Probability & Statistics 2024-2025 PDF
Document Details
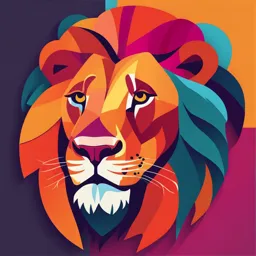
Uploaded by StatuesqueMaple
Koya University
2024
Dr. Azhin T. Sabir
Tags
Related
- Statistics And Probability STAT 303 PDF
- The Nature of Probability and Statistics PDF
- JG University Probability and Statistics Sample Mid-Term Exam Paper 2023-2024 PDF
- JG University Probability and Statistics Mid-Term Exam 2023-24 PDF
- Chapter 8 - Statistics and Probability PDF
- Mathematics of Data Management Notes (Lower Canada College)
Summary
These notes are from a Probability & Statistics course at Koya University, 2024-2025. The course covers topics including data types (qualitative and quantitative), sampling techniques, and descriptive and inferential statistics. The materials will be useful for undergraduate students studying these areas of mathematics.
Full Transcript
Probability & Statistics Dr. Azhin T. Sabir 2024-2025 Probability and Statistics Probability and Statistics form the basis of Data Science. The probability theory is very much helpful for making the prediction. Estimates and predictions form an important part of Data science. With...
Probability & Statistics Dr. Azhin T. Sabir 2024-2025 Probability and Statistics Probability and Statistics form the basis of Data Science. The probability theory is very much helpful for making the prediction. Estimates and predictions form an important part of Data science. With the help of statistical methods, we make estimates for the further analysis. Thus, statistical methods are largely dependent on the theory of probability. And all of probability and statistics is dependent on Data. What Is Data? Look around you, there is data everywhere. Each click on your phone generates more data than you know. This generated data provides visions for analysis and helps us make better business decisions. This is why data is so important. Data — a collection of facts (numbers, words, measurements, observations, etc) that has been translated into a form that computers can process. Data can be collected, measured and analyzed. It can also be visualized by using statistical models and graphs Categories Of Data Data can be categorized into two sub-categories: Qualitative Data Quantitative Data Refer the below figure to understand the different categories of data: Qualitative Data Qualitative data deals with characteristics and descriptors that can’t be easily measured, but can be observed subjectively. Qualitative data is further divided into two types of data: Nominal Data: Data with no inherent order or ranking sequence such as gender or race Ordinal Data: Data with an ordered series of information is called ordinal data Quantitative Data: Quantitative data deals with numbers and things you can measure objectively. This is further divided into two: Discrete Data: Also known as categorical data, it can hold a finite number of possible values. Example: Number of students in a class. Continuous Data: Data that can hold an infinite number of possible values. Example: Weight of a person. Why does Data Matter? Helps in understanding more about the data by identifying relationships that may exist between 2 variables. Helps in predicting the future or forecast based on the previous trend of data. Helps in determining patterns that may exist between data. Helps in detecting fraud by uncovering abnormality in the data. What is statistics ? Statistics is an area of applied mathematics concerned with data collection, analysis, interpretation, and presentation. Statistics permeates all aspects of life from education, work, media, and health, to citizenship. What is statistics ? This area of mathematics deals with understanding how data can be used to solve complex problems. Here are a couple of example problems that can be solved by using statistics: Your company has created a new drug that may cure cancer. How would you conduct a test to confirm the drug’s effectiveness? The latest sales data have just come in, and your boss wants you to prepare a report for management on places where the company could improve its business. What should you look for? What should you not look for? Statistical studies Statistical studies can be classified as: Observational study: is where researchers only observe characteristics and take measurements (i.e. smoker vs. non- smoker to observe the relationship between smoking and lung cancer). Designed experiment: is where researchers impose treatments and controls then observe characteristics and take measurements (i.e. observe the effect of taking folic acid on birth defects by comparing two groups of women taking folic acid vs. placebo). Basic Terminologies In Statistics Before you dive deep into Statistics, it is important that you understand the basic terminologies used in Statistics. The two most important terminologies in statistics are population and sample Population: A collection or set of individuals or objects or events whose properties are to be analysed. Sample: A subset of the population is called ‘Sample’. A well-chosen sample will contain most of the information about a particular population parameter. Sampling Techniques Sampling is a statistical method that deals with the selection of individual observations within a population. It is performed to infer (conclude) statistical knowledge about a population. In a study to test the efficacy of a new drug on diabetics in UK, one cannot evaluate the entire population, rather a group are selected. There are two main types of Sampling techniques: Probability Sampling Non-Probability Sampling Here we’ll be focusing only on probability sampling techniques because non-probability sampling is not within the scope of this course. Probability Sampling: This is a sampling technique in which samples from a large population are chosen using the theory of probability. There are three types of probability sampling: Random Sampling: In this method, each member of the population has an equal chance of being selected in the sample. Systematic Sampling Systematic Sampling: In Systematic sampling, every nth record is chosen from the population to be a part of the sample. Refer the below figure to better understand how Systematic sampling works Systematic Sampling Stratified Sampling Stratified Sampling: In Stratified sampling, a stratum (category) is used to form samples from a large population. A stratum is a subset of the population that shares at least one common characteristic. After this, the random sampling method is used to select a sufficient number of subjects from each stratum Frequency Frequency of an item refers to the number of observations of that item. Frequency distribution: is a listing of all items and their frequencies. Relative frequency: is the ratio of the frequency of an item to the total number of observations. Relative frequency distribution: is a listing of all items and their relative frequencies. Types Of Statistics There are two well-defined types of statistics: Descriptive Statistics Inferential Statistics Descriptive Statistics Descriptive statistics is a method used to describe and understand the features of a specific data set by giving short summaries about the sample and measures of the data. Descriptive Statistics is mainly focused upon the main characteristics of data. It provides a graphical summary of the data. Data can be described or summarized as: Numerical summary : i.e. mean, range, standard deviation, variance, etc... graphical summary : i.e. Bar chart/histogram, pie chart, scatter chart. Descriptive Statistics Suppose you want to gift all your classmate’s t-shirts. To study the average shirt size of students in a classroom, in descriptive statistics you would record the shirt size of all students in the class and then you would find out the maximum, minimum and average shirt size of the class. Inferential Statistics Inferential statistics makes inferences (conclusions) and predictions about a population based on a sample of data taken from the population in question. Inferential statistics generalizes a large dataset and applies probability to draw a conclusion. It allows us to infer data parameters based on a statistical model using sample data. Inferential Statistics So, if we consider the same example of finding the average shirt size of students in a class, in Inferential Statistics, you will take a sample set of the class, which is basically a few people from the entire class. You already have had grouped the class into large, medium and small. In this method, you basically build a statistical model and expand it for the entire population in the class. Measures of location Mean: Measure of the average of all the values in a sample is called Mean. Median: Measure of the central value of the sample set is called Median. Mode: The value most recurrent in the sample set is known as Mode. Measures of location: mean Mean (average): sum of all observations divided by the number of the observations. The mean of sample is denoted by x. The mean of population is denoted by μ. Let X be a random variable. A random sample is an array [x1, x2,..., xn], the mean of the sample is given by: n x i x= i =1. n Example: X=[4 , 3 , 7, 6, 1 , 3 ] where n=6 4 + 3 + 7 + 6 +1+ 3 x = =4 6 Measures of location: median Median: element located in the middle of the list after sorting in ascending order. - If the number of observations is odd, then the median is exactly in the middle of the ordered list. - If the number of observations is even, then the median is the mean of the two observations in the middle of the ordered list. Example: Let X be a random variable. X=[41, 56, 33, 16, 23, 45, 39] Sorted sample X=[16, 23, 33, 39, 41, 45, 56] median (X) = 39 Measures of location: mode Mode: element in the sample with the greatest frequency. - if no observation occurs more than once then the data has no mode. Example: X=[5 , 6, 3, 6, 2, 5, 7, 5, 1] Mode (X) = 5 Measures of location Example Q. The weekly incomes (£) of a random sample of self-employed window cleaners are: [175, 185, 160, 185,165, 195, 205, 185, 170, 250] A. For the calculation of mode and median we need the sorted list: [160, 165, 170, 175,185, 185, 185, 195, 205, 250] The mean is 175 + 185 + 160 + 185 + 165 + 195 + 205 + 185 + 170 + 250 x = 10 = £187.50. The sample Mode is £185.00, and The Sample Median is £185.00. Measures of dispersion Averages do not give the complete picture about the observed sample values and may be misleading. If you stick one foot in a bucket of boiling water and the other in a bucket of melting ice, how much is comforting to you if are told the average temperature in the two buckets is 50°C Statistics is about collecting and analysing data that vary. Measuring dispersion of data about the average (the sample mean) is a good indicator of data variation. Different measures of dispersion include: Range Inter-quartile range Standard deviation Variance Coefficient of variation Range The range of the observed values is defined as range = max- min. The two charts show the pulse rate per minute (x-axis) against no. of students (y-axis) for two cohorts of 50 students. The first set of data are more dispersed than that in the second sample The pulse rate in sample 1 is in the range 96-62, i.e. range=34. The pulse rate in sample 2 is in the range 88-70, i.e. range=18. Sample standard deviation Standard deviation indicates how far, on average, the observations in the sample from the mean of the sample. The standard deviation of sample is denoted by S. The standard deviation of population is denoted by σ. For a random sample [x1, x2,..., xn], STD is defined as follows: n n (x − x) i =1 i 2 (x i =1 2 i ) − nx 2 = or =. n −1 n −1 Definition xi (xi- mean)^2 175 156.25 The weekly incomes (£) of a random sample of 185 6.25 self-employed window cleaners : 160 756.25 [175, 185, 160, 185,165, 195, 205, 185, 170, 250] 185 6.25 165 506.25 Stdev = 25.85 195 56.25 205 306.25 185 6.25 170 306.25 250 3906.25 Total 1875 6012.5 Mean 187.5 Stdev. 25.85 Variance Variance is the square of the standard deviation. It is defined as σ2. n i =1 ( xi − x ) 2 2 = n −1 It is advised for non-statistician to use standard deviation instead of variance. In the weekly income for window cleaners example, the variance of the sample could be calculated as 2 = (25.85)2 = 668.055. Coefficient of variation 100 S S The. coefficient of variance is defined as or as percentage. x x The coefficient of variation is a dimensionless number. This is used when comparing between data sets with different units or widely different means (e.g. it wouldn't make sense to compare the SD of blood pressure with the SD of pulse rate, but it might make sense to compare the two CV values). In the weekly income for window cleaners example: coefficient of variance = (100 x 25.85)/187.5= 13.785. Inter-quartile range Inter-quartile range: the difference between the first and the third quartiles. IQR= Q3-Q1 Just as the median splits the data into 2 halves, the quartiles are the values that split the data into 4 quarters. First sort the data in ascending order. Define the lower-quartile QL =Q1 to be the entry in position int((n+1)/4), and the upper quartile QU =Q3 to be the entry in position int(3(n+1)/4). The inter-quartile range is defined as QU – QL. In the weekly income for window cleaners example: [160, 165, 170, 175,185, 185, 185, 195, 205, 250] QL is the entry in position int((10+1)/4)=2, i.e. QL = 165 QU is the entry in position int(3(10+1)/4)=8, i.e. Qu = 195. hence IQR= 195– 165 = 30. Boxplot (box and whisker diagram) Boxplot is a graphical display of the centre and variation of a data set. Example On average, haemoglobin levels in (1) and (3) are the same. The variation of (3) is the greatest among the three groups. End