .92 3 .91 Dr Artur Semeyutin (BIE0014) MLR Business School10 / 33 Calculating Parameter and Standard Error Estimates for Multiple Regression Models: An Example (Cont’d)The variances are on the leading diagonal: var(ˆ β 1) = 1 .82 SE(ˆ β 1) = 1 .35 var (ˆ β 2) = 0 .91 ⇔SE(ˆ β 2) = 0 .95 var (ˆ β 3) = 3 .91 SE(ˆ β 3) = 1 .98 We write: ˆ y = 1 .10 −4.40 x 2 + 19 .88 x 3 (1 .35) (0 .96) (1 .98) Dr Artur Semeyutin (BIE0014) MLR Business School11 / 33 Model Form Let’s return to a simple linear regression of the form yi = β 0 + β 1 · x i + ϵ i and look into two common cases how we can slightly change this form. Dr Artur Semeyutin (BIE0014) MLR Business School12 / 33 Log-Level Model By taking the natural log of y i (making a log transformation of the dependent variable) and regressing it on x i, we can obtain a log-level regression model given by log(y i) = β 0 + β 1 · x i + ϵ i. This small dependent variable transformation leads to a different coefficient interpretation with β 1 now portraying the percentage change (%∆) in yfrom an extra unit of x. For example, below regression output log( [ wage i) = 0 .584 + 0 .083 ·educ i indicates that an extra unit of education (e.g. year) leads to an 8% increase in wages. Dr Artur Semeyutin (BIE0014) MLR Business School13 / 33 Log-Log Model If we make log transformations of x i and y i, our slope parameter (beta) interpretation shall be similar to the elasticity %∆ x i %∆ y i interpretation. To exemplify, for the model below log(y i) = β 0 + β 1 · log( x i) + ϵ i and its illustrative regression output log(\ salary i) = 4 .822 + 0 .2577 ·log( sales i) log-log transformation leads to the following interpretation: a 1% increase in sales leads to a 0.2577% increase in salaries. Dr Artur Semeyutin (BIE0014) MLR Business School14 / 33 Importance of Functional Form It is important to pay attention to the functional form being used (we use) in a regression as it impacts interpretation of our results. * Why these forms? In some cases, we may be interested in a relative effect of one variable on another. In the other cases, we may be interested in changing the functional form to correct some of the potential estimation problems and improve our estimation output. Let’s look into an example ... Dr Artur Semeyutin (BIE0014) MLR Business School15 / 33 Importance of functional form example (Cont’d) For a data set on Test Scores from the accompanyinge-book, we can obtain two below regression outputs: \ TestScore i= 625 .38 + 1 .878 ·Income i and \ TestScore i= 557 .8 + 36 .42 ·log( Income i) . * Note that in this example we take a log transformation of our independent variable and do not log-transform our dependent variable! Dr Artur Semeyutin (BIE0014) MLR Business School16 / 33 Importance of Functional Form Example (Cont’d)Dr Artur Semeyutin (BIE0014) MLR Business School17 / 33* * * * * * * ** * * * * * * * * ** * * * * * **** ** * * * *** * * * * * *** * * * * ** * * ** * ** * * * * * * * * * * * * * * * ** * ** *** * * ** ** * ** * ** * * * * * ** * * ** * * ** * * * * * * ** * **** * * * * * * * ** * * ** * * * * * * ** * * * ** * * * * ** * * * ** ** * * * **** * * * * * * * * * ** * * * * * * * ** ** * * ** * * * * * * * * * * * * * * * * * * * *** * * * * * * * *** * * * ** * ** * * * * * * ** * * ** * ** * * *** * ** * * * * * * * * * * * * * * ** * * * * * ** *** * *** * * ** * * * * * * * * * * * * ** * * * * * ** * * * * * * * ** *** * * * * * * * ** * * * * * * * * ** * * * * * ** ** * * * * * * ** * * * * * * * * * * * * ** ** * ** * * *** * * * * * * * * * * * * * ** * * * * * * * * * * * * * * * * * * * * * * * 10 20 30 40 50 620 640 660 680 700 Test Scores, Income and log(Income) Income TestScore Importance of Functional Form Example (Cont’d)From the figure in the previous slide, what line fits (represents) Test Scores and Income relationship better? What functional form blue line represents? What functional form red line represents? How would you interpret regression output when one of your independent variables has been log transformed while dependent has not? Dr Artur Semeyutin (BIE0014) MLR Business School18 / 33 Categorical Variables Another focus of this lecture is categorical variables. Categorical variables typically represent something which is not measured numerically. Categorical variables might be referred to as dummy variables, binary variables or as qualitative information. All these terms have the same meaning and we are going to use them interchangeably throughout the module. Categorical variables are used a lotin economics as well as other scientific disciplines. For example, in the second semester we will look into the panel data modelling and dummy variables play an important (special) role in this setting. Dr Artur Semeyutin (BIE0014) MLR Business School19 / 33 Categorical Variables General Examples Dummy variables can be used to explore impact of (differences due to) Gender (e.g. when we are looking at differences in wages between female and male workers) Whether someone is married or not Whether someone smokes or not Whether someone is an economist/engineer/etc. or not Dr Artur Semeyutin (BIE0014) MLR Business School20 / 33 Categorical Variables an Illustrative Example Dummy variables take values of 0 and 1. For example, in our hypothetical data set below wage female married 3.1 1 0 3.24 1 1 3 0 0 6 0 1 5.3 0 1 8.75 0 1 11.25 0 0 5 1 0 3.6 1 0 female and married are categorical variables. When married variable takes the value of 1 it indicates that person is married and 0 otherwise. Similar interpretation applies to the female variable. Dr Artur Semeyutin (BIE0014) MLR Business School21 / 33 Categorical Variables in a Model In the below model wagei= β 0 + β 1 · educ i+ β 2 · female i+ ϵ i β 2 is E(wage |educ ,female )− E(wage |educ ,male ) and represents the difference in wages between males and females. * Note that the base group in a regression is for which we set our dummy variable equal to 0 (males in our case). We could turn this around if set female i= 1 −male i. Dr Artur Semeyutin (BIE0014) MLR Business School22 / 33 Categorical Variables and Functional Form In the below case log(wage i) = β 0 + β 1 · educ i+ β 2 · female i+ ϵ i β 2 represents the percentage difference in wages between our group of females and our control group (males). This can be quite helpful for policy analysis. Dr Artur Semeyutin (BIE0014) MLR Business School23 / 33 More Categorical Variables We can add more dummy variables to our model from the previous slide. For example, log( wage i) = β 0 + β 1educ i+ β 2female i+ β 3married i+ β 4economist i+ ϵ i. Interpretation of the base group becomes less clear when add more categorical variables. To be specific, our base group is now a single male who is not an economist and each dummy looks at the effects of each separate group. Therefore, percentage difference in wage of a married female economist and a single male non-economist is given by β 2 + β 3 + β 4. Dr Artur Semeyutin (BIE0014) MLR Business School24 / 33 Dummy Variable Trap From the G-M assumptions, we cannot have a perfectly linear relationship between variables in our models. If you include two dummy variables for one category in your model (without dropping the intercept), you will fall into the dummy variable trap as these two dummies will form a perfect linear relationship. For example, this may happen if you create a separate dummy variable for males and females to represent gender category. In simple terms, with very high degree of certainty, you can predict that a person is female, if you know that they are not male. Luckily, modern statistical packages will either return an error (warning) message or will drop one of the redundant variables for you. Dr Artur Semeyutin (BIE0014) MLR Business School25 / 33 Categorical and Ordinal Variables An ordinal variable is a categorical variable with more than one category (not binary). For example, if we want to look at whether there is a GPA difference between students from different disciplines at the university, we can set deg = 1 for Accountancy, deg= 2 for Finance, deg= 3 for Economics etc. and run the following regression: GPAi= β 0 + β 1 · attend i+ β 2 · deg i+ ϵ i. However, interpretation of our β 2 parameter becomes problematic (e.g. one unit increase in degvariable leads to higher (lower) GPA does not tell us much). Dr Artur Semeyutin (BIE0014) MLR Business School26 / 33 Categorical and Ordinal Variables (Cont’d)If create a range of dummies for each degree (whether someone has a degree in Accountancy, Finance, Economics, etc.), we can obtain results that are easier to interpret. For example, GPAi= β 0 + β 1 · attend i+ β 2 · account i+ β 3 · fin i+ ϵ i. * Why I did not include a dummy for economists? * Can you guess the base category in the above regression? Dr Artur Semeyutin (BIE0014) MLR Business School27 / 33 Interactions We sometimes (or quite often) let our dummy variables interact with other variables in our models by multiplying them together. If we go back to the wage example, we may want to know the impact of being a female and married in addition to being each individually (e.g. is there a ”marriage premium”?). To specify, log( wage i) = β 0 + β 1 · exper i+ β 2 · female i+ β 3 · fmarried i+ ϵ i, where fmarried i= female i· married i; so, we can observe the extra difference for someone who belongs to both groups. Dr Artur Semeyutin (BIE0014) MLR Business School28 / 33 Interactions (Cont’d)We can also allow our dummy variables to interact with other quantitative variables. This allows us not only to amend the intercept for different categories but also their slope. Similar to the model in the previous slide, we have log( wage i) = β 0 + β 1 · exper i+ β 2 · female i+ β 3 · fexper i+ ϵ i, where fexper i= female i· exper iand β 3 parameter now explains wage percentage difference for an extra year of experience for women compared to men. Next slide provides an illustrative example how dummy variables impact intercepts and slopes of our models using artificial data. Dr Artur Semeyutin (BIE0014) MLR Business School29 / 33 Interactions (Cont’d)* Note that this is an illustrative example with simulated (artificially created) data. Dr Artur Semeyutin (BIE0014) MLR Business School30 / 33* * * * * * * * * ** * * * * * * * * * * * * * * * * * * * * * * * * * * * * * * * * * * * * * * * * * * * * ** * * * * * * * * * * * * * * * * ** * * * * * * * * * * * * * * * * * * * * * * * * * * * * * * * * * * * * * * * * * * * * * * * * * * * * * * * * * * * * * * * * * * * * * * * * * * * * * * * * * * * * * * * * * * * * * * * * * * * * * * * * * * * * * * * * * * * * * * * * * * * * * 0 5 10 15 6.5 7.0 7.5 8.0 8.5 Dummy no Interaction Example X log(Y) * * * * * * * * * ** * * * * * * * * * * * * * * * * * * * * * * * * * * * * * * * * * * * * * * * * * * * * ** * * * * * * * * * * * * * * * * ** * * * * * * * * * * * * * * * * * * * * * * * * * * * * * * * * * * * * * * * * * * * * * * * * * * * * * * * * * * * * * * * * * * * * * * * * * * * * * * * * * * * * * * * * * * * * * * * * * * * * * * * * * * * * * * * * * * * * * * * * * * * * * 0 5 10 15 6.5 7.0 7.5 8.0 8.5 Dummy with Interaction Example X log(Y) Interactions (Cont’d) We could always construct a model where our categorical variable interacts with every quantitative variable, such as log( wage i) = β 0 + θ 0 · female i+ β 1 · exper i+ θ 1 · fexper i+ + β 2 · educ i+ θ 2 · feduc i+ β 3 · age i+ θ 3 · fage i+ ϵ i, where findicates our categorical-quantitative variables interaction. We can test if there is any wage difference due to gender by conducting an F -test (F stands for F distribution in the F-test. Do not be confused by our notation of the categorical variable!) with H 0 : θ 0 = θ 1 = θ 2 = θ 3 = 0. * We will cover tand Ftests in the next lecture (week). Dr Artur Semeyutin (BIE0014) MLR Business School31 / 33 Categorical Variables in the Time Series Setting We can also use categorical variables in a time series model. Often, our motivation is to use dummies in the time series setting when we expect certain periods to have different characteristics. For example, we may expect a different relationship between unemployment and inflation during a recession period and therefore, will formulate a model unemployment i= β 0 + β 1 · inflation i+ β 2 · rinflation i+ ϵ i, where rinflation iis our inflation variable multiplied by a dummy variable for time periods with value of 1 when we are in a recession. Dr Artur Semeyutin (BIE0014) MLR Business School32 / 33 Essential Reading Please read the textbook chapters: Chris Brooks - Introductory Econometrics for Finance, 4th Edition (2019) Cambridge University Press, Chapters 4 (you can stop on section 4.3) and 10 (you can stop on section 10.3). Go through Chapter 8 in the accompanyinge-book. There is an R script with seminar exercises on Brightspace for the upcoming week. Please practice and practice again before our next session - Econometrics is often learnt by doing! Dr Artur Semeyutin (BIE0014) MLR Business School33 / 33