Practical Research 2 - Module 5 PDF
Document Details
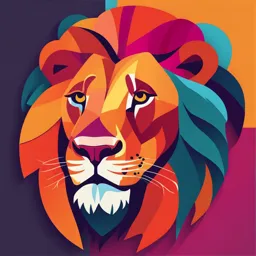
Uploaded by HardyIrrational3171
Consolacion National High School
Tags
Summary
This document details the process of gathering and measuring information in an established systematic fashion. It covers various methods of data collection, such as questionnaires, interviews, and observations, alongside the use of statistical analysis to evaluate data and draw conclusions. It's appropriate for educational contexts focusing on research methods, especially in universities and colleges.
Full Transcript
## Module 5: Finding Answers Through Data Collection ### Introduction Data collection is the process of gathering and measuring information on variables of interest in an established systematic fashion that enables one to answer stated research questions, test hypotheses, and evaluate outcomes. Th...
## Module 5: Finding Answers Through Data Collection ### Introduction Data collection is the process of gathering and measuring information on variables of interest in an established systematic fashion that enables one to answer stated research questions, test hypotheses, and evaluate outcomes. The data collection component of research is common to all fields of study including physical and social sciences, humanities, business, etc., while methods vary by discipline, the emphasis on ensuring accurate and honest collection remains the same. Craddick et.al (2003) ### Intended Learning Outcomes After this lesson, you should be able to: 1. Collect data using appropriate instruments. 2. Present and interpret data in tabular and graphical forms. 3. Use statistical techniques to analyze data - study of differences and relationships limited for bivariate analysis. 4. Use descriptive statistics in analyzing data. ### Performance Standard The learner is able to gather and analyze data with intellectual honesty, using suitable techniques. ## Lesson 12: Quantitative Data Analysis ### Quantitative Data Analysis It is a systematic approach to investigations during which numerical data is collected and/or the researcher transforms what is collected or observed into numerical data. It often describes a situation or event, answering the 'what' and 'how many' questions you may have about something. This is research which involves measuring or counting attributes (i.e., quantities). A quantitative approach is often concerned with finding evidence to either support or contradict an idea or hypothesis you might have. A hypothesis is where a predicted answer to a research question is proposed, for example, you might propose that if you give a a student training in how to use a search engine it will improve their success in finding information on the internet. You could then go on to explain why a particular answer is expected - you put forward a theory. We can gather quantitative data in a variety of ways and from a number of different sources. Many of these are similar to sources of qualitative data, for example: - **Questionnaires**: a series of questions and other prompts for the purpose of gathering information from respondents. - **Interviews**: a conversation between two or more people (the interviewer and the interviewee) where questions are asked by the interviewer to obtain information from the interviewee - a more structured approach would be used to gather quantitative data. - **Observations**: a group or single participants are manipulated by the researcher, for example, asked to perform a specific task or action. Observations are then made of their user behavior, user processes, workflows etc., either in a controlled situation (e.g., lab based) or in a real-world situation (e.g., the workplace). - **Transactional Logs**: recordings or logs of system on website activity. - **Documentary Research**: analysis of documents belonging to an organization. ### Why do we do quantitative data analysis? Once you have collected your data you need to make sense of the responses you have got back. Quantitative data analysis enables you to make sense of data by: - Organising them. - Summarising them. - Doing exploratory analysis. And to communicate the meaning to others by presenting data as: - Tables. - Graphical displays. - Summary statistics. We can also use quantitative data analysis to see: * Where responses are similar, for example, we might find that the majority of students all go to the university library twice a week. * If there are differences between the things we have studied, for example, 1st year students might go once a week to the library, 2nd year students twice a week, and 3rd year students three times a week. * If there is a relationship between the things we have studied. So, is there a relationship between the number of times a student goes to the library and their year of study? ### Using software for statistical analysis ### Some Key Concepts Before we look at types of analysis and tools we need to be familiar with a few concepts first: - **Population**: the whole units of analysis that might be investigated, this could be students, cats, house prices etc. - **Sample**: the actual set of units selected for investigation and who participate in the research. - **Variable**: characteristics of the units/participants. - **Value**: the score/label/value of a variable, not the frequency of occurrence. For example, if age is a characteristic of a participant then the value label would be the actual age, e.g., 21, 22, 25, 30, 18, not how many participants are 21, 22, 25, 30, 18. - **Case/Subject**: the individual unit/participant of the study/research. ### Sampling Sampling is complex and can be done in many ways dependent on 1) what you want to achieve from you research, 2) practical considerations of who is available to participate! The type of statistical analysis you do will depend on the sample type you have. Most importantly, you cannot generalize your findings to the population as a whole if you do not have a random sample. You can still undertake some inferential statistical analysis but you should report these as results of your sample, not as applicable to the population at large. Common sampling approaches include: - Random sampling - Stratified sampling - Cluster sampling - Convenience sampling - Accidental sampling ### Steps in Quantitative Data Analysis According to Baraceros (2016), she identified the different steps in Quantitative data analysis and she quoted that "no data organization means no sound data analysis". 1. **Coding system**: to analyze data means to quantify or change the verbally expressed data into numerical information. Converting the words, images, or pictures into numbers, they become fit for any analytical procedures requiring knowledge of arithmetic and mathematical computations. But it is not possible for the researcher to do the mathematical operations such as division, multiplication, or subtraction in the word level, unless you code the verbal responses and observation categories. For example: As regards gender variable, give number 1 as the code or value for Male and number 2 for Female. As to educational attainment as another variable, give the value of 2 for elementary; 4 for high school, 6 for college, 9 for M.A., and 12 for PhD level. By coding each item with a certain number in a data set, you are able to add the points or values of the respondent answers to a particular interview questionnaire item. ### Step 2: Analyzing the Data Data coding and tabulation are both essential in preparing the data analysis. Before you interpret every component of the data, the researcher decides first what kind of quantitative analysis to use - whether to use a simple descriptive statistical technique or an advance analytical method. The first one that college students often use tells some aspects of categories of data such as: frequency of distribution, measure of central tendency (mean, median, and mode), and standard deviation. However, this does not give information about population from where the sample came. The second one, on the other hand, fits graduate-level studies because this involves complex statistical analysis requiring a good foundation and thorough knowledge of the data-gathering instrument used. The results of the analysis reveal the following aspects of an item in a set of data (Mogan 2014; Punch 2014; Walsh 2010) cited by Baraceros (2016): - **Frequency distribution**: gives you the frequency of distribution and percentage of the occurrence of an item in a set of data. In other words, it gives you the number of responses given repeatedly for one question. **Question: ** By and large, do you find the Senators' attendance in 2015 legislative session awful? | Measurement Scale | Code | Frequency Distribution | Percent Distribution | | ------------- |:-------------:|:-------------:|:-------------:| | Strongly agree | 1 | 14 | 58% | | Agree | 2 | 3 | 12% | | Neutral | 3 | 2 | 8% | | Disagree | 4 | 1 | 4% | | Strongly disagree | 5 | 4 | 17% | - **Measure of Central Tendency**: Indicates the different positions or values of the items, so that in a category of data, you find an item or items serving as the: - **Mean**: average of all the items or scores - **Median**: the score in the middle of the set of items that cuts or divides the set into two groups - **Mode**: refers to the item or score in the data set that has the most repeated appearance in the set. - **Standard Deviation**: shows the extent of the difference of the data from the mean. An examination of this gap between the mean and the data gives you an idea about the extent of the similarities and differences between th respondents. There are mathematical operations that you have to determine the standard deviation. - Step 1: Compute the Mean. - Step 2: Compute the deviation (difference) between each respondent's answer (data item) and the mean. The positive sign (+) appears before the number if the difference is higher; negative sign (-), if the difference is lower. - Step 3: Compute the square of each deviation. - Step 4: Compute the sum of squares by adding the squared figures. - Step 5: Divide the sum of squares by the number of data items to get the variance. - Step 6: Compute the square root of variance figure to get standard deviation. **Example: ** Standard Deviation of the category of data collected from selected faculty members of one university. | Data Item | Deviation | Square of Deviation | | ------------- |:-------------:|:-------------:| | 1 | -8 | 68 | | 2 | -5 | 25 | | 6 | -1 | 1 | | 6 | -1 | 1 | | 8 | +1 | 1 | | 6 | -1 | 1 | | 6 | -1 | 1 | | 14 | +7 | 49 | | 16 | +9 | 81 | Total: 321 - Step 4) Sum of Squares: 321 - Step 5) Variance = 36 (321/9) - Step 6) Standard Deviation = 6 (square root of 6) - Step 1) Mean: 7 2. **Advanced Quantitative Analytical Methods**: An analysis of quantitative data that involves the use of more complex statistical methods needing computer software like the SPSS, STATA, or MINITAB, among others, occurs graduate-level students taking their MA or PhD degrees. Some of the advanced method of quantitative data analysis are the following (Argyous 2011; Levin & Fox 2014; Godwin 2014; as cited by Baraceros 2016) - **Correlation**: uses statistical analysis to yield results that describes the relationship of two variables. The results, however are incapable of establishing casual relationships. - **Analysis of Variance (ANOVA)**: is a statistical method used to test differences between two or more means. It may seem odd that the technique is called "Analysis of Variance" rather than "Analysis of Means." As you will see, the name is appropriate because inferences about means are made by analyzing variance. - **Regression**: In statistical modeling, regression analysis is a statistical process for estimating the relationships among variables. It includes many techniques for modeling and analyzing several variables, when the focus is on the relationship between a dependent variable and one or more independent variables (or 'predictors'). ## Lesson 13: Statistical Methods ### Basic Concept Statistics is a form of mathematical analysis that uses quantified models, representations and synopses for a given set of experimental data or real-life studies. Statistics studies methodologies to gather, review, analyze and draw conclusions from data. Statistical methods analyze large volumes of data and their properties. Statistics is used in various disciplines such as psychology, business, physical and social sciences, humanities, government and manufacturing. Statistical data is gathered using a sample procedure or other method. Two types of statistical methods are used in analyzing data: descriptive statistics and inferential statistics. Descriptive statistics are used to synopsize data from a sample exercising the mean or standard deviation. Inferential statistics are used when data is viewed as a subclass of a specific population. ### Statistical Methodologies 1. **Descriptive Statistics**: Descriptive statistics are brief descriptive coefficients that summarize a given data set, which can be either a representation of the entire population or a sample of it. Descriptive statistics are broken down into measures of central tendency and measures of variability, or spread. Measures of central tendency include the mean, median and mode, while measures of variability include the standard deviation or variance, and the minimum and maximum variables. 2. **Inferential Statistics**: Now, suppose you need to collect data on a very large population. For example, suppose you want to know the average height of all the men in a city with a population of so many million residents. It isn't very practical to try and get the height of each man. This is where inferential statistics comes into play. Inferential statistics makes inferences about populations using data drawn from the population. Instead of using the entire population to gather the data, the statistician will collect a sample or samples from the millions of residents and make inferences about the entire population using the sample. The sample is a set of data taken from the population to represent the population. Probability distributions, hypothesis testing, correlation testing and regression analysis are all fall under the category of inferential statistics. ### Types of Statistical Data Analysis 1. **Univariate Analysis**: analysis of one variable. 2. **Bivariate Analysis**: analysis of two variables (independent and dependent) 3. **Multivariate Analysis**: analysis of multiple relations between multiple variables. ### Statistical Methods of Bivariate Analysis According to the book of Baraceros (2016) bivariate analysis happens by means of the following methods (Argyrous 2011; Babbie 2013; Punch 2014) 1. **Correlation or Covariation** (correlated variation) - describes the relationship between two variables and also tests the strengths or significance of their linear relation. Covariance is the statistical term to measure the extent of the change in the relationship of two random variables. Random variables are data with varied values like those ones in the interval level or scale (Strongly disagree, disagree, neutral, agree, strongly agree) whose values depend on the arbitrariness of the respondents. 2. **Cross Tabulation**: is also called "crosstab or students-contingency table" that follows the format of a matrix that is made up of lines of numbers, symbols, and other expressions. Similar to one type of graph called table, matrix arranges data in rows and columns. If the table compares data on only two variables, such table is called Bivariate Table. **Example:** Secondary School Participants who attend the 1st UCNHS Research Conference | School | Male | Female | Row Total | | ------------- |:-------------:|:-------------:|:-------------:| | QMA | 152 (18.7%) | 127 (15.4%) | 279 | | UNCNHS | 120 (14.8%) | 98 (11.9%) | 218 | | PUNP | 59 (7.2%) | 48 (5.8%) | 107 | | UCU | 61 (7.5%) | 58 (7%) | 119 | | LNL | 81 (10%) | 79 (9.5%) | 159 | | U-Pang. | 79 (9.7%) | 99 (12%) | 178 | | CLLC | 102 (12.6%) | 120 (14.5%) | 222 | | ABE | 69 (8.5%) | 93 (11.3%) | 162 | | STI | 83 (10.2%) | 101 (12.2%) | 184 | | Column Total | 806 (100%) | 823 (100%) | 1,629 | ### Measure of Correlations Correlation is a bivariate analysis that measures the strengths of association between two variables and the direction of the relationship. In terms of the strength of relationship, the value of the correlation coefficient varies between +1 and -1. When the value of the correlation coefficient lies around ± 1, then it is said to be a perfect degree of association between the two variables. As the correlation coefficient value goes towards 0, the relationship between the two variables will be weaker. The direction of the relationship is simply the + (indicating a positive relationship between the variables) or - (indicating a negative relationship between the variables) sign of the correlation. Usually, in statistics, we measure four types of correlations: Pearson correlation, Kendall rank correlation, Spearman correlation, and the Point-biserial ### Pearson R Correlation Pearson r correlation is the most widely used correlation statistic to measure the degree of the relationship between linearly related variables. For example, in the stock market, if we want to measure how two stocks are related to each other, Pearson rcorrelation is used to measure the degree of relationship between the two. The Point-biserial correlation is conducted with the Pearson correlation formula except that one of the variables is dichotomous. The following formula is used to calculate the Pearson r correlation: $r = Pearson \ r \ correlation \ coefficient$ $N = number \ of \ value \ in \ each \ data \ set$ $∑xy = sum \ of \ the \ products \ of \ paired \ scores$ $∑x = sum \ of \ x \ scores$ $∑y = sum \ of \ y \ scores$ $x2 = sum \ of \ squared \ x \ scores$ $∑y2 = sum \ of \ squared \ y \ scores$ Types of research questions a Pearson correlation can examine: - Is there a statistically significant relationship between age, as measured in years, and height, measured in inches? - Is there a relationship between temperature, measure in degree Fahrenheit, and ice cream sales, measured by income? - Is there a relationship among job satisfaction, as measured by the JSS, and income, measured in dollars? ### Assumptions For the Pearson r correlation, both variables should be normally distributed (normally distributed variables have a bell-shaped curve). Other assumptions include linearity and homoscedasticity. Linearity assumes a straight line relationship between each of the variables in the analysis and homoscedasticity assumes that data is normally distributed about the regression line. ### Conduct and Interpret a Pearson Correlation **Key Terms** - **Effect size**: Cohen's standard will be used to evaluate the correlation coefficient to determine the strength of the relationship, or the effect size, where correlation coefficients between .10 and .29 represent a small association, coefficients between .30 and .49 represent a medium association, and coefficients of .50 and above represent a large association or relationship. - **Continuous data**: Data that is interval or ratio level. This type of data possesses the properties of magnitude and equal interval between adjacent units. Equal intervals between adjacent units' means that there are equal amounts of the variable being measured between adjacent units on the scale. An example would be age. An increase in age from 21 to 22 would be the same as an increase in age from 60 to 61. ### Kendall Rank Correlation Kendall rank correlation is a non-parametric test that measures the strength of dependence between two variables. If we consider two samples, a and b, where each sample size is n, we know that the total number of pairings with a b is n(n-1)/2. The following formula is used to calculate the value of Kendall rank correlation: $Nc = number \ of \ concordant$ $Nd = number \ of \ discordant$ ### Conduct and Interpret a Kendall Correlation **Key Terms** - **Concordant**: Ordered in the same way. - **Discordant**: Ordered differently. ### Spearman Rank Correlation Spearman rank correlation is a non-parametric test that is used to measure the degree of association between two variables. It was developed by Spearman, thus it is called the Spearman rank correlation. Spearman rank correlation test does not assume any assumptions about the distribution of the data and is the appropriate correlation analysis when the variables are measured on a scale that is at least ordinal. The following formula is used to calculate the Spearman rank correlation: $P = Spearman \ rank \ correlation$ $di = the \ difference \ between \ the \ ranks \ of \ corresponding \ values \ Xi \ and \ Yi$ $n = number \ of \ value \ in \ each \ data \ set$ ### Questions Spearman Correlation Answers - Is there a statistically significant relationship between participants' responses to two Likert scales questions? - Is there a statistically significant relationship between how the horses rank in the race and the horses' ages? ### Assumptions Spearman rank correlation test does not make any assumptions about the distribution. The assumptions of Spearman rho correlation are that data must be at least ordinal and sores on one variable must be montonically related to the other variable. ### Conduct and Interpret a Spearman Correlation **Key Terms** - **Effect size**: Cohen's standard will be used to evaluate the correlation coefficient to determine the strength of the relationship, or the effect size, where coefficients between .10 and .29 represent a small association; coefficients between .30 and .49 represent a medium association; and coefficients of .50 and above represent a large association or relationship. - **Ordinal data**: Ordinal scales rank order the items that are being measured to indicate if they possess more, less, or the same amount of the variable being measured. An ordinal scale allows us to determine if X > Y, Y > X, or if X = Y. An example would be rank ordering the participants in a dance contest. The dancer who was ranked one was a better dancer than the dancer who was ranked two. The dancer ranked two was a better dancer than the dancer who was ranked three, and so on. Although this scale allows us to determine greater than, less than, or equal to, it still does not define the magnitude of the relationship between units. ### Chi-Square Is the statistical test for bivariate analysis of nominal variables, specifically, to test the null hypothesis. It tests whether or not a relationship exists between or among variables and tells the probability that the relationship is caused by chance. This cannot in any way show extent of the association between two variables. ### Types of Data There are basically two types of random variables and they yield two types of data: numerical and categorical. A chi square (X²) statistic is used to investigate whether distributions of categorical variables differ from one another. Basically categorical variable yield data in the categories and numerical variables yield data in numerical form. Responses to such questions as "What is your major?" or Do you own a car?" are categorical because they yield data such as "biology" or "no." In contrast, responses to such questions as "How tall are you?" or "What is your G.P.A.?" are numerical. Numerical data can be either discrete or continuous. The table below may help you see the differences between these two variables. | Data type | Question Type | Possible Responses | | ------------- |:-------------:|:-------------:| | Categorical | What is your sex? | Male or female | | Numerical | Discrete - How many cars do you own? | Two or three | | Numerical | Continuous - How tall are you? | 72 inches | Notice that discrete data arise from a counting process, while continuous data arise from a measuring process. The Chi Square statistic compares the tallies or counts of categorical responses between two (or more) independent groups. (Note: Chi square tests can only be used on actual numbers and not on percentages, proportions, means, etc.) ## 2 x 2 Contingency Table There are several types of chi square tests depending on the way the data was collected and the hypothesis being tested. We'll begin with the simplest case: a 2 x 2 contingency table. If we set the 2 x 2 table to the general notation shown below in Table 1, using the letters a, b, c, and d to denote the contents of the cells, then we would have the following table: **Table 1. General notation for a 2 x 2 contingency table.** | | | | | -------- | -------- | -------- | | **Variable 2** | **Data type 1** | **Data type 2** | | Category 1 | A | B | | Category 2 | C | D | | Total | a+c | b+d | For a 2 x 2 contingency table the Chi Square statistic is calculated by the formula: $x² = N[(ad-bc)²/(a+b)(c+d)(a+c)(b+d)]$ Note: notice that the four components of the denominator are the four totals from the table columns and rows. Suppose you conducted a drug trial on a group of animals and you hypothesized that the animals receiving the drug would show increased heart rates compared to those that did not receive the drug. You conduct the study and collect the following data: - Ho: The proportion of animals whose heart rate increased is independent of drug treatment. - Ha: The proportion of animals whose heart rate increased is associated with drug treatment. **Table 2. Hypothetical drug trial results.** | | Heart Rate Increased | No Heart Rate Increase | Total | | ------------- |:-------------:|:-------------:|:-------------:| | Treated | 36 | 14 | 50 | | Not treated | 30 | 25 | 55 | | Total | 66 | 39 | 105 | Applying the formula above we get: _χ²_ = 105 [ (36)(25) - (14)(30) ]² / (50)(55)(39)(66) = 3.418 Before we can proceed we need to know how many degrees of freedom we have. When a comparison is made between one sample and another, a simple rule is that the degrees of freedom equal (number of columns minus one) x (number of rows minus one) not counting the totals for rows or columns. For our data this gives (2-1) x (2-1) = 1. We now have our chi square statistic (_χ²_ = 3.418), our predetermined alpha level of significance (0.05), and our degrees of freedom (df = 1). Entering the Chi square distribution table with 1 degree of freedom and reading along the row we find our value of _χ²_ (3.418) lies between 2.706 and 3.841. The corresponding probability is between the 0.10 and 0.05 probability levels. That means that the p-value is above 0.05 (it is actually 0.065). Since a p-value of 0.65 is greater than the conventionally accepted significance level of 0.05 (i.e., p>0.05) we fail to reject the null hypothesis. In other words, there is no statistically significant difference in the proportion of animals whose heart rate increased. What would happen if the number of control animals whose heart rate increased dropped to 29 instead of 30 and, consequently, the number of controls whose hear rate did not increase changed from 25 to 26? Try it. Notice that the new _χ²_ value is 4.125 and this value exceeds the table value of 3.841 (at 1 degree of freedom and an alpha level of 0.05). This means that p < 0.05 (it is now0.04) and we reject the null hypothesis in favor of the alternative hypothesis - the heart rate of animals is different between the treatment groups. When p < 0.05 we generally refer to this as a significant difference. **Table 3: Chi Square distribution table** | Df | 0.5 | 0.10 | 0.05 | 0.02 | 0.01 | 0.001 | | ------------- |:-------------:|:-------------:|:-------------:|:-------------:|:-------------:|:-------------:| | 1 | 0.455 |2.706 | 3.841 | 5.412 | 6.635 | 10.827 | | 2 | 1.386 | 4.605 | 5.991 | 7.824 | 9.210 | 13.815 | | 3 | 2.366 | 6.251 | 7.815 | 9.837 | 11.345 | 16.268 | | 4 | 3.357 | 7.779 | 9.488 | 11.668 | 13.277 | 18.465 | | 5 | 4.351 | 9.236 | 11.070| 13.388 | 15.086 | 20.517 | To make the chi square calculations a bit easier, plug you're observed and expected values into the following applet. Click on the cell and then enter the value. Click the compute button on the lower right corner to see the chi square value printed in the lower left hand corner. **Chi Square Goodness of Fit (One Sample Test)** This test allows us to compare a collection of categorical data with some theoretical expected distribution. This test is often used in genetics to compare the results of a cross with the theoretical distribution based on genetic theory. Suppose you performed a simpe monohybrid cross between two individuals that were heterozygous for the trait of interest. **Aa x Aa** The results of your cross are shown in Table 4. **Table 4. Results of a monohybrid cross between two heterozygotes for the 'a' gene** | | | | | -------- | -------- | -------- | | | A | a | | A | 10 | 42 | | a | 33 | 15 | | Totals | 43 | 57 | The phenotypic ratio 85 of the "A" type and 15 of the a-type (homozygous recessive). In a monohybrid cross between two heterozygotes, however, we would have predicted a 3:1 ratio of phenotypes. In other words, we would have expected to get 75 A-type and 25 a-type. Are our results different? $x² = ∑ (observed- expected)²/ expected$ Calculate the chi square statistic _χ²_ by completing the following steps: 1. For each observed number in the table subtract the corresponding expected number (O — Е). 2. Square the difference [ (O-E)2 ]. 3. Divide the squares obtained for each cell in the table by the expected number for that cell [ (O-E)²/ E]. 4. Sum all the values for (O - E)² / E. This is the chi square statistic. For our example, the calculation would be: _χ²_ = 5.33 | | Observed | Expected | (O — Е) | (O-E)2 | (O-E)2/ E | | -------- | -------- | -------- | -------- | -------- | -------- | | A-type | 85 | 75 | 10 | 100 | 1.33 | | a-type | 15 | 25 | 10 | 100 | 4.0 | Total | 100 | 100 | 5.33 | We now have our chi square statistic (_χ²_ = 5.33), our predetermined alpha level of significance (0.05), and our degrees of freedom (df =1). Entering the Chi square distribution table with 1 degree of freedom and reading along the row we find our value of _χ²_ (5.33) lies between 3.841 and 5.412. The corresponding probability is 0.05<P<0.02. This is smaller than the conventionally accepted significance level of 0.05 or 5%, so the null hypothesis that the two distributions are the same is rejected. In other words, when the computed _χ²_ statistic exceeds the critical value in the table for a 0.05 probability level, then we can reject the null hypothesis of equal distributions. Since our _χ²_statistic (5.33) exceeded the critical value for 0.05 probability level (3.841) we can reject the null hypothesis that the observed values of our cross are the same as the theoretical distribution of a 3:1 ratio. **Table 3: Chi Square distribution table.** | Df | 0.5 | 0.10 | 0.05 | 0.02 | 0.01 | 0.001 | | ------------- |:-------------:|:-------------:|:-------------:|:-------------:|:-------------:|:-------------:| | 1 | 0.455 |2.706 | 3.841 | 5.412 | 6.635 | 10.827 | | 2 | 1.386 | 4.605 | 5.991 | 7.824 | 9.210 | 13.815 | | 3 | 2.366 | 6.251 | 7.815 | 9.837 | 11.345 | 16.268 | | 4 | 3.357 | 7.779 | 9.488 | 11.668 | 13.277 | 18.465 | | 5 | 4.351 | 9.236 | 11.070| 13.388 | 15.086 | 20.517 | To put this into context, it means that we do not have a 3:1 ratio of A_ to aa offspring. To make the chi square calculations a bit easier, plug your observed and expected values into the following java applet. Click on the cell and then enter the value. Click the compute button on the lower right corner to see the chi square value printed in the lower left hand coner. **Chi Square Test of Independence** For a contingency table that has r rows and c columns, the chi square test can be thought of as a test of independence. In a test of independence the null and alternative hypotheses are: - **Ho**: The two categorical variables are independent. - **Ha**: The two categorical variables are related. We can use the equation **Chi Square = the sum of all the (fo - fe)² / fe** Here fo denotes the frequency of the observed data and fe is the frequency of the expected values. The general table would look something like the one below: | | Category I | Category II | Category III | Row Totals | | -------- | -------- | -------- | -------- | -------- | | Sample A | a | b | c | a+b+c | | Sample B | d | e | F | d+e+f | | Sample C | g | h | I | g+h+i | | Column Totals | a+d+g | b+e+h | c+f+i | a+b+c+d+e+f+g+h+i=N | Now we need to calculate the expected values for each cell in the table and we can do that using the the row total times the column total divided by the grand total (N). For example, for cell a the expected value would be (a+b+c)(a+d+g)/N. Once the expected values have been calculated for each cell, we can use the same procedure are before for a simple 2 x 2 table. | | Observed | Expected | IO — EI | (O — E)2 | (O-E)2/ E | | -------- | -------- | -------- | -------- | -------- | -------- | Suppose you have the following categorical data set. **Table. Incidence of three types of malaria in three tropical regions.** | | Asia | Africa | South America | Totals | | -------- | -------- | -------- | -------- | -------- | | Malaria A| 31 | 14 |