Review Of Statistics & Probability PDF
Document Details
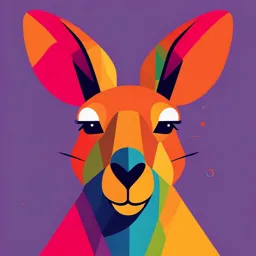
Uploaded by FortuitousHeliotrope7275
Tags
Related
- Statistics and Probability STAT 303 PDF
- AEC301 Probability Theory 2021 PDF
- Gandhinagar Institute of Technology Question Bank PDF 2024-2025
- Probability and Statistics Course Outline (2024/2025)
- Nile College Statistics Test No. 4 PDF
- Buting Senior High School 2nd Quarter Statistics & Probability Reviewer PDF
Summary
This document reviews basic concepts of statistics and probability, for 5th form students. It covers topics like probability definitions and rules, types of probability (objective and subjective), set notation, examples and more, but is not a past paper.
Full Transcript
Review of Concepts … Statistics -- Probability 1 Scenario You have been invited to speak to some 5th Form students on the topic: What is Statistics – the basic elements and purpose? You are given 5 minutes to speak. 2...
Review of Concepts … Statistics -- Probability 1 Scenario You have been invited to speak to some 5th Form students on the topic: What is Statistics – the basic elements and purpose? You are given 5 minutes to speak. 2 Probability Basic Definitions: Events, Sample Space, and Probabilities Basic Rules for Probability Conditional Probability Independence of Events Combinatorial Concepts (used, not taught) The Law of Total Probability and Bayes’ Theorem 3 Probability is: A measure of uncertainty A measure of the strength of belief in the occurrence of an uncertain event A measure of the degree of chance or likelihood of occurrence of an uncertain event Measured by a number between 0 and 1 (or between 0% and 100%) 4 Types of Probability Objective or Classical Probability – based on equally-likely events – based on long-run relative frequency of events – not based on personal beliefs – is the same for all observers (objective) – examples: toss a coins, throw a die, pick a card Subjective Probability – based on personal beliefs, experiences, prejudices, intuition - personal judgment – different for all observers (subjective) – examples: World Cup, elections, new product introduction, rainfall, hurricane 5 Probability & Set Notation Set - a collection of elements or objects of interest – Empty set (denoted by ) a set containing no elements – Universal set (denoted by X or S) a set containing all possible elements – Complement (Not). The complement of A is A – a set containing all elements of X not in A 6 Basic Definitions –Intersection (And) A B – a set containing all elements in both A and B, phrases like and, joint occurrence, happening at the same time, simultaneously, both, etc, all mean intersection. – For intersection we multiply. Union (Or) AB – a set containing all elements in either A, or B or both A and B – Phrases like either, or, at least, at most and similar types mean union – For union we add 7 Basic Definitions Mutually exclusive or disjoint sets –sets having no elements in common, having no intersection, …its intersection is the empty set. Cannot happen at the same time Partition –a collection of mutually exclusive sets which together include all possible elements, whose union is the universal set (collectively exhaustive) 8 Experiment Process that leads to one of several possible outcomes *, e.g.: – Coin toss Heads, Tails – Throw die 1, 2, 3, 4, 5, 6 – Pick a card AH, KH, QH,... – Introduce a new product Each trial of an experiment has a single observed outcome. The precise outcome of a random experiment is unknown before a trial. * Also called a basic outcome, elementary event, or simple event 9 Events : Definition Sample Space or Event Set – Set of all possible outcomes (universal set) for a given experiment E.g.: Throw die – X = (1,2,3,4,5,6) Event (Compound) – Collection of outcomes having a common characteristic E.g.: Even number – A = (2,4,6) What is the probability of getting an even # when a die is tossed? – Event A occurs if an outcome in the set A occurs Probability of an event – Sum of the probabilities of the outcomes of which it consists: P(A) = P(2) + P(4) + P(6) – The relative size of the event with respect to the size of the sample space. [This is the operative definition] 10 Equally-likely Probabilities (Hypothetical or Ideal Experiments) For example: – Throw a die Six possible outcomes (1,2,3,4,5,6) If each is equally-likely, the probability of each is 1/6 = 0.1667 = 16.67% 1 P ( e) n( X ) Probability of each equally-likely outcome is 1 over the number of possible outcomes – Event A (even number) P(A) = P(2) + P(4) + P(6) = 1/6 + 1/6 + 1/6 = 1/2 P( A) P(e) for e in A n( A) 3 1 n( X ) 6 2 11 Basic Rules for Probability 1. Range of Values 0 P( A) 1 2. Complements - Probability of not A P( A) 1 P( A) 3. Intersection - Probability of both A and B P( A B) n( A B) n( S ) 4. Mutually exclusive events (A and C) : P( A C) 0 12 Basic Rules for Probability 5. Union - Probability of A or B or both P( A B) n( A B) P( A) P( B) P( A B) n( S ) P( A B C) P( A) P(B) P(C) P( A B) P( AC) P(B C) P( A B C) P( A B C D) P( A) P(B) P(C) P(D) P( A B) P( AC) P( A D) P(B C) P(B D) P(C D) P( A B C) P( A B D) P(B C D) P( A B C D) 13 Basic Rules for Probability 6. Conditional Probability - Probability of A given B P( A B) P( A B) P( B) The probability that A occurs given that B has already occurred. We also use if and suppose. We can manipulate this formula to change the subject 14 Conditional Probability 6. Rules of conditional probability: a) P( A B) P( A B) so P( A B) P( A B)P(B) P(B) b) P(B A) P( A B) so P( A B) P(B A)P( A) P( A) 6c) P( A B) P( A B)P(B) 6d) P( A B) P(B A)P( A) 15 Contingency Table (Example) As part of a drive to modernize the economy, the government of an Eastern European country is pushing for starting 100 new projects in computer development and telecommunications. Two U.S. giants, IBM and AT&T, have signed contracts for these projects: 40 projects for IBM and 60 projects for AT&T. Of the IBM projects, 30 are in the computer area and 10 are in telecommunications. Of the AT&T projects, 20 are in the computer area and 40 are in telecommunications. What is the probability that a project is undertaken by IBM given it is a telecommunications project? 16 Contingency Table (Example) Define C = the event the project is in computer development T = the event the project is in telecommunications. I = the event the project is undertaken by IBM and A = the event the project is undertaken by AT&T, Given: P(I) = 0.40; P(A) = 0.60; P(I∩T) = 0.10; P(I∩C) = 0.30 P(T) = 0.50; P(A∩T) = 0.40; P(A∩C) = 0.20 P(C|I) = 0.75; P(T|I) = 0.25 (can you prove this?) P(C|A) = 1/3 ; P(T|A) = 2/3 (can you prove this?) Question: P(I|T) =? 17 Independence of Events Conditions for the statistical independence of events A and B: a) P( A B) P( A) b) P( B A) P( B) c) P( A B) P( A) P( B) P( A B) P( A B)P(B) Instead of P(A|B) use P(A) P( A B) P(B A)P( A) Instead of P(B|A) use P(B) 18 Independence (Example) The probability that a consumer will be exposed to a TV ad for a certain product is 0.04. The probability that the consumer will be exposed to a billboard ad for the same product is 0.06. The two events, being exposed to the TV commercial and being exposed to the billboard ad, are assumed to be independent. (a) What is the probability the consumer will be exposed to both ads? (b) What is the probability that he or she will be exposed to at least one of the two ads? 19 Independence of Events (Example) Define T as the event the ad is seen on Television and B as the event the ad is seen on Billboard. Given P(T) = 0.04; P(B) = 0.06 These are assumed to be independent. a) P ( T B ) P ( T ) P ( B ) 0.04 * 0.06 0.0024 b) P ( T B ) P ( T ) P ( B ) P ( T B ) 0.04 0.06 0.0024 0.0976 20 Product Rules for Independent Events The probability of the intersection of several independent events is the product of their separate individual probabilities: P( A A A An ) P( A ) P( A ) P( A ) P( An ) 1 2 3 1 2 3 The probability of the union of several independent events is 1 minus the product of probabilities of their complements: P( A A A An ) 1 P( A ) P( A ) P( A ) P( An ) 1 2 3 1 2 3 21 The Law of Total Probability and Bayes’ Theorem When one event depends on more than one other (for example, if A depends on B and its complement): P( A) P( A B) P( A B ) In terms of conditional probabilities: P( A) P( A B) P( A B ) P( A B) P( B) P( A B ) P( B ) Since P( A B) P( A B)P(B) and P( A B ) P( A B )P(B ) 22 The Law of Total Probability and Bayes’ Theorem When one event depends on more than two (for example, if A depends on B, C and D): P( A) P( A B) P( AC) P( A D) In terms of conditional probabilities: P( A) P( A B)P(B) P( AC)P(C) P( A D)P(D) More generally (where Bi make up a partition): P( A) P( A B ) i P( AB ) P( B ) i i 23 The Law of Total Probability and Bayes’ Theorem In a takeover bid for a certain firm, the management of the raiding firm believes there is a 0.75 chance of success (successful takeover) if a member of the raided firm resigns, and there is a 0.30 chance of success if the member does not resign. They also believe that there is a 0.70 chance of the member resigning. What is the probability of a successful takeover? We can see that in this example, success, which is taking over the firm depends on two events – the board member resigning and the board member not resigning (complements) 24 Solution Let B = the event of a successful takeover A = the event of the member of the board resigns A the event of the member of the board does not resign Then B ( B A) ( B A) But we know from the given information that: P( B | A) 0.75 P( B | A ) 0.30 P( A) 0.70 P ( A ) 0.30 So we can solve like: P ( B) P ( B | A) * P ( A) P ( B | A ) * P ( A ) 0.75 * 0.7 0.3 * 0.3 0.615 25 Example 2: The Law of Total Probability If you do not study for QM, the probability of passing the course is 10%, if you studied “lightly”, the probability of passing the course is 50%, and if you studied “intensely”, the probability of passing the course is 85%. We can see that passing the course depends on three events: (1) Do not study (N), (2) studying “lightly” (L), and (3) studying “intensely” (I). To determine the probability of passing the course (S), we take into account the probability of all three events occurring. Suppose, we know that P(N) = 0.05, P(L) = 0.25, and P(I) = 0.7, what is P(S)? 26 Example 2 -- Solution N L I S S∩N S∩L S∩I We know from the Venn diagram that: S = (S∩N) + (S∩L) + (S∩I) We also know from the given information that: P(S|N) = 0.10; P(S|L) = 0.50; P(S|I) = 0.85; P(N) = 0.05; P(L) = 0.25 and P(I) = 0.70 Which is not like the Venn diagram, but P (S∩N) = P(S|N)*P(N); P (S∩L) = P(S|L)*P(L); P (S∩I) = P(S|I)*P(I) 27 Example 3: The Law of Total Probability An analyst believes the stock market (index) has a 0.75 probability of going up in the next year if the economy should do well, and a 30% probability of going up in the next year if the economy should not do well in the year. The analyst further believes the economy has a 0.80 probability of doing well in the coming year. Using the analyst’s assessments, calculate the probability that the stock market will go up in the next year? 28 Example 3 -- Solution Event U: Stock market will go up in the next year Event W: Economy will do well in the next year P(U W ) 0.75 P(U W ) 0.30 P(W ) 0.80 P(W ) 1 0.8 0.2 P(U ) P(U W ) P(U W ) P(U W )P(W ) P(U W )P(W ) (0.75)(0.80) (0.30)(0.20) 0.60 0.06 0.66 29 Bayes’ Theorem Bayes’ theorem enables you, knowing just a little more than the probability of A given B, to find the probability of B given A. Based on the definition of conditional probability and the law of total probability. Allows us to update or revise the probability of an event, after some other event has occurred. It does so by reversing the order of conditionality. That is, if we need P(B|A) we would have the information on P(A|B). Notice how the positions of A and B have changed. 30 Bayes’ Theorem The new probability of B, P(B|A) is called the posterior probability, posterior to A occurring. We would have information on the prior probability of B, P(B) & P(A|B). 9. Revising probabilities after some other event has occurred: P( A B) P( B A) P( A) P( A B) P( B) Applying the definition of conditional probability throughout P( A B) P( B) P( A B ) P( B ) 31 Example 4: Bayes’ Theorem A medical test for a rare disease affecting 0.1% of the population is imperfect. : – When administered to an ill person, the test will indicate so (positive result) with probability 0.92. – (The event the test result is negative if the person is ill is a false negative.) – When administered to a person who is not ill, the test will erroneously give a positive result (false positive) with probability 0.04 If a person is randomly tested from the entire population and the result is positive, what is the probability that the person is ill (posterior probability - posterior to the test result)? 32 Solution – Example 4 Let Z = the event the test is positive and I = the event the person is ill P(I) = 0.001; P( Z I ) 0.92 P( Z I ) 0.08 P(Z|I) = 0.92 P( Z | I ) 0.04 P( Z I ) 0.04 P( Z I ) 0.96 Question P(I|Z) =? P(I|Z) = P(Z∩I)/P(Z) 33 Solution – Example 4 (continued) P( I ) 0.001 P( I Z ) P( I Z ) P( I ) 0.999 P( Z ) P( Z I ) 0.92 P( Z I ) P( I ) P( Z I ) 0.04 P( Z I ) P( I ) P( Z I ) P( I ) (0.92)(0.001) (0.92)(0.001) (0.04)(0.999) 0.00092 0.00092 0.00092 0.03996 0.04088 0.0225 34 Bayes’ Theorem Extended Given a partition of events B1,B2 ,...,Bn: P( A B ) P( B A) 1 1 P( A) P( A B ) 1 Applying the law of total P( A B ) i probability to the denominator P( A B ) P( B ) 1 1 Applying the definition of P( A B ) P( B ) i i conditional probability throughout 35 Example 5 An economist believes that during periods of high economic growth, the U.S. dollar appreciates with probability 0.70; in periods of moderate economic growth, the dollar appreciates with probability 0.40; and during periods of low economic growth, the dollar appreciates with probability 0.20. During any period of time, the probability of high economic growth is 0.30, the probability of moderate economic growth is 0.50, and the probability of low economic growth is 0.20. Suppose the dollar has been appreciating during the present period. What is the probability we are experiencing a period of high economic growth? Partition: Event A Appreciation H - High growth P(H) = 0.30 P ( A H ) 0.70 M - Moderate growth P(M) = 0.50 P ( A M ) 0.40 L - Low growth P(L) = 0.20 P ( A L) 0.20 36 Solution – Example 5 P( A H ) P( H ) P ( H A) P ( A H ) P ( H ) P ( A M ) P ( M ) P ( A L) P ( L) (0.70)(0.30) (0.70)(0.30) (0.40)(0.50) (0.20)(0.20) 0.21 0.21 0.21 0.20 0.04 0.45 0.467 37 Complete from Example 5 1. Suppose the dollar has been appreciating during the present period. What is the probability we are experiencing a period of medium economic growth? 2. Suppose the dollar has been appreciating during the present period. What is the probability we are experiencing a period of Low economic growth? 38