Food Analysis Sampling Plan PDF
Document Details
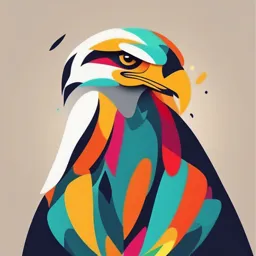
Uploaded by EntertainingMars
Prof. TS.Dr Wan Aida Wan Mustafa
Tags
Summary
This document discusses various aspects of food analysis, including sampling methods, data analysis techniques, and the importance of accurate sampling for reliable results. It details different sampling methods, including good and bad sampling techniques and the rationale for selecting suitable sampling methodologies.
Full Transcript
STKM 2372 FOOD ANALYSIS PROF. TS.DR WAN AI DA WAN MUSTAPHA 1 SAMPLING In a statistical study, sampling methods refer to how we select samples from the population to be in the study. If a sample isn't randomly selected, it...
STKM 2372 FOOD ANALYSIS PROF. TS.DR WAN AI DA WAN MUSTAPHA 1 SAMPLING In a statistical study, sampling methods refer to how we select samples from the population to be in the study. If a sample isn't randomly selected, it will probably be biased in some way and the data may not be representative of the population. 2 SAMPLING FOR FOOD ANALYSIS Sample should be representative of the whole material adequately samples from each of the populations BAD WAYS TO SAMPLE Convenience sample Voluntary response sample 3 GOOD WAYS TO SAMPLE Simple random Stratified random Cluster random Systematic random WHY IT IS NOT ALWAYS POSSIBLE TO INSPECT 100% OF THE PRODUCT? 1. Time consuming 2. Not economical (Expensive) 3. Fatigue 4. Spacious storage 4 Population Sampling Laboratory sample Sample Test sample Sampling : 1. Reduce cost 2. Reduce personnel 3. Reduce time so that information can be obtained quickly MANUAL VS. CONTINUOUS SAMPLING Manual sampling Continuous sampling 5 SAMPLING PLAN Sample size (representative) Locations (from which the sample should be selected- random/systematic) Methods (to collect and to preserve) Documentation procedures (traceability) NATURE OF POPULATION Finite or infinite Continuous or compartmentalized Homogenous or heterogeneous 6 FOOD STANDARDS AGENCY HTTPS://WWW.FOOD.GOV.UK/ FSA food sampling advice 1: https://www.youtube.com/watch? Sampling objectives v=Ki_wCxGlXbA FSA food sampling advice 2: https://www.youtube.com/watch? Sampling for analysis v=tD2KY2nyq1Y FSA food sampling advice 3: https://www.youtube.com/watch? Sampling for microbiological v=lKDqSB9myY8 examination SELECTING AN APPROPRIATE TECHNIQUE Accuracy Precision Reproducibility Speed Cost Good technique 7 FIG. 1 FIG. 2 FIG. 3 Precise Accurate Precise and accurate 8 GROUP WORK 1 Prepare a short video Choose one food product Do good sampling procedure Show to your friends Comments please PREPARATION OF LABORATORY SAMPLES 9 MAKING SAMPLES HOMOGENOUS Homogeneous vs. Heterogeneous Populations METHOD TO HOMOGENIZE SAMPLES Mechanical Enzymatic Chemical 10 SAMPLE PREPARATION/ PRETREATMENT REDUCING SAMPLE SIZE Part of homogenous sample ~laboratory sample Represent population Must be; Reliable Repeatable 11 Quartering procedure Suitable for dry samples. Care must be taken to ensure no moisture uptake occurs A R R A R A A R A R A R I II Step I : Accepted quadrants (A) are mixed and redivided. Rejected quadrants (R) are dismissed Step II : Repeat Step (I) till suitable sample size is obtained PREVENTING CHANGES IN SAMPLE Enzymatic inactivation Lipid protection Microbial growth and contamination Physical changes Freezing, drying, heat treatment, chemical 12 SAMPLE IDENTIFICATION Sample description Time sample was taken Location sample was taken Person who took the sample Method used to select the sample Must be Coded correctly DA TA A NA LYSI S A ND REPORTI NG 13 STATISTICAL ANALYSIS Why is statistics important? It is part of the quantitative approach to knowledge No study is perfect, there is always the chance for error Statistical estimation Every member of the population has the same chance of being selected in the sample Population Parameters Random sample estimation Statistics 14 POINT ESTIMATION AND INTERVAL ESTIMATION to understand the relationship between point estimation and interval estimation to calculate and interpret the confidence interval Statistical estimation Estimate Point estimate Interval estimate sample mean confidence interval for mean sample proportion confidence interval for proportion Point estimate is always within the interval estimate 15 OPTIONS FOR ANALYSING DATA Frequency Add up the responses and count how distribution many times each response was given. The number of times each variable occurs Mean Work out the average of the responses. A measure of central tendency Median Calculate the middle value. The value that appears in the centre of the data set. Mode Calculate the most common response. Mode is the value that appears most frequently. Range/standard See how widely spread the data were deviation Significance testing See how significant the findings were Variance See if the difference between two statistics is due to something more than sampling error Correlation or regression See if there is a relationship between analysis two statistics 16 Interval estimation Confidence interval (CI) provide us with a range of values that we believe, with a given level of confidence, contain a true value 95% - p>0.05 99% - p>0.01 DEFINITION OF P-VALUE. p-value = probability of observing a value more extreme that actual value observed, if the null hypothesis is true The smaller the p-value, the more unlikely the null hypothesis seems an explanation for the data P value > 0.05 not significant different 95% CI P value < 0.05 significantly different 17 MEASURE OF CENTRAL TENDENCY OF DATA Average value Most common Best experimental estimation n = total no of measurements xi = individually measured value DATA ANALYSIS AND REPORTING REPEATABILITY Table 1: Protein content of several types of chicken meat (n=4) Duplication Replication sample prot (%) A X1 B X2 C X3 D X4 18 Used to represent accuracy Comparison with true value True value is unknown:- use standard solution Absolute error Eabs = (xi – xtrue) –ve value shows measured value < true value +ve value shows measured value > true value MEASURE OF SPREAD OF DATA Show precision of a set of data How closely together repeated measurement are to each other Assumption: experimental measurements vary randomly and represent normal distribution ~ standard deviation = SD 19 Another parameter that is commonly used to provide an indication of the relative spread of the data around the mean is: Coefficient of variation (CV) CV = [SD/ min ] X 100 CV small – good data CV large – bad data VARIANCE Caused by a large difference between the statistics Calculated as follows: each deviation from the mean is squared then totalled, and then total is divided by the number of observations minus 1 _ ∑ (xi – x)2 Variance = n–1 20 20 Mean = 40.75 15 High Variance 10 Low Variance 5 0 0$- 20 $20 40 $40 60 $60 80 $80 Ch 14-41 SIGNIFICANCE TESTING For a sample of 400, a 95 per cent confidence level for 22 per cent is in the range +/– 4.1 Significance testing Brand D +/- 4.1 Brand C Brand B Overlap Brand A 0% 10% +/- 4.1 20% 30% 40% 21 CORRELATION ANALYSIS Correlation analysis is used to show the extent to which a change in one variable is associated with a change in another variable and how strong the relationship is: -1 0 +1 Correlation coefficient Negative correlation Positive correlation Age  Bad lecturer  Sports activity  Bad student  SOURCES OF ERROR ▪ Personal Errors (Blunters)  The wrong chemical reagent/ equipment  Volume and mass may have been recorded wrongly  Repeat a few times ▪ Random Errors  Determine SD ▪ Systematic Errors  Error produces results that consistently deviate from the true answer 22 STANDARD CURVES: REGRESSION ANALYSIS Necessary to prepare standard curves to determine unknown material A series of calibration experiments with a series of standard dilution Standard curve is plotted EXAMPLE ▪ Gradient/slope = a ▪ Intersept y (absorbance) = b ▪ The concentration of protein = (y-b)/a 23 CORRELATION COEFFICIENT How well the straight line fits the experimental data is expressed by the correlation coefficient r2 Value between 0 and 1 r2 = 1 the perfect fit Use spreadsheet programs REJECTING DATA When carrying out experimental analysis it will sometimes be observed that one of the measured value is very different from others. Assumption: it is wrong and can be rejected Use Q-test 24 XBAD = bad data XNEXT = the data after XBAD XHIGH = highest value of the data set XLOW = lowest value of the data set Calculated Q value > Q-test table =>reject Q-value for Number of data rejection observation (90% Eg: (n) confidence level) 3 0.94 20, 22, 25, 50, 21 4 0.76 Q = 0.84 > Q test table n=5 5 0.64 (0.64) 6 0.56 =>Reject 7 0.51 8 0.47 9 0.44 10 0.41 25 THANK YOU 26