Overview of Secondary Data Research 2024 PDF
Document Details
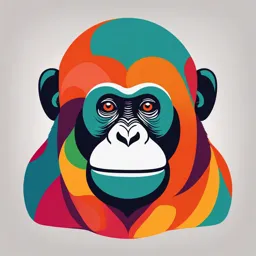
Uploaded by CheapestHammeredDulcimer
Universitas Gadjah Mada
2024
Bayu Satria Wiratama
Tags
Summary
This document provides an overview of secondary data research, including its definition, types, advantages, and challenges. It also discusses the use of secondary data in various fields of study, along with practical examples and considerations.
Full Transcript
Overview of Secondary Data Research Bayu Satria Wiratama, PhD Definition Research using data has already been collected for other purpose It can be collected by the same team Analyzing data provided by other sources Government (Riskesdas, SDKI, BPJS, BPS)...
Overview of Secondary Data Research Bayu Satria Wiratama, PhD Definition Research using data has already been collected for other purpose It can be collected by the same team Analyzing data provided by other sources Government (Riskesdas, SDKI, BPJS, BPS) NGO (IFLS) Universities (HDSS) Literature review Hospital (medical record) Most of them is free except for medical record, HDSS and Riskesdas Type of secondary data Hospital based (medical record) Survey based (Riskesdas, SDKI, IFLS, HDSS) Administrative data (BPS, weather data) Social media data (twitter, facebook) Other country data (UK crash dataset, IFLS for other country) Example of available secondary dataset in Indonesia Indonesia family life survey or IFLS (5 waves) Since 1993, every 5 years Longitudinal survey, same households every wave Indonesia demographic and health survey or IDHS or SDKI Every 5 years since 1997 Repeated cross sectional, different households Basic Health Research or Riskesdas 2007,2010,2013,2018 Repeated cross sectional BPJS dataset 2015-2021 Every years Longitudinal for 1% sample from all BPJS participants Example of available dataset outside Indonesia USA CDC BRFSS (Behavioral risk factor surveillance system) CDC YRBSS (Youth risk behavior surveillance system) FARS (Fatal accident reporting system) and others World bank World bank open data STATS-19 UK Accident database from 1979-now Advantages of secondary data research Cost savings, Time efficiency, Access to large, Complex datasets, To explore new research questions or test hypotheses. Challenge of secondary data research Data quality Quality and bias cannot be modified Data availability Not all of variables is available in each dataset Data compatibility Different sources, different format, different way of handling Data bias May introduce new bias because of new hypothesis Data ethics Ethics on using secondary data no name or identifier in the dataset Data processing Need to have more than basic skill of data management Data documentation Several secondary data have limited information about the dataset Data preparation (overview) Combining data from different sources Example: data from vehicle dataset combined with casualty dataset and accident dataset Data from individual dataset combined with household dataset with community dataset Missing data management Missing at random? Missing not at random? Imputation? Data analysis (overview) Similar with primary data analysis Chi square, t-test, regression, multilevel/panel data To improve novelty, we need to use complex data analysis Such as interaction analysis, mediation, multilevel analysis Ethical consideration Need ethical clearance Usually no informed consent was needed Anonymus data (no name or identifier) Case study This retrospective study analyzed secondary data on UK traffic dataset using UK STATS19 crash data for 1990–2017. The UK STATS19 is a national traffic dataset which includes data for every crash resulting in personal injuries reported to the UK police within 30 days. Public holidays in UK Public holidays in UK Public holidays in UK Halloween in UK Incidents resulting in child pedestrian fatalities are the most frequent during spring and fall, with the highest number of child pedestrian deaths caused by motor vehicle crashes occurring during May and October. The current research used the U.K. STATS19 database, which contains the data of all road traffic accidents. Halloween in UK Halloween in UK From 17:00 to 16:00 (17:00~17:59), pediatric casualties involved in a crash on Halloween were 34.2% more likely to sustain KSIs than were those involved in a crash on a different day type (AOR = 1.342; 95% CI = 1.065–1.692). Case study: Interaction analysis using secondary database Why do we need to research for combined effect? New approach in methodology novelty Growing evidence that two risk factors could have interaction effect For example: unhelmeted and drunk riding, diabetes and hypertension Case study This study found an interaction effect between drunk and un- helmeted riding on motorcyclist fatalities Study data flowchart This study used National Taiwan crash dataset from 2011-2015 Data was combined using Excel Cases with missing data was removed and tested if it is Missing at random (MAR) or not Analysis was conducted in STATA The impact of unhelmeted and drunk riding Motorcyclists with a positive blood alcohol level were 9.47 times (AOR = 9.47; 95% CI = 8.75–10.25) more likely to sustain fatal injuries than those with a negative blood alcohol level. Unhelmeted motorcyclists had risk 2.1 (AOR=2.1; 95% CI = 1.9-2.2) times higher to sustain fatal injuries than those helmeted motorcyclists Interaction effect RERI = 18.1-10.1- 2.3+1 RERI = 6.7 RERI > 0 There is a positive interaction between drunk and un- helmeted riding Conclusion Secondary data analysis provide alternative approach for research Sometimes it cheaper, easier but not all of the time Need complex data management skill Provide an opportunity to analyse a set of data with different approach There is several free dataset available for students