Forecasting Time Series PDF
Document Details
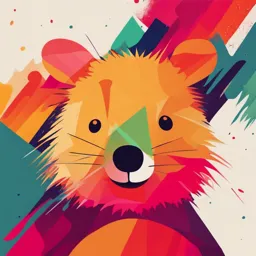
Uploaded by DistinguishedViolet6988
UNC Charlotte
2024
UNC Charlotte
Dr. Zhao
Tags
Related
Summary
This document is a presentation on forecasting time series. It covers topics such as forecasting models, business applications, and cautionary notes about predictions. The presentation was given in Fall 2024 at UNC Charlotte.
Full Transcript
FORECASTING TIME SERIES DSBA 6211 Dr. Zhao 11/27/2024 UNC Charlotte, Fall 2024 2 Overview 1. Introduction 2. Time Series Characteristics and Components 3. Forecasting Models 11/27/2024 UNC Charlotte, Fall 2024 3 I: INTRODUCTION 11/27/2024 UNC...
FORECASTING TIME SERIES DSBA 6211 Dr. Zhao 11/27/2024 UNC Charlotte, Fall 2024 2 Overview 1. Introduction 2. Time Series Characteristics and Components 3. Forecasting Models 11/27/2024 UNC Charlotte, Fall 2024 3 I: INTRODUCTION 11/27/2024 UNC Charlotte, Fall 2024 4 Forecasting Predictive modeling: Predict some outcome variables (e.g., buy/no buy, revenue) Forecasting Predict the future behavior of variables Account for the fact that data points taken over time may have an internal structure (such as autocorrelation, trend or seasonal variation) that should be accounted for. The data for forecasting is known as a time series. 11/27/2024 UNC Charlotte, Fall 2024 5 Forecasting The time series includes information that is gathered over time is for equally spaced time intervals uses the same measurement at each time enables the visualization of a pattern over time enables the quantification of such patterns enables forecasts to be made for future time points based on past behavior. 11/27/2024 UNC Charlotte, Fall 2024 6 Business Applications Inventory management Should you use this shelf space for more peanut butter or for more salsa next week? If you put the item on sale this week, will demand go down next week? Demand management What time of day produces peak server demand? Supply chain management When should you reorder raw materials? Pricing What are the pricing trends in the past quarter, compared to the previous three years? 11/27/2024 UNC Charlotte, Fall 2024 7 Caution “Prediction is very difficult, especially if it's about the future.” (Nils Bohr) A forecast is only as good as the information included in the forecast (past data) History is not a perfect predictor of the future (i.e.: there is no such thing as a perfect forecast) 11/27/2024 UNC Charlotte, Fall 2024 8 II: Time Series Characteristics and Components 11/27/2024 UNC Charlotte, Fall 2024 9 Statistical Time Series A statistical time series is an indexed set of numbers. The index can consist of dates or other numbers. Many business time series are equally spaced. A time series is equally spaced if any two consecutive indices have the same interval time difference. 11/27/2024 UNC Charlotte, Fall 2024 10 Equally Spaced Time Series Equally spaced time series Equally spaced time series with missing values 11/27/2024 UNC Charlotte, Fall 2024 11 The Universal Time Series Model Yt f (Tt , St , X t , Et ) TREND INPUT SEASONAL ERROR (Irregular) 11/27/2024 UNC Charlotte, Fall 2024 12 Airline Passengers 1994-1997 11/27/2024 UNC Charlotte, Fall 2024 13 Time Series Trend Yt f (Tt , St , X t , Et ) TREND INPUT SEASONAL ERROR (Irregular) 11/27/2024 UNC Charlotte, Fall 2024 14 Time Series Trend Trend usually refers to a deterministic function of time. Time series can be made of deterministic and stochastic components. A stochastic component is subject to random variation and can never be predicted perfectly except by chance. A deterministic component exhibits no random variation and can be predicted perfectly. Common deterministic trend functions include linear trend, curvilinear trend, logarithmic trend, and exponential trend. 11/27/2024 UNC Charlotte, Fall 2024 15 Deterministic Trend Models Linear Trend Yt 0 1t Y Time index t Parameters Time index Time series Quadratic Trend 2 Yt 0 1t 2t Y t 11/27/2024 UNC Charlotte, Fall 2024 16 Stochastic Trend Models Random Walk Yt Yt 1 Et Random Walk with Drift Yt Yt 1 Et 11/27/2024 UNC Charlotte, Fall 2024 17 Accommodating Stochastic Trend: Differencing A First Difference of the Random Walk Process Yt Yt 1 Et Yt Yt Yt 1 First Difference 11/27/2024 UNC Charlotte, Fall 2024 18 The Universal Time Series Model Yt f (Tt , St , X t , Et ) TREND INPUT ERROR (Irregular) SEASONAL 11/27/2024 UNC Charlotte, Fall 2024 19 Airline Passengers 1994–1997: Seasonal August February 11/27/2024 UNC Charlotte, Fall 2024 20 Seasonality The seasonal component of a time series represents the effects of seasonal variation. The foundation of seasonal variation is one or more of the cycles produced by the motion of the celestial bodies in the solar system, dominated by the earth circling the sun every year. Another influential activity is the moon circling the earth approximately every 28 days. The most general meaning of seasonality is a component that describes repetitive behavior at known seasonal periods. If the seasonal period is integer S, then seasonal factors are factors that repeat every S units of time. 11/27/2024 UNC Charlotte, Fall 2024 21 Accommodating Seasonal Components Trigonometric functions (sine waves) Seasonal dummy variables Seasonal differences (Box-Jenkins modeling) 11/27/2024 UNC Charlotte, Fall 2024 22 Dummy Variables A dummy variable is an indicator variable. To indicate a specific time point, a dummy variable takes one as the value for that time point. At all other time points, it takes zero as the value. 11/27/2024 UNC Charlotte, Fall 2024 23 Seasonal Dummy Variables For a time series with S seasons, there are S dummy variables, one for each season. Monthly Data: IJAN , IFEB ,…, IDEC Daily Data: ISUN , IMON ,…, ISAT Quarterly Data: IQ1 , IQ2 , IQ3 , IQ4 11/27/2024 UNC Charlotte, Fall 2024 24 Stochastic Seasonal Functions: Seasonal Differencing For seasonal data with period S, express the current value as a function that includes the value S time units in the past. Yt = Yt-S + TRENDt + IRREGULARt SYt = Yt Yt-S is called a difference of order S. Examples: Monthly: This January is a function of last January and so on. Weekly: This Sunday is a function of last Sunday and so on. 11/27/2024 UNC Charlotte, Fall 2024 25 The Universal Time Series Model Yt f (Tt , St , X t , Et ) TREND INPUT ERROR (Irregular) SEASONAL 11/27/2024 UNC Charlotte, Fall 2024 26 The Irregular Component The irregular component of a time series is what remains when trend, seasonal, and input effects are removed. Forecast error 11/27/2024 UNC Charlotte, Fall 2024 27 Additive Decomposition of the Airline Data T: Linear Trend S: Seasonal Average I: Irregular Component 11/27/2024 UNC Charlotte, Fall 2024 28 The Universal Time Series Model Yt f (Tt , St , X t , Et ) TREND INPUT ERROR (Irregular) SEASONAL 11/27/2024 UNC Charlotte, Fall 2024 29 Airline Passengers 1990-2004 Events 11/27/2024 UNC Charlotte, Fall 2024 30 Event Examples Retail promotions Advertising campaigns Negative article in a major publication Mergers and acquisitions Government legislated policy changes Organizational personnel and/or policy changes Strikes Scandal Injury, illness, or death of a key player (such as a CEO, CFO, or chief scientist) 11/27/2024 UNC Charlotte, Fall 2024 31 How Do Event Variables Improve Accuracy? Event variables enable the forecast model to accommodate discrete shifts, also called jumps or bangs, in time series data. Event variables in time series models are primarily intercept shifters. Intercept shifters are included in the model as explanatory variables and are based on columns of 0s and 1s in the data set. 11/27/2024 UNC Charlotte, Fall 2024 32 How Do Event Variables Improve Accuracy? The data is fit with a linear model: salest trend t Bias Data jump at Date = T* causes a large residual. 11/27/2024 UNC Charlotte, Fall 2024 33 How Do Event Variables Improve Accuracy? The linear model can be refined by modifying the intercept term as follows: sales t ( D) trend t D 1 if Date T * and 0 otherwse When Date = T*, the model’s intercept = ( + ), and when Date ≠ T*, the model’s intercept = . 11/27/2024 UNC Charlotte, Fall 2024 34 Event Variable Creation Demand History Resulting Dummy For Sales Column = D 01JAN2002 0 01FEB2002 0 … … 01JUL2003 0 BigStormEvent 01AUG2003 1 T* = '01AUG2003'd 01SEP2003 0 … … 01JUN2003 0 The temporary intercept shift is accomplished by adding a 0-1 or dummy column to the data. 11/27/2024 UNC Charlotte, Fall 2024 35 How Do Event Variables Improve Accuracy? The data is fit with a linear model and a pulse event variable. sales ( D ) trend t Less biased forecast The residual is much smaller. 11/27/2024 UNC Charlotte, Fall 2024 36 Event Variable Qualifiers The pulse event variable qualifies variation in the data as follows: There is a discrete shift in the data at Date = T*. Before and after Date = T*, the series is at its steady-state intercept and slope. That is, the series is impacted only for one time interval: Date = T*. How might the linear model be refined if the shift in the data resembles what is depicted on the next slide? 11/27/2024 UNC Charlotte, Fall 2024 37 How Do Event Variables Improve Accuracy? The data is fit with a linear model. salest trend A permanent Intercept shift at this date 11/27/2024 UNC Charlotte, Fall 2024 38 How Do Event Variables Improve Accuracy? The linear model can be refined by modifying the intercept term as follows: sales t ( D) trend t D 1 if Date T * and 0 otherwse When Date => T*, the model’s intercept = ( + ), and when Date < T*, the model’s intercept = . This is the same model specification as before, but the dummy column is changed as shown on the next slide. 11/27/2024 UNC Charlotte, Fall 2024 39 Event Variable Creation Demand History Resulting Dummy For Sales Column = D 01JAN2002 0 01FEB2002 0 … … 01JUL2003 0 New Law Enacted 01AUG2003 1 T* = '01AUG2003'd 01SEP2003 1 … … 01JUN2003 1 The permanent intercept shift is accomplished by adding a 0-1 or dummy column to the data. 11/27/2024 UNC Charlotte, Fall 2024 40 How Do Event Variables Improve Accuracy? The data is fit with a linear model and a step event variable. salest ( D) trend The permanent shift is accommodated in the model. 11/27/2024 UNC Charlotte, Fall 2024 41 III: FORECASTING MODELS 11/27/2024 UNC Charlotte, Fall 2024 42 Forecasting Models Regression-based model Trend & Seasonality Smoothing methods Moving average Simple exponential smoothing Autoregressive Integrated Moving Average Model (ARIMA) 11/27/2024 UNC Charlotte, Fall 2024 43 Model Performance Evaluation Forecast error: Scale-dependent error measures Cannot compare time series with different units MA: mean error RSMA: root mean squared error MAE: mean absolute error Percentage errors: Unit free & does not work if y is close to 0 MPE: mean percentage error MAPE: mean absolute percentage error Scaled error Scaling the errors based on the training MAE MASE: mean absolute scaled error 11/27/2024 UNC Charlotte, Fall 2024 44 Regression-Based Models 11/27/2024 UNC Charlotte, Fall 2024 45 Regression-Based Model Using suitable predictors to capture trend and/or seasonality Linear trend Quadratic trend: Addictive seasonality Create seasonality dummies Add dummies into the regression model 11/27/2024 UNC Charlotte, Fall 2024 46 Smoothing Methods 11/27/2024 UNC Charlotte, Fall 2024 47 Moving Average The moving average model uses the last t periods in order to predict demand in period t+1. There can be two types of moving average models Simple moving average Weighted moving average The moving average model assumption is that the most accurate prediction of future demand is a simple (linear) combination of past demand. 11/27/2024 UNC Charlotte, Fall 2024 48 Simple Moving Average Moving average through the forecast horizon n t: the current period n: the forecast horizon (how far back we look) 11/27/2024 UNC Charlotte, Fall 2024 49 Weighted Moving Average We may want to give more importance to some of the data wt-1 + … + wt-n = 1 t: the current period n: the forecast horizon (how far back we look) w: the importance (the weight) we give to each period Depending on the importance that we feel past data has Depending on known seasonality 11/27/2024 UNC Charlotte, Fall 2024 50 Moving Average Advantages of Moving Average Method Easily understood Easily computed Provides stable forecasts Disadvantages of Moving Average Method Requires saving lots of past data points Lags behind a trend Ignores complex relationships in data 11/27/2024 UNC Charlotte, Fall 2024 51 Exponential Smoothing Exponential Smoothing Models (ESM) The prediction of the future depends mostly on the latest forecast (or smoothed observation), and on the error for the latest forecast. Use less storage space for data Easy to understand The smoothing constant α expresses how much our forecast will react to observed differences… If α is low: there is little reaction to differences. If α is high: there is a lot of reaction to differences. 11/27/2024 UNC Charlotte, Fall 2024 52 Exponential Smoothing Expand the formula The method applies a set of exponentially declining weights to past data. The sum of the weights is exactly one. 11/27/2024 UNC Charlotte, Fall 2024 53 Exponential Smoothing 11/27/2024 UNC Charlotte, Fall 2024 54 MA vs. ESM ESM carries all past history MA eliminates “bad” data after N periods ESM only requires last forecast and last observation of “demand” to continue MA requires all N past data points to compute new forecast estimate 11/27/2024 UNC Charlotte, Fall 2024 55 A Simple Example: MA vs. ESM 11/27/2024 UNC Charlotte, Fall 2024 56 Kroger: Forecasting Sales Bottled spring water Month Bottles Jan 1,325 Feb 1,353 Mar 1,305 What Apr 1,275 will the sales be May 1,210 for July? Jun 1,195 Jul ? 11/27/2024 UNC Charlotte, Fall 2024 57 Moving Average 3-month simple moving average 5-month simple moving average 11/27/2024 UNC Charlotte, Fall 2024 58 Stability vs. Responsiveness 5-month average smoothes data more; 3-month average more responsive 1400 1350 1300 5-month 1250 MA forecast 1200 3-month 1150 MA forecast 1100 1050 1000 0 1 2 3 4 5 6 7 8 11/27/2024 UNC Charlotte, Fall 2024 59 6-month simple moving average because we used equal weights, a slight downward trend that actually exists is not observed… AJun + AMay + AApr + AMar + AFeb + AJan FJul = = 1,277 6 11/27/2024 UNC Charlotte, Fall 2024 60 Weighted Moving Average The higher the importance we give to recent data, the more we pick up the declining trend in our forecast. 6-month WMA WMA WMA SMA 40% / 60% 30% / 70% 20% / 80% July 1,277 Forecast 11/27/2024 UNC Charlotte, Fall 2024 61 Exponential Smoothing Main idea: The prediction of the future depends mostly on the latest forecast, and on the error for the latest forecast. 11/27/2024 UNC Charlotte, Fall 2024 62 Exponential Smoothing = 0.2 Month Actual Forecasted Jan 1,325 1,370 Feb 1,353 Mar 1,305 Apr 1,275 May 1,210 Jun 1,195 Jul 11/27/2024 UNC Charlotte, Fall 2024 63 Exponential Smoothing = 0.8 Month Actual Forecasted Jan 1,325 1,370 Feb 1,353 Mar 1,305 Apr 1,275 May 1,210 Jun 1,195 Jul 11/27/2024 UNC Charlotte, Fall 2024 64 Impact of the smoothing constant 1380 1360 1340 1320 Actual 1300 a = 0.2 1280 1260 a = 0.8 1240 1220 1200 0 1 2 3 4 5 6 7 11/27/2024 UNC Charlotte, Fall 2024 65 ARIMA 11/27/2024 UNC Charlotte, Fall 2024 66 Box-Jenkins (ARIMA) Models A set of of autoregressive integrated moving average (ARIMA) models. ARIMA models are regression models that use lagged values of the dependent variable and/or random disturbance term as explanatory variables. ARIMA models rely heavily on the autocorrelation pattern in the data This method applies to both non-seasonal and seasonal data. 11/27/2024 UNC Charlotte, Fall 2024 67 ARIMA Models A series which needs to be differenced to be made stationary is an “integrated” (I) series Lags of the series are called “autoregressive” (AR) terms Lags of the forecast errors are called “moving average” (MA) terms 11/27/2024 UNC Charlotte, Fall 2024 68 ARIMA Models ARIMA (p, d, q) Nonseasonal models p: the number of autoregressive terms d: the number of nonseasonal differences q: the number of moving-average terms May (or may not) include a constant term ARIMA (p, d, q)(P, D, Q) Seasonal ARIMA models 11/27/2024 UNC Charlotte, Fall 2024 69 Stationary Time Series A stationary time series is one whose properties does not depend on time Constant mean Constant variance (homoscedasticity) Constant covariance 11/27/2024 UNC Charlotte, Fall 2024 70 White Noise Zero mean Constant variance Independent from each other Diagnostic test: ACF 11/27/2024 UNC Charlotte, Fall 2024 71 Autoregressive Process Autoregressive model of order p (AR(p)) Forecasting depends on its p previous values Yˆt 1Yt 1 2Yt 2 pYt p t p 1 Yˆt 1Yt 1 t p 3 Yˆ Y Y t 1 t 1 2 t 2 3Yt 3 t 11/27/2024 UNC Charlotte, Fall 2024 72 Moving Average Process Moving Average model of order q (MA(q)) Forecasting depends on q previous random error terms or deviations Yˆt t 1 t 1 2 t 2 q t q , q 1 Yˆt t t 1 q 3 Yˆt t 1 t 1 2 t 2 3 t 3 11/27/2024 UNC Charlotte, Fall 2024 73 Differencing Often non-stationary series can be made stationary through differencing. Examples: 11/27/2024 UNC Charlotte, Fall 2024 74 Differencing The number of times that the original series must be differenced in order to achieve stationarity is called the order of integration, denoted by d. 10.0 12.0 -2.0 0.0 2.0 4.0 6.0 8.0 1990/Q2 11/27/2024 1991/Q1 1991/Q4 1992/Q3 1993/Q2 Differencing 1994/Q1 1994/Q4 1995/Q3 1996/Q2 1997/Q1 d t Yt Yt 4 1997/Q4 1998/Q3 UNC Charlotte, Fall 2024 U.S. Electricity Consumption 1999/Q2 2000/Q1 2000/Q4 75 2001/Q3 2002/Q2 2003/Q1 11/27/2024 UNC Charlotte, Fall 2024 76 ARIMA Models ARIMA(0,0,0): mean (constant) model ARIMA(1,0,0): first-order autoregressive model Yˆt 1Yt 1 t ARIMA(0,1,0): random walk Purely random series with mean and variance Yˆt Yt 1 t ARIMA(0,1,1): exponential smoothing Yˆt Yˆt 1 (1 )(Yt 1 Yˆt 1 ) 11/27/2024 UNC Charlotte, Fall 2024 77 ARIMA(0,1,1) ) )