Segmentation Analysis PDF
Document Details
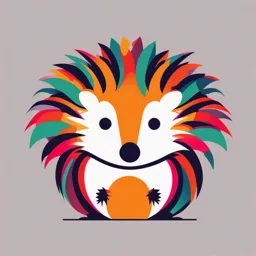
Uploaded by ConciseTrust
Tags
Summary
This document provides a comprehensive overview of segmentation techniques, focusing on both theoretical underpinnings and practical application. The content highlights the importance of segmentation in achieving market success and provides a detailed analysis of different methods such as algorithmic-analytical and classical approaches, with examples.
Full Transcript
The Value Creation and Value Communication/Delivery Pods Value Creation: Forecasting primary demand evolution Exploratory research for customer decision factor identification Customer preference measurement Optimal product and price specification Value Communication and Delivery: Characterizing custom...
The Value Creation and Value Communication/Delivery Pods Value Creation: Forecasting primary demand evolution Exploratory research for customer decision factor identification Customer preference measurement Optimal product and price specification Value Communication and Delivery: Characterizing customer segments Formulating the positioning statement Assessing advertising creative Salesforce and channels choices Segmentation 1 Segmentation: Done Often, Often Done Wrong 81% of senior executives say it is critical tool for achieving market success 59% of companies run a major segmentation initiative at least once in 2 years 87% execute one at least once every 3 years Only 14% say they used it effectively and got value Companies which successfully execute on a segmentation strategy post annual profit growth of about 15% versus for companies that don’t Segmentation 2 Two approaches to value creation and customer characterization The algorithmic-analytical approach: Use market share modeling + optimization to find the core features of your product Identify the characteristics of those likely to buy your product (your product’s “affinity segment”) in terms of the preference parameters and the background parameters The classical Segmentation-Targeting 3Cs approach: Do cluster analysis based on the preference parameters Characterize each segment in terms of the preference parameters and the background parameters Do a 3Cs analysis to identify the most attractive segment(s) Construct product(s) likely to appeal to the identified segment(s), ideally using market share modeling + optimization to find the core features of your product. Segmentation 3 Characterizing the Affinity Segment in the algorithmic-analytical approach For a candidate Product-Price P (though usually we consider just the “optimal” P), and for each available customer descriptor, compute the across-customer weighted average of the descriptor, weighted by the probability of a customer buying P. Example with Brand Importance as the descriptor Incumbents Our Candidate 1: $30, 20 oz, Clean Easy, Brand A 2: $10, 20 oz, Clean Fair, Brand B 3: $30, 20 oz, Clean Easy, Brand C Importances Pr Cp Cl Br ----------9 55 12 24 12 35 21 31 71 6 10 12 17 4 56 23 59 20 9 12 11 0 70 19 57 16 10 17 41 15 31 12 26 32 17 25 53 13 13 20 Purchase Probs Weighted ave of descriptor x with weight w P1 P2 P3 P3*Br = (sum w_i*x_i)/(sum w_i) Cust -------------------1 0.703 0.202 0.095 2.28 2 0.555 0.403 0.042 1.30 3 0.009 0.968 0.024 0.28 4 0.614 0.062 0.324 7.45 5 0.125 0.633 0.243 2.91 6 0.254 0.015 0.731 13.88 7 0.006 0.991 0.004 0.06 8 0.058 0.912 0.030 0.36 9 0.434 0.512 0.054 1.35 10 0.006 0.991 0.003 0.06 ------------SUM 2.76 5.69 1.55 29.95 Weighted Average of Brand Importance for Buyers of Product 3 = 29.95/1.55 = 19.3 Segmentation 4 Repeat for every customer descriptor available: Attribute Importance Preference level for an attribute level × Importance of the attribute Demographics and other behavior/profile variables For insight on differences with respect to competing products analysis, we typically do this also for every other product in the category. Segmentation 5 Developing the personas for the affinity segments For each segment: Identify the “focal” descriptors where that segment is very different from the other segments. The segments will be generally be pretty different on the descriptors that constitute the segmentation basis, but often will be (but not necessarily) different on the profile descriptors as well. – Look at whether the mean value of the descriptor is extreme in terms of z-score – Compute the log lift for each segment for each descriptor for each segment and identify large negative or positive values. The lift is the mean value of the descriptor for the segment relative to the overall mean value of the descriptor. This approach is right strictly for just ratio-scaled measures but in practice is used for interval-scaled and ordinal-scaled measures as well Use the “focal” descriptors to characterize the segment Segmentation 6 seg.1.mean 17.55 seg.2.mean 48.10 seg.3.mean 21.90 ovr.mean 35.6 Cust IPr ICp 1 9 2 12 3 71 4 17 5 59 6 11 7 57 8 41 9 26 10 53 28.24 18.06 9.86 19.6 29.96 15.92 48.85 24.9 ICl 55 35 6 4 20 0 16 15 32 13 24.03 17.35 19.34 19.5 IBr 12 21 10 56 9 70 10 31 17 13 24 31 12 23 12 19 17 12 25 20 62.17 49.09 49.96 42.77 61.36 45.91 55.1 45 income age sports 63 50 64 50 45 37 59 50 46 41 68 45 45 41 47 44 67 51 47 41 0.36 0.30 0.84 0.4 0 0 0 1 1 1 0 0 0 1 0.10 0.17 0.49 0.2 gradschl 0 0 1 0 0 1 0 0 0 0 seg.1.mean 4.90 7.00 2.36 5.34 5.29 1.00 4.42 7.00 seg.2.mean 5.93 7.00 2.96 6.24 3.43 1.00 4.30 7.00 seg.3.mea 5.00 7.00 2.36 5.63 5.09 1.00 4.08 7.00 g n ovr.mean 5.5 7 2.7 5.9 4.2 1 4.3 7 Cust pPr10 pPr05 pCp12 pCp20 pCp32 pClD pClF pClE 1 3 7 1 7 6 1 2 7 2 6 7 1 7 6 1 6 7 3 6 7 7 4 1 1 3 7 4 6 7 7 1 3 1 5 7 5 4 7 1 7 2 1 3 7 6 5 7 1 7 7 1 4 7 7 7 7 1 7 7 1 3 7 8 6 7 1 7 2 1 6 7 9 5 7 1 5 7 1 6 7 10 7 7 6 7 1 1 5 7 seg.1.mean 6.373777 seg.2.mean 3.809586 seg.3.mean 4.338208 ovr.mean 4.6 Cust pBrA 1 7 2 7 3 1 4 7 5 3 6 3 7 3 8 5 9 7 10 3 3.450061 5.087992 1.631705 4.1 pBrB 5 5 3 1 1 1 7 7 4 7 2.730355 2.747557 5.698965 3.2 pBrC 1 1 7 5 7 7 1 1 1 1 P1 P2 0.703 0.555 0.009 0.614 0.125 0.254 0.006 0.058 0.434 0.006 P1 P3 0.202 0.403 0.968 0.062 0.633 0.015 0.991 0.912 0.512 0.991 P2 0.703 0.555 0.009 0.614 0.125 0.254 0.006 0.058 0.434 0.006 P1 P3 0.202 0.403 0.968 0.062 0.633 0.015 0.991 0.912 0.512 0.991 P2 0.703 0.555 0.009 0.614 0.125 0.254 0.006 0.058 0.434 0.006 0.095 0.042 0.024 0.324 0.243 0.731 0.004 0.030 0.054 0.003 0.095 0.042 0.024 0.324 0.243 0.731 0.004 0.030 0.054 0.003 P3 0.202 0.403 0.968 0.062 0.633 0.015 0.991 0.912 0.512 0.991 0.095 0.042 0.024 0.324 0.243 0.731 0.004 0.030 0.054 0.003 Two approaches to value creation and customer characterization The algorithmic-analytical approach: Use market share modeling + optimization to find the core features of your product Identify the characteristics of those likely to buy your product (your product’s “affinity segment”) in terms of the preference parameters and the background parameters The classical Segmentation-Targeting 3Cs approach: Do cluster analysis based on the preference parameters Characterize each segment in terms of the preference parameters and the background parameters Do a 3Cs analysis to identify the most attractive segment(s) Construct product(s) likely to appeal to the identified segment(s), ideally using market share modeling + optimization to find the core features of your product. Segmentation 7 High-level objectives of classical segmentation Exploiting differences among customers: Increase effectiveness/attractiveness of the offering by identifying key differences among customers to develop a customized offering that will be received better than a generic offering Exploiting similarities among customers: Increase the cost efficiency of the offering by identifying irrelevant differences among customers in order to deliver the same offering to customers within each segment Grow profits by a proper balance between the two. A segmentation project’s impact is known only after the product line or marketing mix has changed and there is evidence of growth. Segmentation 8 Here is a scatterplot of price importance and brand importance. Suppose we want to segment the market into 3 parts. What is the best partitioning into 3 parts? Segmentation 9 Cluster Analysis: Generic Description What cluster analysis does: Partitioning items into groups such that 1. Items within the same group are similar to one another (“homogeneity within”) 2. Items across groups are different from one another (“heterogeneity across”) Constructs item “archetypes”, and identifying each item with exactly one archetype. Segmentation 10 6 Cluster Analysis (cont’d) o 5 oo o o o o oo o 4 o o oo o o o o o o 3 Variable2 o o 2 o o o o 1 o o o o oo o o o 0 o o oo o o o o o o o o o ooo o o o o o oo o o o oo o o o 0 1 2 3 4 Variable 1 Cluster analysis appears an easy task but is in fact extremely difficult. Segmentation 11 The K-Means Method for Cluster Analysis Assume that you have a dataset and that you are looking for K clusters. Goal: To Identify K “archetypes” that are the center of each cluster First, pick any K items at random and take them to be K archetypes. Improve these archetypes by following the steps below: 1. For each item, identify the archetype that is closest to the item and say that the item is “associated with” the archetype. 2. For each archetype, do the following: Identify all the items associated with that archetype, and compute the average point for those items. This average point is the new and improved archetype. 3. Go back to step 1 and repeat, improving the archetype on each repetition. In the K-Means method, the archetypes at any stage are referred to as “seeds”. At the end, the items associated with an archetype are seen as belonging to one clusters. We have K archetypes and hence K clusters. Segmentation 12 K-Means Method Illustrated 2 Variable 2 4 6 Dataset and three items A, B, C chosen randomly to be the archetypes 0 BC A 0 2 4 6 Variable 1 Segmentation 13 0 2 6 4 Variable 2 4 C 2 3 23 3 3 33 33C 3 3 B 233 1 1 A1 1 1 2 3 33 3 333 3333 1 1 11111 1 1 1 B A 0 2 4 3 33 33 333 33333 3 33 33 33 3 3 0 Variable 2 6 K-Means Method Illustrated (Improvement Attempt 1) 0 6 2 4 Variable 1 Variable 1 Segmentation 14 6 6 4 C Variable 2 4 3 33 33 333 33333 3 33 3 33 3 3 3 C 0 2 B 0 2 1 11 1 111 1111 1 1 11111 A 1 1 1 4 2 2 22 2 3 2 2 2 222 2 1 1 22 2 A B 0 2 Variable 2 6 K-Means Method Illustrated (Improvement Attempt 2) 0 6 2 4 6 Variable 1 Variable 1 Segmentation 15 2 0 2 6 4 Variable 2 1 11 1 111 111A1 1 1 11111 1 1 1 4 2 2 22 2 2 22 22B 2 222 2 2 2 22 2 C A B 0 2 4 3 33 33 333 33333 3 33 33 3 3C 3 3 0 Variable 2 6 K-Means Method Illustrated (Improvement Attempt 3) 0 6 2 4 Variable 1 Variable 1 Segmentation 16 6 Problems with Cluster Analysis All cluster analysis methods known today break down when the number of variables is large (the “high-dimensionality problem”). We cannot have too many variables on which we are segmenting The variables all need to be numbers and cannot be categorical variables The number of archetypes is very rarely known in advance – Some managerial situations create exceptions to this. The commonly used methods identify only spherical clusters. Non-spherical clusters are often encountered because of correlations in preference parameters. Segmentation 17 Looking with Number of Archetypes greater than the true number of clusters 2 Variable 2 4 6 Dataset and four items A, B, C, D chosen randomly to be the archetypes 0 BC A 0 D 2 4 Variable 1 Segmentation 18 6 0 2 0 2 6 4 Variable 2 4 44 4 444 4444 4 4 44444 4 4 4 4 2 3 23 3 3 3 3C 3 3 B 233 4 4 A1 D 1 4 C D B A 0 4 3 33 33 333 33333 3 33 3 33 3 3 3 2 Variable 2 6 Improvement Attempt 1 0 6 2 4 6 Variable 1 Variable 1 Segmentation 19 6 4 C Variable 2 4 3 33 33 333 33333 3 33 33 33 3 3 2 B 0 2 4 44 4 444 444D4 4 4 44444 4 4 4 4 2 2 22 2 2 11 22 1 221 1 1 1 1A1 1 B D A 0 2 C 0 Variable 2 6 Improvement Attempt 2 0 6 2 4 Variable 1 Variable 1 Segmentation 20 6 Looking with Number of Archetypes less than the true number of clusters 2 Variable 2 4 6 Dataset and two items A, B chosen randomly to be the archetypes B 0 A 0 2 4 6 Variable 1 Segmentation 21 2 0 2 6 4 Variable 2 1 11 1 111 1111 1 1 11111 1 1 1 4 2 2 22 2 2 22 22 2 B 222 1 1 1 A1 1 1 B A 0 2 4 2 22 22 222 22222 2 22 22 22 2 2 0 Variable 2 6 Improvement Attempt 1 0 6 2 4 Variable 1 Variable 1 Segmentation 22 6 6 4 Variable 2 4 2 22 22 222 22222 2 22 2 22 2 2 2 B 0 2 0 2 1 11 1 111 1111 1 A 1 11111 1 1 1 4 2 2 22 2 2 2 2 1 222 1 1 1 11 1 B A 0 2 Variable 2 6 Improvement Attempt 2 0 6 2 4 6 4 6 Variable 1 Variable 1 Segmentation 23 2 0 2 6 4 2 1 11 1 111 1 1 111 1 1 1 11 1 11 A1 4 A 0 2 B 2 22 2 2 11 21 1 111 1 1 1 11 1 B Variable 2 4 2 22 22 222 22222 2 22 22 22 2 2 0 Variable 2 6 Improvement Attempt 3 0 6 2 Variable 1 Variable 1 Segmentation 24 How many segments? Consider: Within-Class Variance Managerial value of Targeting Between the two the first is more commonly used but may not be meaningful from a managerial or financial viewpoint. Segmentation 25 4 3 2 1 Average Within Group Variance 5 6 Within-Class Variance as a function of number of segments: For the small dataset used earlier to illustrate the K-Means procedure, we get the following graph: 1 2 3 4 5 Number of clusters Segmentation 26 6 7 Managerial considerations: Segmentation is beneficial when the incremental value from differentiating the needs of resulting segments and from separating the communications/distribution strategies exceeds the costs of implementing the distinct strategies. To achieve the required benefit/cost ratio, the segments should be Different in Responsiveness to offerings Identifiable: it should be possible to characterize a segment and determine whether a customer belongs to a segment Reachable: so that we can target marketing messages to a segment uniquely be Sizeable: In terms of potential profits be Stable: over time Segmentation 27 Variables used in the K-Means procedure need to be comparable In the K-Means procedure, a key step is figuring out which archetype (A, B, etc) is closest to some point i. (xi − xA )2 + (yi − yA )2 (xi − xB )2 + (yi − yB )2 etc It is critical that x and y be comparable conceptually. It is also critical that the variation in x is approximately the same as the range of variation of y. Example: What happens if x is Brand Importance and y is Income? If there are two sets of variables that are not comparable, we do not put them together into a K-Means procedure. Instead we use one set of variables for the K-Means procedure and use the other set to describe (“profile”) each cluster obtained from the K-Means procedure. Segmentation 28 Steps in Clustering and Profiling If several variables are available, first group them into variables that are of the same type and are comparable to one another. Example: One group is preference parameters, another group is demographics. Then decide which group of variables is more central to the marketing problem at hand. The chosen of variables is referred to as the segmentation basis set. The other groups of variables are the profiling set. If the variables within the segmentation basis set have very different variances, rescale to make them comparable: eg. standardize each variable or express each variable in percentiles. This makes each variable contribute equally to the distance metric. If we want some variables to contribute more then we need to weight them more in the distance metric. Clustering: Run K-Means clustering process using variable in the segmentation basis set. For each segment, compute the mean value of each variable. Caution: K-Means works only if the variable are interval-scaled. If the variables are not interval-scaled then we need to define a dissimilarity measure and use hierarchical clustering. Segmentation 29 Profiling: Now consider the variables in the profile set. For each segment, compute the mean value of each variable. Interpretation: For each segment, look the mean value computed in the two steps above and try to understand each segment and give it a name. It often helps to express each mean value relative to the global mean. Segmentation 30 What should you do cluster analysis on? We can do cluster analysis on: Background/Profile Factors – Interests, Demographics – Activities, Behaviors Value Drivers and Decision Factors – Keep in mind that these may be occasion-specific Segmentation 31 Mini Example CustID loprpref hibrpref age income 1 61 17 24 50700 2 26 28 16 54000 3 9 56 56 126300 4 22 26 56 112600 5 64 27 29 84600 6 54 20 22 56600 7 31 14 63 110600 8 55 21 23 33600 9 13 67 66 146000 10 27 55 43 145200 11 16 15 17 50000 12 7 68 65 109900 Two sets of variables: Preference for low price, Preference for a Premium (Hi) Brand Segmentation 32 Try Brand/Price Preferences as the Segmentation Basis Set We do K-Means with the preference levels as the variables. 12 60 9 40 50 10 30 Hi Brand Preference 3 2 5 4 20 8 6 1 11 0 7 20 40 60 Lo Price Preference Segmentation 33 Clustering: Run K-Means with various values for the number of segments. It turns out that 3 segments is best. Tag each customer with the segment ID, indicated below as impseg CustID loprpref hibrpref age income 1 61 17 24 50700 2 26 28 16 54000 3 9 56 56 126300 4 22 26 56 112600 5 64 27 29 84600 6 54 20 22 56600 7 31 14 63 110600 8 55 21 23 33600 9 13 67 66 146000 10 27 55 43 145200 11 16 15 17 50000 12 7 68 65 109900 impseg 1 2 3 2 1 1 2 1 3 3 2 3 Segmentation 34 Cluster Analysis Output Mean Values (or “Centroids”) of the segmentation basis set variables for each cluster impseg 1 2 3 loprpref hibrpref 58.5 21.25 23.75 20.75 14 61.5 Profiling Output Mean Values of the profile set variables for each cluster impseg 1 2 3 age 24.5 38 57.5 income 56375 81800 131850 It is helpful to name clusters. How would we name these? By developing personas for each cluster using the ideas described earlier. Segmentation 35 Now we try Demographics as the Segmentation Basis Set First, because Age and Income are of such different ranges, we standardize each. age income mean std.dev 40 20 90008 39998 stdage = (age - 40)/20 stdincome = (income - 90008)/39998 CustID loprpref hibrpref age income stdage 1 61 17 24 50700 -0.80 2 26 28 16 54000 -1.19 3 9 56 56 126300 0.80 4 22 26 56 112600 0.80 5 64 27 29 84600 -0.55 6 54 20 22 56600 -0.90 7 31 14 63 110600 1.14 8 55 21 23 33600 -0.85 9 13 67 66 146000 1.29 10 27 55 43 145200 0.15 11 16 15 17 50000 -1.14 12 7 68 65 109900 1.24 stdincome -0.98 -0.90 0.91 0.56 -0.14 -0.84 0.51 -1.41 1.40 1.38 -1.00 0.50 Segmentation 36 We do K-Means with the standardized age and standardized income as the variables. 9 3 7 12 0.0 0.5 4 -0.5 5 6 2 11 -1.0 Standardized Income 1.0 10 1 8 -2 -1 0 1 2 Standardized Age Segmentation 37 Clustering: Run K-Means with various values for the number of segments. It turns out that 2 segments is best. Tag each customer with the segment ID, indicated below as demseg CustID loprpref hibrpref age income 1 61 17 24 50700 2 26 28 16 54000 3 9 56 56 126300 4 22 26 56 112600 5 64 27 29 84600 6 54 20 22 56600 7 31 14 63 110600 8 55 21 23 33600 9 13 67 66 146000 10 27 55 43 145200 11 16 15 17 50000 12 7 68 65 109900 demseg 1 1 2 2 1 1 2 1 2 2 1 2 Segmentation 38 Cluster Analysis Output Mean Values (or “Centroids”) of the segmentation basis set variables for each cluster demseg 1 2 age 21.83 58.17 income 54917 125100 Profiling Output Mean Values of the profile set variables for each cluster demseg 1 2 loprpref 46.00 18.17 hibrpref 21.33 47.67 Segmentation 39 Importances vs Within-Attribute Ratings Which to use as a segmentation basis Using Importances as the segmentation basis is to done only as a first cut. An importance-based segment often contains many distinct segments within it. Example: Within the segment Brand as important, some people may prefer the premium brand and some people may prefer a value brand. These are each distinct segments within the segment that considers Brand important. Solution: For more detailed analysis, do segmentation based on attribute importances and the within-attribute preference levels. Segmentation 40 After Cluster Analysis and Profiling Consider the various segments and evaluate the potential attractiveness of each segment If the potential attractiveness of a segment is high, evaluate various candidate products for that segment. If the candidate product has a likelihood of success, modify the product line and marketing to accommodate the candidate product and grow line profits. Segmentation 41 Select One or More Target Segments to Serve The key factors on which we evaluate the attractiveness of a segment: Opportunities for Profit – Unmet Needs – Size/Growth Potential Structural Characteristics – Segment saturation – Rivalry – How easy is to enter? – Environmental Risk Segmentation 42 Product-Market fit – Fit between firm’s core competence and target market opportunity – Production/marketing capabilities and costs – Synergies across segments, product lines and channels – Startup Costs – Margin levels Segmentation 43 Savings Very Important Segment-C (15%) Segment-A (49%) Service Not Important A B C Age Income 21 3 45 40 39 10 Service Very Important Segment-B (36%) Age Income 16–25 0-5 33–55 25-71 39–54 8-20 Savings Not Important Segmentation 44 Common Mistakes in Targeting Focusing only on Customer in targeting We should target XYZ because they are the largest segment We should target XYZ because they are the most lucrative segment Problem: This may be wishful thinking given Competition and Company analyses Lack of a good segmentation of all Customers We should target people like our existing customers because our existing customers must like us, and so would people like them Problem: This could miss out on opportunities Not anticipating competitive reaction Segmentation 45