Week 11 Quantitative Research Methods PDF
Document Details
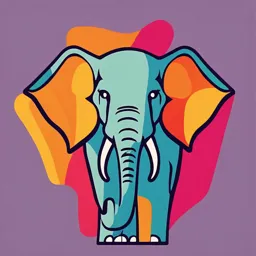
Uploaded by SnazzyMossAgate2157
Western University
Tags
Summary
This document provides an overview of quantitative research methods, focusing on data collection techniques, reliability, and validity. It discusses various methods, including biological/physiological measures, structured observation, and questionnaires, highlighting their strengths and weaknesses. The importance of ensuring data consistency and inter-rater reliability is emphasized, along with factors affecting validity and reliability of measurement instruments. A conceptual overview is given for researchers and students.
Full Transcript
Week 11 – Quantitative research con’t – Data collection, reliability/validity, interpreting findings Data Collection Methods Success of a study depends on the quality of the data-collection methods chosen and employed. Types – Bio/physio, observational, questionaries, surveys, records or available...
Week 11 – Quantitative research con’t – Data collection, reliability/validity, interpreting findings Data Collection Methods Success of a study depends on the quality of the data-collection methods chosen and employed. Types – Bio/physio, observational, questionaries, surveys, records or available data, interview (qualitative). Many studies use a multi-method approach. In nursing we do all the following – dependent on the research purpose and question Control – Achieved through data consistency Achieved by measuring the data in the same manner for each participant. A data collection protocol is needed to ensure intervention fidelity. Co-investigators and assistants need to be trained to ensure all follow the protocol. Ensures interrater reliability (people coding data are doing so in a consistent way with a high degree of agreement) Biological/Physiological measures Types: physical (blood pressure, oxygen saturation), anatomical (e.g. brain scan), chemical (e.g. cortisol, blood glucose), or microbiological (e.g. bacterial cultures). Arguably, most objective measure you can obtain (most accurate, least margin for error) Advantages: objective, precise, & sensitive Disadvantages: can be invasive, expensive, hard to obtain; may need special training; may cause reactive effects Structured Observation For some research questions, direct observation of people’s behaviour is used as an alternative to self-report measurement (i.e. surveys, questionnaires). Observational methods can be used to gather such information as patients’ conditions (e.g. sleep-wake states), verbal communication (e.g. exchange of information at discharge), non-verbal communication (e.g. body language), activities (e.g. geriatric patients’ self-grooming activities), and environmental conditions (e.g. noise levels). Advantages - ideal for studying complex interactions & measuring people’s actions / reactions Disadvantages - reactivity effects, observer bias Consider - “control” of experiment / study – Consistent with study objectives, Conceptual / theoretical basis for observations, Standardized & systematic observation & recording, Control & check of observations Records/Available Data Types - medical records, administrative data, death certificates, census, NPHS, etc. Advantages - usually inexpensive, historical Disadvantages - availability, ethics, bias, missing data Consider - changes over time, inter-rater reliability, what is collected may differ by place/person, data often collected for other purpose Questionnaires (aka “instruments” “standardized measurement tools”) Can be collected face-to-face, over the phone, paper & pencil, or electronic/web-based Advantages - fast, economical, variety, participants can remain anonymous Disadvantages - breadth vs. depth, response rates, recall bias, social desirability bias, incomplete items Can look like - Questions: open- and close-ended, Scales: Likert-type (e.g., 1-7), visual analogue, etc., Validity & reliability (in a few slides) Dichotomous questions require respondents to make a choice between two response alternatives, such as yes/no or male/female. MCQ: 3 or more responses. Getting more information than dichotomous--Better for ascertaining things like opinions—account for intensity & direction of opinions Rank-order questions ask respondents to rank target concepts along a continuum, such as most to least important. Forced-choice--choose between two statements that represent polar positions or characteristics. Rating questions ask respondents to evaluate something along an ordered dimension. Common Issues with Self-reported survey Data Response biases – social desirability, extreme responses, acquiescence responses set (yea sayers), Nay sayers response set Reliability and Validity of Measurement instruments Two key attributes for judging the quality of any measurement tool Reliability - How “stable” or “consistent” is the measurement? How “repeatable” is the measurement? Validity - is the instrument measuring what it is supposed to measure? Concerned with systematic error Reliability – Homogeneity aka consistency Internal Reliability sometime called Internal consistency Stability within an instrument – looking to ensure items measure the same attribute (e.g., if the tool is measuring depression, all items on the tool measure some aspect of depression) Most widely used reliability approach - Measured using Cronbach alpha (coefficient alpha) statistic – normal range is between 0 to +1, higher values are associated with greater internal reliability Item-total correlation - Measures stability among subjects, Performance on a single-item is consistent with performance on all other items Interrater reliability - Stability among raters - Equivalence – agreement between multiple raters regarding the scoring - Expressed as a percentage (92% inter-rater reliability), Cohen’s Kappa value, or Intraclass correlation coefficient, Cohen’s Kappa values of 0.75 is considered good, Intraclass correlation coefficient (ICC) looks at the consistency between the raters Test-retest correlation coefficient (stability) Stability over time – ability to obtain similar scores in different situations and the effect of external factors on the instrument, An instrument’s ability to detect differences in subjects over time, Reliability coefficient – comparison of multiple administrations of the same measure to subjects - Give information about the magnitude of reliability, Reliability coefficient above 0.80 is considered good , Looks at the magnitude and direction of a relationship ranging from +1—0--(-1) - (There can be a perfect (0), positive (+1) or negative (-1) relationship, The higher the coefficient, the more the stable the relationship) Reliability and Validity Reliability – results of repeated measures, coefficient 0-1.0, Coefficient: ≥0.70 is reliable for non-biological (higher expected for biological), Stability – test-retest reliability, Cronbach alpha is common Validity (of Measures) Recall validity of results (threats are internal: selection, attrition, maturation, instrumentation, testing, & history bias & external: selection, measurement, & reactive effects) Validity of measurement tool (*more on next slides) Content - Does the tool & its items measure the construct under study? Criterion-related - Does the outcome equate with the behaviour? Construct - How well does the tool measure a construct? Content Validity Degree to which items in instrument represents the concept. Classical approaches to assessing - evidence that the content domain of an instrument is appropriate relative to its intended use, the use of lay and expert panel (clinician) judgments Subtype - Face validity – “looks like” it measures concept, very rudimentary Criterion-Related Validity Relationship between instrument and some external criterion. Types - Concurrent validity = degree of correlation between two measures of the same concept given at the same time – e.g., correlating Self-efficacy for exercise scale results with whether subjects engaged in regular exercise Construct Validity Construct validity is concerned with the questions: What is this instrument really measuring? Does it adequately measure the construct of interest? Establishing construct validity is a complex process, often involving several studies and approaches. Most forms of validity evidence fall under “construct” validity, Construct = an abstract concept such as intelligence, self-concept, motivation, aggression, pain. The construct can be observed by some type of instrument Hypothesis testing - Do scores on measure correlate with hypothesized behaviour? Example: Breastfeeding Self-Efficacy Scale-Short Form (BSEF-SF). Construct validity was tested positively on the basis of this hypotheses: (1) women who had previously breastfed would have higher breastfeeding self-efficacy than those who had not Convergent validity - exists when two or more tools that are intended to measure the same construct are administered to participants and are found to be positively correlated. We can validate a new depression scale by comparing it to a “gold standard” instrument like the Beck Depression Inventory - If total scores are both scales are highly, positively correlated, then our new scale has good construct validity. Divergent validity - requires measurement approaches that differentiate one construct from others that may be similar. Sometimes researchers search for instruments that measure the opposite of the construct. If the divergent measure is negatively related to other measures, the measure’s validity is strengthened…. e.g. compare BDI scores with scores on a “happiness” scale—we would expect there to be a strong negative correlation. Validity vs Reliability Validity is about the appropriateness of a test – highly valid test (should) has by definition high reliability Reliability is about the consistency of the scores produced – if a test is measuring what it is supposed to be measuring, it will be reliable. Highly reliable tests give no warrantee to be highly valid. A reliable test can consistently measure the wrong thing and be invalid. Validity is a lot harder to determine than reliability. Most research papers will only report the attributes of reliability Data Analysis Data analysis is the process of making meaning from the data The outcome of data collection is gathering considerable numerical data that are typically disorganised and made up of separate bits of information with unclear meaning. Statistical analysis help to condense a vast body of data into an amount of information that the mind can more easily understand. Statistical analysis also helps to identify patterns and relationships within the data that they may otherwise go unnoticed. Capture variability (variance) – how the scores vary across participants - And identify what is typical and atypical among the data. Discover meaning and relationships between & across variables Answer research questions or test hypotheses Results Analyzing the research data results Results and Findings - Usually results are provided in text and through the use of tables and figures. Should reflect the study objectives, questions and/or hypothesis tested. Negative results (no statistical significance; unsupported hypotheses ☹) & positive (statistical significance; supported hypotheses ☺) Statistical differences presented Interpreting the data Descriptive Statistic Analysis: data reduction: to summarize or describe the characteristics of a set of data (i.e., frequency, mean, mode, range,… Frequency – how many times in a data set Mean – average of the data set Mode – value that appears most often in a set of data Range – difference between largest and smallest values in a data set Descriptive – Mean and SD Mean is the “average value” from a data set, It is a single number summary for a mass of data points which allows for easy comparison between groups, However, it is sensitive to extreme scores (e.g. outliers) Standard Deviation (SD) is the most common measure of variance, SD describes how far the values stray from the Mean, A small SD will have scores closer to the Mean, while data with a large SD will have scores scattered over a wider range around the mean. Inferential Statistics Combines mathematical processes and logical; allow researchers to test hypotheses about a population using data obtained from probability and nonprobability samples Tests of difference (i.e. Chi-square, t statistic, ANOVA, ANCOVA, MANOVA) Tests of relationships – Correlations Assist in generalizing findings from a sample to a larger population Statistical Significance To learn whether the difference is statistically significant, we need to compare the probability number (the p-value) to the critical probability value determined ahead of time (aka the alpha level which we usually set 0.05 or 0.01). If the p-value is less than the alpha value, you can conclude that the difference you observed is statistically significant. P-Value (stands for “probability value”: is the probability that the results were due to chance and not based on your program/intervention. The lower the p-value, the more likely it is that a difference occurred as a result of your program. P-values range from 0 – 1. p = 0.01 (statistically significant; results are not due to chance alone but due to a true effect) Confidence Interval Range of values that is likely to contain the population (as opposed to sample) mean. Most reported in research is a 95% degree of certainty, meaning 95% of the time, the findings will fall within the range of values given as the CI. Reported as 95% CI -0.10 to 0.08 Most quantitative research studies report: Significance, Means (average values), Some measure of standard deviation/variance, Confidence intervals, Effect size Effect Size Measure of the magnitude (how big) of the effect (group difference) is. Small (correlations around 0.20) - Requires larger sample size Medium (correlations around 0.40) -Requires medium sample size Large (correlations around 0.60) -Requires smaller sample size Inferential… the p Value The long-held minimum threshold for acceptance is 95% or p <.05, Any result that achieves this threshold is considered statistically significant, So what about these: p <.06 p <.10. They crossed the threshold which means the differences observed involve too much chance for us to accept The Importance of Results Statistically Significance does not mean Clinically Important. Statistical Significance indicates that the results were unlikely to be due to chance. With a large sample even modest relationships are statistically significant Conversely, the absence of statistically significant results does not mean that the finding is unimportant. Example: If an adequately powered study was comparing the end-result of two wound debridement techniques in terms of healing at 3 weeks, and found no difference, that finding of no significant difference would be clinically important if one of the techniques happened to be less painful and less costly.