SHS_09_Causality_confusion_interaction PDF
Document Details
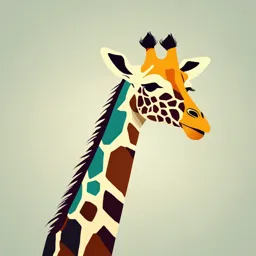
Uploaded by PraiseworthyHammeredDulcimer
UAB
Jose Barrera
Tags
Summary
This document details a B.Sc. degree in Applied Statistics covering causality, confusion, and interaction within health sciences. The author, Jose Barrera, discusses these statistical concepts, providing definitions, examples, and modeling approaches. The document is part of a larger course or module related to the topic.
Full Transcript
B.Sc. Degree in Applied Statistics Statistics in Health Sciences 9. Causality, confusion and interaction Jose Barreraab [email protected] https://sites.google.com/view/josebarrera a ISGlobal Barcelona Institute for Global Health - Campus MAR b Department of Mathematics (UAB) This work is lice...
B.Sc. Degree in Applied Statistics Statistics in Health Sciences 9. Causality, confusion and interaction Jose Barreraab [email protected] https://sites.google.com/view/josebarrera a ISGlobal Barcelona Institute for Global Health - Campus MAR b Department of Mathematics (UAB) This work is licensed under a Creative Commons “Attribution-NonCommercial-ShareAlike 4.0 International” license. Statistics in Health Sciences 1 Introduction 2 Causality 3 Confusion 4 Interaction Jose Barrera (ISGlobal & UAB) Statistics in Health Sciences, 2023/2024 2 / 19 Causality, confusion and interaction: Introduction Introduction • Does a positive correlation between milk consumption and cancer imply a causal relationship? • What should we take account for before assuming a significant association between a given exposure and a given health outcome? https://xkcd.com/552/ Correlation does not imply causation. Jose Barrera (ISGlobal & UAB) Statistics in Health Sciences, 2023/2024 3 / 19 Causality Definition In the context of epidemiology, a casual relationship between an exposure E and an outcome D is defined as a relationship that meets the following conditions: 1 E precedes D (as in the cases of cohorts studies and randomized epidemiological trials). 2 There is a significant statistical association between E and D. 3 Such an association is not the result of an association between a third variable and D and E. Jose Barrera (ISGlobal & UAB) Statistics in Health Sciences, 2023/2024 4 / 19 Causality: Causal relationships between E and D Cause-effect Cause-effect: examples • Smoke and lung cancer. • Sedentary lifestyle and obesity. E actually causes D status. E D Common causes Common causes: examples X actually causes both E and D, which results in an association between E and D. E X D Common effects Common effects: examples E and D share a common effect on Y , so E|Y and D|Y are correlated. E • Having a lighter in the pocket and lung cancer (X = smoker). • Milk consumption and life expectancy (X = country development level). Y Jose Barrera (ISGlobal & UAB) D • If a gene of interest (E) and drugs consumption (D) have a common effect such as mental disruption (Y ), an association between E and D could be expected if data were gathered only among individuals with mental disruption (i.e., for a given level of Y ). Statistics in Health Sciences, 2023/2024 5 / 19 Causality: Causal relationships between E and D Causal mediation Some or all of the total effect of E on D operates through a mediator M, which is an effect of E and a cause of D. M E E M D D Partial mediation Total mediation Example and modeling approach Mediating effect of lifestyle factors (M) on the association between social networks (E) and metabolic syndrome (D) (see Jung [1] ). Jose Barrera (ISGlobal & UAB) Statistics in Health Sciences, 2023/2024 6 / 19 Causal inference in epidemiology Causal inference • Modeling causation in epidemiology is a difficult issue because the natural mechanism that links the exposure(s) to the outcome could be complex and involving a large number of characteristics (i.e. variables) of the individuals. • Usually, the true mechanisms are unknown and researchers can only hypothesize about such mechanisms and try to model them with mathematical and statistical methods. • Causal inference in epidemiology comprises a number of such methods to try to deal with the problem. • For an introduction to causal inference in epidemiology see, for instance, Rothman and Greenland [2] and the work by Miguel Hernana . a https://www.hsph.harvard.edu/miguel-hernan/causal-inference-book/ Jose Barrera (ISGlobal & UAB) Statistics in Health Sciences, 2023/2024 7 / 19 Confusion Introductory example Suppose we are interested in analyze the possible help that a new diet complement could provide to reduce blood pressure. 2400 adult people participate in an experiment (50% women and 50% men). Participants are divided in two groups, A and B, of equal sizes. Group A is treated with the complement while group B is treated with a placebo. At the end of the experiment we analyze the resulting data. . . Data Results Improvement? Group Placebo Complement Total Total No Yes 780 528 420 672 1200 1200 1308 1092 2400 Jose Barrera (ISGlobal & UAB) c = • RR 672/1200 420/1200 = 1.6 • The probability of reducing blood pressure in the diet complement group was estimated to be 60% higher than in placebo group. Statistics in Health Sciences, 2023/2024 8 / 19 Confusion Introductory example (cont.) Now, we repeat the analysis but stratifying by sex. . . Data stratified by sex Women Improvement? Group Men No Yes Total Group Placebo Complement 60 336 180 624 240 960 Total 396 804 1200 c♀ = RR 624/960 180/240 Improvement? No Yes Total Placebo Complement 720 192 240 48 960 240 Total 912 288 1200 ≈ 0.867 c = RR ♂ 48/240 240/960 = 0.8 Interpretation What happens? What could be the reasons? Which results would you report? Jose Barrera (ISGlobal & UAB) Statistics in Health Sciences, 2023/2024 9 / 19 Confusion Introductory example (cont.) We can see that most of the women received the diet complement while most of the men received the placebo. What would we expected if 50% of women and 50% of men received the diet complement? Data stratified by sex, with 50% of women and 50% of men receiving the diet complement Women Improvement? Group Men No Yes Total Group Placebo Complement 150 210 450 390 600 600 Total 360 840 1200 c♀ = RR 390/600 450/600 Improvement? No Yes Total Placebo Complement 450 480 150 120 600 600 Total 930 270 1200 ≈ 0.867 c = RR ♂ 120/600 150/600 = 0.8 Interpretation Results by sex are the same but now we can aggregate data to estimate RR adjusted by sex. . . Jose Barrera (ISGlobal & UAB) Statistics in Health Sciences, 2023/2024 10 / 19 Confusion Data aggregated by sex, with 50% of women and 50% of men receiving the diet complement All Improvement? Group Yes 600 690 600 510 1200 1200 1290 1110 2400 Placebo Complement Total Total No c = RR 510/1200 600/1200 = 0.85 Interpretation • Now, RR estimate for data aggregated by sex is coherent with RR estimate for each sex. We have controlled the confusion induced by sex. • We see that the diet complement seems not to help to reduce blood pressure. • Women could naturally reduce blood pressure, which confounds the relationship between the diet complement and blood pressure reduction. Jose Barrera (ISGlobal & UAB) Statistics in Health Sciences, 2023/2024 11 / 19 Confusion Introductory example (cont.) Balancing diet complement vs placebo within sex doesn’t need to be at 50%-50%. . . New analysis with 50(1 + p)% of women and 50(1 + p) of men with diet complement, p ∈ (−1, +1) Women Group Improvement? Men No Yes Total Placebo Comp. 150(1 − p) 210(1 + p) 450(1 − p) 390(1 + p) 600(1 − p) 600(1 + p) Total 360 + 60p 840 − 60p 1200 c♀ = RR 390/600 450/600 ≈ 0.867 Improvement? Group All No Yes Total Placebo Comp. 450(1 − p) 480(1 + p) 150(1 − p) 120(1 + p) 600(1 − p) 600(1 + p) Placebo Comp. Total 930 + 30p 270 − 30p 1200 Total c = RR ♂ 120/600 150/600 = 0.8 Group Improvement? No Yes Total 600(1 − p) 690(1 + p) 600(1 − p) 510(1 + p) 1200(1 − p) 1200(1 + p) 1290 + 90p 1110 − 90p 2400 c = RR 510/1200 600/1200 = 0.85 Interpretation • To control the confusion induced by sex, the proportion of women receiving the diet complement should be the same than among men. That proportion doesn’t need to be 50%. • Controlling the confusion induced by sex, we get coherent estimates for RR♀ , RR♂ and RR. Jose Barrera (ISGlobal & UAB) Statistics in Health Sciences, 2023/2024 12 / 19 Confusion Definition A variable C is a confounder in the association between an exposure E and an outcome D if it has an effect on D and it is associated with E. Consequences • Potential inconsistent results when including or excluding C in the analysis. C E • Bias in the estimation of the association of interest between E and D. D Simpson’ paradox An extreme case of confusion is the Simpson’s paradox, which arises when the marginal association (i.e. ignoring C) can have a different direction from each conditional (to C) association. For details and examples, see Agresti [3] . Jose Barrera (ISGlobal & UAB) Statistics in Health Sciences, 2023/2024 13 / 19 Confusion Confusion control • In order to control potential confusion problems, we should take into account all possible risk factors as well as controlling for all potential confounders. • In the regression models framework, confusion is commonly controlled by including the potential confounders in the linear predictor. Then, adjusted effects (i.e. comparisons within the same level of the confounder) are estimated. • In very simple analysis such as contingency table analysis with just one categorical confounder, stratification or balanced design (i.e. a similar distribution of C between exposed and non exposed) could help. • In epidemiology, when assessing the effect of an exposure of interest, typical confounders are sex and age. Jose Barrera (ISGlobal & UAB) Statistics in Health Sciences, 2023/2024 14 / 19 Confusion Confusion control in regression models: example Our aim is to assess the association between intima media thickness, IMTa , (T , in mm) and age (A, in years). We assume T |A is normally distributed and T and A are linearly related. We decide to fit a linear regression model. We suspect that sex (S) could be a confounder in the relationship of interest. 1 Linear regression model for the crude association between T and A (i.e. ignoring S): T |A ∼ N (µ(A), σ), µ(A) = β0 + βA A. Interpretation: βA is the crude expected change in the mean of the IMT for each 1-year increase in age, regardless of if the individuals compared have or not the same gender. 2 Linear regression model for the adjusted association for sex between T and A: T |(A, S) ∼ N (µ(A, S), σ), µ(A, S) = β0 + βA A + βS S. Interpretation: βA is the adjusted (for sex) expected change in the mean of the IMT for each 1-year increase in age, when individuals compared have the same gender. Note that with this model we are assuming that the size of the association between age and IMT is the same among women than among men. . . a Carotid IMT is used to detect the presence of atherosclerosis. Jose Barrera (ISGlobal & UAB) https://en.wikipedia.org/wiki/Intima- media_thickness. Statistics in Health Sciences, 2023/2024 15 / 19 Interaction Definition If the association between E and D varies among different levels of a third variable X , we say that X modifies the effect of E on D and there is an interaction between E and X . Comments • In case of interaction, providing an overall measure of association between E and D would be incorrect. Contrary, the association should be described for each level of X . • In the case of a continuous X , a graphical representation of the measure of the association between E and D as a function of X can be useful. • The effect of the interaction does not depend on the distribution of X |E. • Not dealing properly with an interaction can result in a biased estimation of the association of interest (i.e. between E and D). • The result of an interaction could vary depending on the measure of association. For instance, the OR could change for all levels of X while the PR could be invariant across some levels. Jose Barrera (ISGlobal & UAB) Statistics in Health Sciences, 2023/2024 16 / 19 Interaction: examples Modeling an interaction with a linear regression models: example In the example in slide 15 about the association between IMT and age, adjusted for sex (as a potential confounder), we suspect that the effect of age on IMT could depend on glucose levels in blood (G, in mg/dl). We assume that, for each level of glucose, T |A is still normally distributed and T and A are linearly related. We decide to fit a linear regression model. 1 Linear regression model for the adjusted association for sex and glucose between T and A: T |(A, G, S) ∼ N (µ(A, G, S), σ), µ(A, G, S) = β0 + βA A + βG G + βS S. Interpretation: βA is the adjusted (for sex and glucose) expected change in the mean of the IMT for each 1-year increase in age, when individuals compared have the same gender and glucose level. The association between age and IMT does not depend neither on sex nor glucose level. . . 2 Linear regression model for the adjusted association for sex between T and A, modified by G: T |(A, G, S) ∼ N (µ(A, G, S), σ), µ(A, G, S) = β0 + βA A + βG G + βAG A · G + βS S. Interpretation: ( Prove that) (βA + βAG G) is the adjusted (for sex) expected change in the mean of the IMT for each 1-year increase in age, when individuals compared have the same gender and the same glucose level G. The association between age and IMT is the same among women than among men but depends on glucose level. Jose Barrera (ISGlobal & UAB) Statistics in Health Sciences, 2023/2024 17 / 19 Interaction: examples Graphical interpretation of interaction: example Continuing with the example in slide 17, suppose we have categorized the glucose level in blood as Z = 0 if G ⩽ 140 mg/dl or Z = 1 if G > 140 mg/dl. Describe each of the following patterns in terms of a possible interaction between glucose and age in the effect on IMT. b) Age Jose Barrera (ISGlobal & UAB) d) Z =0 Z =1 IMT Z =0 Z =1 IMT IMT Z =0 Z =1 c) Age Z =0 Z =1 IMT a) Age Statistics in Health Sciences, 2023/2024 Age 18 / 19 References [1] SJ. Jung. Introduction to mediation analysis and examples of its application to real-world data. Journal of Preventive Medicine and Public Health, 54(3):166–172, 2021. URL https://doi.org/10.3961/jpmph.21.069. [2] KJ. Rothman and S. Greenland. Causation and causal inference in epidemiology. American Journal of Public Health, 95(S1):S144–S150, 2005. URL https://10.2105/AJPH.2004.059204. [3] Alan Agresti. Categorical Data Analysis (3rd Edition). John Wiley & Sons, Inc., Hoboken, New Jersey, 2013. Jose Barrera (ISGlobal & UAB) Statistics in Health Sciences, 2023/2024 19 / 19