Remote Sensing for Insect Pests and Diseases Management PDF
Document Details
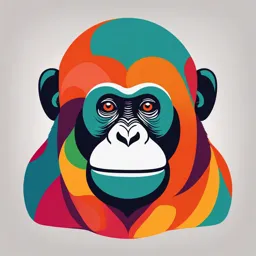
Uploaded by HardWorkingAgate2270
Universidade de São Paulo
Nesreen M. Abd El-Ghany, Shadia E. Abd El-Aziz, Shahira S. Marei
Tags
Summary
This review examines the applications of remote sensing in managing insect pests and plant diseases, highlighting the historical and current strategies. The techniques measure and process electromagnetic radiation from the ground to detect, forecast, and manage problems in orchards and crops. The method may reduce chemical pesticide use and provide fast and accurate pest forecasting.
Full Transcript
Environmental Science and Pollution Research (2020) 27:33503–33515 https://doi.org/10.1007/s11356-020-09517-2 REVIEW ARTICLE A review: application of remote sensing as a promising strategy for insect pests and diseases management Nesreen M. Abd El-Ghany 1 & Shadia E. Abd El-Aziz 1...
Environmental Science and Pollution Research (2020) 27:33503–33515 https://doi.org/10.1007/s11356-020-09517-2 REVIEW ARTICLE A review: application of remote sensing as a promising strategy for insect pests and diseases management Nesreen M. Abd El-Ghany 1 & Shadia E. Abd El-Aziz 1 & Shahira S. Marei 1 Received: 12 January 2020 / Accepted: 28 May 2020 / Published online: 20 June 2020 # Springer-Verlag GmbH Germany, part of Springer Nature 2020 Abstract The present review provides a perspective angle on the historical and cutting-edge strategies of remote sensing techniques and its applications, especially for insect pest and plant disease management. Remote sensing depends on measuring, recording, and processing the electromagnetic radiation reflected and emitted from the ground target. Remote sensing applications depend on the spectral behavior of living organisms. Today, remote sensing is used as an effective tool for the detection, forecasting, and management of insect pests and plant diseases on different fruit orchards and crops. The main objectives of these applications were to collate data that help in decision-making for insect pest management and decreasing the environmental pollution of chemical pesticides. Airborne remote sensing has been a promising and useful tool for insect pest management and weed detection. Furthermore, remote sensing using satellite information proved to be a promising tool in forecasting and monitoring the distribution of locust species. It has also been used to help farmers in the early detection of mite infestation in cotton fields using multi-spectral systems, which depend on color changes in canopy semblance over time. Remote sensing can provide fast and accurate forecasting of targeted insect pests and subsequently minimizing pest damage and the management costs. Keywords Remote sensing. Applications. Plant protection. Insect pests. Plant disease. Management Introduction understanding of data collection. This technology is utilized for measuring and recording the reflection and the emission of Recently, the development of information technologies as re- the electromagnetic radiation from the target area using the mote sensing sensors, GIS (geographic information systems), sensor instruments. Various sensor instruments are digital and GPS (global position systems) support a new approach for cameras, electro-mechanical scanners, video cameras, and ra- detecting and controlling insect pests. Remote sensing tech- dar systems. Such technologies depend on the electromagnetic nologies are mainly depending on receiving and interpreta- energy and the interactions between radiation and ground tar- tions of information about the earth’s surface without any gets (Yang and Everitt 2011). The electromagnetic spectrum physical contact it (Nilsson 1995). Remote sensing is mainly is classified into visible and invisible (UV, gamma, X-ray, dependent on the electromagnetic energy which influences the infrared microwave and radio) radiation. Naturally, electro- magnetic energy is produced by the sun and in a straight path at the speed of light across millions of kilometers of free space Responsible Editor: Philippe Garrigues to attain the earth atmosphere and surface. These radiations are reflected upward from the earth’s surface while others are * Nesreen M. Abd El-Ghany [email protected] absorbed or emitted again from the earth’s surface as thermal energy. These electromagnetic radiation consists of an electri- Shadia E. Abd El-Aziz cal field and magnetic field which are oriented at right angles [email protected] to the electrical field. There are two main characteristics of Shahira S. Marei electromagnetic radiations, the wavelength and frequency, [email protected] which are important for understanding remote sensing. The wavelength is the length of one wave cycle represented by 1 Department of Pests and Plant Protection, Agricultural and the Greek letter lambda (λ), which can be measured as the Biological Division, National Research Centre, 33 EL-Buhouth St. (former EL-Tahrir St.), Dokki, Giza 12622, Egypt distance between successive wave crests and measured in 33504 Environ Sci Pollut Res (2020) 27:33503–33515 meters or its smaller units (cm, μm, nm). Frequency (the can be achieved either in photographic or electronic form. The Greek letter mu; ν) measured in hertz (Hz) equivalent to one first is called a photograph, while the second is called an cycle per second (Yang and Everitt 2011). image. Most of the natural surfaces behave as diffuse reflec- Highly valuable remote sensing applications reported in tors, which associate remote sensing applications as a spectral various fields include: weather, agriculture, forest ecosystems, behavior of living plant leaves. water resources, insect pest management, and marine sci- ences. These applications are divided into three main strate- Components of remote sensing system gies: monitoring and mapping of various earth resources, fore- casting and prediction models, and finally management and Remote sensing systems involve 6 main components: energy decision-making depends on the data collected. Forecasting source, interaction with the target, sensors, platforms, trans- and prediction models depend on the retrieval data from mission and reception processing, interpretation and analysis geo-and bio-physical parameters (Oumar and Mutanga process, and applications (Natural Resources Canada 2019). 2011; Piekarczyk 2014). Today, remote sensing systems are carried on satellites and involve multi-spectral imaging de- vices characterized by achievement of synoptic view, fast sur- Energy source vey, high accuracy, inaccessible coverage area, etc., compared with the conventional methods for monitoring and mapping. It illuminates electromagnetic energy to the target. Sensors For example, remote sensing systems carried on satellites are have been classified as active and passive systems with re- used to characterize vegetation or calculate water surfaces spect to energy source. during floods which supplies ecologists and hydrologists with fast and accurate data on monitoring the water cycle and its Passive system It represents solar radiations, either absorbed, influence upon the ecosystem (Malinowski et al. 2015). This or reflected (visible wavelengths), or emitted (thermal infrared review highlights various topics on the principals of remote wavelengths) from the earth’s materials. sensing systems and their applications for approaches to agri- culture; in particular, management of insect pests and plant Active system It represents the artificial energy that produces diseases. its own electromagnetic radiation such as microwaves, laser fluoro sensor, and radar. Remote sensing systems Interaction with the target Principals of remote sensing systems Once the energy travels through the atmosphere from its source, it interacts with the target, relying on the target’s prop- Today, electromagnetic energy is produced artificially with no erties and radiation. Various interactions are possible such as fundamental physical variations between natural and artificial the following: (1) absorbed by a substance, (2) emitted by a ones. The electromagnetic energy can be detected by either substance, (3) scattered, and (4) reflected. frequency or wavelength. The electromagnetic spectrum is classified according to Avery and Berlin (1992) into various Sensors spectral regions (Table 1). Remote sensors are devices collecting data of the reflected and released electromagnetic Various devices were registered to gather and track the elec- radiation from a distance. Detection of electromagnetic energy tromagnetic radiation emitted or scattered from the target (camera, scanner, etc.). Remote sensors are classified as opti- Table 1 Major spectral regions of electromagnetic spectrum cal (sensors work within the visible, near, middle, and thermal infrared wavelength regions) and microwave (sensors work Spectral region Wavelength (μm) within frequencies of electromagnetic waves between 109 and 1012 Hz). Gamma and X-rays < 0.01 Ultraviolet 0.01–0.4 Visible 0.4–0.7 Platforms Near-infrared 0.7–1.5 Mid-infrared 1.5–5.6 It represents the sensor carriers that include the following: Far infrared 5.6–1000 ground-based (e.g., Handheld Radiometers), airborne (e.g., Microwave and radio > 1000 aircraft, AVIRIS Sensor of NASA), or space borne (e.g., space shuttle and satellite). Environ Sci Pollut Res (2020) 27:33503–33515 33505 Transmission, reception, and processing and down, which make a camera capture photographs within a limited time (about 8 min only). However, satellites revolve It represents the handling of data recorded by the sensor, around the earth following a specific orbit. Satellites provide which was transmitted to the earth station, often in electronic constant surveillance of the earth, e.g., low-level satellites form, where the data was stored as photographs, digital im- (700–1500 km) and high-level satellites (36,000 km). ages, etc. Satellites are classified according to their orbital characteristic into three types: equatorial geosynchronous orbits (e.g., Interpretation and analysis NOAA weather satellites), polar geosynchronous orbits (e.g., NPOESS: National Polar-orbiting Operational The process of interpreting the data obtained to extract desti- Environmental Satellite System), and sun orbits. In 1972, nation information using different image processing tools Landsat sensors represent the first international satellite pro- (hardware and software). Subsequently, it is the obtained in- gram managed by NASA (the National Aeronautics and formation used in fixing a particular problem. Space Administration) designed for collecting multi-spectral image data of the earth’s surface. Subsequently, in 1982, the Applications French government contrives the SPOT program for satellite sensors. On the other hand, high-resolution satellite systems It reveals the performance consequences of the method of have been launched (i.e., IKONOS (Earth observation satel- remote sensing which has achieved a useful application of lite, with high-resolution imagery at 1- and 4-m resolution): in the information obtained to solve a particular problem. the late 1990s, QuickBird: in 2001, GeoEye-1: 2008, WorldView-2: in 2009). These systems were used in different Devices and platforms of remote sensing systems agriculture approaches (Malinowski et al. 2015; Chuang and Shiu 2016; Johansen et al. 2018). The last space borne plat- The remote sensing devices are categorized into two main form is a manned space vehicle that provides the availability types: non-imaging devices such as a spectroradiometer and of direct human control during imagery of the earth from imaging devices such as different types of cameras. space. Also, such platform type supports the ability to carry Classification of remote sensing can be divided according to the remote sensing devices into the space and return them to wavelength regions into visible and reflective infrared, ther- the ground for recalibrating and refurnishing. mal infrared, and microwave remote sensing. Also, it was Different remote sensing systems are recorded as (i) divided into three types according to sensor-carrying plat- ground-based spectroradiometers, (ii) aerial photographic forms as ground-based, airborne, and space borne sensors. cameras (visible and NIR spectrum covered), (iii) electro- Ground-based platforms include ground, vehicles, and towers mechanical scanners (transform the electromagnetic radiation (up to 50 m). Examples of the ground-based platforms are into electrons or electrical signals from a scene viewed), (iv) atmospheric radiation program (DOE ARM: The airborne digital multi-spectral system (< 10 bands of spectra, Atmospheric Radiation Measurement (ARM) in Department covering the spectrum of visible and NIR portion), (v) o f E n e r g y ( D O E ) ) a n d N A S A R o b o ti c N e t w o r k hyperspectral imaging systems (10–100 s of bands, spanning (AERONET). Airborne sensor platforms using airplanes, a broader spectrum frequency range allows for better target high-altitude aircraft, balloons, and helicopters represent con- characterization and identification), (vi) thermal infrared scan- venient, flexible platforms that covered a few tens/thousands ner (longer wave infrared emittance spectra covered that gen- of meters (up to 50 km), where atmospheric effects are negli- erates imagery outside the spectral region of the photographic gible. The airborne observation using such platforms has lim- film. It is a characteristic of multi-faceted rotating mirror in- ited duration for few hours, as compared with space borne clined at 45°to the rotation axis, which scan the ground surface sensors. Examples of airborne sensor platform are the US along the lines perpendicular to the flight direction), (vii) mi- National Center for Atmospheric Research (NCAR), crowave imagers (collect radiation information covering the National Oceanic and Atmospheric Administration (NOAA), microwave wavelength using the antenna and commonly and NASA research aircrafts. On the other hand, space borne known as radar which, distinguished by wide operation ability sensor platforms overcome the challenges of lower altitude, where conventional aerial photography cannot be applied), atmospheric conditions, and limited duration of data collec- and (viii) satellite imaging systems (multi-spectral scanners tion. Space borne platforms include rockets, shuttles, satel- can sense different separate spectral channels designed to pro- lites, and manned space vehicles which cover from high alti- vide images of the earth simultaneously). The most recent tudes that ranged between 100 to 36,000 km. Rockets are used satellite sensors are super spectral image systems which are for recording the orientation of the rocket in flight using pho- capable of acquiring images from about 36 spectral wave- tographic system. Disadvantages of rockets as restricted peri- length bands (visible, near-infrared (NIR) portion, and short- od coverage of fine weather, and fast fired vertically upward wave infrared to the thermal infrared of the spectrum 33506 Environ Sci Pollut Res (2020) 27:33503–33515 coverage). The bands have narrower bandwidths, which allow applications, i.e., the hyper-spectral sensors and multi- the sensor to capture the finer spectral characteristics of the spectral sensors (Fig. 1). These systems used either video targets (Fernando et al. 2018). and/or single-band cameras with a certain filter lens which Various factors should be considered when choosing the makes the availability of imaging specific wave (blue band, suitable system of remote sensing including spatial, spectral, green band, red band, NIR band, thermal band) (Coops et al. radiometric, and temporal resolutions. The spatial resolution 2006; Fletcher and Everitt 2007; Yang et al. 2009a; Oumar demonstrates the estimate of the littlest protest that can be and Mutanga 2011; Zhang et al. 2018). Applications of air- found within the field or detected in an image. The fundamen- borne remote sensing were investigated by many authors for tal unit of a picture is called a pixel. One-meter spatial resolu- detection and analyses of insect pest damage in the field tion implies each pixel speak to a zone of 1 m2. The spectral (Oumar and Mutanga 2011; Acharya and Thapa 2015; Senf resolution alludes to the number of bands and each band’s et al. 2017). Furthermore, it has been used for mapping crop wavelength width. So the shorter wavelength width can be diseases and assessing its impact on crop productivity (Qin recognized in higher spectral resolution images. However, and Zhang 2005; Franke and Menz 2007; Dutta et al. 2014; the main factor that affects the sensitivity of the remote sen- Pretorius et al. 2017; Dhau et al. 2018a, b). For example, sors to divergent reflectance levels is the radiometric resolu- delineate damage gives rise to a number of serious pests like tion. Finally, the temporal resolution represents how the re- hemlock looper and bark beetles; color and color-infrared ae- mote sensing platform can scan across an area. For example, rial photography with conventional camera have been used the normal orbiting satellites provide data each time they pass effectively (Fig. 2). Also, the damage caused by aphid species over an area. On the other hand, geostationary satellites used is revealed by mapping of the Mississippi river delta, and to support continuous sensing and data scanning all over the detection of the most probable areas where insects might at- detected area. tack the wheat crop is predicted through remote sensing (Yang et al. 2009b). Remote sensing systems in agricultural approaches Remote sensing applications for pest management Remote sensing has become an important tool for sustainable agricultural management. Various applications in agriculture For successful pest management programs, basic information field were developed as crop discrimination, crop growth is a critical point of view. Available information on the insect monitoring, stress detection, soil moisture estimation, soil fer- damage level, weed infestation, and plant diseases can explore tility, insect infestation, plant disease infestation, water salin- a clear vision for decision-making of management programs. ity, underground water level, computation of evapo- Remote sensing technology provides data assists in decision transpiration for crops, site-specific management, crop acre- support systems for various pest management programs de- age, and estimation of yield prediction (Zhang et al. 2016; pending on monitoring, mapping, and predicting outbreak Blaes et al. 2016; Odi-Lara et al. 2016). Precision agriculture patterns. The accurate and efficient monitoring of insect pop- offers knowledge of the spatial complexities of biotic and ulations is the key point to improve pest control. Also, the abiotic environments, demonstrating the advantages of precise rapidity and thoroughness of forecasting and reducing man- amendments and effective applications of pesticides, fertil- agement costs have increased by using computer-based sys- izers, and herbicides leading to reduce chemical applications tems. The important component of the IPM strategy is forecast and maintaining crop profitability. For instance, site-specific for pests. Forecasts and early warnings based on biophysical crop management (SSCM) reliefs increasing of crop produc- methods supply time for managing close pest attacks and can tivity with minimal input in precision agriculture (Peralta et al. consequently optimize pest control, reduce crop loss, and min- 2016). Moreover, Fitzgerald (2000) used multi-spectral re- imize the cost of cultivation. Detecting crop stresses like nu- mote sensing to detect early infestation of mites in cotton trient deficiency, pest infestation, and disease development, fields due to color shifts and subsequently the changes that and monitoring drought by remote sensing techniques are occur in the canopy appearance. Identification of infected useful. Remote sensing improves spatial and temporal resolu- areas was determined on the map using portable GPS equip- tion compared with traditional methods for pest monitoring ment by field scouts to verify the mite populations in the such as sex pheromone traps for selective monitoring of indi- investigated areas, which lead to discriminate specific regions vidual species, light traps for various species, and multi- in the cotton fields that require pesticide application. location long-term suction traps for detecting migration pat- Remote sensing provides valuable information for IPM terns of insect pests (Prasad and Prabhakar 2012). These tech- context in different agricultural approaches. For example, var- nologies based on plant protection research for major pests are ious airborne remote sensing were used for agricultural of extreme importance to attain sustainability in agriculture. Environ Sci Pollut Res (2020) 27:33503–33515 33507 Fig. 1 Airborne digital multi- spectral and hyperspectral imag- ing systems composed of 4 multi- spectral imaging cameras (Yang 2012) and one hyperspectral im- aging camera (Yang et al. 2003) fixed on USDAARS kika de la Garza Subtropical Agricultural Research Center in Weslaco, Texas Remote sensing techniques are being inspected around the Jones conducted the remote sensing technique on whitefly world for their suitability in automating or supplementing this infestations depending on direct and indirect effects on vari- system. The available remote sensing technologies take con- ous crops (Fig. 3). The direct damages are explored by a trol by acquiring and processing multi-spectral images reduction in plant vigor due to feeding activities, while the (Andreo 2013; Sivanpillai and Latchininsky 2013). indirect damages include the transmission of important plant Moreover, integration of remote sensing and other GPS and diseases, in addition to the growth of sooty mold fungi on GIS technologies is applied for detecting and mapping of in- honeydew excreted by feeding nymphs. Also, Yan et al. sect infestations. For example, aerial videography is integrated (2017) established volunteered geographic information with GPS and GIS to develop regional mapping for insect (VGI)–based IPM system in Shuibian (province of Jiangxi), infestations over a large agricultural and forest area China, to assess the effectiveness of such approach for the (Robertson et al. 2008). Such combinations help the agricul- analysis of infestation level, and the timing outbreak of striped tural consultant in decision-making. In 2004, Morales and rice stem borers depends on voluntary mobile phone reports Fig. 2 Aerial photograph through remote sensing-illustrated map- ping of probable areas of hemlock looper and bark beetle (Rani et al. 2018) 33508 Environ Sci Pollut Res (2020) 27:33503–33515 Fig. 3 Mapping of whitefly infestations in cotton fields at Hidalgo County using GIS technology. The triangles explore detailed map within the study area clarify GPS latitude longitude coordinates of different locations of whitefly infestations (Yang and Everitt 2011) from farmers and spatiotemporal infestation pattern of the entomologists and other scientist’s disciplines, e.g., informatics borers throughout the study area. This technique shows a and electrical engineering. Remote sensing techniques are used unique merit that enhances IPM of the target pest. for disclosure of insect activity depending on the damage caused by the target insect pest, not on the actual organism. The damage Applications in insect pest managements levels are usually detectable as sooty mold deposits produced by honeydew-producing insects, plant defoliation, color changes, Recently, remote sensing plays a major role in pest management. and geometric distortion of the plant shape. Remote sensing It is acquired at high spatial resolution, or airborne at lower spatial sensors are widely used as new tools for pest scouting, monitor- resolution for large area coverage. Benchtop remote sensing has ing of migration swarms, the detection of infestation level, pre- become an important application in insect systematics, behavior, diction of outbreaks, and management of insect pests which in- and physiology (Nansen and Elliott 2016). In this concern, Wang vades different fruit orchards and crops (Abdullah and Umer et al. (2016) revealed the feasibility of using hyperspectral imag- 2004; Oumar and Mutanga 2011; Yang and Everitt 2011; Yan ing data in insect classification. They are used that the integrative et al. 2017; Fernando et al. 2018). Also, remote sensing is ac- taxonomy as a recent taxonomic approach for insect species is complished with spatial statistics tools for detecting and model- used to distinguish the closely related and cryptic insect species ing spatial links and correlations between the climatic and envi- by integrating various taxonomic data depending on the genetic, ronmental factors, e.g., mapping, modeling habitat, and popula- ecological, and morphological characters. Seven species that be- tion density of Ommatissus lybicus Bergevin (Hemiptera: long to the evacanthine leafhopper genus Bundera Distant Tropiduchidae) in The Sultanate of Oman (Al-Kindi et al. (Hemiptera: Cicadellidae) were identified based on an integrated 2017). Different techniques have been used for monitoring insect analysis of mitochondrial DNA, morphological characters, and pests, including optical and optoelectronic devices such as vide- hyperspectral reflectance profiling using 37 spectral bands that ography, radio frequency identification (RFID), radio-telemetry, ranged between 411 and 870 nm. Data of reflectance-based clas- X-rays radiography, thermal imaging, computed tomography, sification verified 91.3% classification accuracy for radar, and satellites. In the detection of insect infestation, there distinguishing the seven species. Also, these technologies are are many successful applications reported using airborne remote creating interdisciplinary bridges that help interaction between sensing. For example, detection of insect infestation on trees in Environ Sci Pollut Res (2020) 27:33503–33515 33509 some citrus orchards using aerial CIR (color-infrared) photogra- entomological radars incorporate airborne entomological radar phy and multi-spectral videography was used to figure out citrus (AER) (Hobbs and Wolf 1989), scanning entomological radar blackfly and brown soft scale problems in citrus as well as the (SER) (Riley et al. 1995), tracking entomological radar (TER) whitefly infestation in cotton (Hart et al. 1973; Everitt et al. (Westbrook et al. 1995), and vertical-looking radar (VLR) (Hu 1996). Subsequently, detection of mite early infestation in et al. 2016). Among these radars, SER and VER are the foremost large-scale cotton fields was investigated using multi-spectral commonly utilized to investigate the migration behavior of high- remote sensing (MRS) with coarse spatial resolutions. It mainly altitude migratory insects. Ordinary entomological radars work depends on the changes in canopy appearance through time and on the X-band (9.4 GHz) with a vertical pencil beam. Vertical- color shifts (Fitzgerald 2000). Also, ground-based hyperspectral looking radar (VLR) gives quantitative gauges of aerial insect remote sensing system was applied in pest management density, diversity, and biomass over a range of transient scales; it (Prabhakar et al. 2011; Rani and Jyothi 2017). For example, in this manner has incredible potential for monitoring insect pop- detection of leafhopper damages in cotton fields was performed ulation dynamics (Smith et al. 1993; Chapman et al. 2002). The by Prabhakar et al. (2011). A hyperspectral radiometer with a constrained recurrence extent of current insect radars has blocked spectral range of 350–2500 nm is used for estimating chlorophyll the procurement of more valuable data for the recognizable proof and relative water content was in selected plants. Reflectance of individual insects. In this concern, Hu et al. (2019) utilized measurements of healthy plants and leafhopper-infested ones multi-frequency radar in East China for recording the recurrence reveal significant difference values in near infrared (NIR) and reliance of 15 insect pests (having a place of 11 species and 3 visible regions (VIS). Chlorophyll A pigment shows significant families). The insect mass and body length can be extracted from decrease than chlorophyll B pigment in the infested plants. the multi-frequency RCSs (radar cross-section) with uncertainties Additionally, near-infrared spectroscopy (NIRS) is a promising of 16.31% and 10.74%, respectively. The thorax width was also method for the detection and identification of insects infesting estimated and aspect ratio is achieved with uncertainties of stored food products, and the rapid, low-cost and non-lethal pro- 13.37% and 7.99%, respectively. By analyzing the statistical data filing of cuticular hydrocarbons of individual insects (Johnson of 5532 insects representing 23 species, they concluded that the and Naiker 2019). right identification odds for all 23 species are greater than 0.5 and Moreover, in Egypt, remote sensing technology has be- reach more than 0.8 for 15 of the 23 species under the achievable come a part of entomological strategies for monitoring and measurement precision of the proposed technique. detecting the distribution of Neochetina eichhorinae Warner Entomological radar provides monitoring of migrant insect and N. bruchi Hustache weevils (biological control agent for populations (Smith et al. 1993; Brydegaard and Jansson water hyacinth) in Mariout and Edko lakes using multi- 2019). The high-altitude flight of migratory insects, beside spectral SPOT4 (Satellite Probatoire de l’Observation de la their generally little body measure and the truth that numerous Terre) imaging remote sensing technique (Fayad et al. species are nocturnal, implies that it is exceptionally trouble- 2003). The remote sensing technique using multi-spectral some to observe their movements. The study of insect migra- SPOT imaging was applied for detecting water hyacinth in- tion has noteworthy confinements and in fact challenging festation in the mentioned lakes before and after releasing when utilizing light traps and other ground-based observa- N. eichhorinae Warner and N. bruchi Hustache weevils. An tions, especially about high-altitude movement. In addition, obvious reduction in the water hyacinth infestation was mon- keeping up inspecting stages within the discus (e.g., flying itored after 1 year. The reduction in water hyacinth reaches machine, fastened inflatable) is costly and impracticable 30% and 34.35% of Mariout and Edko lakes, respectively. over long periods. Recently, Maggiora et al. (2019) developed Subsequently, GIS maps of insect vectors of malaria and fila- innovative harmonic radar effective in tracking insects flying riasis were adopted (Hassan et al. 2003). (Vespa velutina Lepeletier) at high altitude up to a range of Remote sensing capabilities of entomological radar provide 500 m which covers a large field of view in elevation. This monitoring abundance and dispersal of agricultural, medical, and radar system considered a potential tool for monitoring and forestry pests. It is used as opposed to insect sampling with controlling V. velutina Lepeletier by track the flight trajectory sweep nets and traps. The great advantages of radar are (a) its of insects and locate nests to be destroyed. unique capacity to detect insects simultaneously at a range of Monitoring and forecasting of different species of locust altitudes that can reach more than 1 km agl (Above Ground using various remote sensing technologies including entomo- Level) and (b) the large sampling volume that it provides relative logical radars and normalized difference vegetation index to traditional sampling methodologies (Chapman et al. 2002). (NDVI) satellite indicator were performed (Smith et al. Moreover, it provides an unparalleled opportunity to investigate 1993; Rani and Jyothi 2017; Hunt and Rondon 2017). This aspects of insect migration behavior, such as orientation in high- insect is harmful, especially during swarming and migration flying migrants (Reynolds and Riley 1997). The distinctive ca- for long distances causing severe damages to cultivated areas. pacity of radar to monitor nocturnal insects makes it widely used A single swarm can cover 1200 km2 and contains about 40–80 in the research of insect migrations. Different sorts of million locust/km2. More than eight million people were 33510 Environ Sci Pollut Res (2020) 27:33503–33515 affected during the 2003–2005 plague in West Africa. Losses change, is reported by Meigs et al. (2011). They used the were reported in cereals (100%), on legumes (90%), and on LandTrend algorithm over the Cascade Range in OR, USA, pasture (85%). To bring the plague under control, huge quan- to determine the mortality due to bark beetle and finding a tity of pesticides (13 million liters) and nearly $600 million significant relationship between field-based measures and were required after a good rain and in rapid vegetation growth Landsat spectral data. coming after a period of drought, locust swarming occurs. Remote sensing technologies (satellite imaging (Landsat- Satellites can monitor the conditions that can cause swarming SPOT-The Moderate Resolution Imaging Spectroradiometer of the locusts, such as soil moisture and green vegetation (MODIS)), Advanced Very High Resolution Radiometer which help to predict locust plagues. The body shape and (AVHRR) systems) were also applied for estimating popula- size are recorded by entomological radar data, plus tion fluctuation and mapping of some disease vector insects measurement of wing-beat frequency, which were intended such as mosquitoes, Anopheles sp., Culex sp., Aedes sp.; and to make the radar to detect the key task of distinguishing tsetse fly, Glossina morsitans Wiedemann (Hayes et al. 1985; desert locusts from other high-flying insects. The desert and Roger and Randolph 1991; NASA’S Godard Space Flight the Australian locusts using satellite information recorded Centre 2002; Lacaux et al. 2007). Furthermore, these proved to be a promising tool for management of such species technologies were applied for field crop insect pests. For (Sivanpillai and Latchininsky 2013). Recently, ESA’s satellite example, the corn leaf aphid, Rhopalosiphum maidis Fitch, cooperated with international partners from the UN Food and and sweet potato whitefly, Bemicia tabaci Glover, were Agriculture Organization (FAO), Algeria, France, Morocco, successfully detected using remote sensing technology Mauritania, Mali, and Spain to predict locust plagues (Fig. 4). depending on detectable sooty mold growing on as the Moreover, remote sensing was applied as a successful tool honeydew produced by these insect pests. In a case study, for monitoring, mapping, and controlling forest insect damages. good resolution of mold growth patterns was detected in a The aerial sketch mapping technique is effective for assessment photography of cotton fields from 300 to 2000 m above of forest health via color and color-infrared photos using low- (Acharya and Thapa 2015). flying aircraft. Also, other remote sensing tools such as airborne The list of remote sensing applications in the field of video, digital camera systems, and earth-observing satellites entomology has also included social insect pests. were used. Application of such remote sensing technologies Application of such tools was reported for various termite provides a good tool for monitoring and decreasing the growing species: subterranean and non-subterranean. These insect problems caused by bark beetle (Scolytus sp.), mountain pine pests have economic importance because of the great damage beetle (Dendroctonus ponderosae Hopkins), southern pine they cause in timber plantations, homes, furniture, and histor- beetle (D. frontalis Zimmermann), Douglas fir beetle (D. ical structures worldwide. Detection of termite infestation can pseudotsugae Hopkins), and spruce beetle (D. rufipennis be performed by acoustic signal and millimeter wave imaging. Kirby) on various conifers forests (Coops et al. 2006; This technique depends on the energy reflected from the body Robertson et al. 2008; Meddens et al. 2013; Hart and Veblen subjected to millimeter waves (non-ionizing electromagnetic 2015). A clear example of the relationship between field-based waves) at a frequency of 100 GHZ with 10-mW output power measures of pest damages, i.e., bark beetle and Landsat spectral (Yoshihisa et al. 2007). Fig. 4 Mapping of locust infestation for casting using soil moisture data outbreak appeared in red during November 2016) (European Space from the SMOS satellite and the MODIS instrument obtained during 4 Agency: https://phys.org/news/2017-06-satellites-forewarn-locust- months (July–October 2016) illustrated expected areas for locust plagues.html#jCp) infestation depending on favorable locust swarming conditions (locust Environ Sci Pollut Res (2020) 27:33503–33515 33511 Applications in plant disease management Recently, spectral sensors have been applied to contribute to site-specific disease management. For example, yellow rust dis- Security of crops against plant infections is significant. Logical ease detection and quantification in wheat crop was evaluated inquiry about agrarian hone is stood up to with modern chal- using the hyperspectral technology (Kuska and Mahlein 2018). lenges. Feasible and naturally neighborly arrangements are pro- In field experiments, a hyperspectral camera is mounted on two gressively requested. The precise detection of primary infection measurement platforms: (1) ground-based vehicle and (2) an sites and disease dynamics is fundamental to make a decision for unmanned aerial vehicle (UAV). These platforms allow discrim- a subsequent management practice. In this circumstance, optical ination of different developmental stages and severities of yellow sensors can give a precise and objective discovery of plant infec- rust disease in wheat field. Spectral sensors measure the light tions. In plant phenotyping, the optical sensor–based approaches reflected during a pathogen attack and disease developing on are considered a key element (Kuska and Mahlein 2018). the crop leaf that provides a spectral fingerprint in the reflected When electromagnetic energy of the sun strikes plants, leaf signature. These shifts of the signature from the crop canopy three things can happen: reflection, absorption, or transmis- can be detected using spectral sensors, particularly in the electro- sion. Relying on the wavelength of the energy and character- magnetic spectrum from 400–2500 nm. istics of individual plants, the energy reflected, transmitted, or Also, airborne imaging and high spatial resolution satellite absorbed can be detected by remote sensing. The differences devices have been used for examining some crop disease in leaf colors, textures, shapes, or even how the leaves are problems and assessing its impact on productivity (Qin and attached to plants can be determined by how much energy is Zhang 2005; Sankaran et al. 2010; Rani and Jyothi 2017; reflected, absorbed, or transmitted. Generally, the higher re- Zheng et al. 2018). For application of crop production and flection in the near-infrared region indicates healthy plants and protection materials, the outcome image can provide critical at least one in the visible region and opposite is the situation input leading to output prescription data (Huang et al. 2008). with the infected plants (Fig. 5). The plant stress generally Satellites, aircraft, or ground-based platforms are the tools of results in an increase in the visible reflectance of the plant remote sensing. For instance, detection and quantification of surface by reducing the chlorophyll level that decreased the site-specific wheat streak mosaic disease is recorded using the absorption of visible light (Rani et al. 2018). The first use of Landsat 5 TM image (Mirik et al. 2013) for wheat crop man- visible aerial photography in the detection of viral diseases agement. Moreover, aerial photography was used for prepar- was investigated for potato and tobacco crops by Bawden ing forest cover maps and measuring quantities of trees har- (1933). Colwell (1956), however, used infrared imaging to vested. Also, it was used for detection of diseases in forest discriminate against disease-related changes that occur in the trees. Generally, reactive field-by-field control, area wide pest cereal crop’s internal leaf structure. These mechanisms were management (APM) is a concept of preventive suppression of subsequently used for a broad spectrum of different crop dis- a pest species throughout its geographic distribution. The air- eases such as potato blight (Brenchley 1968), bacterial blight borne remote sensing was commonly used for the detection of beans (Jackson and Wallen 1975), cotton root rot (Toler and analysis of weed distribution (Huang et al. 2008). et al. 1981), and sheath blight on rice (Qin et al. 2003). Recently, radiance ratio and the normalized difference Fig. 5 Typical spectral curve of healthy plant (Rani et al. 2018) 33512 Environ Sci Pollut Res (2020) 27:33503–33515 Fig. 6 Spectral profiles of healthy, early, medium, and severely infested maize leaves (Dhau et al. 2018a) vegetation index (NDVI) systems are used for distinguished highlights the potential of remotely sensed data in the precise pure wheat and solid weed plots of Dwarf mallow, Malva discovery of editing infections such as MSV and their sever- neglecta Wallr (Kaur and Jaidka 2014). ity, which is fundamental in the editing of food safety obser- On Ofcolaco farm, Limpopo Province, Tzaneen, South vations, especially in the resource-limited sub-Saharan (Dhau Africa, to utilize further detecting methods for identifying et al. 2018a). RapidEye multi-spectral sensor combined with the Maize Streak Gemini virus (MSV), contaminated maize vegetation indices was utilized for recognizing and mapping was assessed. The ability of hyperspectral knowledge to clas- the Maize Streak Gemini virus (MSV)–infected maize (Fig.7). sify distinctive levels of MSV contamination in maize was Comes around appeared that the use of RapidEye unearthly evaluated on the basis of the guided regularized random forest bunches in the discovery and mapping of MSV showed an (GRRF) algorithm. GRRF algorithm can be utilized to viably expansive accuracy of 82.75%. In conclusion, the satellite- identify and select ideal wavelengths required to segregate based vegetation indices moved forward the classification pre- between distinctive levels of MSV trim diseases. The ideal cision. The classification handle utilizing RapidEye ghastly groups for recognizing diverse levels of maize streak infection groups appeared 82.75%, while the computed vegetation file in maize were 552 nm, 603 nm, 683 nm, 881 nm, and records advanced the classification precision by 3.4% (Dhau 2338 nm based on the GRRF algorithm (Fig. 6). This study et al. 2018b). Fig. 7 Map of infected maize and other land cover classification in Ofcolaco farm (Dhau et al. 2018b) Environ Sci Pollut Res (2020) 27:33503–33515 33513 Conclusion system of Mali. Remote Sens 8(6):531. https://doi.org/10.3390/ rs8060531 Brenchley GH (1968) Aerial photography for the study of plant diseases. In conclusion,the recent advance in farther detecting innova- Annu Rev Phytopathol 6:1–20. https://doi.org/10.1146/annurev.py. tion in remote sensing technology provides increasing and 06.090168000245 developing applications in different agricultural approaches, Brydegaard M, Jansson S (2019) Advances in entomological laser radar. The Journal of Engineering 2019:7542–7545. https://doi.org/10. especially in pests and plant disease management. The revo- 1049/joe.2019.0598 lution of information technology, interpretation, and data anal- Chapman JW, Smith AD, Woiwod IP, Reynolds DR, Riley JR (2002) ysis of such high-tech applications allows great advantages in Development of vertical-looking radar technology for monitoring growing and supporting the input knowledge which help de- insect migration. Comput Electron Agric 35(2-3):95–110. https:// doi.org/10.1016/S0168-1699(02)00013-3 velopment of many research fields. Recently, remote sensing Chuang Y-CM, Shiu Y-S (2016) A comparative analysis of machine is considered general-purpose data for a broad community of learning with WorldView-2 pan-sharpened imagery for tea crop users who may have diverse requirements. Remote sensing mapping. Sensors 16(5):594. https://doi.org/10.3390/s16050594 has become one of the most promising technologies that sup- Colwell RN (1956) Determining the prevalence of certain cereal diseases by means of aerial photography. Hilgardia 26(5):223–286 port integrated pest management via the possibility of the Coops NC, Johnson M, Wulder MA, White JC (2006) Assessment of investigation of different environmental parameters and avail- QuickBird high spatial resolution imagery to detect red attack dam- able natural sources as a complete system. However, develop- age due to mountain pine beetle infestation. Remote Sens Environ ment in designing, and operating of remote sensing systems to 103(1):67–80. https://doi.org/10.1016/j.rse.2006.03.012 decrease the cost and increase the quality of output imaging is Dhau I, Adam E, Mutanga O, Ayisi KK (2018a) Detecting the severity of maize streak virus infestations in maize crop using in situ a demand. hyperspectral data. TransR Soc S Afr 73(1):8–15. https://doi.org/ 10.1080/0035919X.2017.1370034 Authors’ contribution Abd El-Ghany NM had the idea for the review Dhau I, Adam E, Mutanga O, Ayisi KK, Mutanga O (2018b) Detection article. All authors performed the literature search. Abd El-Ghany NM and mapping of maize streak virus using RapidEye satellite imagery. wrote and drafted the paper, and Abd El-Ghany NM, Abd El- Aziz SE, Geocarto Int 34:856–866. https://doi.org/10.1080/10106049.2018. and Marei SS critically revised the work. 1450448 Dutta S, Singh SK, Panigrahy S (2014) Assessment of late blight induced diseased potato crops: a case study for West Bengal district using Compliance with ethical standards temporal AWiFS and MODIS data. J Indian Soc Remote Sens 42(2):353–361. https://doi.org/10.1007/s12524-013-0325-9 Conflict of interest The authors declare that they have no conflict of Everitt JH, David E, Kenneth S, Mario A, Michael D (1996) Using spatial interest. information technologies for detecting and mapping whitefly and harvester ant infestations in south Texas. Southwest Entomol 21(4):421–432 https://www.researchgate.net/publication/ 285540383_Using_spatial_information_technologies_for_ References detecting_and_mapping_whitefly_and_harvester_ant_infestations_ in_South_Texas Abdullah A, Umer MZ (2004) Applications of remote sensing in pest Fayad YH, Badawy M, Wahby A (2003) Monitoring the biological con- scouting: evaluating options and exploring possibilities. Proc VII trol success of water hyacinth using multispectral SPOT images. Int Conf on Precision Agriculture and Other Precision Resources Egypt J Biol Pest Co1 3(1):35–39 Management, Hyatt Regency, Minneapolis, MN, St. Paul, MN: Fernando V, Dmitry B, Kevin P, John W, Felipe G (2018) A novel Precision Agriculture Center, University of Minnesota (USA), July methodology for improving plant pest surveillance in vineyards 25–28 pp:14. https://www.researchgate.net/publication/265996671 and crops using UAV-based hyperspectral and spatial data. Acharya MC, Thapa RB (2015) Remote sensing and its application in Sensors 8(1):260–281. https://doi.org/10.3390/s18010260 agricultural pest management. J Agric Environ 6:43–61. https://doi. Fitzgerald G (2000) Bug checking for mites – from the sky. Australian org/10.3126/aej.v16i0.19839 CottonGrower 21(1):29–31 Al-Kindi KM, Kwan P, Andrew NR, Welch M (2017) Remote sensing Fletcher RS, Everitt JH (2007) A six-camera digital video imaging system and spatial statistical techniques for modelling Ommatissus lybicus sensitive to visible, red edge, near-infrared, and mid-infrared wave- (Hemiptera: Tropiduchidae) habitat and population densities. Peer J lengths. Geocarto Int 22(2):75–86. https://doi.org/10.1080/ 5:e3752. https://doi.org/10.7717/peerj.3752 10106040701201608 Andreo V (2013) Remote sensing and geographic information systems in Franke J, Menz G (2007) Multi-temporal wheat disease detection by precision farming. Technical report available: http://aulavirtual.ig. multi-spectral remote sensing. Precis Agric 8(3):161–172. https:// conae.gov.ar /moodle/pluginfile.php/513/mod_page/content/71/ doi.org/10.1007/s11119-007-9036-y seminario_andreo_2013.pdf (Retrieved 16 April 2015) pp. 34. Hart SJ, Veblen TT (2015) Detection of spruce beetle-induced tree mor- Avery TE, Berlin GL (1992) Fundamentals of remote sensing and tality using high-and medium-resolution remotely sensed imagery. airphoto interpretation, 5th edn, Prentice Hall, p 472. https://www. Remote Sens Environ 168:134–145 flipkart.com/fundamentals-remote-sensing-airphoto-interpretation- Hart WG, Ingle SJ, Davis MR, Mangum C (1973) Aerial photography 5th-edition-5-ed/p/itmczymfk6w3jwh6 with infrared color film as a method of surveying for citrus blackfly. Bawden FC (1933) Infra-red photography and plant virus diseases. J Econ Entomol 66(1):190–194. https://doi.org/10.1093/jee/66.1. Nature 132:168. https://doi.org/10.1038/132168a0 190 Blaes X, Chomé G, Lambert M-J, Traoré PS, Schut AGT, Defourny P Hassan A, Kenawy MA, Kamal H, Abdel Sattar AA, Sowilem MM (2016) Quantifying fertilizer application response variability with (2003) GIS-based prediction of malaria risk in Egypt. East VHR satellite NDVI time series in a rainfed smallholder cropping Mediterr Health J (La Revue de Santé de la Méditerranée orientale) 33514 Environ Sci Pollut Res (2020) 27:33503–33515 9(4 ):5 48 –5 58. https:/ /pdfs.sema nti cscholar.org/ f2b3/ mortality and surface fuels in conifer forests. Remote Sens c080855306c33acdf817bdac73a8a1dd522d.pdf. Accessed 21 Environ 115:3707–3718. https://doi.org/10.1016/j.rse.2011.09.009 Feb 2005 Mirik M, Ansley RJ, Price JA, Workneh F, Rush CM (2013) Remote Hayes RO, Maxwell EL, Mitchell CJ, Woodzik TL (1985) Detection, monitoring of wheat streak mosaic progression using sub-pixel clas- identification, and classification of mosquito larval habitats using sification of Landsat 5 TM imagery for site specific disease man- remote sensing scanners in earth-orbiting satellites. Bull World agement in winter wheat. Adv Remote Sens 2:16–28. https://doi. Health Organ 63(2):361–374 https://www.ncbi.nlm.nih.gov/pmc/ org/10.4236/ars.2013.21003 articles/PMC2536406/ Morales FJ, Jones PG (2004) The ecology and epidemiology of whitefly- Hobbs SE, Wolf WW (1989) An airborne radar technique for studying transmitted viruses in Latin America. Virus Res 100(1):57–65. insect migration. Bull Entomol Res 79(4):693–704. https://doi.org/ https://doi.org/10.1016/j.virusres.2003.12.014 10.1017/S000748530001885X Nansen C, Elliott N (2016) Remote sensing and reflectance profiling in Hu C, Deng YK, Wang R, Liu CJ, Long T (2016) High accuracy acqui- entomology. Annu Rev Entomol 61:139–158. https://doi.org/10. sition of 3-d flight trajectory of individual insect based on phase 1146/annurev-ento-010715-023834 measurement. Sensors 16(12):2166. https://doi.org/10.3390/ NASA’S Godard Space Flight Centre (2002) NASA researchers devel- s16122166 oping tools to help track and predict West Nile Virus. www.nasa. Hu C, Kong S, Wang R, Zhang F (2019) Radar measurements of mor- gov. 8 October2002. phological parameters and species identification analysis of migra- Natural Resources Canada (2019) https://www.nrcan.gc.ca/earth- tory insects. Remote Sens 11(17):1977. https://doi.org/10.3390/ sciences/geomatics/satellite-imagery-air-photos/satellite-imagery- rs11171977 products/educational-resources/9363 Huang Y, Lan YC, Hoffmann W (2008) Use of airborne multi-spectral Nilsson HE (1995) Remote sensing and image analysis in plant patholo- imagery for area wide pest management. Agric Eng Int: CIGR gy. Annu Rev Phytopathol 33:489–528. https://doi.org/10.1146/ Ejournal, Manuscript IT 07010:14 http://www.cigrjournal.org/ annurev.py.33.090195.002421 index.php/Ejounral/article/view/1019/1012. Accessed Feb 2008 Odi-Lara M, Campos I, Neale CMU, Ortega-Farías S, Poblete-Echeverría Hunt JER, Rondon SI (2017) Detection of potato beetle damage using C, Balbontín C, Calera A (2016) Estimating evapotranspiration of remote sensing from small unmanned aircraft systems. J Appl an apple orchard using a remote sensing-based soil water balance. Remote Sens 11(2):026013. https://doi.org/10.1117/1.JRS.11. Remote Sens 8(3):253. https://doi.org/10.3390/rs8030253 026013 Oumar Z, Mutanga O (2011) The potential of remote sensing technology Jackson HR, Wallen VR (1975) Microdensitometer measurements of for the detection and mapping of Thaumastocoris peregrinus in plan- sequential aerial photographs of field beans infected with bacterial tation forests. South Forests: J Forest Sci 73(1):23–31. https://doi. blight. Phytopathol 65:961–968 https://www.apsnet.org/ org/10.2989/20702620.2011.574814 publications/phytopathology/backissues/Documents/1975Articles/ Peralta NR, Assefa Y, Du J, Barden CJ, Ciampitti IA (2016) Mid-season Phyto65n09_961.PDF high-resolution satellite imagery for forecasting site-specific corn Johansen K, Sallam N, Robson A, Samson P, Chandler K, Derby L, yield. Remote Sens 8:848–864. https://doi.org/10.3390/rs8100848 Eaton A, Jennings J (2018) Using GeoEye-1 imagery for multi- Piekarczyk J (2014) Application of remote sensing in agriculture. temporal object-based detection of canegrub damage in sugarcane Geoinformatica Polonica 13:69–75. https://doi.org/10.2478/gein- fields in Queensland, Australia. GISci Remote Sen 55(2):285–305. 2014-0007 https://doi.org/10.1080/15481603.2017.1417691 Prabhakar M, Prasad YG, Thirupathi M, Sreedevi G, Dharajothi B, Johnson JB, Naiker M (2019) Seeing red: a review of the use of near- Venkateswarlu B (2011) Use of ground based hyperspectral remote infrared spectroscopy (NIRS) in entomology. Appl Spectrosc Rev: sensing for detection of stress in cotton caused by leafhopper 1–30. https://doi.org/10.1080/05704928.2019.1685532 (Hemiptera: Cicadellidae). Comput Electron Agric 7(9):189–198. Kaur R, Jaidka M (2014) Spectral reflectance characteristics to distin- https://doi.org/10.1016/j.compag.2011.09.012 guish Malva neglecta in wheat (Triticum aestivum). Indian J Agric Prasad YG, Prabhakar M (2012) Pest monitoring and forecasting. In: Sci 84(10):1243–1249. http://epubs.icar.org.in/ejournal/index.php/ Abrol DB, Shankar U (eds) Integrated Pest Management. CAB IJAgS/article/view/44208/19470. Accessed Oct 2014 International, Wallingford, pp 41–57 Kuska MT, Mahlein A-K (2018) Aiming at decision making in plant Pretorius ZA, Lan CX, Prins R, Knight V, McLaren NW, Singh RP, disease protection and phenotyping by the use of optical sensors. Bender CM, Kloppers FJ (2017) Application of remote sensing to Eur J Plant Pathol 152(4):987–992. https://doi.org/10.1007/s10658- identify adult plant resistance loci to stripe rust in two bread wheat 018-1464-1 mapping populations. Precis Agric 8(4):411–428. https://doi.org/10. Lacaux JP, Tourre YM, Vignolle C, Ndione JA, Lafayet M (2007) 1007/s11119-016-9461-x Classification of ponds from high-spatial resolution remote sensing: Qin Z, Zhang M (2005) Detection of rice sheath blight for in-season application to Rift Valley fever epidemic in Senegal. Remote Sens disease management using multispectral remote sensing. Int J Environ 106(1):66–74. https://doi.org/10.1016/j.rse.2006.07.012 Appl Earth Obs 7(2):115–128. https://doi.org/10.1016/j.jag.2005. Maggiora R, Saccani M, Milanesio D, Porporato M (2019) An innovative 03.004 harmonic radar to track flying insects: the case of Vespa velutina. Sci Qin Z, Zhang M, Christensen T, Li W, Tang H (2003) Remote sensing Rep 9:11964. https://doi.org/10.1038/s41598-019-48511-8 analysis of rice disease stresses for farm pest management using Malinowski R, Groom G, Schwanghart W, Heckrath G (2015) Detection wide-band airborne data. In Proc IEEE International Geoscience and delineation of localized flooding from WorldView-2 multispec- and Remote Sensing Symposium. pp: 2215-2217 https://doi.org/ tral data. Remote Sens 7(11):14853–14875. https://doi.org/10.3390/ 10.1109/IGARSS.2003.1294393 rs71114853 Rani AS, Jyothi S (2017) A study on hyper spectral remote sensing pest Meddens AJH, Hicke JA, Vierling LA, Hudak AT (2013) Evaluating management. Int J Recent Innov Trends Comput Commun 5(6): methods to detect bark beetle-caused tree mortality using single- 497–503. http://www.ijritcc.org/index.php/ijritcc/issue/view/8. date and multi-date Landsat imagery. Remote Sens Environ 132: Accessed June 2017 49–58. https://doi.org/10.1016/j.rse.2013.01.002 Rani DS, Venkatesh MN, SriCh NS, Kumar KA (2018) Remote sensing Meigs GW, Kennedy RE, Cohen WB (2011) A Landsat time series ap- as pest forecasting model in agriculture. Int J Curr Microbiol Appl proach to characterize bark beetle and defoliator impacts on tree Sci 7(3):280–2689. https://doi.org/10.20546/ijcmas.2018.703.310 Environ Sci Pollut Res (2020) 27:33503–33515 33515 Reynolds DR, Riley JR (1997) The flight behaviour and migration of Yang C (2012) A high-resolution airborne four-camera imaging system insect pests: radar studies in developing countries. Chatham: for agricultural remote sensing. Comput Electron Agric 88:13–24. Natural Resources Institute. NRI Bulletin no. 71. https://doi.org/10.1016/j.compag.2012.07.003 Riley JR, Reynolds DR, Smith AD, Edwards AS, Zhai BP (1995) Yang C, Everitt JH (2011) Remote sensing for detecting and mapping Observations of the autumn migration of the rice leaf roller whitefly (Bemisia tabaci) infestations, In: Thompson, Winston, cnaphalocrocis medinalis (Lepidoptera: Pyralidae) and other moths M.O. (Eds.), The Whitefly, Bemisia tabaci (Homoptera: in eastern china. Bull Entomol Res 85(3):397–414. https://doi.org/ Aleyrodidae) Interaction with Geminivirus-Infected Host Plants: 10.1017/S0007485300036130 Bemisia tabaci, Host Plants and Geminiviruses. Springer, Robertson C, Wulder M, Nelson T, White J (2008) Risk rating for moun- Netherlands. pp: 357-381. https://doi.org/10.1007/978-94-007- tain pine beetle infestation of lodgepole pine forests over large areas 1524-0_13 with ordinal regression modelling. Forest Ecol Manag 256:900– Yang C, Everitt JH, Davis MR, Mao C (2003) A CCD camera-based 912. https://doi.org/10.1016/j.foreco.2008.05.054 hyperspectral imaging system for stationary and airborne applica- Roger D, Randolph SE (1991) Mortality rates and population density of tions. Geocarto Int 18(2):71–80. https://doi.org/10.1080/ Tsetse flies correlated with satellite imagery. Nature 351(6329): 10106040308542274 734–741. https://doi.org/10.1038/351739a0 Yang C, Everitt JH, Bradford JM, Murden D (2009a) Comparison of Sankaran S, Mishra A, Ehsani R, Davis C (2010) A review of advanced airborne multispectral and hyperspectral imagery for estimating techniques for detecting plant diseases. Comput Electron Agric grain sorghum yield. Trans ASABE 52(2):641–649. https://doi. 72(1):1–13. http://128.227.177.113/pa/Publications/Sankaran_ org/10.13031/2013.26816)@2009 Mishra_Ehsani_Davis_2010.pdf. Accessed June 2010 Yang Z, Rao MN, Elliott NC, Kindler SD, Popham TW (2009b) Senf C, Seidl R, Hostert P (2017) Remote sensing of forest insect distur- Differentiating stress induced by greenbugs and Russian wheat bances: Current state and future directions. Int J Appl Earth Obs 60: aphids in wheat using remote sensing. Comput Electron Agric 49–60. https://doi.org/10.1016/j.jag.2017.04.004 67(1):64–70. https://doi.org/10.1016/j.compag.2009.03.003 Sivanpillai R, Latchininsky AV (2013) Publisher’s Note: Special section Yoshihisa F, Yoko F, Yoshiyuk Y, Shogo O, Koichi N, Tadao N, Tsyoshi on advances in remote sensing applications for locust habitat mon- Y, Yuji I (2007) Nondestructive detection of termites using a itoring and management. J Appl Remote Sens 7(1):075001.33. millimeter-wave imaging technique. Forest Prod J 57(10):75–79 https://doi.org/10.1117/1.JRS.7.075001 https://www.engineeringvillage.com/search/quick.url? Smith AD, Riley JR, Gregory RD (1993) A method for routine monitor- SEARCHID=a1a4044128cd4ee3bbcab7962df9da70&COUNT= ing of the aerial migration of insects by using a vertical looking 1&usageOrigin=&usageZone=#foo redar. Philos Trans R Soc Lond B 340:393–404. https://doi.org/10. Zhang J, Yang C, Song H, Hoffmann WC, Zhang D, Zhang G (2016) 1098/rstb.1993.0081 Evaluation of an airborne remote sensing platform consisting of two Toler RW, Smith BD, Harlan JC (1981) Use of aerial color infrared to consumer-grade cameras for crop identification. Remote Sens 8(3): evaluate crop disease. Plant Dis 65(1):24–31. https://doi.org/10. 257. https://doi.org/10.3390/rs8030257 1094/pd-65-24 Wang Y, Nansen C, Zhang Y (2016) Integrative insect taxonomy based Zhang J, Wang X, Yang C, Zhang J, He D, Song H (2018) Image on morphology, mitochondrial DNA and hyperspectral reflectance dehazing based on dark channel prior and brightness enhancement profiling. Zool J Linnean Soc 177:378–394. https://doi.org/10.1111/ for agricultural remote sensing images from consumer-grade cam- zoj.12367 eras. Comput Electron Agric 151:196–206. https://doi.org/10.1016/ Westbrook JK, Eystera RS, Wolf WW, Lingrena PD, Raulstonb JR j.compag.2018.06.010 (1995) Migration pathways of corn earworm (Lepidoptera: Zheng Q, Huang W, Cui X, Shi Y, Liu L (2018) New spectral index for Noctuidae) indicated by tetroon trajectories. Agric For Meteorol detecting wheat yellow rust using sentinel-2 multispectral imagery. 73(1):67–87. https://doi.org/10.1016/0168-1923(94)02171-F Sensors 18(3):868–887. https://doi.org/10.3390/s18030868 Yan Y, Feng C-C, Chang KTT (2017) Towards enhancing integrated pest management based on volunteered geographic information. Int J Publisher’s note Springer Nature remains neutral with regard to jurisdic- Geo Inform 6(7):224. https://doi.org/10.3390/ijgi6070224 tional claims in published maps and institutional affiliations.