Fundamentals of HR Analytics PDF
Document Details
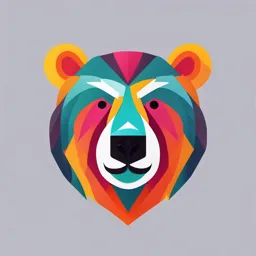
Uploaded by BoomingChiasmus
JAIN Online University
Tags
Summary
This document provides an overview of predictive modeling in HR analytics for Bachelors of Business Administration. It covers topics such as data preparation, modeling techniques, and key HR metrics.
Full Transcript
Fundamentals of HR Analytics Fundamentals of HR Analytics Unit-04 Predictive Analytics in HR Semester-05 1 Bachelor Bachelors of Commerce of Business Administration ...
Fundamentals of HR Analytics Fundamentals of HR Analytics Unit-04 Predictive Analytics in HR Semester-05 1 Bachelor Bachelors of Commerce of Business Administration UNIT 04: Predictive Analytics in HR UNIT 04 Predictive Analytics in HR Names of Sub-Unit Introduction to predictive modelling in HR analytics, Predictive modelling techniques for HR- related predictions, Data Preparation for Predictive HR Analytics, HR Metrics for Predictive Analytics, Challenges and Ethical Considerations in Predictive HR Analytics, Improving Decision-Making with Predictive Analytics. Overview This course provides a comprehensive introduction to predictive modeling in HR analytics. You'll explore techniques for making data-driven predictions in various HR domains, understand the critical process of data preparation, and delve into HR metrics for predictive analytics. Additionally, you'll address challenges and ethical considerations, ultimately improving decision-making with predictive analytics. Learning Objectives In this Unit you will learn: Understand the concept and significance of predictive modeling in HR analytics. Explore various predictive modeling techniques tailored for HR-related predictions. Master the essential data preparation steps for predictive HR analytics. Recognize the key HR metrics and ethical considerations in predictive HR analytics. 2 Fundamentals of HR Analytics Learning Outcomes At the end of this Unit you would be equipped with the knowledge and skills to harness predictive analytics for more informed HR decision-making enhanced workforce management. Pre-Unit Preparatory Material "Predictive Analytics in HR: A Practical Guide" by Ian J. Cook and Keith McNulty. "Ethical Considerations in HR Analytics" - An article from the Harvard Business Review. Table of Topics 4.1 Introduction to Predictive Modeling in HR Analytics 4.2 Predictive Modeling Techniques in HR 4.3 Data Preparation for Predictive HR Analytics 4.4 HR Metrics for Predictive Analytics 4.5 Challenges and Ethical Considerations in Predictive HR Analytics 4.6 Improving Decision-Making with Predictive Analytics in HR 4.7 Conclusion 3 UNIT 04: Predictive Analytics in HR 4.1 Introduction to Predictive Modeling in HR Analytics Predictive modeling is a powerful tool in the field of HR analytics, enabling organizations to make informed and data-driven decisions regarding their workforce. By harnessing the potential of data, predictive modeling helps HR professionals anticipate future trends, identify potential issues, and optimize various aspects of workforce management. This article explores the fundamentals of predictive modeling and its application in HR, shedding light on the concept of using data to make future workforce-related predictions. What is Predictive Modeling in HR Analytics? Predictive modeling in HR analytics involves using statistical and machine learning techniques to analyze historical data, identify patterns, and make predictions about future workforce-related events and outcomes. These predictions can relate to a wide range of HR functions, including talent acquisition, employee retention, performance management, and workforce planning. Key Elements of Predictive Modeling in HR: Data Collection: To build predictive models, HR teams gather and organize relevant data from various sources. This data might include employee information, performance metrics, recruitment data, and more. The quality and quantity of data are crucial for the accuracy of predictions. Data Preprocessing: Before modeling, the collected data needs to be cleaned, transformed, and prepared for analysis. This step involves handling missing values, removing outliers, and encoding categorical variables, among other tasks. Model Selection: HR analysts select the most suitable predictive modeling techniques for their specific objectives. Common approaches include regression analysis, decision trees, random forests, and machine learning algorithms such as neural networks. Feature Engineering: Feature engineering is the process of selecting and engineering the most relevant variables (features) from the data that will have the most significant impact on the predictive model's performance. Training the Model: This step involves using historical data to train the predictive model. The model learns from past patterns and relationships within the data. Validation and Testing: After training, the model is tested using a separate set of data to evaluate its accuracy, generalization, and ability to make predictions about future events. Cross-validation techniques may also be applied. Applications of Predictive Modeling in HR: 4 Fundamentals of HR Analytics Talent Acquisition: Predictive modeling can help identify the best sources of talent, optimize recruitment strategies, and forecast hiring needs based on historical data and market trends. Employee Retention: By analyzing factors that contribute to employee turnover, predictive modeling can predict which employees are most likely to leave and allow HR to take proactive steps to retain key talent. Performance Management: HR can use predictive modeling to identify factors that impact employee performance, helping in the development of personalized performance improvement plans. Workforce Planning: Predictive models can assist in workforce planning by forecasting future staffing needs, allowing organizations to align their workforce with strategic goals. Diversity and Inclusion: Predictive modeling can help organizations track and improve diversity and inclusion initiatives by identifying areas where intervention is needed. Benefits of Predictive Modeling in HR: Data-Driven Decision Making: Predictive modeling enables HR professionals to make informed, data-driven decisions, reducing reliance on intuition and guesswork. Cost Reduction: By predicting workforce-related issues, organizations can reduce recruitment costs, lower employee turnover, and optimize resource allocation. Enhanced Talent Management: HR can better identify high-potential employees and provide them with development opportunities, improving talent management and succession planning. Increased Efficiency: Predictive models help in streamlining HR processes, reducing administrative burden, and focusing resources on strategic initiatives. In conclusion, predictive modeling in HR analytics is a valuable approach that empowers organizations to make proactive and strategic decisions related to their workforce. By leveraging data and advanced analytics techniques, HR professionals can better anticipate future trends and challenges, ultimately leading to more effective and efficient workforce management. 4.2 Predictive Modeling Techniques in HR Predictive modeling in HR leverages various techniques to forecast HR-related outcomes such as employee turnover, performance, and other workforce-related metrics. Here, we'll delve into some of the key predictive modeling techniques commonly employed in HR analytics: 5 UNIT 04: Predictive Analytics in HR Regression Analysis: Linear Regression: Linear regression is a simple yet powerful technique for predicting a continuous outcome variable (e.g., employee performance) based on one or more predictor variables (e.g., training hours, years of experience). It provides insight into the strength and direction of relationships between variables. Logistic Regression: Logistic regression is used when the outcome is binary (e.g., turnover - stay or leave). It estimates the probability of an event occurring based on predictor variables. In HR, it is often used to predict employee turnover. Decision Trees: Classification Trees: Decision trees are used to classify data into categories or classes. In HR, they can be employed to predict outcomes like employee turnover or performance. The tree structure is built by splitting the data into subsets based on the most significant predictor variables. Regression Trees: Regression trees predict a continuous outcome. They are useful for forecasting metrics such as employee performance ratings. Each branch of the tree provides a prediction for the outcome variable based on the values of the predictor variables. Random Forests: Random forests are an ensemble learning technique that builds multiple decision trees and combines their predictions to improve accuracy and reduce overfitting. They are valuable in HR for predicting outcomes like turnover and performance, often outperforming individual decision trees. Gradient Boosting: Gradient boosting algorithms, like XGBoost and LightGBM, are widely used for predictive modeling in HR. These techniques iteratively improve the model's predictions by combining the results of multiple weak learners. They are effective for predicting turnover, identifying top performers, and other HR-related outcomes. Neural Networks: Artificial neural networks are part of deep learning and can be used in HR to predict complex relationships within HR data. They are particularly useful when dealing with large datasets and can be applied to a variety of HR prediction tasks, including employee performance and retention. Survival Analysis: Survival analysis is a statistical technique used in HR to model time-to- event data, such as employee turnover. It accounts for censored data (employees who have not yet experienced the event) and provides insights into how long employees are likely to stay with the company. 6 Fundamentals of HR Analytics Time Series Analysis: Time series analysis is applied when HR data is collected over time, and the objective is to make predictions about future workforce-related events. It can be used to forecast staffing needs, seasonal hiring patterns, or employee performance trends. Natural Language Processing (NLP): NLP techniques can be employed to analyze text data from sources like employee feedback, performance reviews, and surveys. By extracting insights from text, NLP can help predict employee sentiment, engagement, and performance. How These Techniques are Employed in HR: Employee Turnover Prediction: Logistic regression, decision trees, random forests, and gradient boosting can be used to predict which employees are at risk of leaving the organization. HR can then take preventive measures to retain valuable talent. Employee Performance Prediction: Linear regression, regression trees, neural networks, and NLP can help forecast employee performance ratings. This information is valuable for personalized performance improvement plans and talent development. Talent Acquisition: Predictive modeling techniques are used to identify the most effective recruitment sources, estimate hiring needs, and predict the success of candidates during the selection process. Workforce Planning: Time series analysis, regression, and machine learning can help organizations forecast future staffing requirements and align the workforce with business goals. In HR analytics, the choice of predictive modeling technique depends on the specific problem and data available. A combination of techniques may also be used to create more accurate and robust predictive models. By applying these techniques, HR professionals can make data-driven decisions that lead to improved workforce management and organizational success. 4.3 Data Preparation for Predictive HR Analytics Effective data preparation is a critical step in the process of predictive modeling in HR analytics. High-quality, well-organized data is essential for accurate predictions. Here are the essential steps for data cleaning, preprocessing, and feature engineering to prepare HR data for predictive modeling: 7 UNIT 04: Predictive Analytics in HR Data Collection: Gather relevant HR data from various sources, which may include HRIS (Human Resource Information Systems), performance records, surveys, and external sources like job boards and social media. Data Exploration: Explore the dataset to understand its structure and the variables it contains. Identify the target variable (the one you want to predict) and potential predictor variables (features). Handling Missing Data: Identify and address missing data in the dataset. Missing values can affect the performance of predictive models. Common approaches to handling missing data include: Imputation: Replacing missing values with a specific value (e.g., mean, median, mode) or using advanced imputation techniques like regression or k-nearest neighbors. Deleting rows or columns with a high proportion of missing values if appropriate. Outlier Detection and Handling: Outliers can introduce noise into the data and affect model performance. Use statistical methods like the z-score, IQR (Interquartile Range), or visualization techniques like box plots to identify and handle outliers. Options for handling outliers include transformation, imputation, or, in some cases, excluding them. Data Encoding: Categorical variables need to be converted into numerical format for most predictive models. Common encoding methods include one-hot encoding (for nominal variables) and label encoding (for ordinal variables). Feature Scaling: Standardize or normalize numerical variables to ensure they have similar scales. This step is particularly important for models sensitive to the scale of input features, like support vector machines and k-nearest neighbors. Feature Engineering: Feature engineering involves creating new variables or modifying existing ones to better represent the relationships in the data. Some common techniques include: Creating interaction terms: Combining two or more variables to capture synergistic effects. Binning: Grouping continuous variables into bins or categories. Dimensionality reduction: Reducing the number of features using techniques like principal component analysis (PCA) or feature selection methods to avoid overfitting. 8 Fundamentals of HR Analytics Extracting information from text data using natural language processing (NLP) for sentiment analysis or keyword extraction. Data Splitting: Split the data into training and testing sets. The training set is used to build the predictive model, while the testing set is used to evaluate the model's performance. Common splits include 70-30 or 80-20 ratios. Handling Imbalanced Data: In HR scenarios such as turnover prediction, the data might be imbalanced, with more employees staying than leaving. Techniques like oversampling, undersampling, or using synthetic data generation (SMOTE) can help balance the dataset. Data Normalization: In cases where the data distribution is skewed, transformation techniques like log transformation or Box-Cox transformation can help make the data more normally distributed. Data Validation: Regularly validate and update your dataset to ensure it reflects the current state of HR data. Outdated data can lead to inaccurate predictions. Model-Dependent Preprocessing: Depending on the chosen predictive modeling technique, there may be specific preprocessing steps. For instance, neural networks may require additional data scaling or normalization. Documentation: Maintain comprehensive documentation of all data preprocessing steps. This documentation is crucial for reproducibility and troubleshooting. Data preparation is often an iterative process, and data scientists and HR professionals may need to revisit these steps as they fine-tune models or work with new data. A well-prepared dataset is the foundation for accurate and reliable predictions in HR analytics. 4.4 HR Metrics for Predictive Analytics To effectively apply predictive analytics in HR, it's essential to identify the key HR metrics and data sources that serve as inputs for predictive modeling. These metrics and data sources provide the foundation for making data-driven predictions and decisions in various HR functions. Here are some of the key HR metrics and the associated data sources: Employee Turnover Rate: Metric: Employee turnover rate is a fundamental HR metric that measures the percentage of employees who leave the organization over a specific period. 9 UNIT 04: Predictive Analytics in HR Data Sources: HRIS (Human Resource Information System), payroll records, and exit interviews. Employee Engagement Scores: Metric: Employee engagement scores reflect the level of employee commitment, satisfaction, and emotional connection to the organization. It is often measured through surveys and feedback mechanisms. Data Sources: Employee engagement surveys, pulse surveys, feedback from performance reviews, and other employee feedback mechanisms. Recruitment Efficiency Metrics: Metrics: Recruitment efficiency metrics help assess the effectiveness of the talent acquisition process and include metrics like time-to-fill, cost- per-hire, and quality-of-hire. Data Sources: HRIS, applicant tracking systems (ATS), and recruitment software. Performance Metrics: Metrics: Performance metrics encompass various aspects of employee performance, including key performance indicators (KPIs), goals achievement, and performance ratings. Data Sources: Performance management systems, 360-degree feedback, and performance appraisals. Absenteeism and Attendance Metrics: Metrics: These metrics help track employee attendance, absenteeism, and related patterns. Data Sources: Time and attendance records, HRIS, and employee time- tracking systems. Compensation and Benefits Metrics: Metrics: Compensation metrics include measures of total compensation, salary increases, and benefits utilization. These metrics provide insights into the organization's rewards and recognition practices. Data Sources: Payroll records, compensation and benefits systems, and HRIS. Training and Development Metrics: Metrics: These metrics evaluate the effectiveness of training and development programs and may include training completion rates, skills development, and employee training satisfaction. Data Sources: Learning management systems (LMS), training records, and HRIS. Diversity and Inclusion Metrics: Metrics: Diversity and inclusion metrics help track diversity demographics, inclusion scores, and the effectiveness of diversity initiatives. Data Sources: HRIS, diversity and inclusion surveys, and employee demographic data. 10 Fundamentals of HR Analytics Employee Satisfaction and Net Promoter Score (NPS): Metrics: Employee satisfaction and NPS metrics assess overall employee satisfaction and the likelihood that employees would recommend the organization to others. Data Sources: Employee surveys, NPS surveys, and feedback channels. Succession Planning Metrics: Metrics: These metrics evaluate the readiness and availability of talent to fill key leadership and critical roles within the organization. Data Sources: Talent management systems, employee profiles, and performance data. Workforce Productivity Metrics: Metrics: Workforce productivity metrics measure the output or value generated by the workforce in relation to the resources and time invested. Data Sources: HRIS, project management tools, and productivity software. Time-to-Productivity for New Hires: Metric: This metric measures how long it takes for new hires to become fully productive in their roles, which is crucial for assessing the onboarding process. Data Sources: Onboarding systems, performance data, and new hire surveys. Workforce Demographics: Metrics: Workforce demographics include data on age, gender, ethnicity, and other characteristics that contribute to diversity and inclusion metrics. Data Sources: HRIS, diversity surveys, and demographic self- identification. These HR metrics, when collected and analyzed from the relevant data sources, provide valuable insights that can be used as inputs for predictive analytics. By understanding historical trends and relationships within these metrics, HR professionals can make predictions and data-driven decisions related to talent acquisition, employee engagement, retention, performance management, and other critical HR functions. 4.5 Challenges and Ethical Considerations in Predictive HR Analytics Predictive HR analytics offers substantial benefits, but it also comes with a set of challenges and ethical considerations that organizations and HR professionals need to address. Here are some of the primary challenges and ethical dilemmas associated with predictive HR analytics: 11 UNIT 04: Predictive Analytics in HR Data Quality and Availability: Challenge: Data used for predictive HR analytics may be incomplete, inaccurate, or outdated, which can lead to erroneous predictions. Ethical Consideration: Relying on flawed data may result in unfair or discriminatory decisions affecting employees. Bias in Data and Models: Challenge: Data used in predictive models can contain biases, reflecting historical discrimination in hiring, promotion, and performance evaluations. Ethical Consideration: Using biased data or building models that perpetuate bias can lead to unfair and discriminatory outcomes, negatively impacting underrepresented groups. Privacy Concerns: Challenge: Predictive HR analytics often involve collecting and analyzing personal and sensitive employee data. Ethical Consideration: Balancing the need for data with employees' privacy rights is essential to maintain trust and comply with privacy regulations (e.g., GDPR, CCPA). Model Transparency and Interpretability: Challenge: Some predictive models, particularly complex machine learning algorithms, can lack transparency, making it challenging to understand how they arrive at their predictions. Ethical Consideration: Lack of transparency can lead to distrust among employees, who may question the fairness and objectivity of the decisions made by these models. Fairness and Discrimination: Challenge: Predictive models may inadvertently reinforce systemic biases against certain groups or individuals. Ethical Consideration: It's critical to ensure that models are designed to be fair and unbiased, with appropriate safeguards in place to prevent discrimination. Employee Trust and Morale: Challenge: If employees perceive predictive analytics as invasive or as a means to micromanage them, it can erode trust and reduce morale. Ethical Consideration: HR analytics should be used to benefit employees and the organization, not as a tool for excessive surveillance or control. Security and Data Protection: Challenge: The more data is collected and analyzed, the greater the risk of data breaches or misuse. Ethical Consideration: Organizations must invest in robust data security measures and ensure data is handled in compliance with data protection laws. Consent and Informed Decision-Making: 12 Fundamentals of HR Analytics Challenge: Ensuring that employees provide informed consent for data collection and analysis can be challenging. Ethical Consideration: Organizations should be transparent with employees about the purpose and implications of data analytics and seek their consent when appropriate. Regulatory Compliance: Challenge: The legal landscape for HR analytics is evolving, with new regulations and legal requirements. Ethical Consideration: Organizations must stay informed about and comply with applicable laws and regulations, ensuring that their analytics practices are lawful and ethical. Accountability and Responsibility: - Challenge: Determining who is responsible for the decisions made by predictive HR analytics models can be complex. - Ethical Consideration: Clearly defining accountability and responsibility within the organization is necessary to address potential issues and biases. Addressing these challenges and ethical considerations in predictive HR analytics requires a comprehensive approach. Organizations should establish ethical guidelines, invest in employee training, conduct regular audits of models, and engage with ethicists and experts to ensure that HR analytics practices are not only effective but also fair, transparent, and respectful of individual rights and privacy. 4.6 Improving Decision-Making with Predictive Analytics in HR Predictive analytics in HR empowers organizations to make more informed and data- driven decisions, resulting in enhanced workforce management and more effective HR strategies. Here's how the insights generated through predictive analytics in HR can lead to improved decision-making: Talent Acquisition and Recruitment: Predictive analytics can help HR professionals identify the most effective recruitment channels, select the best-fit candidates, and estimate future hiring needs. This information allows organizations to optimize their recruitment strategies, reduce time-to-fill positions, and make cost-effective hiring decisions. Employee Retention and Turnover: Predictive models can forecast which employees are at risk of leaving the organization. HR can then take proactive measures, such as targeted retention initiatives and improved management practices, to reduce turnover and retain valuable talent. 13 UNIT 04: Predictive Analytics in HR Performance Management: Predictive analytics can identify factors that impact employee performance, allowing HR to develop personalized performance improvement plans and allocate resources effectively. This leads to a more engaged and productive workforce. Workforce Planning: By forecasting future staffing needs based on historical data and market trends, HR can align the workforce with strategic goals. This results in cost-effective and efficient workforce planning, ensuring the right people are in the right roles at the right time. Diversity and Inclusion: Predictive modeling can help organizations track and improve diversity and inclusion initiatives by identifying areas where intervention is needed. HR can use data to create more inclusive workplaces and ensure diverse talent is retained and promoted. Employee Engagement: Predictive analytics can reveal drivers of employee engagement and satisfaction. HR can use this information to tailor engagement initiatives, improve workplace culture, and boost employee morale, resulting in a more engaged and committed workforce. Compensation and Benefits Optimization: Predictive models can assist HR in determining appropriate compensation and benefits packages to attract and retain talent. This leads to a balanced approach to reward management and cost savings. Training and Development: Predictive analytics can identify which employees are most likely to benefit from specific training programs. HR can allocate resources more efficiently, resulting in a better-trained and skilled workforce. Succession Planning: Predictive modeling helps HR assess the readiness and availability of talent to fill key leadership and critical roles. Organizations can groom high-potential employees and ensure a smooth transition when key positions become vacant. Data-Driven Decision Culture: - Implementing predictive analytics fosters a data- driven decision culture within the organization. HR professionals and business leaders learn to rely on data, not intuition, to make critical workforce management decisions. Risk Mitigation: - Predictive analytics can help organizations identify and mitigate risks related to compliance, employee misconduct, and legal issues. This proactive approach minimizes potential HR crises and legal liabilities. 14 Fundamentals of HR Analytics Continuous Improvement: - Regularly updating predictive models and analytics practices allows organizations to continuously improve their HR strategies. As they collect more data and refine their models, they become more adept at predicting and addressing workforce challenges. Resource Allocation: - Predictive analytics helps HR allocate resources more effectively, whether for recruitment, training, benefits, or employee development. This results in cost savings and optimized resource allocation. In summary, predictive analytics in HR provides valuable insights that enable organizations to make data-driven decisions across all aspects of workforce management. By harnessing the power of data, HR professionals can optimize talent acquisition, retention, performance, diversity, and other critical HR functions, ultimately leading to a more engaged and productive workforce and more successful HR strategies. 4.7 Conclusion Predictive modeling in HR analytics is a transformative approach, leveraging data- driven insights to optimize talent management. Techniques like regression, decision trees, and machine learning enhance predictions for turnover, performance, and more. Data preparation ensures data quality, while HR metrics drive evidence-based decision-making. Ethical challenges, such as bias and privacy, must be addressed. Ultimately, predictive analytics empowers HR to make informed choices, leading to better workforce management and strategies. 4.8 Glossary Predictive Modeling: The process of using statistical and machine learning techniques to make predictions about future HR-related events and outcomes. Regression Analysis: A predictive modeling technique that is used to understand the relationship between a dependent variable (e.g., employee performance) and one or more independent variables. Decision Trees: A predictive modeling technique that uses a tree-like structure to make decisions and classify data into different categories. Feature Engineering: The process of selecting, creating, or transforming variables (features) from the data to improve predictive model performance. Data Preprocessing: The steps taken to clean, format, and organize data before it is used in predictive modeling. 15 UNIT 04: Predictive Analytics in HR Employee Turnover: The rate at which employees leave an organization, often measured as a percentage of the total workforce. Bias in Predictive Analytics: The presence of systematic errors or inaccuracies in predictive models that can result in unfair or discriminatory outcomes. Privacy Concerns: Worries related to the ethical collection and use of employee data, ensuring data protection and respect for privacy rights. Feature Scaling: The process of standardizing or normalizing numerical variables to ensure they have similar scales. Employee Engagement: A measure of an employee's emotional connection to their job and the organization, reflecting their commitment and satisfaction. 4.9 Self-Assessment Questions Answers for Self- Assessment questions B. Descriptive Questions: 1. How can organizations overcome bias in predictive HR analytics to ensure fairness in decision-making? 2. What are the key HR metrics that serve as inputs for predictive analytics, and how do they impact talent management? 3. How can HR professionals address data privacy concerns while implementing predictive analytics in their practices? 4. What role do transparency and interpretability of models play in the ethical use of predictive analytics in HR? 5. How can predictive HR analytics be applied to improve diversity and inclusion within an organization? 4.10 Post Unit Reading Material Society for Human Resource Management (SHRM): www.shrm.org HR Technologist: www.hrtechnologist.com 4.11 Discussion Forum "The Role of Predictive Analytics in Enhancing Employee Engagement and Retention Strategies." "Ethical Considerations in HR Analytics: Mitigating Bias and Ensuring Data Privacy in Workforce Predictive Modeling." 16 Fundamentals of HR Analytics 17