Dynamical Systems in Psychological Science Lecture (Macquarie University) PDF
Document Details
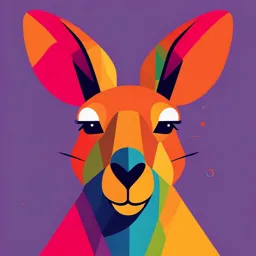
Uploaded by SuperbMagic
Macquarie University
2023
Michael J. Richardson
Tags
Summary
This lecture details dynamical systems in psychological science, presented by Professor Michael J. Richardson on 19/10/2023 at Macquarie University. The lecture covers definitions and examples in various contexts.
Full Transcript
PSYU3333 LECTURE: 19/10/2023 Dynamical Systems in Psychological Science PROF. MICHAEL J. RICHARDSON What is a Complex Dynamical System? What is a Dynamical System? GENERAL DEFINITION Dynamical: changes over time; time-evolving System: a collection of micro-scale (smaller scale) components, proc...
PSYU3333 LECTURE: 19/10/2023 Dynamical Systems in Psychological Science PROF. MICHAEL J. RICHARDSON What is a Complex Dynamical System? What is a Dynamical System? GENERAL DEFINITION Dynamical: changes over time; time-evolving System: a collection of micro-scale (smaller scale) components, processes, variables or parameters (things) that are linked together or work together to produce a macro-scale (larger scale) process, behaviour or behavioural outcome. Dynamical System: a system that evolves or changes over time. • • • • • • • • Is the human brain a dynamical system? Is the act of walking a dynamical system? Is conversation a dynamical system? Is personality a dynamical system? Is a society and culture a dynamical system? Is the ecosystem a dynamical system? Is the solar-system a dynamical system? Is a rock a dynamical system? 3 Non-Complex Dynamical Systems COMPLICATED ≠ COMPLEX Any machine (analog or digital) is a hard-molded or non-complex dynamical system, in that it is composed of a series of components (parts), each of which plays a specific, pre-determined and unchanging role that completely defines (a-priori) behavior over time. 4 Complex Dynamical Systems NATURAL AND BIOLOGICAL SYSTEMS = COMPLEXITY All biological or natural systems are soft-molded complex dynamical systems. The parts, components or agents of the system are flexible and interact in an adaptive and responsive manner, such that the role that each component (or agent) plays can change over time. 5 Complex Dynamical Systems DEFINITION – FOUR KEY ASPECTS TO A COMPLEX SYSTEM 1. A complex system consists of a large number of interacting components or agents. 2. The dynamics of complex systems are nonlinear, rather than linear. A nonlinear system is one in which the system’s outputs are not directly proportional to the system’s inputs. 3. Complex systems exhibit emergence. The collective behavior or organization of a complex system’s components or agents depends on the context or constraints imposed on the system – the stable behavior of a complex system is context dependent. 4. The behavior of a complex system is also self-organized. The collective behavior or organization of a complex system results from the local interaction of system components, rather than from a centralized controller or processes and can be difficult to anticipate. 6 Nonlinear Systems IN CONTRAST TO LINEAR SYSTEMS Output (y) Linear (proportional) relationship Nonlinear (non-proportional) relationship Input (x) A nonlinear system is one in which the system’s outputs are not directly proportional to the system’s inputs. Nonlinearity can also result from circular casual relations, a common property of complex dynamical systems. 7 Emergence and Self-Organization ORDER (ORGANIZATION) FORM NON-ORDER (NON-ORGANIZATION) Examples From Biology: Coordinated Activity of Social Insects: Ants always find the shortest path between their nest and a food source. How is this possible? 8 Emergence and Self-Organization ORDER (ORGANIZATION) FORM NON-ORDER (NON-ORGANIZATION) Examples From Biology: Coordinated Activity of Social Insects: Termites can build large elaborate structures together; including cathedral like halls inside. How is this possible? 9 Emergence and Self-Organization ORDER (ORGANIZATION) FORM NON-ORDER (NON-ORGANIZATION) Examples From Biology: Coordinated Activity of Social Insects: Termites can build large elaborate structures together; including cathedral like halls inside. How is this possible? 10 Emergence and Self-Organization ORDER (ORGANIZATION) FORM NON-ORDER (NON-ORGANIZATION) Examples From Biology: Coordinated Activity of Social Insects: Termites can build large elaborate structures together; including cathedral like halls inside. How is this possible? 11 Emergence and Self-Organization ORDER (ORGANIZATION) FORM NON-ORDER (NON-ORGANIZATION) Examples From Biology: Coordinated Activity of Social Insects: Termites can build large elaborate structures together; including cathedral like halls inside. How is this possible? 12 Flocking and Schooling Behaviour ORDER (ORGANIZATION) FORM NON-ORDER (NON-ORGANIZATION) https://www.youtube.com/watch?v=V5up0yaYz9E 13 Flocking and Schooling Behaviour ORDER (ORGANIZATION) FORM NON-ORDER (NON-ORGANIZATION) https://www.youtube.com/watch?v=xVaSiF2JVqQ 14 Flocking and Schooling Behaviour ORDER (ORGANIZATION) FORM NON-ORDER (NON-ORGANIZATION) Craig W. Reynold's Flocking Model: Agents in a 2-D or 3-D world with the following rules: 1. Alignment: Agents attracted towards the average heading (velocity) of other agents around them. 2. Cohesion: Agents attracted towards the average position of other agents around them. Simulated Flocking Behavior 3. Separation: Agents avoid colliding into other local agents. 15 Self-Organization ORDER (ORGANIZATION) FORM NON-ORDER (NON-ORGANIZATION) https://www.youtube.com/watch?v=Txrs4ssiAz0 https://www.youtube.com/watch?v=J4J__lOOV2E 16 Dynamical Human Behaviour and Fractal Time-Series Data Dynamics of Behavioural Processes TIME-SERIES OR EVENT-SERIES DATA 30 20 10 Daily Hedonic Level 9 8 7 6 5 4 3 2 3 2 1 0 -1 -2 -3 0 5 10 15 20 25 Session 30 35 40 45 12 9 6 3 50 0 1 2 3 4 5 6 7 8 9 Time (seconds) 10 11 12 13 14 15 Stochastic 1500 RT (msec) Self-Esteem 0 Periodic 15 1 1000 500 0 65 129 193 257 321 385 449 513 577 641 705 769 833 897 961 Trial 64 128 192 256 Trial 320 384 448 512 Aperiodic & Complex Numeric Code Anxiety 40 Limb Position Monotonic 50 1 8 15 22 29 36 43 50 Time (seconds) 57 64 71 78 6 5 4 3 2 1 0 0 500 1000 1500 2000 Time (msec) 2500 3000 3500 18 Dynamics of Behavioural Processes COMPLEX TIME- AND EVENT-SERIES DYNAMICS Language Dynamics Postural Sway Dynamics 1 Word sequence of Hello Goodbye lyrics 0.8 20 Numeric code (word) Behavioral Series 25 0.6 0.4 0.2 0 0 1000 500 1500 15 10 5 0 Time (points) 60 40 20 0 100 80 Time (word) 120 180 160 140 j, time (word) 12 10 8 6 4 2 say go go go oh no 14 say no you say stopand i 16 you say yes i 18 Y(j) points Recurrence Plot of the Dynamic Structure Recurrence Plot 0 X(i) points you say yes i 0 2 4 say no you say stopand i 6 8 10 i, time (word) say go go go oh no 12 14 16 18 19 Dynamics of Behavioural Processes STOCHASTIC BEHAVIOURS 9 Self-Esteem 8 7 6 5 4 3 2 1 65 129 193 257 321 385 449 513 577 641 705 769 833 897 961 Trial 1400 RT (msec) 1200 1000 Is Human Behavioral Variability Random? No 800 600 400 200 0 64 128 192 256 Trial 320 384 448 512 20 Fractal Structures Measurement Measurement Measurement SELF-SIMILAR OR SCALE-INVARIANT STRUCTURES 9 8 7 6 5 4 3 2 9 8 7 6 5 4 3 2 9 8 7 6 5 4 3 2 1 512 Trial 1024 1 256 Trial 512 1 128 Trial 256 21 Human Behavioural Variability EXHIBITS FRACTAL DYNAMICS Brown Deterministic (persistent) Pink 0 64 128 192 256 320 384 448 512 0 64 128 192 256 320 384 448 512 Anti-Persistent 0 64 128 192 256 320 384 448 512 Blue Random 0 64 128 192 256 320 Time (samples) 384 448 512 White Pink or Fractal Noise is Characteristic of Adaptive, Flexible and Robust human and animal behavior, at nearly every scale of behavior. 22 Human Behavioural Variability EXHIBITS FRACTAL DYNAMICS Fluctuations in self-esteem and physical self-worth are fractal (pink) A result of an individuals coupling to everyday social and environmental events. Delignières, D., Fortes, M., & Ninot, G. (2004) The fractal dynamics of self-esteem and physical self. Nonlinear Dynamics in Psychology and Life Science, 8, 479-510 23 Constraints on Behavioural Variability INCREASE OR DECREASE DETERMINISTIC STRUCTURE Brown Deterministic (persistent) Pink 0 64 128 192 256 320 384 448 512 Random 0 64 128 192 256 320 384 448 512 White Anti-Persistent 0 64 128 192 256 320 384 448 512 Blue 0 64 128 192 256 320 Time (samples) 384 448 512 Washburn et al., 2015 Under constrained/unstable critical stable behavior (balanced) Overly constrained/stable 24 Coupled Human Behavioural Variability COMPLEXITY MATCHING Correlated Fractal = Complexity Matching Person 2 High Structure Low Structure Low Structure Person 1 High Structure 25 Coupled Human Behavioural Variability COMPLEXITY MATCHING IN THE WILD (RIGOLI ET AL., 2020) Fractal Structure of an Individuals Movements were different for different tasks and task goals Complexity-Matching between Individuals who completed a task together was invariant to task or task goal. Provide a Task Independent Index of Co-Action. 26 Dynamics of Social Motor Coordination Dynamics of Behavioural Processes Interpersonal Coordination Intrapersonal Interlimb Coordination COUPLED PERIODIC/RHYTHMIC BEHAVIOURS 28 Social Motor Coordination COUPLED PERIODIC/RHYTHMIC BEHAVIOURS Manipulated Competition (∆ω) Attached weights to the chairs (ch): Peripheral information (weak coupling) ∆ω ≠ 0 (Lch ≠ Rch: competition) Focal information (strong coupling) ∆ω = 0 ∆ω ≠ 0 0 30 50 70 90 110 130 150 180 Relative Phase Peripheral Information 25 20 15 10 5 0 Focal Information % Occurence 25 20 15 10 5 0 No information (no coupling) ∆ω = 0 (Lch = Rch: no competition) % Occurence % Occurence No Information Manipulated Cooperation (visual coupling) 0 30 50 70 90 110 130 150 180 Relative Phase 50 40 30 20 10 0 0 30 50 70 90 110 130 150 180 Relative Phase 29 Social Motor Coordination COUPLED PERIODIC/RHYTHMIC BEHAVIOURS • More harmonious interactions (e.g., Miles et al., 2010, 2009) • More rapport (e.g., Hove & , 2010). • More social connection (e.g., Marsh et al., 2009). Participants instructed to completing a stepping task behind a ‘confederate’ participant who was either on-time or late the to experimental session. Miles et al., (2010) • Can reduce perceived interpersonal differences and decrease prejudice (e.g., Miles et al., 2011). • Results in better individual and interpersonal task performance (e.g., Richardson et al., 2005; Tolston et al. 2010). 30 Social Motor Coordination COUPLED PERIODIC/RHYTHMIC BEHAVIOURS Group status manipulated by ‘art preference’, and a resulting categorization of red (in-group) and blue (out-group) Recorded oscillatory forearm movements about the elbow (participants told that movements were to ensure arousal prior to meeting with coparticipant) . % Occurence Miles et al., 2011. In-group Out-group 50 40 30 20 10 0 0 30 50 70 90 110 130 150 180 Relative Phase 31 Dynamics of Rhythmic Coordination COMPETITIVE RUNNING AND SPRINTING (VARLET & RICHARDSON, 2015) Coordination in Final Chance Coordination Determine from Semifinals Examined the stride coordination between Usain Bolt and Tyson Gay in100-m final of the 12th IAAF World Championship in Athletics (Berlin, 2009) in which Bolt broke the world record. How do we Model the Dynamics of Human Behaviour? What is a Dynamical System? FORMAL (MATHEMATICAL) DEFINITION Dynamical System: a set of rules or equations that determine (define) the future state of a system given the system’s current state. What is a state? A state could be anything… • The position or velocity of your hand as you wave to someone • Your body weight or height • Your reaction time to perceiving or comprehending an environmental stimulus • Your attitude about a product or food • Your level of self-esteem • Your speaking rate • The number of items you can recall during a memory test • The number of fiends you have on Facebook 34 Computational Dynamical System FORMAL (MATHEMATICAL) EXAMPLE A set of rules or equations that determine the future states of a dynamical system at discrete time steps. 𝑥𝑥𝑡𝑡+1 = 𝑅𝑅𝑥𝑥𝑡𝑡 𝑥𝑥𝑡𝑡 1− 𝑎𝑎 x : number of students attending lecture t : lecture number (i.e., 1, 2, … n lectures) R : professors teacher rating/ability on a scale of 0 (low) to 4 (high) a : number seats available in lecture hall (e.g., 50, 100, … etc.) Note that 1 − when xinitial > 0. 𝑥𝑥𝑡𝑡 𝑎𝑎 restricts the value of x to a range of 0 to the value of a, 35 Discrete Dynamical System FORMAL (MATHEMATICAL) EXAMPLE 𝑥𝑥𝑡𝑡+1 = 𝑅𝑅𝑥𝑥𝑡𝑡 Lecture # 𝑥𝑥𝑡𝑡 1− 𝑎𝑎 Lecture # Lecture # 36 Discrete Dynamical System FORMAL (MATHEMATICAL) EXAMPLE 𝑥𝑥𝑡𝑡+1 = 𝑅𝑅𝑥𝑥𝑡𝑡 Lecture # 𝑥𝑥𝑡𝑡 1− 𝑎𝑎 Lecture # Lecture # 37 Complexity from Non-Complexity SIMPLE RULES CAN RESULT IN COMPLEX, UNPREDICTABLE BEHAVIOUR xt+1 = Rxt(1-xt) Logistic Equation 𝑥𝑥𝑡𝑡+1 = 𝑅𝑅𝑥𝑥𝑡𝑡 1 − 𝑥𝑥𝑡𝑡 Chaos when R > 3.57 38 Chaos UNPREDICTABLE, YET DETERMINISTIC BEHAVIOUR => HUMAN BEHAVIOUR? Behavior that is determinism, aperiodic, bounded and exhibits a sensitive dependence on initial conditions. o Deterministic: Definite rule with no random or stochastic processes. o Aperiodic: Never repeats the same state twice. o Bounded: System state stays within a finite range and does not approach ± infinity. o Sensitive dependence on initial conditions: Two points that are initially close will drift apart as time proceeds. 39 Continuous Dynamical System FORMAL (MATHEMATICAL) EXAMPLE A set of rules or equations that determine the future states of a dynamical system continuously over time (typically using differential equations). Hodgkin-Huxley Model of Neuron Firing/Spiking 40 Dynamical Models of Stable Behavioural States ORDER PARAMETER EQUATIONS – EXAMPLE 1 An equation that determines the stable states of a dynamical system. Attitude Change x = −k + x − x 3 k is scaled from -1 to 1 to capture ratio of positive to negative information about person/group/thing. • Nonlinear Transition: Attitude change occurs abruptly. neg pos neg pos neg pos neg pos neg pos pos neg pos Negative to Positive Information • Multi-stability: Certain ration of information could result in negative OR positive attitude. • Hysteresis: Need greater pos/neg information to result in a chance in attitude (state is history dependent). neg pos neg pos neg pos neg Positive to Negative Information 41 Dynamical Models of Stable Behavioural States ORDER PARAMETER EQUATIONS – EXAMPLE 2 𝑥𝑥̇ = 𝑎𝑎 + 𝑏𝑏𝑏𝑏 − 𝑥𝑥 3 Here, social pressure refers to any family or societal pressure on an individual "not to date" a certain type of individual. Thus, social pressure is known as a splitting factor High Behavioral manifold Cusp (fold curve) Chance of Dating (x) Has been used to describe the sudden changes in the dating behavior of couples given two interacting forces: a = love and b = social pressure (Tesser, 1980; Tesser & Achee, 1994). Low Cusp Model Points on manifold are the ‘fixed point’ attractors for different values of a and b. (High) a (Low) (Low) b (High) 42 Agent Based Dynamical Modelling (ABMs): NETWORKS OR STRCUTURES OF DYNAMICAL RULES • Computer simulation of individual ‘agents’ in a dynamic or social system (environment or space). • Agents represent heterogeneous individuals that interact with each other and/or their environment based on a set rules. • The aim of ABM is capture global consequences of local agent-based interactions (i.e., how the local (micro) behavior of interacting agents generates complex emergent (macro) behavioral order. • Each agent is defined by a dynamical; rule or set of rules that are coupled/interact with the dynamical rules of the agents around them. (see e.g., Axelrod & Dion, 1988; Freeman and Ambady; 2011; Smith & Collins, 2009) Flocking and Schooling Behaviour ORDER (ORGANIZATION) FORM NON-ORDER (NON-ORGANIZATION) Craig W. Reynold's Flocking Model: Agents in a 2-D or 3-D world with the following rules: 1. Alignment: Agents attracted towards the average heading (velocity) of other agents around them. 2. Cohesion: Agents attracted towards the average position of other agents around them. Simulated Flocking Behavior 3. Separation: Agents avoid colliding into other local agents. 44 Schelling’s Segregation Model: SELF-ORGANIZATION IN HUMAN BEHAVIOUR 45 Schelling’s Segregation Model: SELF-ORGANIZATION IN HUMAN BEHAVIOUR Red and Blue Agents live in a 2-D world with the following rules: • Stay if at least third of neighbors are the same kind • Move to random location otherwise Parameters: • Tolerance: acceptance of diversity (main manipulation) • Vacancies: percentage of empty houses in the neighborhood • Ratio of Races: average fraction of reds (vs. blues) in the population (or visa versa). • Relocation: percentage of the population group seeking a new house. 46 How do we Test and Validate Dynamical Models of Human Behaviour? Simulation, Virtual-Reality and… Turing Tests (again). Case Study: Human Shepherding Validating Dynamical Models of Social and Multiagent Activities using VR and Artificial Agents. Object Pick & Place Tasks Small Space Coordination – Bartending Multi-Agent Shepherding Nalepka, Kallen, Chemero, Saltzman, & Richardson (2017). Two novice players required to herd a small flock of sheep into a central containment region 3-Sheep Condition 5-Sheep Condition 7-Sheep Condition Multi-Agent Shepherding Nalepka, Kallen, Chemero, Saltzman, & Richardson (2017). Coupled Oscillatory Containment (COC) Seek and Recover (S&R) Evolution of the Behavioral Dynamics Pairs initially adopt S&R behavior Over time pairs get better at herding sheep Spontaneously discover COC behavior Pairs naturally converge on the same stable modes of behavioral coordination, with the emergence of COC a functional consequence of the physical and informational constraints of the task space and ongoing task performance. Multi-Agent Shepherding Model Nalepka, Kallen, Chemero, Saltzman, & Richardson (2017). Herders follow two basic rules: 1. Corral: Move to the position of the target furthest from the containment region and closest to me + some positive preferred distance offset. 2. Contain: When all targets are in containment region, oscillate around the herd on ‘my’ side of game field. https://www.youtube.com/watch?v=9dKMJImp9zY Human Intelligence vs. Artificial Intelligence TURING TEST To pass the Turing Test, the computer program only needs to fool people 30% of the time. 52 Multi-Agent Shepherding: Turing Test Nalepka, Kallen, Chemero, Saltzman, & Richardson (2019). Participants in Human-AI condition: 70% of People thought they were interacting with another Human actor Turing Test Caveat: In recent and other task contexts, research has shown how 30% of people in human-human conditions often think their partner is an AI. Reverse Turing Test Suggested/Further Reading IF YOU ARE INTERESTED IN LEARNING MORE Suggested Reading Richardson, M. J. Dale R., & Marsh, K. L., (2014). Complex Dynamical Systems in Social and Personality Psychology: Theory, Modelling and Analysis. In H. T. Reis, and C. M. Judd. (Eds.). Handbook of Research Methods in Social and Personality Psychology, 2nd Edition. (pp. 253-282) New York, NY: Cambridge University Press. Other General References • Anderson, M. L., Richardson, M. J. and Chemero, A. (2012). Eroding the boundaries of cognition: implications of embodiment. Topics in Cognitive Science. doi: 10.1111/j.17568765.2012.01211.x. • Arrow, H., & McGrath, J.E., & Berdahl, J.L. (2000). Small groups as complex systems: formation, coordination, development, and adaptation. Thousand Oaks, CA: Sage. • Baron,R.M.,Amazeen,P.G.,&Beek,P.J.(1994).Local and global dynamics of social relations. In R.R.Vallacher & A. Nowak (Eds.). Dynamical systems in social psychology (pp. 111–138). New York: Academic Press • Brown, K. W., Moskowitz, D. S. (1998): Dynamic stability: The rhythms of our daily lives. Journal of Personality, 66, 105–134 • Dale, R., & Spivey, M. J. (2005). Categorical recurrence analysis of child language. Proceedings of the 27th Annual Meeting of the Cognitive Science Society. 530–535. • Delignières, D., Fortes, M., & Ninot, G. (2004) The fractal dynamics of self-esteem and physical self. Nonlinear Dynamics in Psychology and Life Science, 8, 479-510 • Gilden, D. L. (2001). Cognitive emissions of 1⁄ƒ noise. Psychological Review, 108, 33–56. • Gottman, J., Murray, J., Swanson, C., Tyson, R., & Swanson, K., (2003). The mathematics of marriage: Dynamic nonlinear models. Cambridge, MA: MIT Press. • Guastello, S. J., Koopmans, M., & Pincus, D. (2009). Chaos and complexity in psychology: Theory of nonlinear dynamics. New York: Cambridge University Press. • Heath, R. A. (2000). Nonlinear dynamics: techniques and applications in psychology. Hillsdale, NJ: Erlbaum • Kaplan, D., & Glass, L. (1995). Understanding nonlinear dynamics. New York, NY: SpringerVerlag. • Kelso, J. A. S. (1995). Dynamic patterns. Cambridge, MA: MIT Press. • Miles, L. K., Lumsden, J., Richardson, M. J., & Macrae, N. C. (2011). Do Birds of a Feather Move Together? Group Membership and Behavioral Synchrony. Experimental Brain Research, 211, 495-503. • Miles, L. K., Griffiths, J. L., Richardson, M. J., & Macrae, N. C. (2010). Too Late to Coordinate: Target Antipathy Impedes Behavioral Synchrony. European Journal of Social Psychology, 40, 52–60 • Miller, J. H., & Page, S. E. (2009). Complex adaptive systems: An introduction to computational models of social life (Vol. 17). Princeton university press. • Nalepka, P., Lamb, M., Kallen, R. W., Shockley, K., Chemero, A., Saltzman, E., & Richardson, M. J. (2019). Human social motor solutions for human–machine interaction in dynamical task contexts. Proceedings of the National Academy of Sciences, 116(4), 1437-1446. • Nowak, A., & Vallacher, R. R. (1998). Dynamical social psychology. New York: Guilford Press. • Rigoli, L. M., Lorenz, T., Coey, C., Kallen, R., Jordan, S., & Richardson, M. J. (2020). co-actors exhibit Similarity in their Structure of Behavioural Variation that Remains Stable Across Range of naturalistic Activities. Scientific reports, 10(1), 1-11. • Schmidt, R. C., & Richardson, M. J. (2008). Dynamics of Interpersonal Coordination. In A. Fuchs & V. Jirsa (Eds.). Coordination: Neural, Behavioral and Social Dynamics. (pp. 281-308). Heidelberg: Springer-Verlag. • Shelling, T. (1971). Dynamic models of segregation. Journal of Mathematical Sociology 1, 143186. • Smith, E. R., & Conrey, F. R. (2007). Agent-based modeling: A new approach for theory building in social psychology. Personality and Social Psychology Review, 11, 87-104 • Tesser, A., & Achee, J. (1994). Aggression, love, conformity, and other social psychological catastrophes. In R. R. Vallacher & A. Nowak (Eds.), Dynamical systems in social psychology (pp. 96-109). San Diego, CA: Academic. • Thelen, E., & Smith, L. B. (1994). A dynamical systems approach to the development of cognition and action. Cambridge, MA: Bradford-MIT Press. • Vallacher, R. R. & Nowak, A. (1994). Dynamical systems in social psychology. San Diego: Academic Press. • Van Orden, G. C, Kloos, H., & Wallot, S. (2011). Living in the pink: intentionality, wellbeing, and complexity (pp. 639-684). In C. A. Hooker (Ed.), Philosophy of complex systems. handbook of the philosophy of science. Amsterdam: Elsevier. 54 Questions? [email protected] www.mq.edu.au