Basic Data Science - Symbiosis Skills & Professional University
Document Details
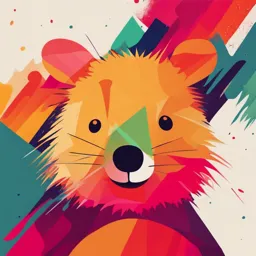
Uploaded by NicerLaplace
Symbiosis Skills & Professional University
Dr. Nagnath Biradar
Tags
Related
- Introduction to Clustering PDF
- Lecture 6: Introduction to Graphs PDF
- Introduction to Computer Systems and Applications in Chemistry PDF
- Big Data Analysen in Anwendung unter Verwendung der Programmiersprache R PDF
- Taller de Data Analysis - Academia Desafío Latam PDF
- Introducción al Análisis de Datos (2.ª Ed.) - Sanz y Torres PDF
Summary
This document is a set of lecture notes on the introduction to data science, offering a fundamental understanding of data science, encompassing its core principles, and an overview of data analysis methods.
Full Transcript
Subject: Basic Data Science Course Code:DS101 Dr.Nagnath Biradar Sr. Assistant Professor Data Science Department Introduction of Data Science …. Introduction ,data analysis, data analytics, Venn diagram and Pipeline, Roles and Team in Data Science, Big Data, Programming, Statistic...
Subject: Basic Data Science Course Code:DS101 Dr.Nagnath Biradar Sr. Assistant Professor Data Science Department Introduction of Data Science …. Introduction ,data analysis, data analytics, Venn diagram and Pipeline, Roles and Team in Data Science, Big Data, Programming, Statistics, Ethics in Data Science 1.1 What is data Science? 1.2 What is data analysis? 1.3 Importance of data science 1.4 Roles and team in data Science 1.5 Importance of mathematics, statistics, computer science and IT in data science. 1.6 Ethics in data science 1.7 What is Big data Introduction of Data Science …. What is data Science? Understand what is data analysis Understand the Importance of data science Learn the roles and team in data Science Understand the Importance of domains like mathematics, statistics, computer science and IT in data science. Understand the importance of ethics in data science Understand what ethics should be followed in Data science. Understand the concept of Big data Introduction of Data Science …. What is data Science? Data science : Data science is a multidisciplinary field that uses scientific methods, processes, algorithms, and systems to extract knowledge and insights from structured and unstructured data. It combines statistics, mathematics, computer science, and domain expertise to interpret data and solve complex analytical problems. Data analysis : Data analysis involves examining raw data with the purpose of drawing conclusions about the information it contains. It includes cleaning, transforming, and modeling data to uncover patterns, trends, and insights that inform decision-making or solve problems within a specific context or domain. Introduction of Data Science …. Importance of data science: Data science is crucial because it enables organizations to make data-driven decisions, optimize processes, predict outcomes, and gain competitive advantages. It helps uncover insights from large volumes of data that traditional methods may overlook, leading to innovation, efficiency improvements, and better understanding of customer behavior and market trends. Roles and team in data Science : In data science, roles typically include data scientists who analyze data, build models, and derive insights; data engineers who manage and process large datasets; and domain experts who provide context and interpret results. Teams collaborate to tackle complex problems, innovate with data-driven solutions, and drive business outcomes through informed decision-making. Introduction of Data Science …. Data Science Relies Heavily On Mathematics, Statistics, Computer Science, And IT, Each Playing A Crucial Role: These Mathematics, Statistics, Computer Science, And IT disciplines form are the backbone of data science, enabling professionals to extract meaningful insights, build predictive models, and solve complex problems using data. Mathematics: Provides the foundational theories and techniques for data analysis, including linear algebra, calculus, and discrete mathematics. These mathematical tools are essential for developing algorithms, optimizing models, and understanding complex patterns in data. Statistics: Essential for analysing and interpreting data. Statistical methods help in summarizing data, estimating probabilities, testing hypotheses, and making inferences. Techniques such as regression analysis, hypothesis testing, and probability distributions are central to making data-driven decisions. Introduction of Data Science …. Computer Science: Supplies the algorithms and data structures needed to handle and process large volumes of data efficiently. Skills in computer science are crucial for developing software, implementing machine learning algorithms, and optimizing performance in data processing tasks. IT (Information Technology): Provides the infrastructure and tools required for storing, retrieving, and managing data. IT encompasses database management, cloud computing, and data security, which are vital for ensuring that data is accessible, secure, and efficiently managed. ETHICS IN DATA SCIENCE Ethics is based on well-founded standards of right and wrong that prescribe what humans ought to do, usually in terms of rights, obligations, benefits to society, fairness, or specific virtues. Ethics in data science encompasses principles and guidelines that govern the responsible and ethical use of data throughout its lifecycle, from collection to analysis and application. Here are some key aspects: In data science, several key ethical principles ETHICS IN DATA SCIENCE Ethics in data science encompasses principles and guidelines that govern the responsible and ethical use of data throughout its lifecycle, from collection to analysis and application. In Data Science, Several Key Ethical Principles Should Be Followed Transparency: Ensure transparency in data collection, analysis methods, and decision-making processes to build trust and accountability(Being transparent about data practices, methodologies used for analysis, and potential biases in data. Data scientists should be accountable for the implications of their work). Fairness: Strive to mitigate biases in data and algorithms to ensure fair treatment and equal opportunities for all individuals(Mitigating bias in data collection and algorithms to ensure fair outcomes for all groups. This involves recognizing and addressing biases that may be present in datasets or models). Privacy: Respect individuals' privacy rights by anonymizing data, obtaining consent for data use, and implementing secure data handling practices(Ensuring that data is collected with consent and that individuals' privacy is protected. This includes anonymizing data where possible and handling sensitive information appropriately) Accountability: Take responsibility for the outcomes of data-driven decisions and algorithms, and be prepared to address any unintended consequences. Societal Impact: Consider the broader societal implications of data science projects and prioritize ethical considerations over purely technical goals. Ethics In Data Science….. Data Integrity and Quality: Maintaining the accuracy, reliability, and integrity of data throughout its lifecycle. This includes addressing issues such as data errors, completeness, and ensuring data is used in context-appropriate ways( means Maintain the accuracy, completeness, and reliability of data throughout its lifecycle to ensure the validity of analyses and interpretations). Professionalism: Uphold ethical standards and codes of conduct within the data science community, and continuously educate oneself on emerging ethical challenges and best practices. Impact on Society: Considering the broader impact of data science projects on individuals, communities, and society as a whole. This involves evaluating potential risks and benefits and striving for positive societal outcomes. Regulatory Compliance: Adhering to legal and regulatory requirements related to data protection, such as GDPR(EU general data protection regulation), CCPA(Privacy. California Consumer Privacy Act), or industry-specific regulations. Ethical Decision-Making: Applying ethical frameworks and principles to guide decision-making in data science, especially in complex situations where ethical dilemmas may arise. Professional Standards: Upholding professional standards and ethical codes of conduct set forth by organizations like the ACM(The Association for Computing Machinery) or IEEE(Institute of Electrical and Electronics Engineers). Ethics In Data Science….. Protects Privacy: It ensures that personal information is kept safe and used only with permission, respecting people's privacy. Builds Trust: When data practices are transparent and ethical, people trust the organizations using their data, which is important for long-term relationships. Prevents Bias: Ethical data practices help identify and reduce biases in data, leading to fairer and more accurate results. Maintains Accuracy: It ensures that data is accurate and used correctly, which helps make reliable decisions. Ensures Accountability: It holds data scientists accountable for their work, making sure they act responsibly and ethically. Complies with Laws: Ethical practices help organizations follow legal requirements for data protection, avoiding legal issues. Reduces Risks: It helps identify potential risks and address them before they cause problems, protecting individuals and society. Promotes Positive Impact: It ensures that data science projects benefit society and do not cause harm. Ethics In Data Science …. Ethics in data science is critical not only for safeguarding individual rights and societal values but also for ensuring the long-term trust and sustainability of data-driven technologies in various fields. The ethics helps trust, minimize risks, and maximize the positive impact of data science on individuals, organizations, and society as a whole. The importance of ethics in data science lies in its role in protecting individual rights, ensuring fairness, maintaining data quality, and fostering trust and accountability. Ethical practices not only safeguard against potential misuse but also contribute to the responsible and beneficial use of data, ultimately supporting a positive impact on society. Ethics in data science is important because it protects people’s rights, ensures fairness, builds trust, and promotes responsible use of data. Who use data? Who use data? Data analysts: A data analyst reviews data to identify key insights into a business's customers and ways the data can be used to solve problems. They also communicate this information to company leadership and other stakeholders Data scientist: Data scientists are people who use their statistical, programming and industry domain expertise to transform data into insights. Put another way, data scientists are part mathematician, part computer scientist and part trendspotter. They use their IT smarts to help companies calculate risk and drive positive results Statisticians: Statisticians develop or apply mathematical or statistical theory and methods to collect, organize, interpret, and summarize numerical data to provide usable information. They may specialize in fields such as bio- statistics, agricultural statistics, business statistics, or economic statistics Market Researcher: A Market Researcher collects and studies information about customers, sales trends, products, and services to develop future marketing plans. They may also use the data gathered to write reports that are used to direct business plans. Business analysts: Business analysts assess how organisations are performing and help them improve their processes and systems. They conduct research and analysis in order to come up with solutions to business problems and help to introduce these solutions to businesses and their clients Why data is important Why data is important of Feed Back system? Data analytics and its types Professor James Evans : Defined Data Analytics Data analytics and its types Data Analysis? Data analysis Is the process of examining, transforming and arranging raw data in a specific way to generate useful information from it. So data analysis allows for the evaluation of data through analytical and logical reasoning to lead to some sort of outcome or conclusion in some context. Data analysis is a multi-faceted process that involves a number of steps approaches and diverse techniques Data analytics and its types CONTRARY OF DATA ANALYSIS AND DATA ANALYTICS The analysis is data analysis and data analytics. When you say analysis when you say data analysis it is something about what has happened in the past. So we will explain why that has happened? We will explain how it has happened? We can explain why it has happened? For example, when we say data analysis that is nothing about studying about what has happened it is like kind of a post-mortem analysis. What has happened in the past? The contrary the analytics is studying about what will happen in future and with the help of analytics. We can predict explore possible potential future events. Data Analysis Vs Data Anaytics Data analysis? Data Analytic? Difference between Data Analytics and Data Analysis Data analytics and its types There Are Four Major Types Of Data Analytics: 1. Descriptive (business intelligence and data mining) 2.Diagnostic analytics 3.Predictive (forecasting) 4.Prescriptive (optimization and simulation) Data analytics and its types Data analytics and its types Descriptive Analytics: Descriptive analytics is the process of using current and historical data to identify trends and relationships. It’s sometimes called the simplest form of data analysis because it describes trends and relationships but doesn’t dig deeper. Descriptive analytics is a statistical interpretation used to analyze historical data to identify patterns and relationships. Summarizes past data (e.g., monthly sales reports). Data analytics and its types Let’s look at the Example of Descriptive Analytics: Imagine a hospital analyzing patient records. They might calculate average wait times in the emergency room, categorize the most frequent diagnoses, or track year-over-year trends in admissions. By summarizing data and using visuals like bar charts or line graphs, they can identify patterns and understand what’s happening within the hospital. This descriptive analysis allows them to focus on areas for improvement, like reducing wait times or allocating resources based on patient needs. Data analytics and its types Data analytics and its types Steps for Descriptive Analytics Work: 1. Data Collection: Collecting useful information is the initial stage in the descriptive analytics process. By using multiple resources such as databases, spreadsheets, and other data repositories. 2. Cleaning the Data and Preprocessing: The obtained data usually needs to be cleaned and preprocessed before analysis can start. This includes converting data into a uniform structure, standardizing formats, and handling missing or incorrect values. 3. Data analysis: It provides an understanding of the structure and features of the dataset. Here EDA (exploratory data analysis) methods helps to find the patterns, trends, and possible outliers in the data. These methods include making histograms, scatter plots, and summary statistics. Data analytics and its types Steps for Descriptive Analytics Work: 4. Compilation and Summary: The goal of descriptive analytics is to offer an overview of the data at a high level. To get important metrics and statistics, such as mean, median, mode, range, and standard deviation, this frequently requires combining the data. 5. Visualization: In descriptive analytics, visualizations are extremely useful tools. It helps us to communicate complex information with a variety of charts, graphs, and other visual representations are employed. Data patterns and trends can be highlighted with the use of visualization, which also makes it easier to convey insights to a wide range of audiences. 6. Fiction Creation: Descriptive analytics can include the creation of descriptions that offer a logical and contextualized explanation of the data, in addition to visuals. When communicating findings to those in the audience who might not be familiar with the complexities of the data, this can be especially helpful. Data analytics and its types Steps for Descriptive Analytics Work: 7. Interpretation: To obtain significant knowledge, analysts interpret the outcomes of descriptive analytics. This involves knowing the effects of the trends and patterns seen in the data. While interpretation provides the foundation for more in-depth analyses that investigate “why” and “what might happen in the future,” descriptive analytics concentrates on the “what happened” topic. 8. Testing Actively: The process of descriptive analytics is not one-time. Organizations continually repeat the descriptive analytics when new data becomes available in order to keep informed about the latest developments and patterns. This way, people making decisions get the newest information. Data analytics and its types Tabular data Data analytics and its types Data analytics and its types Applications of descriptive analytics include: Business Intelligence: Companies use descriptive analytics to track key performance indicators (KPIs) and measure business performance. Market Research: Analyzing consumer data to understand market trends and customer preferences. Healthcare: Monitoring patient data to track health outcomes and identify areas for improvement. Finance: Examining financial statements to assess the financial health of an organization. Data analytics and its types Diagnostic Analytics: Diagnostic analytics is a branch of data analytics that focuses on examining past data in order to identify the causes of specific events. It involves analyzing data to understand why something happened. Explains reasons behind past outcomes (e.g., sales decline analysis). Diagnostic analytics goes a step beyond descriptive analytics by answering the question, "Why did it happen?" It involves identifying the causes of past events and understanding the relationships between different variables. This type of analysis helps organizations pinpoint the reasons behind successes or failures. It uses techniques such as: 1.Data Discovery, 2.Data Mining , 3. Correlations Data analytics and its types Diagnostic analytics often involves the use of advanced analytical techniques and tools, such as: Regression Analysis: Understanding the relationship between dependent and independent variables. Time Series Analysis: Analyzing data points collected or recorded at specific time intervals to identify trends and seasonal patterns. Machine Learning Models: Leveraging algorithms to uncover complex patterns and make sense of large datasets. Data analytics and its types Predictive Analytics: Predictive analytics turn the data into valuable, actionable information. predictive analytics uses data to determine the probable outcome of an event or a likelihood of a situation occurring. Forecasts future events (e.g., sales forecasting). Data analytics and its types Predictive Analytics: Predictive analytics uses statistical algorithms and machine learning techniques to identify the likelihood of future outcomes based on historical data. It answers the question, "What could happen?" and helps organizations make proactive, data-driven decisions. It totally deals with future predictions which has to be taken. Deals with question: What will happen ? Generally with the help of Python or R Programming we do Predictive analysis. Data analytics and its types Applications: Marketing: Predicting customer behavior, such as response to campaigns and churn rates. Finance: Credit scoring, fraud detection, and risk assessment. Healthcare: Predicting patient outcomes and disease outbreaks. Retail: Inventory management and demand forecasting. Manufacturing: Predictive maintenance and quality control. Data analytics and its types Prescriptive Analytics: Recommends actions to achieve specific outcomes (e.g., supply chain optimization). Descriptive and Predictive Analytics = Prescriptive Analytics. Why data analytics is important Reducing inefficiencies and streamlining operations Driving revenue growth Enhancing decision-making Lowering operational expenses Improving customer experience Why data analytics is important Data Analytics serves as a powerful catalyst for business growth and performance optimization. By transforming raw data into actionable insights, it empowers businesses to make informed decisions, bolster risk management strategies, and enhance customer experiences. Informed Decision-Making Improved Efficiency and Productivity Enhanced Customer Experience Cost Reduction Performance Measurement Innovation Future Scope of Data Analytics Data Science Process… Data Analytics, Data Scientist & Business Analysts Data analysts: A data analyst reviews data to identify key insights into a business's customers and ways the data can be used to solve problems. They also communicate this information to company leadership and other stakeholders Data scientist: Data scientists are people who use their statistical, programming and industry domain expertise to transform data into insights. Put another way, data scientists are part mathematician, part computer scientist and part trendspotter. They use their IT smarts to help companies calculate risk and drive positive results Business analysts: Business analysts assess how organizations are performing and help them improve their processes and systems. They conduct research and analysis in order to come up with solutions to business problems and help to introduce these solutions to businesses and their clients Responsibilities Data Analyst Roles and Responsibilities: Data Analyst 1. Data Cleaning and Preparation: Data analysts spend a significant amount of time cleaning and organizing data to ensure it is accurate and ready for analysis. 2. Exploratory Data Analysis (EDA): They perform initial investigations on data to discover patterns, spot anomalies, test hypotheses, and check assumptions. 3. Reporting and Visualization: Data analysts create reports, dashboards, and visualizations to communicate findings to stakeholders. 4. Statistical Analysis: They apply basic statistical techniques to analyze data and provide actionable insights. 5. Business Intelligence: Analysts often work closely with business teams to provide insights that inform decision-making processes. 6. Ad-hoc Queries: They handle ad-hoc data requests from various departments to support business needs. Role and Responsibities Data Scientist Roles and Responsibilities: Data Scientist 1. Advanced Data Analysis: Data scientists conduct more complex data analysis, often involving advanced statistical methods and machine learning algorithms. 2. Model Building: They develop predictive models and algorithms to solve specific business problems. 3. Experimentation: Data scientists design and conduct experiments to test hypotheses and validate models. 4. Big Data Handling: They work with large datasets, often using distributed computing frameworks. 5. Programming and Scripting: Data scientists write and maintain complex scripts for data analysis and model deployment. 6. Research and Development: They stay updated with the latest advancements in data science and continuously improve models and methods. Difference between Data Analytics and Data Scientist Difference Data Analyst vs Data Scientist vs Data Engineer Data Analyst vs Data Scientist vs Data Engineer Difference between Data Analytics and Data Analysis Data Analyst vs Data Scientist vs Data Engineer Difference between Data Analytics and Data Analysis Data Analyst vs Data Scientist vs Data Engineer Difference between Data Analytics and Data Analysis Data Analyst vs Data Scientist vs Data Engineer Difference between Data Analytics and Data Analysis Data Analyst vs Data Scientist vs Data Engineer Difference between Data Analytics and Data Analysis Data Analyst vs Data Scientist vs Data Engineer Difference between Data Analytics and Data Analysis Data Analyst vs Data Scientist vs Data Engineer Difference between Data Analytics and Data Analysis Data Analyst vs Data Scientist vs Data Engineer Difference between Data Analytics and Data Analysis Data Analyst vs Data Scientist vs Data Engineer Difference between Data Analytics and Data Analysis Data Analyst vs Data Scientist vs Data Engineer skills Difference between Data Analytics and Data Analysis Data Analyst vs Data Scientist vs Data Engineer skills Data Scientist : The data scientist develops model like econometric and statistical for various problems like projection, classification, clustering, pattern analysis. Data Analytics : The data scientist supports the construction of the base of futuristic and various planned and continuing data analytics projects. Data Engineer : Data engineers process the real-time gathered data or stored data and create and maintain data pipelines that create interconnected ecosystem within an company. Difference between Data Analytics and Data Analysis Data Analyst vs Data Scientist vs Data Engineer Salary may differ Difference between Data Analytics and Data Analysis Data Analyst vs Data Scientist vs Data Engineer Difference between Data Analytics and Data Analysis Difference between Data Analytics and Data Analysis Data Analysts focus on interpreting data and generating insights. Data Engineers focus on the architecture and infrastructure that supports data collection, storage, and analysis. Data Scientists focus on creating models and algorithms to make predictions and automate decision-making. Difference between Data Analytics and Data Analysis www.sspu.ac.in