Robin Hood Effects on Math Motivation (2017) - PDF
Document Details
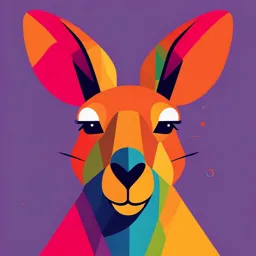
Uploaded by AccommodativeMeadow
2017
Isabelle Häfner, Barbara Flunger, Anna-Lena Dicke, Hanna Gaspard, Brigitte M. Brisson, Benjamin Nagengast, and Ulrich Trautwein
Tags
Summary
This study investigates the impact of relevance interventions on math motivation, particularly considering the role of family interest. Researchers tracked students' motivational beliefs after interventions and found that these interventions were particularly effective for students with lower levels of family math interest, highlighting the potential for "Robin Hood" effects. The study used data from German 9th-grade math classrooms.
Full Transcript
Developmental Psychology 2017, Vol. 53, No. 8, 1522–1539 © 2017 American Psychological Association 0012-1649/17/$12.00 http://dx.doi.org/10.1037/dev0000337 Robin Hood Effects on Motivation in Math: Family Interest Moderates the Effects of Relevance Interventions Isabelle Häfner and Barbara Flunger A...
Developmental Psychology 2017, Vol. 53, No. 8, 1522–1539 © 2017 American Psychological Association 0012-1649/17/$12.00 http://dx.doi.org/10.1037/dev0000337 Robin Hood Effects on Motivation in Math: Family Interest Moderates the Effects of Relevance Interventions Isabelle Häfner and Barbara Flunger Anna-Lena Dicke University of Tübingen University of California, Irvine Hanna Gaspard, Brigitte M. Brisson, Benjamin Nagengast, and Ulrich Trautwein This document is copyrighted by the American Psychological Association or one of its allied publishers. This article is intended solely for the personal use of the individual user and is not to be disseminated broadly. University of Tübingen Using a cluster randomized field trial, the present study tested whether 2 relevance interventions affected students’ value beliefs, self-concept, and effort in math differently depending on family background (socioeconomic status, family interest (FI), and parental utility value). Eighty-two classrooms were randomly assigned to either 1 of 2 intervention conditions or a control group. Data from 1,916 students (Mage ⫽ 14.62, SDage ⫽ 0.47) and their predominantly Caucasian middle-class parents were obtained via separate questionnaires. Multilevel regression analyses with cross-level interactions were used to investigate differential intervention effects on students’ motivational beliefs 6 weeks and 5 months after the intervention. Socioeconomic status, FI, and parental utility values were investigated as moderators of the intervention effects. The intervention conditions were especially effective in promoting students’ utility, attainment, intrinsic value beliefs, and effort 5 months after the intervention for students whose parents reported lower levels of math interest. Furthermore, students whose parents reported low math utility values especially profited in terms of their utility and attainment math values 5 months after the intervention. No systematic differential intervention effects were found for socioeconomic status. These results highlight the effectiveness of relevance interventions in decreasing motivational gaps between students from families with fewer or more motivational resources. Findings point to the substantial importance of motivational family resources, which have been neglected in previous research. Keywords: utility value, expectancy-value theory, cluster randomized controlled trial, family background, socioeconomic status Supplemental materials: http://dx.doi.org/10.1037/dev0000337.supp Why are some students motivated to learn whereas others are not? One factor associated with interindividual differences in students’ motivation to learn is their family background (Eccles, 2005, 2007). Family background is often defined as a set of structural family characteristics such as its socioeconomic status (SES) and family members’ migration background. A plethora of studies focusing on structural family characteristics demonstrated that students from families with low SES show lower levels of motivation and achievement (e.g., Dotterer, McHale, & Crouter, 2009; Dumont et al., 2012; Sirin, 2005; Steinmayr, Dinger, & Spinath, 2012). Alternatively, recent studies highlight the importance of conceptualizing family background as a set of process related characteristics such as motivational resources within a family (Eccles, 2007; Grolnick, Friendly, & Bellas, 2009). In particular, if parents hold low motivational beliefs toward a specific subject, their children are at risk to develop Isabelle Häfner and Barbara Flunger, Hector Research Institute of Education Sciences and Psychology, University of Tübingen; Anna-Lena Dicke, School of Education, University of California, Irvine; Hanna Gaspard, Brigitte M. Brisson, Benjamin Nagengast, and Ulrich Trautwein, Hector Research Institute of Education Sciences and Psychology, University of Tübingen. Barbara Flunger is now at the Department of Social and Behavioural Sciences, Utrecht University. Brigitte M. Brisson is now at the Department of Educational Quality and Evaluation, German Institute for International Educational Research in Frankfurt am Main. This research was funded in part by German Research Foundation Grant TR 553/7-1 awarded to Ulrich Trautwein, Oliver Lüdtke, and Benjamin Nagengast. In addition, this research project was supported by the Postdoc Academy of the Hector Research Institute of Education Sciences and Psychology, University of Tübingen, funded by the Baden-Württemberg Ministry of Science, Education and the Arts. Hanna Gaspard, Brigitte M. Brisson, and Isabelle Häfner were members of the Cooperative Research Training Group of the University of Education, Ludwigsburg, and the University of Tübingen, which was financed by the Ministry of Science, Research and the Arts in BadenWürttemberg. Hanna Gaspard and Isabelle Häfner are also members of the LEAD Graduate School & Research Network (GSC1028), funded by the Excellence Initiative of the German federal and state governments. We thank Katharina Allgaier and Evelin Herbein for their help conducting this research. Correspondence concerning this article should be addressed to Isabelle Häfner, Hector Research Institute of Education Sciences and Psychology, University of Tübingen, Europastr. 6, 72072 Tübingen, Germany. E-mail: [email protected] 1522 This document is copyrighted by the American Psychological Association or one of its allied publishers. This article is intended solely for the personal use of the individual user and is not to be disseminated broadly. ROBIN HOOD EFFECTS low interest and values for the respective subject as well (Frenzel, Goetz, Pekrun, & Watt, 2010; Lazarides, Harackiewicz, Canning, Pesu, & Viljaranta, 2015; Simpkins, Fredricks, & Eccles, 2012). Despite these important findings, there is a lack of knowledge about potential remedies. One arguably promising avenue is the use of motivational interventions. Several recent studies indicate that relevance interventions can successfully promote student motivation by raising students’ perceptions of the usefulness of a task or subject (Hulleman, Godes, Hendricks, & Harackiewicz, 2010; Hulleman & Harackiewicz, 2009). It remains unclear, however, whether these interventions are also a good means to decrease motivational gaps between students from families with more motivational resources and those from families with fewer resources. On the one hand, students with better initial conditions might benefit more strongly from relevance interventions, due to their initial advantage in motivational resources (e.g., Durik, Hulleman, & Harackiewicz, 2015), thereby increasing gaps between over- and underprivileged students (Ceci & Papierno, 2005). This phenomenon is also known as the Matthew effect (Walberg & Tsai, 1983; the Bible states, “For whosoever hath, to him shall be given, and he shall have more abundance...” [Matthew 13:12, King James version]). On the other hand, Harackiewicz, Tibbetts, Canning, and Hyde (2014) recently argued that relevance interventions might be especially effective for students growing up in families with fewer resources (see also Harackiewicz, Canning, Tibbetts, Priniski, & Hyde, 2016), a phenomenon that we call the Robin Hood effect. Just as Robin Hood gave resources to the ones in need, relevance interventions might pass on important utility information to students in need—students from families with fewer motivational resources. Moreover, if relevance interventions create Robin Hood effects, it remains unclear if they occur immediately or over time. Students from families with low motivational resources might receive new utility information during the intervention. These students might process this utility information more deeply and look for the relevance of math in their everyday life, leading to cascading effects of the intervention on their utility value beliefs over time. Thus, sleeper effects (responses that may not surface for several weeks or months; Kagan & Moss, 1962) might occur because of small changes in the beginning leading to recursive intervention effects over time for students with lower resources (Cohen et al., 2009). The aim of the present study was, thus, to investigate whether the effects of two relevance intervention conditions (either writing a text or evaluating interview quotations) differed depending on students’ family background (both SES and motivational family resources) producing either a Matthew effect or a Robin Hood effect. We used data from the Motivation in Mathematics (MoMa) study, in which two relevance interventions were implemented in a cluster randomized controlled study design with 82 German ninth grade math classrooms. Prior analyses have shown these relevance interventions to improve students’ motivational beliefs (Brisson et al., in press; Gaspard, Dicke, Flunger, Brisson, et al., 2015). Extending these findings, we examined differential effects of these two relevance interventions on a variety of students’ motivational outcomes depending on parent-reported structural and motivational family resources. More specifically, we investigated effects 6 weeks and 5 months after the relevance interven- 1523 tions had taken place, which allowed us to differentiate between short-term effects and potential sleeper effects (Kagan & Moss, 1962). Family Factors and Students’ Academic Outcomes Coleman et al. (1966) concluded that the most crucial factor for students to be successful in school was family background. Although more recent research also highlights the powerful effects of various factors at the school, classroom, and teacher level (Hattie, 2009), an overwhelming number of studies have demonstrated the importance of family background for students’ academic outcomes (see meta-analysis by Sirin, 2005). Most studies investigating the effects of students’ family background focused on so-called structural family resources (e.g., SES) that characterize a family’s economic and social position within societal structures and are associated with students’ academic outcomes (see Sirin, 2005). Students from families with a lower SES tend to live in less motivating and cognitively activating environments and, thus, have been found to show lower academic motivation and achievement (e.g., Dumont et al., 2012; Dumont, Trautwein, Nagy, & Nagengast, 2014; McLoyd, 1998; Sirin, 2005; Steinmayr et al., 2012). In addition to structural family resources, recent research has identified motivational family resources as one important factor. For instance, motivational beliefs within a family have been shown to shape students’ academic outcomes (Eccles, 2007; Grolnick et al., 2009). Compared with structural family resources such as SES, motivational beliefs within a family are more proximal to the child and directly influence the processes within a family (Bronfenbrenner & Morris, 2006). As motivational beliefs within a family shape students’ environment more directly, they might be even more important for students’ academic outcomes than SES (Dumont et al., 2012; Grolnick et al., 2009; Häfner, Flunger, et al., 2017). Eccles et al. (1983) expectancy-value theory (EVT) and more specifically, the parent socialization model (Eccles, 2007; Jacobs & Eccles, 2000) embedded within EVT, suggest that parents act as role models and thus pass on their own motivational beliefs to their children (Jacobs & Eccles, 2000). Motivational beliefs within a family include parents’ motivational beliefs about their own values and competence beliefs as well as the more general motivational climate within the family. In more detail, EVT assumes that parents as well as students hold distinct value beliefs regarding a task and competence beliefs about the probability of mastering a task. Value beliefs can be separated into four different components: utility, attainment, intrinsic value, and cost. Utility value describes the perceived individual usefulness of a task, whereas attainment value is defined as the importance of mastering a task. Intrinsic value indicates the enjoyment of doing a task, and cost is described as the negative consequences of engaging in a task, including opportunity cost, effort required, and negative emotions associated with the task (Eccles & Wigfield, 2002). Students’ domain-specific competence beliefs can be operationalized as students’ ability self-concepts describing students’ evaluation of their competence in a domain (Eccles et al., 1983; Eccles & Wigfield, 2002). Regarding motivational family resources, two motivational beliefs are important for the development of students’ motivational beliefs. First, family interest (FI) refers to the general motivational attitudes toward a subject within the family (e.g., Frenzel et al., 2010). If families are This document is copyrighted by the American Psychological Association or one of its allied publishers. This article is intended solely for the personal use of the individual user and is not to be disseminated broadly. 1524 HÄFNER ET AL. interested in math, these families might enjoy math-related activities together, talk more about math, and thus pass on their interest to their children (e.g., Dabney, Chakraverty, & Tai, 2013). Therefore, if parents like a subject, chances are high that the child also likes the subject (Dotterer et al., 2009; Tenenbaum & Leaper, 2003). Second, parents’ utility values have been shown to affect students’ utility value beliefs and other academic outcomes (Harackiewicz, Rozek, Hulleman, & Hyde, 2012; Lazarides et al., 2015; Rozek, Hyde, Svoboda, Hulleman, & Harackiewicz, 2015). If parents hold high math utility beliefs, they might be more capable of elaborating on the usefulness of math and talk more about it to their child (e.g., Hyde et al., in press). To conclude, FI and parents’ utility value beliefs are two central motivational family resources. Interventions Targeting Students’ Relevance Beliefs Previous research has shown that students’ motivational beliefs in mathematics decrease during secondary school (Jacobs, Lanza, Osgood, Eccles, & Wigfield, 2002; Watt, 2004). Students’ motivational beliefs, however, are important predictors of students’ academic effort, achievement, and choices (e.g., Nagengast, Trautwein, Kelava, & Lüdtke, 2013; Simpkins, Davis-Kean, & Eccles, 2006; for a review, see Wigfield, Tonks, & Klauda, 2009). Researchers have, thus, started to develop interventions to buffer against the decrease of student motivation (see Karabenick & Urdan, 2014). Several types of interventions from different theoretical backgrounds have been found to foster students’ academic outcomes (for an overview, see Karabenick & Urdan, 2014; Yeager & Walton, 2011). One of these approaches is based on EVT and addresses subject-specific motivational beliefs such as utility value, to foster students’ subject-specific motivation (for an overview, see Harackiewicz et al., 2014; Rosenzweig & Wigfield, 2016). Compared with intrinsic and attainment value, utility value is more extrinsic in nature. Because the utility value of a task relates to future goals, the task itself is not necessarily valued for its own sake (Eccles & Wigfield, 2002). Thus, utility value beliefs can be influenced through interventions by highlighting the relevance of a subject for possible future careers, occupations, and everyday life (e.g., Woolley, Rose, Orthner, Akos, & JonesSanpei, 2013). Several studies demonstrated the success of interventions promoting students’ utility value beliefs in enhancing students’ self-concept, interest, effort, and achievement in psychology, mathematics, and science (Brisson et al., in press; Durik & Harackiewicz, 2007; Gaspard, Dicke, Flunger, Brisson, et al., 2015; Hulleman et al., 2010; Woolley et al., 2013). Two different approaches have been used to increase students’ utility value beliefs in these interventions (Canning & Harackiewicz, 2015; Durik et al., 2015). Students were either provided with information about the relevance of a learning task for their own life (see Study 2 in Durik & Harackiewicz, 2007; Woolley et al., 2013) or were encouraged to self-generate reasons for the relevance of a task by writing an essay (Hulleman et al., 2010; Hulleman & Harackiewicz, 2009). Are relevance interventions also a means to reduce motivational gaps between students with distinct family backgrounds? And if so, how stable are these effects? According to Ceci and Papierno (2005), two reasons often lead interventions to result in Matthew effects: First, utilization-based benefits can result for students with better initial conditions, due to higher access of this group to the intervention. Second, performance-based benefits can result for students with better initial conditions, if the intervention itself builds on prior knowledge (of which students with better initial conditions tend to have more). As the intervention under investigation was administered in the classroom (thus, enabling all students to participate) and did not require any specific prior knowledge, finding Matthew effects was unlikely. Moreover, some evidence suggests particularly strong ameliorative effects of relevance interventions for students from families with fewer resources: Harackiewicz et al. (2016) found a relevance (i.e., utility value) intervention in college to be especially helpful for underrepresented minority students which were also first generation students. Yet, the sample in the current study is rather distinct from the sample in the Harackiewicz et al. (2016) study so that other sample characteristics might play an important role. Thus, relevance interventions might create Robin Hood effects for students from families with low motivational resources: During the intervention, these students might receive relevant utility information, which they are not exposed to in their families because of fewer motivational resources. Students from families with fewer motivational resources could, thus, profit most from relevance interventions. It is unclear if Robin Hood effects—the decrease of motivational gaps between students from families with more motivational resources and those from families with fewer resources— occur immediately or over time. Students from families with high motivational resources might have been exposed to information about the relevance of math within their families, whereas this information might be new for students from families with lower motivational resources. Processing of new information differs from the processing of familiar information (e.g., Hasselmo & Stern, 2006), with processing of novel information leading to higher attention and thus eliciting deeper information processing over time (e.g., Berlyne, 1960; Petty & Cacioppo, 1986). Students from families with low motivational resources might process new utility information more deeply and start to reflect more on the usefulness of math looking for the relevance of math in their everyday life. This would lead to cascading effects of the intervention on their utility value beliefs over time. Similarly, Cohen, Garcia, Purdie-Vaughns, Apfel, and Brzustoski (2009) suggested that small psychological changes in the beginning can lead to recursive processes for students with lower resources. Therefore, follow-up designs are necessary to uncover potential sleeper effects (Kagan & Moss, 1962). The Present Study In the current research, we reanalyzed data from a large (82 ninth grade classes) cluster randomized controlled intervention study. Previous analyses demonstrated that the two relevance interventions (text condition [self-generating a text about the usefulness of mathematics] vs. quotations condition [evaluating quotations about the usefulness of mathematics]) successfully promoted students’ value beliefs, competence beliefs, effort, and achievement (see Brisson et al., in press; Gaspard, Dicke, This document is copyrighted by the American Psychological Association or one of its allied publishers. This article is intended solely for the personal use of the individual user and is not to be disseminated broadly. ROBIN HOOD EFFECTS Flunger, Brisson, et al., 2015) up to 5 months after the intervention. The present study investigates whether the effects of these two relevance interventions on students’ motivation in math were moderated by family background. In detail, we investigated parent-reported FI and parental utility values as moderators next to SES, because prior research has shown that these indicators are highly relevant for students’ motivation. In addition, students whose parents hold low utility beliefs might profit most from the intervention, as these students might receive new utility information during the intervention, which they did not receive from their parents. Thus, we chose parents’ utility value beliefs as a potential moderator. Several crucial motivational variables including students’ value beliefs, selfconcept, and effort were assessed to get a comprehensive picture of the impact of the intervention conditions on students’ motivation. We chose mathematics as the target subject because student motivation in mathematics declines dramatically during secondary school (Jacobs et al., 2002; Watt, 2004). At the same time, math is an important prerequisite for STEM careers (National Science Board, 2007). We had three major research questions. First, we analyzed whether the effects of the relevance intervention conditions were moderated by family motivational resources. More specifically, is there empirical support for a “no differential effects” perspective, for Matthew effects (i.e., already privileged students profit most; Walberg & Tsai, 1983), or for Robin Hood effects (i.e., students from families with low motivational resources profit most)? The intervention conditions provided students from families with low motivational resources with relevant utility information that they may not be exposed to in their families. Therefore, we speculated that our two intervention conditions might have the power to create Robin Hood effects. Second, it is unclear whether the exposure to relevant utility information at one time point will result in immediate effects for students from families with low motivational resources or if effects will develop over time. Students from families with fewer resources might need time to reflect on the content of the intervention, before incorporating the new information into their motivational beliefs (e.g., Mitchell, 1993). We assumed that differential effects for students from families with low motivational resources might be stronger in the long run and therefore included a follow-up measurement 5 months after the intervention to uncover potential sleeper effects (Kagan & Moss, 1962). Third, how does students’ socioeconomic background affect intervention effects in comparison to families’ motivational resources? The sample in the present study consisted of students from the academic track schools in Germany. Therefore, we investigated if relevance interventions can particularly foster academic track students from relatively low SES families (compared with students from relatively high SES families in the academic track). However, results from correlational studies indicate that families’ motivational resources might be even more important for students’ motivational beliefs than SES because they shape students’ environment more directly (e.g., Dumont et al., 2012; Häfner, Flunger, et al., 2017). As relevance interventions particularly target students’ motivational beliefs, parents’ motivational beliefs might be more likely to moderate the intervention effects. Accordingly, Harackiewicz et al. (2016) did not find any interac- 1525 tion effects between a utility value intervention and the level of poverty students were exposed to in high school on outcome variables. We therefore speculated that our intervention might be specifically helpful for students from families with low motivational resources and not for students from relatively low SES families. Method Sample The data of students and their parents were collected as part of a large longitudinal intervention study (i.e., MoMa) in academic track schools in the German state of Baden-Württemberg from September 2012 to March 2013. A total of 1,978 ninth grade students with active parental consent participated in the study, reflecting a high participation rate of 96%. Out of the total sample, 1,916 students (age at the beginning of the study: M ⫽ 14.62, SD ⫽ 0.47; 53.5% female) participated in the intervention (720 students in the text condition, 561 students in the quotations condition) or were in the waiting control group (635 students). Students absent during the intervention were not included in the present analyses (N ⫽ 65). Students were enrolled in 82 classrooms from 25 schools. Teachers and their classes were randomly assigned within each school to the waiting control group or one of two intervention conditions (text and quotations condition) resulting in 27 classes in the control condition, 30 classes in the text condition, and 25 classes in the quotations condition. It was ensured that all classes taught by the same teacher were in the same experimental condition and that conditions were distributed equally within each school. The majority of parents from participating students responded to the parent questionnaire (79.5%). We asked the parent most involved in each student’s homework to fill out the parent questionnaire: 56.3% of the questionnaires were filled out by students’ mothers, 23.6% by mothers and fathers together, and 16.7% by fathers (0.4% were filled out by a different person). Because data were collected from the highest educational track in Germany, parents’ educational level was above the national average, with 56.4% of mothers and 66.9% of fathers holding qualifications for college education (i.e., obtained a university of applied sciences entrance qualification or the Abitur certificate). However, with about 40% of all students attending elementary school going on to academic track schools in Germany (Statistisches Bundesamt [Federal Office of Statistics], 2016), this school track has the highest heterogeneity in terms of students’ socioeconomic background (Trautwein & Neumann, 2008). Accordingly, the present sample comprised students with parents from a broad range of educational backgrounds: 56.4% of mothers and 66.9% of fathers had qualifications for college education (university of applied sciences entrance qualification or the Abitur certificate, equivalent to 13 years of school), 35.4% of mothers and 18.5% of fathers had graduated from the intermediate track schools (equivalent to 10 years of school), and 6.8% of mothers and 11.8% of fathers had graduated from the lowest track schools or did not graduate at all (equivalent to 9 years of school or less). Concerning our SES measure (the International Socio-Economic Index of Occupational Status; Ganzeboom & Treiman, 2003), we also found a substantial amount of variance in the sample under investigation (RangeISEI ⫽ 16 to 90; x ⫽ 65.24, SD ⫽ 16.21). Regard- 1526 HÄFNER ET AL. ing immigrant background, 10.4% of students came from families with one parent born outside of Germany and 10.8% of students came from families with both parents born outside Germany (mothers: 20.6% born in Turkey; fathers: 21.2% born in Turkey; rest mostly born in East European countries). The majority of parents reported German as the main language spoken within their family by themselves (mothers: 90.2%; fathers: 90.8%) and their child (93.9%). This document is copyrighted by the American Psychological Association or one of its allied publishers. This article is intended solely for the personal use of the individual user and is not to be disseminated broadly. Procedure Data from both students and parents were collected via separate questionnaires at the pretest (Time 1 [T1]) which took place at the beginning of the school year. Classes in the experimental conditions received the intervention approximately one week after the pretest. Both experimental groups took part in a relevance intervention which consisted of a 90-min course unit. The first part was a psycho-educational presentation including two main topics. In the first part, research findings on the importance of students’ self-concept, effort, and frame of reference for students’ math achievement were presented. We included these topics because low competence beliefs might hinder the effectiveness of relevance interventions (see Durik, Shechter, Noh, Rozek, & Harackiewicz, 2014). In the second part of the presentation, examples for the usefulness of mathematics for students’ future careers and their daily life were displayed. We included this information to stimulate students with a lack of knowledge about the possible relevance of math for their own lives. Afterwards, students worked on individual writing assignments. Each student was assigned to either a text or a quotations condition. In the text condition, students wrote a text about the personal relevance of math for their own lives, whereas in the quotations condition, students evaluated quotations of young adults about the usefulness of math. One and 2 weeks after the intervention, students in both intervention conditions received an intervention reinforcement exercise similar to the writing assignments. Six weeks (Time 2 [T2]) and 5 months (Time 3 [T3]) after the intervention, students filled out questionnaires again. More details on the intervention can be found in Gaspard, Dicke, Flunger, Brisson, et al. (2015). Instruments Family background measures were assessed via parents at the beginning of ninth grade using the parent questionnaire. Students’ academic motivation was measured via the student questionnaire at T1, T2, and T3. Items can be found in Table A1 in the Appendix (items for students’ value beliefs can be found in Gaspard, Dicke, Flunger, Schreier, et al., 2015). SES. SES was based on information about parents’ occupation provided by both students and parents. Occupations were first coded using the International Standard Classification of Occupations (ILO, 2012) and then ranked based on the International Socio-Economic Index of Occupational Status (ISEI; Ganzeboom & Treiman, 2003). The ISEI is an international standard measure indicating the status of the occupation, ranging from 16 to 90. For the analyses, we included the highest score of the occupational status of either father or mother. Migration background. In the parent questionnaire, parents were asked to indicate for both parents if they were born in Germany or elsewhere. A dummy variable was created with 1 indicating that either one or both parents were born outside of Germany and 0 indicating no migration background. Family motivational resources. The response format for all items assessing family motivational resources ranged from 1 (completely disagree) to 4 (completely agree). We assessed family math interest (e.g., “In our family we are interested in math”; ␣ ⫽.92) using three items and asking for an evaluation of math interest of the whole family (adapted from Ramm et al., 2006). This is the first study to report findings using this measure of FI. In the same sample, these scales have also been found to be predictive for students’ motivational beliefs and achievement (Häfner, Flunger, et al., 2017). In contrast, parents’ math utility values (e.g., “Math is directly applicable in everyday life”; ␣ ⫽.86) represent subjective attitudes of the parent filling out the questionnaire and were measured using four items (Gaspard, Dicke, Flunger, Schreier, et al., 2015, adapted for parents). This is the first study measuring parents’ math utility values with these items, however, the items have been adapted from a well-validated student questionnaire (Gaspard, Dicke, Flunger, Schreier, et al., 2015; Gaspard, Häfner, Parrisius, Trautwein, & Nagengast, 2017; Guo et al., 2016). Students’ task values, self-concept, and effort. For all items, a 4-point point Likert-type scale, ranging from 1 (completely disagree) to 4 (completely agree) was used as a response format. Students’ math values were measured using the value instrument of Gaspard, Dicke, Flunger, Schreier, et al. (2015): Students’ intrinsic math value was assessed using four items (e.g., “I like doing math”;.92 ⱕ ␣ ⱕ.93). Students’ attainment value (e.g., “Math is very important to me personally”;.91 ⱕ ␣ ⱕ.92), utility value (e.g., “Understanding math has many benefits in my daily life”;.88 ⱕ ␣ ⱕ.89), and cost (e.g., “Math is a real burden to me”;.93 ⱕ ␣ ⱕ 94) were measured with 10, 12, and 11 items, respectively. Students’ math self-concept (e.g., “Math just isn’t my thing” [reverse coded];.92 ⱕ ␣ ⱕ. 93) was assessed using five items (Gaspard, Dicke, et al., 2016). Students’ effort in math (e.g., “I really work hard on homework assignments in mathematics”;.81 ⱕ ␣ ⱕ.87) was measured using five items (adapted from Trautwein, Lüdtke, Roberts, Schnyder, & Niggli, 2009). Using the same instruments, Guo et al. (2016) found that students’ selfconcept was particularly predictive of students’ achievement, whereas students’ values beliefs especially predicted students’ effort—thereby supporting the validity of these instruments. Auxiliaries. A nonverbal cognitive ability score, student gender, migration background, parents’ education, an indicator of families being dual- or single-parent families, students’ math grades at the end of eighth grade, and test scores from a state-wide standardized, curriculum-based math achievement test, which was conducted at the beginning of ninth grade, were used as auxiliaries in the imputation model. Students’ nonverbal cognitive abilities were measured using the Figure Analogies subscale (␣ ⫽.79) from the Cognitive Abilities Test 4 –12 ⫹R (Heller & Perleth, 2000). Statistical Analyses In our analyses, students were nested within their classrooms. To account for the hierarchical structure of the data (Raudenbush & Bryk, 2002), multilevel regression analyses were conducted to analyze the interaction effects of the intervention with the different This document is copyrighted by the American Psychological Association or one of its allied publishers. This article is intended solely for the personal use of the individual user and is not to be disseminated broadly. ROBIN HOOD EFFECTS indicators of students’ family background on students’ motivation using Mplus 7 (Muthén & Muthén, 1998 –2012). Measurement invariance. To investigate measurement properties across time (T1, T2, and T3) and across intervention groups, we tested for measurement invariance with separate models for each dependent variable (value components, self-concept, and effort) and increasing invariance constraints. Test of measurement properties confirmed strict measurement invariance (Widaman & Reise, 1997) across time and interventions groups and following Chen (2007) resulted in an acceptable model fit (see Table S1 in the online supplemental material for fit indices for self-concept and effort; test for measurement invariance of value beliefs can be found in Gaspard, Dicke, Flunger, Brisson, et al., 2015). Multilevel regression analyses. To assess whether students from families with fewer resources profited more from the intervention compared with students from families with more resources, multilevel regression analyses including cross-level interactions (Raudenbush & Bryk, 2002) were calculated separately for each indicator of students’ family background and each dependent variable at posttest and follow-up, respectively. To account for the assumptions of multilevel regression (Maas & Hox, 2004), all analyses used the robust maximum-likelihood estimator in Mplus 7.1, which corrects test statistics and standard errors for nonnormality in the manifest variables (Muthén & Muthén, 1998 – 2012). In addition, because the current sample consists of 82 classrooms, the estimation of the parameters can be considered trustworthy (Maas & Hox, 2005). For all analyses, group mean centering was used for the indicators at the student level (Enders & Tofighi, 2007), and manifest aggregation was used for the class level predictors (Marsh et al., 2009). To estimate the effects of the intervention more precisely, all models included students’ respective motivational indicator at the pretest as a covariate at the student level and at the class level (Raudenbush, 1997). These effects were freely estimated at both levels to account for contextual effects (Korendijk, Hox, Moerbeek, & Maas, 2011; Marsh et al., 2009). In addition, an indicator of students’ migration background was included as a predictor on both levels to control for students’ migration background with effects on both levels being freely estimated. Furthermore, we included the respective indicator of family background as a predictor on both levels. As we did not find any contextual effects of the indicators of family background on any student outcome, we constrained the effects on both levels to be equal for parsimony (Korendijk et al., 2011; Marsh et al., 2009). Thereby, the overall effect of the respective indicator of family background on the dependent variable was estimated. To control for the main effects of the intervention, we included two dummy variables indicating the two intervention conditions (respective intervention condition ⫽ 1, reference group ⫽ control group) as predictors at the class level. To determine whether intervention effects varied depending on students’ family background, we added a nonrandomly varying slope of the respective indicator of students’ family background (Raudenbush & Bryk, 2002) and included two cross-level interaction effects (Quotations ⫻ Indicator of Family Background; Text ⫻ Indicator of Family Background). Before running the analyses, all continuous variables were standardized to facilitate the interpretation of results. As a result, variance at the student level is expressed in the class level coefficient. Thereby, the coefficients of the cross-level interactions 1527 indicating the effects of the intervention conditions depending on family background compared with the control condition can directly be interpreted as effect sizes (for effect sizes in multilevel models, see Marsh et al., 2009). To facilitate the interpretation of the interactions, we calculated simple slopes for one standard deviation above and below the mean on the respective indicator of family background on the dependent variable (Aiken & West, 1991). Missing data. Missingness on student variables ranged from 0% to 11.8%. Because 394 parent questionnaires were not filled out, missingness on family background variables ranged from 22.2% to 29.9%. Thus, multiple imputation (Rubin, 1987) was used to account for missing data. We imputed data from an unrestricted two-level model in Mplus, as these “models are general enough so that model misspecification cannot occur” (Asparouhov & Muthén, 2010, p. 2) and included a nonverbal cognitive ability score, gender, previous math grade, achievement data for math at Time 1, migration background, parents’ education, and an indicator of families being dual- or single-parent families in the imputation model. We imputed 20 data sets separately for each condition (i.e., quotations condition, text condition, control group) to account for the cross-level interactions and afterwards matched these condition specific data sets. Results presented thus represent average results over 20 data sets.1 Results Randomization Check and Descriptive Statistics Descriptive statistics (means, standard deviations, intraclass correlation coefficients) of all variables under study are summarized in Table 1. As a randomization check, we tested for differences between the three experimental conditions at the pretest by calculating multilevel multigroup models for students’ outcomes (utility, attainment, intrinsic value, as well as cost, self-concept, and effort) and family background (SES, family math interest, and math utility values). We conducted several omnibus tests comparing the means of the three groups by Wald chi-square tests. No statistically significant differences between the conditions were found at the pretest—neither in terms of pretest scores of student outcomes (all ps ⱖ.292), nor for family background (all ps ⱖ.071). Reported family motivational resources differed depending on the person filling out the questionnaire (see Table S2 in the online supplemental material): Reported math FI was higher when fathers ( ⫽.21, p ⫽.002) or both parents ( ⫽.22, p ⫽.001) filled out the questionnaire than when mothers filled out the questionnaire. Similarly, fathers ( ⫽.46, p ⬍.001) and both parents ( ⫽.45, p ⬍.001) reported significantly higher math utility values than did mothers. Correlations at the pretest are presented in Table 2 (correlation patterns at T2 and T3 were comparable). Family SES showed positive but small associations with parents’ utility values (r ⫽.08) and FI (r ⫽.20), thereby supporting the theoretical differen1 The results were nearly identical to the results based on the sample without the imputed data and can be obtained from the corresponding author. HÄFNER ET AL. 1528 Table 1 Descriptive Statistics for Student and Parent Variables at all Measurement Waves Pretest This document is copyrighted by the American Psychological Association or one of its allied publishers. This article is intended solely for the personal use of the individual user and is not to be disseminated broadly. Variable Student questionnaire Utility value Attainment value Intrinsic value Cost Self-concept Effort Parent questionnaire Socioeconomic status Family interest Parents’ utility value Note. n M 1,460 1,457 1,453 1,458 1,456 1,457 2.53 2.79 2.28 2.09 2.74 2.84 1,491 1,459 1,472 65.24 2.46 2.92 Posttest SD Follow-up ICC n M SD ICC n M SD ICC.49.59.85.69.81.55.06.05.07.04.04.03 1,444 1,434 1,429 1,433 1,443 1,438 2.53 2.82 2.18 2.10 2.74 2.81.53.62.83.74.80.61.10.06.10.06.06.04 1,363 1,360 1,357 1,364 1,363 1,357 2.52 2.85 2.25 2.06 2.79 2.74.52.61.81.73.77.63.08.07.08.07.06.05 16.21.85.70.06.02.02 ICC ⫽ intraclass correlation coefficient. tiation between structural and process-related family resources (see also Dumont et al., 2012). A higher correlation was found between family motivational resources: Parents’ utility value beliefs were significantly correlated with FI (r ⫽.53). Correlations between SES and students’ outcomes varied in direction and were small in magnitude (⫺.13 ⱕ r ⱕ.11). In contrast, students’ motivational outcomes (with the exception of cost) were positively related to parents’ utility value beliefs (.09 ⱕ r ⱕ.20) and family math interest (.11 ⱕ r ⱕ.34). Students’ cost perceptions were negatively correlated with parents’ utility value beliefs (r ⫽ ⫺.15) and family math interest (r ⫽ ⫺.31). In line with previous research (e.g., Dumont et al., 2012), these results suggest that family motivational resources are more closely related to students’ motivational outcomes than to SES. Testing for Moderation by Family Motivational Variables Our first research question dealt with the exploration of differential intervention effects depending on motivational family resources controlling for students’ pretest scores. With our second research question, we investigated potential sleeper effects and compared differential effects 6 weeks and 5 months after the intervention.2 To provide a good overview of the central results without neglecting any relevant additional information, we will report the results in the following manner: (a) the central results concerning the interaction terms for all moderators (possible Matthew or Robin Hood effects) are described in Table 3. Figures 1 and 2 illustrate moderation effects of FI. Figures S1 and S2 in the online supplemental material show these effects for the other moderators. (b) Illustrating our modeling approach, the full model investigating FI as a moderator (including main effects and effects of the covariates) can be found in Table A2 in the Appendix, full models investigating parents’ utility values and SES are reported in Tables S3 and S4 in the online supplemental material. (c) For a better interpretation of significant interaction effects, conditional effects of both intervention conditions on the respective student outcome are reported for subgroups of students 1 standard deviation above and below the mean of the respective indicator of family back- ground in Table A2 in the Appendix as well as in Tables S3 and S4 in the online supplemental material (Aiken & West, 1991). Family math interest as a moderator of the intervention effects. First, we asked if students from families with low FI profited more from our intervention than students from families with higher FI, resulting in Robin Hood effects. To this end, we calculated separate models for each dependent variable at each time point.3 Regarding the main effects of FI on student outcomes, students from families with higher FI reported significantly higher levels of utility values ( ⫽.13) and fewer costs ( ⫽ ⫺.06) at the follow-up. In addition, these students showed higher attainment (.09 ⱕ  ⱕ.13), and intrinsic values (.08 ⱕ  ⱕ.13), as well as higher levels of self-concept (.07 ⱕ  ⱕ.09) and effort (.09 ⱕ  ⱕ.10) at the posttest and follow-up (see Table A2 in the Appendix). Because of the presence of interaction effects, we can interpret the effects of FI (and other respective indicators of family background) on students’ outcomes as the effects within the control group. The main intervention effects in the present models reflect the intervention effects for students with mean values on the respective indicator of family background (see Table A2 in the Appen2 Because the intervention was more effective for girls than for boys (Gaspard, Dicke, Flunger, Brisson, et al., 2015), we additionally investigated three-way interactions between each intervention condition, gender, and the respective indicators of family background. Our results confirmed the results of Gaspard, Dicke, Flunger, Brisson, et al. (2015), and none of the three-way interactions were significant. 3 Because the differences in family motivational beliefs depended on the person filling out the questionnaire, we calculated additional models. Two dummy variables were included as covariates with questionnaires filled out by mothers as the reference group: one dummy variable for questionnaires filled out by fathers, one for questionnaires filled out by both parents. When trying to include these two dummy variables in our models as covariates, including correlations between the two dummy variables and the respective indicator of motivational family beliefs resulted in nonconverging models in Mplus. Therefore, we ran additional models using the design-based correction of standard errors and fit statistics (“type⫽ complex” procedure) in Mplus instead of multilevel regression analyses and reran all reported models. The results did not differ substantially from the multilevel models without controlling for the person filling out the questionnaire. Therefore, results from the multilevel models are reported. Note. Bivariate correlations at the pretest are presented. Pattern of correlations at Time 2 and Time 3 is comparable. Coding of migration background: 0 ⫽ no migration background, 1 ⫽ migration background. † p ⬍.10. ⴱ p ⬍.05. ⴱⴱ p ⬍.01. ⴱⴱⴱ p ⬍.001. — —.31ⴱⴱⴱ — ⫺.75ⴱⴱⴱ ⫺.28ⴱⴱⴱ — ⫺.68ⴱⴱⴱ.73ⴱⴱⴱ.42ⴱⴱⴱ —.64ⴱⴱⴱ ⫺.41ⴱⴱⴱ.48ⴱⴱⴱ.58ⴱⴱⴱ —.68ⴱⴱⴱ.54ⴱⴱⴱ ⫺.31ⴱⴱⴱ.39ⴱⴱⴱ.39ⴱⴱⴱ —.21ⴱⴱⴱ.21ⴱⴱⴱ.33ⴱⴱⴱ ⫺.31ⴱⴱⴱ.35ⴱⴱⴱ.11ⴱⴱⴱ —.53ⴱⴱⴱ.18ⴱⴱⴱ.17ⴱⴱⴱ.18ⴱⴱⴱ ⫺.15ⴱⴱⴱ.20ⴱⴱⴱ.09ⴱⴱ —.08ⴱⴱ.20ⴱⴱⴱ ⫺.04 ⫺.06ⴱ.05† ⫺.13ⴱⴱⴱ.11ⴱⴱⴱ ⫺.07ⴱ — ⫺.11ⴱⴱⴱ ⫺.05† ⫺.05† ⫺.04† ⫺.04† ⫺.06ⴱ.05ⴱ ⫺.08ⴱⴱ ⫺.07ⴱⴱ — ⫺.08ⴱⴱ.52ⴱⴱⴱ.05†.15ⴱⴱⴱ ⫺.04 ⫺.07ⴱⴱ.06ⴱ ⫺.12ⴱⴱⴱ.13ⴱⴱⴱ ⫺.09ⴱⴱ — ⫺.04.19ⴱⴱⴱ ⫺.04 ⫺.09ⴱⴱ ⫺.11ⴱⴱⴱ ⫺.04 ⫺.02.01.02 ⫺.02 ⫺.04 — ⫺.05†.15ⴱⴱⴱ ⫺.19ⴱⴱⴱ.16ⴱⴱⴱ.13ⴱⴱⴱ.25ⴱⴱⴱ.22ⴱⴱⴱ.24ⴱⴱⴱ.40ⴱⴱⴱ ⫺.44ⴱⴱⴱ.50ⴱⴱⴱ.20ⴱⴱⴱ —.58ⴱⴱⴱ ⫺.08ⴱⴱ.15ⴱⴱⴱ ⫺.16ⴱⴱⴱ.15ⴱⴱⴱ.13ⴱⴱⴱ.23ⴱⴱⴱ.22ⴱⴱⴱ.30ⴱⴱⴱ.42ⴱⴱⴱ ⫺.47ⴱⴱⴱ.58ⴱⴱⴱ.28ⴱⴱⴱ —.06ⴱ.10ⴱⴱⴱ ⫺.02.07ⴱⴱ.05ⴱ.06ⴱ ⫺.02.10ⴱⴱⴱ.09ⴱⴱⴱ.09ⴱⴱⴱ.16ⴱⴱⴱ ⫺.13ⴱⴱⴱ.23ⴱⴱⴱ ⫺.07ⴱⴱ — ⫺.03.24ⴱⴱⴱ.30ⴱⴱⴱ.00.09ⴱⴱ ⫺.09ⴱⴱⴱ.08ⴱⴱ.08ⴱⴱ.13ⴱⴱⴱ.05ⴱ.04†.19ⴱⴱⴱ ⫺.22ⴱⴱⴱ.28ⴱⴱⴱ ⫺.01 Students’ cognitive ability Student gender (male ⫽ 1) Math grade end of 8th grade Math achievement Single vs. dual parent Parent education Migration background Socioeconomic status Parents’ utility value Family math interest Students’ utility value Students’ attainment value Students’ intrinsic value Students’ costs Students’ self-concept Students’ effort 1. 2. 3. 4. 5. 6. 7. 8. 9. 10. 11. 12. 13. 14. 15. 16. 14 13 12 11 10 9 Variable 1 2 3 4 5 6 7 8 Family background Auxiliaries Table 2 Manifest Intercorrelations of Variables Measured at Time 1 This document is copyrighted by the American Psychological Association or one of its allied publishers. This article is intended solely for the personal use of the individual user and is not to be disseminated broadly. Students‘ academic motivation 15 16 ROBIN HOOD EFFECTS 1529 dix). Analyses of the main intervention effects without including indicators of family background as independent variables can be found in Gaspard, Dicke, Flunger, Brisson, et al. (2015) and Brisson et al. (in press). Students in both intervention conditions reported statistically significant higher utility values at both time points than did students in the control group, controlling for their initial values (see Table A2 in the Appendix). Additionally, we found a significant positive effect of the quotations condition on students’ attainment values 5 months after the intervention, controlling for their initial values. An overview of the cross-level interactions between the respective moderator and the intervention conditions on students’ motivational beliefs (effects on different outcomes are presented in the rows) resulting from the multilevel models at posttest (upper half of the table) and follow-up (lower half of the table) are presented in Table 3. As is shown in Table 3, the intervention effects on students’ motivational beliefs were not systematically moderated by FI at posttest (with the exception of effort)—neither Matthew effects nor Robin Hood effects occurred. At follow-up, in contrast, we found support for our hypotheses: Several significant crosslevel interaction effects between FI and both intervention conditions emerged—thereby supporting our sleeper effect hypothesis (see Figures 1 and 2).4 The effects of the intervention conditions on students’ utility values were moderated by FI at follow-up: As is shown in Table 3, for students whose families reported math interest one standard deviation below the mean, the effect of the quotations condition on students’ math utility values was higher ( ⫽.12 SD) compared with the effect of the quotations conditions for students from families with mean levels of FI. Similarly, the effect of the text condition on students’ utility value beliefs was higher ( ⫽.14 SD) for students with FI one standard deviation below the mean, compared with the effect of the text condition for students from families with mean levels of FI. In other words, both intervention conditions resulted in compensatory effects for students with lower FI. Receiving either intervention condition, these students showed similar levels of utility value beliefs 5 months after the intervention compared with students from families with higher FI— thereby creating Robin Hood effects. Although we did not find significant interaction effects at posttest on students’ utility values, the interactions of FI with both intervention conditions at posttest and follow-up are shaped similarly (see Figure 1). Simple slope analyses indicate that students from families with FI one standard deviation below the mean show an increase in math utility value ( ⫽.37 SD) when being in the quotations condition and in math utility value ( ⫽.29 SD) when being in the text condition, compared with students with FI one standard deviation below the mean in the control condition. Regarding students’ attainment value, the effects of both intervention conditions differed depending on different levels of FI at follow-up. Both intervention conditions promoted attainment value beliefs for students from families with low FI, but not with high FI (see Figure 1). Thereby, both conditions counteracted 4 We analyzed the mean differences on students’ outcomes depending on the respective family background variables to investigate possible ceiling effects for students from families with higher SES or higher motivational family characteristics (see Table S6 in the online supplemental material). We did not find any indication of possible ceiling effects. HÄFNER ET AL. 1530 Table 3 Intervention Effects Depending on the Respective Indicators of Family Background on Students’ Motivation at Posttest and Follow-Up (N ⫽ 1,916) Utility Interaction Est. Attainment (SE) Est. Intrinsic (SE) Est. Cost Self-concept Effort (SE) Est. (SE) Est. (SE) Est. (SE) This document is copyrighted by the American Psychological Association or one of its allied publishers. This article is intended solely for the personal use of the individual user and is not to be disseminated broadly. Posttest Family Interest (FI) Quotations ⫻ FI Text ⫻ FI Parents’ Utility Values (PUV) Quotations ⫻ PUV Text ⫻ PUV Socioeconomic status (SES) Quotations ⫻ SES Text ⫻ SES ⫺.08† ⫺.01 (.04) (.05) ⫺.04 ⫺.07† (.04) (.04) ⫺.04 ⫺.06 (.04) (.04).03.03 (.05) (.04) ⫺.01 ⫺.04 (.04) (.04) ⴚ.10ⴱ ⴚ.10ⴱ (.04) (.04).03 ⫺.03 (.05) (.04) ⫺.02 ⫺.06 (.05) (.04).05 ⫺.06 (.04) (.04).04.02 (.04) (.04) ⫺.01 ⫺.03 (.03) (.03) ⴚ.09ⴱ ⫺.05 (.05) (.04).03.04 (.04) (.05).04 ⫺.02 (.04) (.04) ⫺.03 ⴚ.07ⴱ (.03) (.03) ⫺.01 ⫺.04 (.04) (.04) ⫺.03 ⫺.01 (.03) (.04) ⫺.03 ⫺.05 (.05) (.05) Follow-up FI Quotations ⫻ FI Text ⫻ FI Difference PUV Quotations ⫻ PUV Text ⫻ PUV Difference SES Quotations ⫻ SES Text ⫻ SES ⴚ.12ⴱ ⴚ.14ⴱⴱ.02 (.05) (.05) ⴚ.10ⴱ ⴚ.13ⴱⴱ.03 (.05) (.04) ⴚ.09ⴱ ⫺.07.02 (.04) (.04).05.01.04 (.04) (.04) ⫺.07 ⫺.01.06 (.04) (.04) ⴚ.14ⴱ ⴚ.14ⴱⴱ.00 (.06) (.05).03 ⴚ.11ⴱ.14ⴱⴱ (.05) (.05).01 ⴚ.11ⴱ.12ⴱ (.04) (.05).00 ⫺.04.04 (.04) (.04).06.02.04 (.05) (.05) ⫺.06† ⫺.07†.01 (.04) (.04) ⫺.02 ⫺.08†.06 (.05) (.05) ⫺.04 ⫺.08 (.05) (.05).04 ⫺.05 (.04) (.04) ⫺.06 ⫺.03 (.04) (.04) ⫺.02 ⫺.06 (.04) (.04).02 ⫺.01 (.04) (.04) ⫺.03 ⫺.00 (.05) (.04) Note. Significant effects are presented bold. Est. ⫽ Estimated Parameters. Quotations ⫻ ‘respective indicator of family background’ ⫽ Cross-level interaction effect between respective indicator of family background and quotations condition; Text ⫻ ‘respective indicator of family background’ ⫽ Cross-level interaction effect between ‘respective indicator of family background’ and text condition. Difference ⫽ Test if the interactions differ depending on the intervention condition using Wald-2 tests. † p ⬍ 0.10. ⴱ p ⬍ 0.05. ⴱⴱ p ⬍ 0.01. ⴱⴱⴱ p ⬍ 0.001. motivational gaps between students from families with low and high FI, resulting in similarly high levels in attainment value 5 months after the intervention. For students’ intrinsic value, there were significant cross-level interactions between the quotations condition and FI at follow-up. Again, the quotations condition resulted in Robin Hood effects (see Figure 1). For students’ effort, there were significant cross-level interactions between both intervention conditions and FI at posttest and follow-up (see Figure 2). At both time points, students from families with lower FI profited more from the text condition in terms of their effort in math compared with students from families with higher FI. Furthermore, using the Wald chi-square tests (Bakk & Vermunt, 2016), we tested whether the interaction effects differed depending on the intervention condition. We did not find any significant differences in the interactions between the two intervention conditions and FI (see Table 3). As a test of robustness of our results, we reran the analyses using parents’ intrinsic math values as a moderator. Parents’ intrinsic math values and FI are conceptually different, with FI reflecting a broader motivational atmosphere within the family (e.g., Frenzel et al., 2010) that also includes siblings and both parents and parents’ intrinsic math values referring to the intrinsic math value of the particular parent filling out the questionnaire. Parents’ intrinsic math values were highly correlated with FI. Thus, investigating parents’ intrinsic values as moderators of the intervention effects should lead to similar results as using FI as a moderator. Analyzing parents’ intrinsic values as a moderator, we found very similar results concerning differential intervention effects depending on FI: Students whose parents reported low intrinsic math values particularly profited from both intervention conditions in terms of their intrinsic values at follow-up. In addition, these students especially profited from the text condition in terms of their utility and attainment value at follow-up (see Table S5 in the online supplemental material for the results of these additional analyses). Therefore, these results support the validity of our findings. Parents’ math utility value as a moderator of the intervention effects. Further models including the relevant cross-level interactions were calculated to analyze the influence of parents’ math utility values on the intervention effects (see Table S3 in the online supplemental material for details). At the posttest, parents’ utility values did not systematically moderate the effects of the intervention conditions on student outcomes (again, with the exception of effort; see Table 3). With regard to students’ utility and attainment values, we found significant cross-level interactions between the text condition and parents’ utility values at follow-up (for illustration see Figure S1 in the online supplemental material): Whereas the quotations condition promoted students’ utility and attainment values regardless of parents’ utility values, the text condition only promoted students’ utility and attainment beliefs if their parents reported low levels of parents’ utility values, thereby creating Robin Hood effects. For This document is copyrighted by the American Psychological Association or one of its allied publishers. This article is intended solely for the personal use of the individual user and is not to be disseminated broadly. ROBIN HOOD EFFECTS 1531 Figure 1. Adjusted means for students’ utility, attainment, and intrinsic value beliefs at posttest (upper half) and follow-up (lower half) by family interest (FI) in math and intervention group. Predicted values were generated for low (⫺1 standard deviation) and high (⫹1 standard deviation) family interest in math from the multilevel regression model. students’ intrinsic values, cost, self-concept, and effort, we did not find significant cross-level interactions at follow-up. Using the Wald chi-square tests (Bakk & Vermunt, 2016), we found two significant differences in the intervention effects of the two conditions (see Table 3): the interaction between parents’ utility values and the text condition differed statistically significantly from the interaction between parents’ utility values and the quotations condition in terms of their effects on students’ utility values (difference of interaction between the two conditions ⫽.14, p ⬍.01) and attainment values (difference of interaction between the two conditions ⫽.12, p ⬍.05). Thus, the text condition promoted utility and attainment values particularly for students whose parents held low math utility values, whereas the quotations conditions promoted these values for students irrespective of their parents’ utility values. Testing for Moderation of Effects by SES To answer our third research question, whether or not the intervention would yield Robin Hood effects depending on structural family resources, we examined whether students from families with a relatively lower SES profited more from our intervention than did students from families with a relatively higher SES, again calculating separate models for each dependent variable at each time point (see Table S4 in the online supplemental material for details). In contrast to family motivational beliefs, families’ relative SES was not significantly related to any student outcome—neither at posttest nor at follow-up. Regarding the cross-level interaction effects (see Table 3), we found only one significant cross-level interaction between the text condition and relative SES on students’ intrinsic values at posttest. We did not find any other significant interactions between families’ relative SES and either intervention condition at both time points; thus, the effects of both intervention conditions on student outcomes did not differ systematically due to families’ relative SES (for illustration see Figure S2 in the online supplemental material). There was no significant difference between the interaction effects of the two conditions and relative SES. To conclude, with one exception, family SES in relation to the overall SES of academic track students did not moderate the intervention effects on students’ outcomes at both time points. Therefore, in line with our third hypothesis and in contrast to the effects found for family motivational beliefs, we found neither Matthew effects nor Robin Hood effects when investigating families’ relative SES. Discussion This study investigated whether a relevance intervention in the classroom could be a promising tool to foster academic motivation for students with fewer motivational family resources: Was the intervention specifically promising for students from families with fewer resources and did it yield a Robin Hood effect? For four out of six indicators of student motivation (namely utility, attainment, HÄFNER ET AL. This document is copyrighted by the American Psychological Association or one of its allied publishers. This article is intended solely for the personal use of the individual user and is not to be disseminated broadly. 1532 Figure 2. Adjusted means for students’ cost, self-concept, and effort at posttest (upper half) and follow-up (lower half) by family interest (FI) in math and intervention group. Predicted values were generated for low (⫺1 standard deviation) and high (⫹1 standard deviation) family interest in math from the multilevel regression model. intrinsic value, and effort), we found significant interaction effects between either one or both intervention conditions and family math interest at follow-up. Moreover, for students’ utility and attainment values, we found significant interactions between the text condition and parents’ utility values at follow-up. Findings yielded no support for Matthew effects and convincing support for Robin Hood effects. The relevance intervention resulted in compensatory effects for students whose parents reported lower levels of FI and lower levels of parents’ utility values. As expected, families’ and parents’ motivational resources moderated the effects of the relevance interventions, but families’ relative SES did not.5 The differential effects of the intervention were found five months after the intervention, not at posttest. Family Background as a Moderator of Relevance Interventions: Support for Robin Hood Effects Regarding family math interest as a moderator, we found several Robin Hood effects: The effects of both intervention conditions on students’ utility and attainment values as well as on students’ effort 5 months after the intervention were higher for students whose parents reported lower FI. Students from families with lower FI also profited more in terms of their intrinsic values when in the quotations condition. A similar pattern occurred when looking at parents’ utility values. Comparing students with similar initial levels in each outcome, the effects of the text condition on students’ utility and attainment values were higher for students whose parents reported lower math utility values. We did not find any significant interaction effects on students’ self-concept. One potential reason might be that students’ self-concept is influenced mainly by achievement information and only to a small degree by student motivation (e.g., Marsh, Trautwein, Lüdtke, Köller, & Baumert, 2005). Therefore, the differential intervention effects on students’ motivational beliefs might not have carried over to students’ self-concepts. Interaction effects in the social sciences are often very small. In conjunction with the measurement error in manifest variables, they are often difficult to detect and biased (Busemeyer & Jones, 1983). 5 To test the robustness of our results, we reran these models investigating additional structural family characteristics (i.e., migration background, parents’ education, and an indicator of families being dual- or single-parent families) as potential moderators of the intervention effects. As is shown in Table S7 in the online supplemental material, neither parents’ education nor migration background significantly moderated the intervention effects on students’ motivational beliefs at either time point. Regarding single-versus dual-parent families, we only found a significant interaction with the quotations conditions on students’ utility values at both time points, suggesting that students from single-parent families specifically benefitted from the quotations conditions in terms of their utility values. Because the vast majority of single parents were mothers (88.2%), who reported lower motivational resources (see Table S2 in the online supplemental material), motivational family resources are likely to drive this effect rather than is being a single parent. To summarize, these results support our assumption that structural family characteristics did not systematically moderate the intervention effects on students’ motivation. This document is copyrighted by the American Psychological Association or one of its allied publishers. This article is intended solely for the personal use of the individual user and is not to be disseminated broadly. ROBIN HOOD EFFECTS Therefore, finding a systematic pattern of interaction effects in four of six indicators of student motivation is noteworthy. The magnitude of the interaction effects found in the present study ranged from  ⫽.07 to  ⫽.14, which is similar to interaction effect sizes found in other studies (e.g., Trautwein et al., 2012). As we did not control for measurement error in the predictor variables, the effect size of the interaction is most likely underestimated (Busemeyer & Jones, 1983; Dimitruk, Schermelleh-Engel, Kelava, & Moosbrugger, 2007). In addition, it is common to find lower effect sizes of interventions in natural settings compared with laboratory interventions (Bronfenbrenner & Morris, 2006). Although reported effect sizes might seem rather small, seemingly small effects are meaningful in the educational setting. As is shown in Figure 1, both intervention conditions resulted in similar levels of students’ utility and attainment value beliefs, and effort for students from families with FI one standard deviation above and below the mean—thereby compensating the motivational gaps. Moreover, simple slope analyses indicated that the text condition resulted in an increase of.29 standard deviation of utility value beliefs and the quotation condition in an increase of.37 standard deviation for students from families with FI one standard deviation below the mean. For students with FI two standard deviations below the mean, the effect sizes were even larger (.43 standard deviation for the text condition and.49 standard deviation for the quotation condition). To investigate the direction and magnitude of the effects for students with distinct family backgrounds, additional simple slopes analyses were calculated following the recommendations of Aiken and West (1991). Simple slopes analyses revealed no considerable negative effects of the intervention for students with higher levels of FI and parents’ math utility values. Just as Robin Hood wanted to help the less privileged by providing them with resources they did not have, we expected to foster academic motivation for students in need by giving relevant utility information to them. Students whose families have high motivational resources (e.g., high value beliefs) have higher levels of academic motivation themselves (Frenzel et al., 2010; Rozek et al., 2015). By providing some of the resources available to students whose families have high motivational resources within our intervention, students from families who did not already hold these motivational resources profited most from the intervention. At the same time, we did not find any considerable adverse effects for students from families with higher motivational resources. However, some would argue that students from families with high motivational resources had to attend the intervention of 90 minutes, but did not profit as much as their counterparts. Nevertheless, all students actually profited from the intervention in terms of their utility value beliefs—with students from families with high math interest simply profiting less. Considering the positive associations of students’ utility value beliefs with students’ academic outcomes and choices (Harackiewicz et al., 2014), the 90-minutes intervention appears worthwhile for all students. Additionally, relevance interventions might be a promising tool to establish similar initial conditions for students from families with distinct levels of FI. The Importance of Family Interest Why is families’ math interest a systematic moderator of the intervention effects and not SES? One reason might be that the 1533 sample under investigation was comprised solely of academic track students, which were positively selective in terms of SES. However, this student population has the highest heterogeneity in terms of SES in Germany (Trautwein & Neumann, 2008). Academic track students from relatively low SES families have worse educational outcomes compared with academic track students from relatively high SES families: They perform worse in achievement tests (Ehmke, Hohensee, Heidemeier, & Prenzel, 2004), are at higher risk of dropping out of the academic track (Hillmert & Jacob, 2010; Stamm, 2010), and have a lower probability of attending a university after graduating from high school (Autorengruppe Bildungsberichterstattung, 2012; Hillmert & Jacob, 2005, 2010; Kramer et al., 2011). Thus, students’ SES still plays an important role in academic track schools in Germany, particularly concerning students’ achievement, transitions, and dropout. However, regarding student motivation, our results suggest that motivational family resources are more important for the development of students’ motivational beliefs (correlations between motivational family resources and students’ motivational beliefs ranged from.09 ⱕ r ⱕ.35) than structural family characteristics such as SES. In fact, the correlations between SES and students’ motivational beliefs varied in direction and were small in magnitude (⫺.13 ⱕ r ⱕ.11). Moreover, research demonstrated that motivational family resources positively predict students’ achievement (Häfner, Flunger, et al., 2017; Häfner, Hulleman, et al., 2016), course-taking (Häfner, Hulleman, et al., 2016; Harackiewicz et al., 2012), and career aspirations (Häfner, Hulleman, et al., 2016; Jodl, Michael, Malanchuk, Eccles, & Sameroff, 2001). Replicating these findings in a more diverse SES sample will be necessary to understand the ongoing mechanisms even better. The higher correlations of students’ motivational beliefs with family motivational resources than with SES could result from a common method factor because both are self-report measures. However, these measures stem from different sources (i.e., parents and students), thus, reducing the common methods factor. In line with theoretical assumptions and previous research (e.g., Bronfenbrenner & Morris, 2006; Dumont et al., 2012), these results are more likely to suggest that family motivational resources are more closely related to students’ motivational outcomes than to SES. As we manipulated students’ utility values, one could have expected that the intervention would be most beneficial for students whose parents do not see math as a useful subject and pass this attitude on to their children (e.g., Harackiewicz et al., 2012). However, students’ motivational beliefs were more strongly related to FI than to parents’ utility values. Fewer student outcomes were moderated by parents’ utility value beliefs than by FI. Utility value is more extrinsic in nature (Eccles & Wigfield, 2002) compared with interest and can thus be more easily influenced by outside interventions. In contrast, interest describes affective components of motivation (Eccles & Wigfield, 2002; Hidi & Renninger, 2006), which might be more readily expressed and more easily observed compared with utility value beliefs. Family interest could be especially important for students’ motivational outcomes as it reflects a broader motivational atmosphere within the family (e.g., Frenzel et al., 2010), which includes siblings and both parents. If families are interested in math, students might observe their parents and siblings enjoying math-related activities and expressing a positive attitude toward math. This might result in greater social stimulation regarding math that is expressed openly 1534 HÄFNER ET AL. in students’ everyday life. As fewer moderating effects were found for parents’ utility values, it might not be enough for parents to perceive math as a useful subject to foster students’ motivation—it might be more important for parents to like it. Our findings suggest that differentiating between motivational resources of the family and of parents is an important factor in understanding how family resources influence students’ academic well-being and which resources matter most. We demonstrated that a relevance intervention can effectively counteract lower family motivational resources. resulting incorporation of the value of math might have taken some time. In contrast, students from families with high motivational resources might have had prior knowledge about the relevance of math resulting in a short-term attitude change, which readjusted to their prior attitudes about the relevance of math over time. To conclude, finding Robin Hood effects in the long run for students from families with low motivational resources is essential to decrease motivational gaps between students from families with more versus fewer resources. Implications This document is copyrighted by the American Psychological Association or one of its allied publishers. This article is intended solely for the personal use of the individual user and is not to be disseminated broadly. Intervention Conditions and Long-Term Effects Regarding the effects of our two intervention conditions (quotations vs. text), there were no significant differences between the patterns of interaction effects of the two intervention conditions regarding FI and SES. Yet, for parents’ utility values we found significant interaction effects only of the text condition: Thus, students whose parents reported lower levels of utility values benefitted more in terms of their utility and attainment values in the text condition than did students whose parents reported higher levels of utility values. In contrast, all students in the quotations condition profited in terms of their utility and attainment values, irrespective of their parents’ utility values. This difference might be due to the different nature of the two intervention conditions: Previous research demonstrated that the text condition specifically promoted student motivation for students with lower initial conditions (e.g., Canning & Harackiewicz, 2015; Durik et al., 2015). Therefore, the text condition might have been less engaging for students with higher initial conditions, whereas the quotations condition was equally engaging for students regardless of their initial conditions. Family motivational resources (especially family math interest) did moderate the effects of the relevance interventions on students’ motivation 5 months after the intervention, but not at posttest. The intervention was especially successful in fostering a positive motivational development for students with lower initial motivational family resources 5 months after the intervention. At the posttest, all students profited to the same extent. Yet, the intervention effects for students from families with low motivational resources increased even until follow-up, in contrast to their counterparts. One possible explanation of the sleeper effects found could be different forms of information processing for students from families with more motivational family resources (for whom the utility information might have been familiar) and students from families with less motivational resources (for whom the utility information might have been new). Thus, students whose parents reported lower levels of math interest might have reflected more about the content of the intervention, namely the usefulness of math, and— over time—realized and adopted the usefulness of math more and more (e.g., Berlyne, 1960; Petty & Cacioppo, 1986). Similarly, Mitchell (1993) considered stimulation and empowerment as the key incentives for the consolidation of interest (Mitchell, 1993), which could also be relevant for the consolidation of other motivational processes. Thus, one has to identify with a new content first, and only subsequently will it “be experienced as personally meaningful and thus empowering” (Mitchell, 1993, p. 426). Students from families with lower math interest might have received new information from the intervention, providing stimulation. The The results of our study have several implications for intervention studies and the academic context in general. Comparing our results with the results of Gaspard, Dicke, Flunger, Brisson, et al. (2015) investigating the main intervention effects, we also found that all students profited from the quotations condition in terms of their utility and attainment values 6 weeks and 5 months after the intervention and in terms of their intrinsic values 5 months after the intervention. In addition, we also found that all students profited from the text condition in terms of their utility values 6 weeks and 5 months later. Yet, our results highlight some additional findings: Not all students profited from the intervention conditions to the same degree. In detail, students from families with lower math interest profited more in terms of their utility, attainment and intrinsic value beliefs compared with students from families with higher math interest. With regard to future intervention studies, our findings suggest that families need to be seen as an important factor moderating intervention effects. Harackiewicz et al. (2012) demonstrated that the effects of a lack of information on the utility of math can be counteracted by handing information on the utility of math to parents directly: That is, parents may act as a buffer against the decrease in students’ motivation during secondary school. Our findings also indicate that FI is another important factor that should be taken into account in future intervention studies targeting families. Providing parents with information about how interesting a specific subject is might change the ways parents think about a domain and, ultimately, affect the way they communicate about this issue with their children. Interventions counteracting motivational gaps between students from families with different motivational resources can facilitate equal opportunities for students’ academic development. Relevance interventions may foster academic motivation for students whose families show lower levels of interest in math and whose parents report lower levels of math utility values. These compensatory effects demonstrated that it is possible to foster academic motivation for students in need within the school setting. Limitations and Future Research Although our study provided evidence that parents’ motivation plays a major role in explaining the differential effects of classroom interventions, it has some limitations which should be kept in mind when interpreting the results. First, students from families with lower motivational resources might have benefited most from our intervention as they received new information about the utility of math they did not receive from their families. However, future research is needed to investigate the processes at play: Parents’ This document is copyrighted by the American Psychological Association or one of its allied publishers. This article is intended solely for the personal use of the individual user and is not to be disseminated broadly. ROBIN HOOD EFFECTS motivational beliefs are assumed to translate into parents’ behavior through which students’ motivational beliefs are influenced (Eccles, 2007; Simpkins et al., 2012). Students from families with lower motivational beliefs might therefore encounter little social stimulation regarding math during their daily life at home. In addition, further studies are needed to investigate the mechanisms through which family background and motivational family resources influence student motivation. One potential mediator refers to students’ perceptions of their parents’ motivational beliefs (e.g., Eccles, 2007). So far, the processes through which students establish their perceptions of parents’ motivational beliefs are still unknown. To investigate the processes at play, future research might include other methods, such as observational studies. However, such methods are rarely found in current studies investigating the importance of family background as it is difficult to apply these methods to large samples. Second, we were able to demonstrate that relevance interventions can be used to promote motivational beliefs for students from families with low motivational resources. However, it remains to be seen whether students from families with lower math interest are more prone to taking science classes after taking part in the relevance intervention or if there are further differential effects on other academic choices in the long term. Future studies should therefore investigate if Robin Hood effects from relevance interventions also influence long-term outcomes, such as students’ career decisions. Third, we were able to demonstrate the effectiveness of our relevance intervention by drawing on a particularly large sample. For relevance interventions to be most effective, the intervention needs to be specifically adapted to the sample under investigation. Because our intervention focused on the usefulness of math for students’ future lives, including examples of usefulness of math in possible future university majors, the intervention could only be delivered to academic track students in its present form. Therefore, our sample consisted of German academic track schools only. Despite the heterogeneity of SES in this sample, it is still positively selected. Future research should investigate the generalizability of our results by testing whether the Robin Hood effects are replicable in other age groups, different school types, and samples with different and more diverse ethnic backgrounds. In addition, so far no conclusions can be drawn on intervention effects in other domains, such as German or English. Conclusion In line with recent research investigating students’ motivational development, our results highlight the importance of motivational family resources, besides SES. The relevance interventions were especially effective in fostering value beliefs and effort for students whose parents reported lower FI in math and lower math utility values compared with their counterparts. Thereby, relevance interventions seem to be an effective tool to foster motivational outcomes for students from families with fewer motivational resources while not harming their counterparts. By investigating the moderating role of several family resources, we could show that families are complex interpersonal networks with a variety of specific characteristics that shape the environment students grow up in. It is important to account for this complexity when investigating family influences on students’ academic outcomes. 1535 References Aiken, L. S., & West, S. G. (1991). Multiple regression: Testing and interpreting interactions. Thousand Oaks, CA: SAGE. Asparouhov, T., & Muthén, B. (2010). Multiple imputation with Mplus. Retrieved from MPlus Web Notes, 2010, from www.statmodel2.com Autorengruppe Bildungsberichterstattung. (2012). Bildung in Deutschland 2012 [Education in Germany 2012]. Bielefeld, Germany: Bertelsmann Verlag. Bakk, Z., & Vermunt, J. K. (2016). Robustness of stepwise latent class modeling with continuous distal outcomes. Structural Equation Modeling, 23, 20 –31. http://dx.doi.org/10.1080/10705511.2014.955104 Berlyne, D. E. (1960). Conflict, arousal, and curiosity. New York, NY: McGraw-Hill. http://dx.doi.org/10.1037/11164-000 Brisson, B. M., Dicke, A.-L., Gaspard, H., Häfner, I., Flunger, B., Nagengast, B., & Trautwein, U. (in press). Short intervention, sustained effects: Promoting students’ math competence beliefs, effort, and achievement. American Educational Research Journal. Bronfenbrenner, U., & Morris, P. A. (2006). The bioecological model of human development. In W. Damon & R. M. Lerner (Eds.), Handbook of child psychology: Vol. 1. Theoretical models of human development (pp. 793– 828). New York, NY: Wiley. Busemeyer, J., & Jones, L. (1983). Analysis of multiplicative combination rules when the causal variables are measured with error. Psychological Bulletin, 93, 549 –562. http://dx.doi.org/10.1037/0033-2909.93.3.549 Canning, E. A., & Harackiewicz, J. M. (2015). Teach it, don’t preach it: The differential effects of directly-communicated and self-generated utility–value information. Motivation Science, 1, 47–71. http://dx.doi.org/10.1037/mot0000015 Ceci, S. J., & Papierno, P. B. (2005). The rhetoric and reality of gap closing: When the “have-nots” gain but the “haves” gain even more. American Psychologist, 60, 149 –160. http://dx.doi.org/10.1037/0003066X.60.2.149 Chen, F. (2007). Sensitivity of goodness of fit indexes to lack of measurement invariance. Structural Equation Modeling, 14, 464 –504. http://dx.doi.org/10.1080/10705510701301834 Cohen, G. L., Garcia, J., Purdie-Vaughns, V., Apfel, N., & Brzustoski, P. (2009). Recursive processes in self-affirmation: Intervening to close the minority achievement gap. Science, 324, 400 – 403. http://dx.doi.org/10.1126/science.1170769 Coleman, J. S., Campbell, H. Q., Hobson, C. J., McPartland, J., Mood, A. M., Weinfeld, F. D., & York, R. L. (1966). Equality of educational opportunity. Washington, DC: Office of Education. Dabney, K. P., Chakraverty, D., & Tai, R. H. (2013). The association of family influence and initial interest in science. Science Education, 97, 395– 409. http://dx.doi.org/10.1002/sce.21060 Dimitruk, P., Schermelleh-Engel, K., Kelava, A., & Moosbrugger, H. (2007). Challenges in nonlinear structural equation modeling. Methodology: European Journal of Research Methods for the Behavioral and Social Sciences, 3, 100 –114. http://dx.doi.org/10.1027/1614-2241.3.3.100 Dotterer, A. M., McHale, S. M., & Crouter, A. C. (2009). The development and correlates of academic interests from childhood through adolescence. Journal of Educational Psychology, 101, 509 –519. http://dx.doi.org/10.1037/a0013987 Dumont, H., Trautwein, U., Lüdtke, O., Neumann, M., Niggli, A., & Schnyder, I. (2012). Does parental homework involvement mediate the relationship between family background and educational outcomes? Contemporary Educational Psychology, 37, 55– 69. http://dx.doi.org/10.1016/j.cedpsych.2011.09.004 Dumont, H., Trautwein, U., Nagy, G., & Nagengast, B. (2014). Quality of parental homework involvement: Predictors and reciprocal relations with academic functioning in the reading domain. Journal of Educational Psychology, 106, 144 –161. http://dx.doi.org/10.1037/a0034100 This document is copyrighted by the American Psychological Association or one of its allied publishers. This article is intended solely for the personal use of the individual user and is not to be disseminated broadly. 1536 HÄFNER ET AL. Durik, A. M., & Harackiewicz, J. M. (2007). Different strokes for different folks: How individual interest moderates the effects of situational factors on task interest. Journal of Educational Psychology, 99, 597– 610. http://dx.doi.org/10.1037/0022-0663.99.3.597 Durik, A. M., Hulleman, C. S., & Harackiewicz, J. M. (2015). One size fits some: Instructional enhancements to promote interest. In K. A. Renninger, M. Nieswandt, & S. Hidi (Eds.), Interest, the self, and K-16 mathematics and science learning (pp. 42– 62). Washington, DC: American Educational Research Association. http://dx.doi.org/10.3102/978-0935302-42-4_3 Durik, A. M., Shechter, O. G., Noh, M. S., Rozek, C. S., & Harackiewicz, J. M. (2014). What if I can’t? Success expectancies moderate the effects of utility value information on situational interest and performance. Motivation and Emotion, 39, 104 –118. http://dx.doi.org/10.1007/ s11031-014-9419-0 Eccles, J. S. (2005). Subjective task values and the Eccles et al. model of achievement related choices. In A. J. Elliott & C. S. Dweck (Eds.), Handbook of competence and motivation (pp. 105–121). New York, NY: Guilford Press. Eccles, J. S. (2007). Families, schools, and developing achievement-related motivations and engagement. In J. E. Grusec & P. D. Hastings (Eds.), Handbook of socialization (pp. 665– 691). New York, NY: Guilford Press. Eccles, J. S., Adler, T. F., Futterman, R., Goff, S. B., Kaczala, C. M., Meece, J. L., & Midgley, C. (1983). Expectancies, values and academic behaviors. In J. T. Spence (Ed.), Achievement and achievement motives: Psychological and sociological approaches (pp. 75–146). New York, NY: Freeman. Eccles, J. S., & Wigfield, A. (2002). Motivational beliefs, values, and goals. Annual Review of Psychology, 53, 109 –132. http://dx.doi.org/10.1146/annurev.psych.53.100901.135153 Ehmke, T., Hohensee, F., Heidemeier, H., & Prenzel, M. (2004). Familiäre Lebensverhältnisse, Bildungsbeteiligung und Kompetenzerwerb [Family living conditions, educational participation, and ability acquisition] In M. Prenzel, J. Baumert, W. Blum, R. Lehmann, D. Leutner, M. Neubrand,... U. Schiefele (Eds.), Der Bildungsstand der Jugendlichen in Deutschland—Ergebnisse des zweiten internationalen Vergleichs [Educational attainment of adolescents in Germany—Results from the second international comparison] (pp. 225–254). Münster, Germany: Waxman. Enders, C. K., & Tofighi, D. (2007). Centering predictor variables in cross-sectional multilevel models: A new look at an old issue. Psychological Methods, 12, 121–138. http://dx.doi.org/10.1037/1082-989X.12.2.121 Frenzel, A. C., Goetz, T., Pekrun, R., & Watt, H. M. (2010). Development of mathematics interest in adolescence: Influences of gender, family, and school context. Journal of Research on Adolescence, 20, 507–537. http://dx.doi.org/10.1111/j.1532-7795.2010.00645.x Ganzeboom, H. B. G., & Treiman, D. J. (2003). Three internationally standardised measures for comparative research on occupational status. In J. H. P. Hoffmeyer-Zlotnik & C. Wolf (Eds.), Advances in crossnational comparison. A European working book for demographic and socio-economic variables (pp. 159 –193). New York, NY: Kluwer Academic Press. http://dx.doi.org/10.1007/978-1-4419-9186-7_9 Gaspard, H., Dicke, A.-L., Flunger, B., Brisson, B. M., Häfner, I., Nagengast, B., & Trautwein, U. (2015). Fostering adolescents’ value beliefs for mathematics with a relevance intervention in the classroom. Developmental Psychology, 51, 1226 –1240. http://dx.doi.org/10.1037/ dev0000028 Gaspard, H., Dicke, A.-L., Flunger, B., Häfner, I., Brisson, B. M., Trautwein, U., & Nagengast, B. (2016). Side effects of motivational interventions? Effects of a motivational intervention in math classrooms on motivation in verbal domains. AERA Open, 2, 1–14. http://dx.doi.org/ 10.1177/2332858416649168 Gaspard, H., Dicke, A.-L., Flunger, B., Schreier, B., Häfner, I., Trautwein, U., & Nagengast, B. (2015). More value through higher differentiation: Gender differences in value beliefs about math. Journal of Educational Psychology, 107, 663– 677. http://dx.doi.org/10.1037/edu0000003 Gaspard, H., Häfner, I., Parrisius, C., Trautwein, U., & Nagengast, B. (2017). Assessing task values in five subjects during secondary school: Measurement structure and mean level differences across grade level, gender, and academic subject. Contemporary Educational Psychology, 48, 67– 84. http://dx.doi.org/10.1016/j.cedpsych.2016.09.003 Grolnick, W. S., Friendly, R. W., & Bellas, V. M. (2009). Parenting and children’s motivation at school. In K. Wentzel, A. Wigfield, & D. Miele (Eds.), Handbook of motivation at school (pp. 279 –300). New York, NY: Routledge. Guo, J., Nagengast, B., Marsh, H. W., Kelava, A., Gaspard, H., Brandt, H.,... Trautwein, U. (2016). Probing the unique contributions of selfconcept, task values, and their interactions using multiple value facets and multiple academic outcomes. AERA Open, 2, 1–20. http://dx.doi.org/10.1177/2332858415626884 Häfner, I., Flunger, B., Dicke, A. L., Gaspard, H., Brisson, B. M., Nagengast, B., & Trautwein, U. (2017). The role of family characteristics for students’ scademic outcomes: A person-centered approach. Child Development. Advance online publication. http://dx.doi.org/10.1111/cdev.12809 Häfner, I., Hulleman, C. S., Harackiewicz, J. M., Rozek, C. S., Nagengast, B., Trautwein, U., & Hyde, J. S. (2016, April). STEM career paths from middle school to college: Parent and student interrelations. Paper presented at the Annual Meeting of the American Educational Research Association (AERA 2016), Washington, DC. Harackiewicz, J. M., Canning, E. A., Tibbetts, Y., Priniski, S. J., & Hyde, J. S. (2016). Closing achievement gaps with a utility-value intervention: Disentangling race and social class. Journal of Personality and Social Psychology, 111, 745–765. http://dx.doi.org/10.1037/pspp0000075 Harackiewicz, J. M., Rozek, C. S., Hulleman, C. S., & Hyde, J. S. (2012). Helping parents to motivate adolescents in mathematics and science: An experimental test of a utility-value intervention. Psychological Science, 23, 899 –906. http://dx.doi.org/10.1177/0956797611435530 Harackiewicz, J. M., Tibbetts, Y., Canning, E., & Hyde, J. S. (2014). Harnessing values to promote motivation in education. In S. A. Karabenick & T. C. Urdan (Eds.), Motivational interventions (pp. 71–105). Bingley, UK: Emerald Group Publishing Limited. http://dx.doi.org/10.1108/S0749-742320140000018002 Hasselmo, M. E., & Stern, C. E. (2006). Mechanisms underlying working memory for novel information. Trends in Cognitive Sciences, 10, 487– 493. http://dx.doi.org/10.1016/j.tics.2006.09.005 Hattie, J. A. C. (2009). Visible learning: A synthesis of over 800 metaanalyses relating to achievement. Oxford, UK: Routledge. Heller, K. A., & Perleth, C. (2000). Kognitiver Fähigkeitstest für 4.-12. Klassen, Revision (KFT 4 –12⫹ R) [Cognitive abilities test for Grades 4 to 12, revised version (KFT 4 –12 ⫹R)]. Göttingen, Germany: Hogrefe. Hidi, S., & Renninger, K. A. (2006). The four-phase model of interest development. Educational Psychologist, 41, 111–127. http://dx.doi.org/ 10.1207/s15326985ep4102_4 Hillmert, S., & Jacob, M. (2005). Institutionelle Strukturierung und interindividuelle Variation [Institutional allocation and inter-individual variation]. KZfSS Kölner Zeitschrift für Soziologie und Sozialpsychologie, 57, 414 – 442. http://dx.doi.org/10.1007/s11577-005-0183-8 Hillmert, S., & Jacob, M. (2010). Selections and social selectivity on the academic track: A life-course analysis of educational attainment in Germany. Research in Social Stratification and Mobility, 28, 59 –76. http://dx.doi.org/10.1016/j.rssm.2009.12.006 Hulleman, C. S., Godes, O., Hendricks, B. L., & Harackiewicz, J. M. (2010). Enhancing interest and performance with a utility value intervention. Journal of Educational Psychology, 102, 880 – 895. http://dx.doi.org/10.1037/a0019506 This document is copyrighted by the American Psychological Association or one of its allied publishers. This article is intended solely for the personal use of the individual user and is not to be disseminated broadly. ROBIN HOOD EFFECTS Hulleman, C. S., & Harackiewicz, J. M. (2009). Promoting interest and performance in high school science classes. Science, 326, 1410 –1412. http://dx.doi.org/10.1126/science.1177067 Hyde, J. S., Canning, E. A., Rozek, C. S., Clarke, E., Hulleman, C. S., & Harackiewicz, J. M. (2016). The role of mothers’ communication in promoting motivation for math and science course-taking in high school. Journal of Research on Adolescence, 27, 49 – 64. http://dx.doi.org/10.1111/jora.12253 ILO. (2012). International standard classification of occupations. ISCO08. Geneva, Switzerland: Author. Jacobs, J. E., & Eccles, J. S. (2000). Parents, task values, and real-life achievement-related choices. In C. Sansone & J. M. Harackiewicz (Eds.), Intrinsic and extrinsic motivation: The search for optimal motivation and performance (pp. 405– 439). San Diego, CA: Academic Press. http://dx.doi.org/10.1016/B978-012619070-0/50036-2 Jacobs, J. E., Lanza, S., Osgood, D. W., Eccles, J. S., & Wigfield, A. (2002). Changes in children’s self-competence and values: Gender and domain differences across grades one through twelve. Child Development, 73, 509 –527. http://dx.doi.org/10.1111/1467-8624.00421 Jodl, K. M., Mic